Personalization Engines in Action
The revelation struck Arun during a routine analysis of their e-commerce performance metrics. Despite substantial traffic to the product pages, conversion rates remained stubbornly low. Seeking answers, he conducted a simple experiment by creating dummy accounts with different browsing histories to experience the site as various customer types would. The results were startling: regardless of past behavior, every visitor saw identical homepage features, product recommendations, and promotional messaging. Their one-size-fits-all approach was effectively speaking to no one. That evening, Arun drafted the framework for what would become their personalization initiative—reimagining their digital experience not as a single website but as thousands of individualized experiences dynamically assembled for each visitor. Within four months of implementation, conversion rates increased by 63%, average order value grew by 31%, and customer satisfaction scores rose by 47%. This transformation fundamentally changed Arun's understanding of digital experiences—not as static destinations, but as responsive environments that adapt to each visitor's unique context and needs. This realization launched his exploration into personalization technology, revealing how dynamic content adaptation has become perhaps the most powerful driver of digital performance.
Introduction: The Relevance Revolution in Digital Experiences
Digital experience personalization has evolved from basic segmentation to sophisticated individual-level adaptation that transforms static content into responsive environments. This evolution has progressed through several distinct phases: from broad demographic targeting to behavioral segmentation, from rules-based adaptation to algorithmic personalization, and now to the frontier of predictive personalization that anticipates individual needs and preferences before they're explicitly expressed.
The implementation of advanced personalization represents what the MIT Technology Review has identified as "a fundamental shift from broadcast thinking to individual conversation" in digital strategy. In high-performing organizations, personalization now serves not just as a conversion tactic but as the foundation of the entire customer experience strategy.
Research from Gartner indicates that organizations with sophisticated personalization capabilities achieve 67% higher conversion rates and 56% greater customer lifetime value compared to those using basic personalization approaches. Meanwhile, a study published in the Journal of Interactive Marketing found that properly implemented personalization generates 4.2x higher engagement rates and 3.7x greater revenue per session than non-personalized experiences.
1. On-Site and In-App Customizations: Creating Responsive Environments
Modern personalization creates individualized digital experiences:
a) Experience Adaptation Frameworks
Sophisticated systems modify entire user experiences:
- Layout optimization for individual behaviors
- Navigation prioritization based on usage patterns
- Functionality exposure aligned with user proficiency
- Content density calibration to cognitive preferences
Example: E-commerce retailer ASOS implemented an AI-driven experience adaptation system that automatically adjusts their mobile app interface based on individual usage patterns. For visually-driven browsers, the system emphasizes image galleries and visual search, while for data-driven shoppers, it prioritizes detailed specifications and comparison features. This approach increased session depth by 34% and conversion rates by 28% by aligning the experience with each user's natural shopping style.
b) Content Relevance Optimization
Personalization engines prioritize high-relevance content:
- Individual interest affinity models
- Content consumption pattern analysis
- Topic relevance prediction algorithms
- Engagement likelihood forecasting
Example: Media company Condé Nast developed a personalization system that analyzes reading patterns across their publications to identify content affinity signals beyond explicit topic interests. This system revealed that format preferences (long-form vs. quick reads) and consumption context (weekday vs. weekend) were stronger predictors of engagement than declared interests, resulting in a 43% increase in article completion rates through smarter content selection.
c) Next-Best Experience Orchestration
Advanced systems anticipate optimal next steps:
- Journey stage identification algorithms
- Intent prediction frameworks
- Next-action recommendation engines
- Conversion path optimization
Example: Banking institution Capital One implemented a next-best-action system that identifies the optimal experience path for individual customers based on their financial situation, recent interactions, and inferred objectives. This capability increased digital self-service completion rates by 41% by guiding customers through personalized pathways aligned with their specific needs rather than generic conversion funnels.
2. Email Dynamic Content: Creating Individualized Communications
Modern email personalization creates unique messages for each recipient:
a) Intelligent Content Selection
Advanced systems optimize message composition:
- Individual relevance scoring models
- Multi-variant element selection algorithms
- Engagement probability forecasting
- Optimal content combination identification
Example: Travel platform Booking.com developed an email personalization engine that dynamically assembles each message from a content library containing over 500,000 destination descriptions, images, and offers. This system analyzes individual search history, booking patterns, and engagement data to create uniquely relevant communications, resulting in a 49% increase in email-generated booking revenue compared to their previous segmentation approach.
b) Behavioral Trigger Enhancement
Sophisticated triggers create timely, contextual relevance:
- Behavioral pattern recognition
- Engagement opportunity identification
- Perfect timing optimization
- Context-appropriate message selection
Example: Sporting goods retailer REI implemented an advanced trigger system that identifies optimal messaging moments based not just on direct behaviors but on contextual factors such as local weather conditions, proximity to recreational areas, and seasonal activity patterns. This approach increased trigger email conversion rates by 57% by reaching customers at moments of highest relevance and receptivity.
c) Dynamic Offer Personalization
Intelligent systems optimize promotional elements:
- Individual price sensitivity modeling
- Incentive preference identification
- Offer magnitude optimization
- Promotion format effectiveness prediction
Example: Food delivery service Grubhub created a dynamic incentive system that analyzes individual order history, price sensitivity, and competitive exposure to determine optimal offer structures for each customer. This capability revealed that while some customers respond best to percentage discounts, others are more motivated by free delivery or minimum order reductions. This personalized approach increased redemption rates by 38% while decreasing promotional costs by 27%.
3. Personalization Rules: Creating Intelligent Adaptation Logic
Strategic rule development drives personalization effectiveness:
a) Multi-Dimensional Rule Frameworks
Sophisticated approaches combine multiple signals:
- Behavioral recency-frequency-monetary value weighting
- Contextual relevance factor incorporation
- Interest affinity signal integration
- Engagement pattern analysis
Example: Retailer Target developed a personalization framework that combines over 50 data signals including purchase history, browsing behavior, life stage indicators, and even weather conditions to create what their chief digital officer calls "contextual relevance scores." This approach increased product recommendation click-through rates by 61% by presenting offers at the intersection of individual preference and situational context.
b) Progressive Profiling Methodologies
Advanced systems build understanding over time:
- Incremental knowledge acquisition strategies
- Confidence threshold modeling
- Assumption validation techniques
- Profile enhancement prioritization
Example: Software company Adobe implemented a progressive profiling system in their digital experience platform that begins with broad behavioral clustering but refines its understanding with each interaction. This approach allowed them to achieve personalization effectiveness with new visitors equal to 73% of known customer personalization within just three sessions by rapidly building relevant profiles through observed behavior.
c) Testing and Optimization Architectures
Intelligent systems continuously improve through experimentation:
- Multi-armed bandit testing implementations
- Self-optimizing rule adjustments
- Performance-based algorithm selection
- Continuous hypothesis testing
Example: Streaming service Netflix developed a personalization system that automatically generates and tests thousands of algorithmic variations to identify optimal recommendation approaches for different user types. This capability continuously improves performance without manual intervention, resulting in a 37% increase in content discovery and a 29% reduction in browsing time before content selection compared to their previous optimization approach.
Conclusion: The Relevance Imperative
As noted by personalization researcher and former Amazon executive Brent Franson, "The most effective personalization doesn't feel like marketing technology—it feels like mind reading, as if the experience knows exactly what you want before you do." For digital leaders, this insight suggests that personalization should be evaluated not just on its technical sophistication but on its ability to create experiences that feel naturally intuitive to each individual user.
The implementation of advanced personalization represents more than technical evolution—it requires a fundamental transformation in how organizations conceive of digital experiences. Those who master this discipline create significant competitive advantage through deeper customer understanding, more relevant engagement, and more efficient conversion optimization.
As these technologies continue to evolve, successful organizations will be those that maintain a balance between algorithmic intelligence and human empathy, creating systems that enhance rather than manipulate the customer experience.
Call to Action
For digital leaders seeking to implement effective personalization:
- Conduct a cross-channel audit of current personalization capabilities and gaps
- Develop a unified customer data strategy that enables consistent personalization
- Create ethical guidelines that balance personalization effectiveness with privacy respect
- Build cross-functional teams that blend marketing, design, and data science expertise
- Implement measurement frameworks that evaluate personalization impact on both conversion and customer satisfaction
The future of digital effectiveness belongs not to those with the most advanced technology or the largest data assets, but to those who create the most naturally relevant experiences that anticipate and fulfill customer needs in the moment they arise.
Featured Blogs
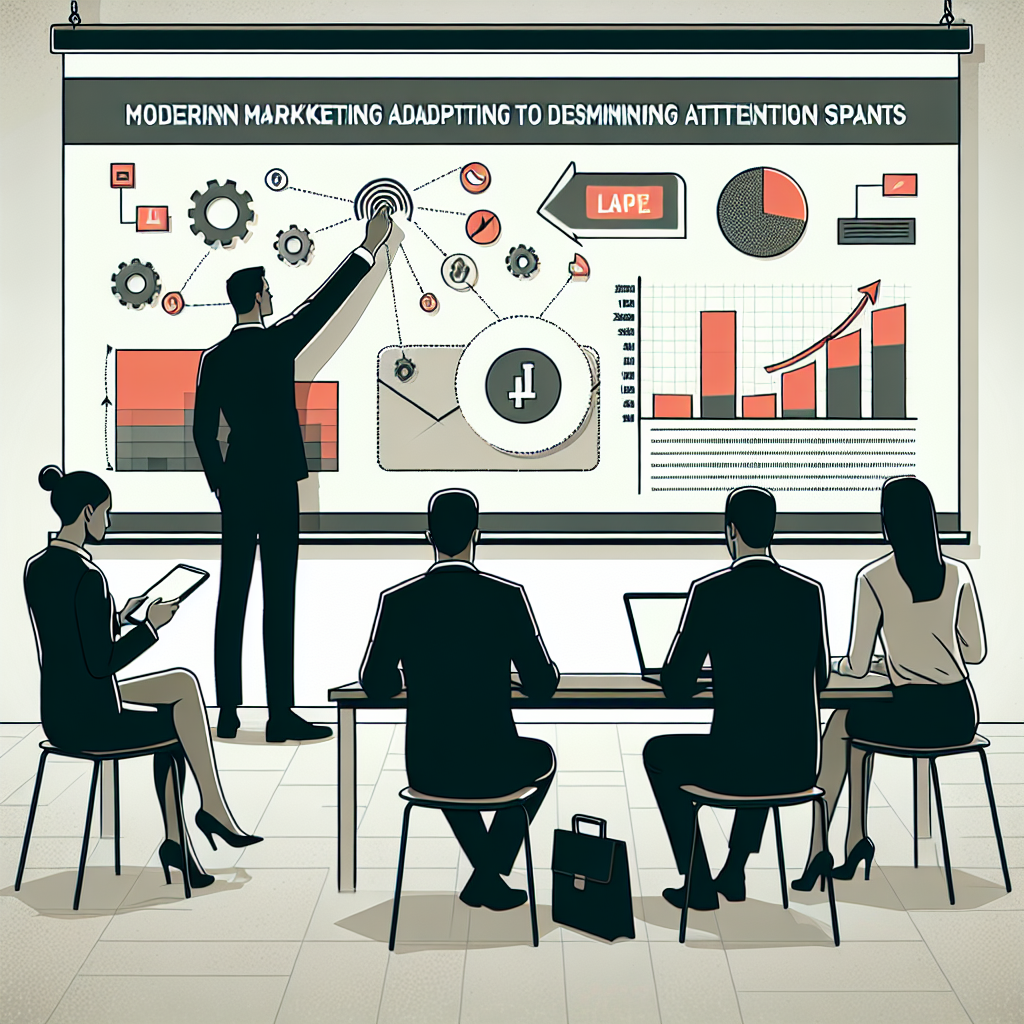
How the Attention Recession Is Changing Marketing
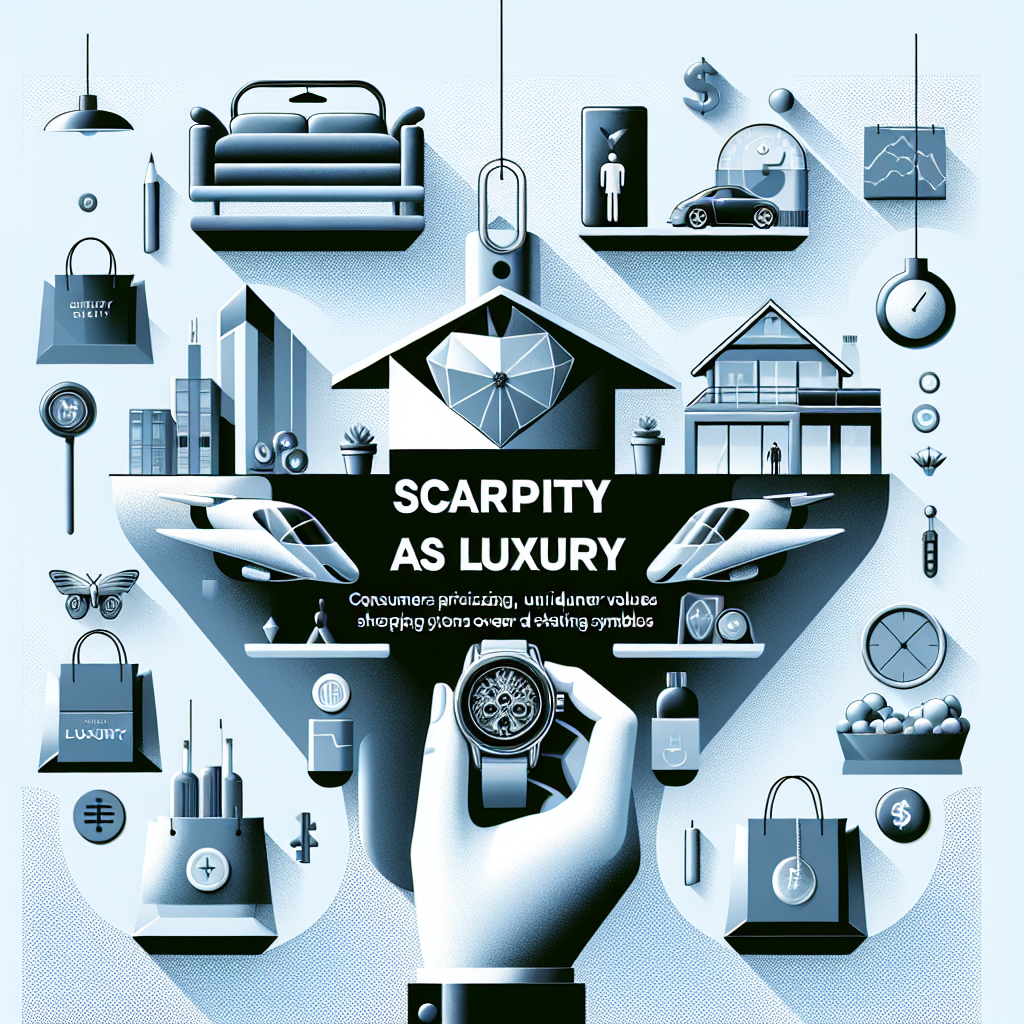
The New Luxury Why Consumers Now Value Scarcity Over Status
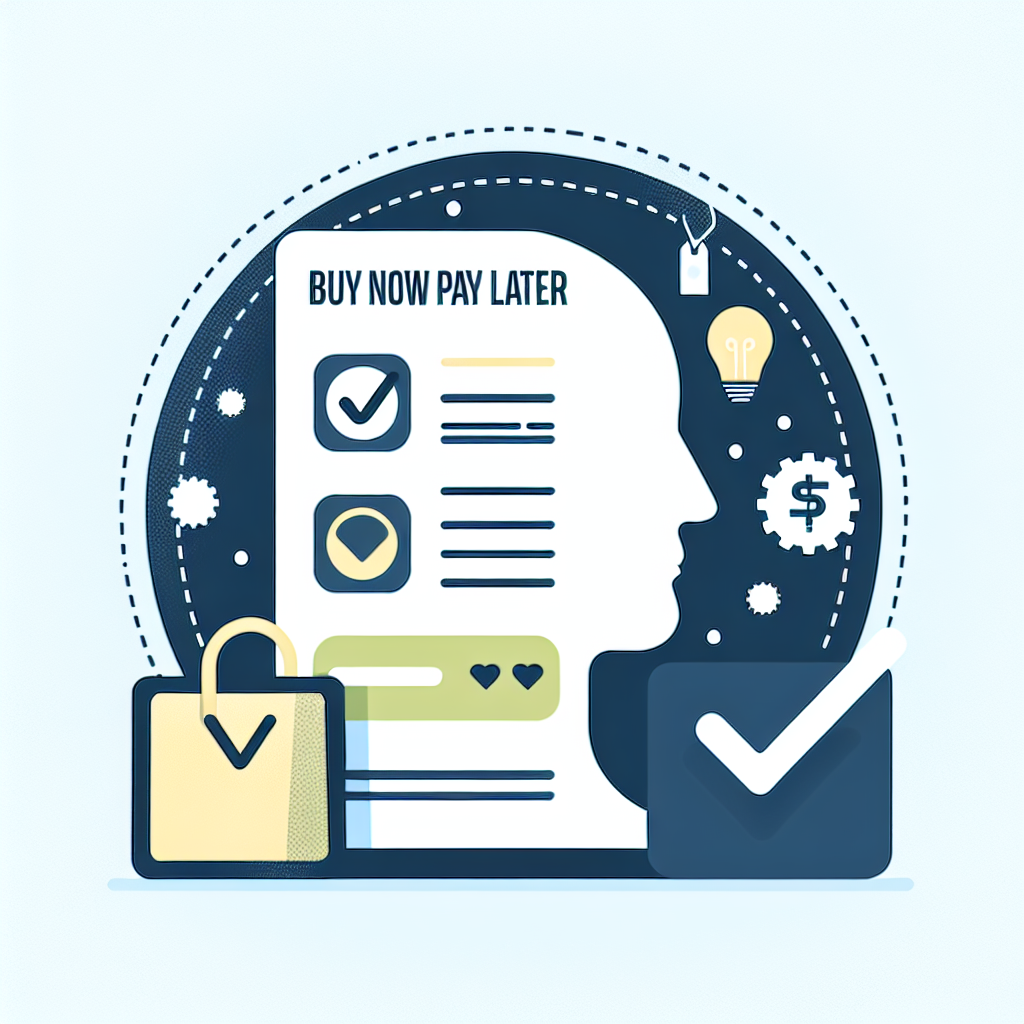
The Psychology Behind Buy Now Pay later
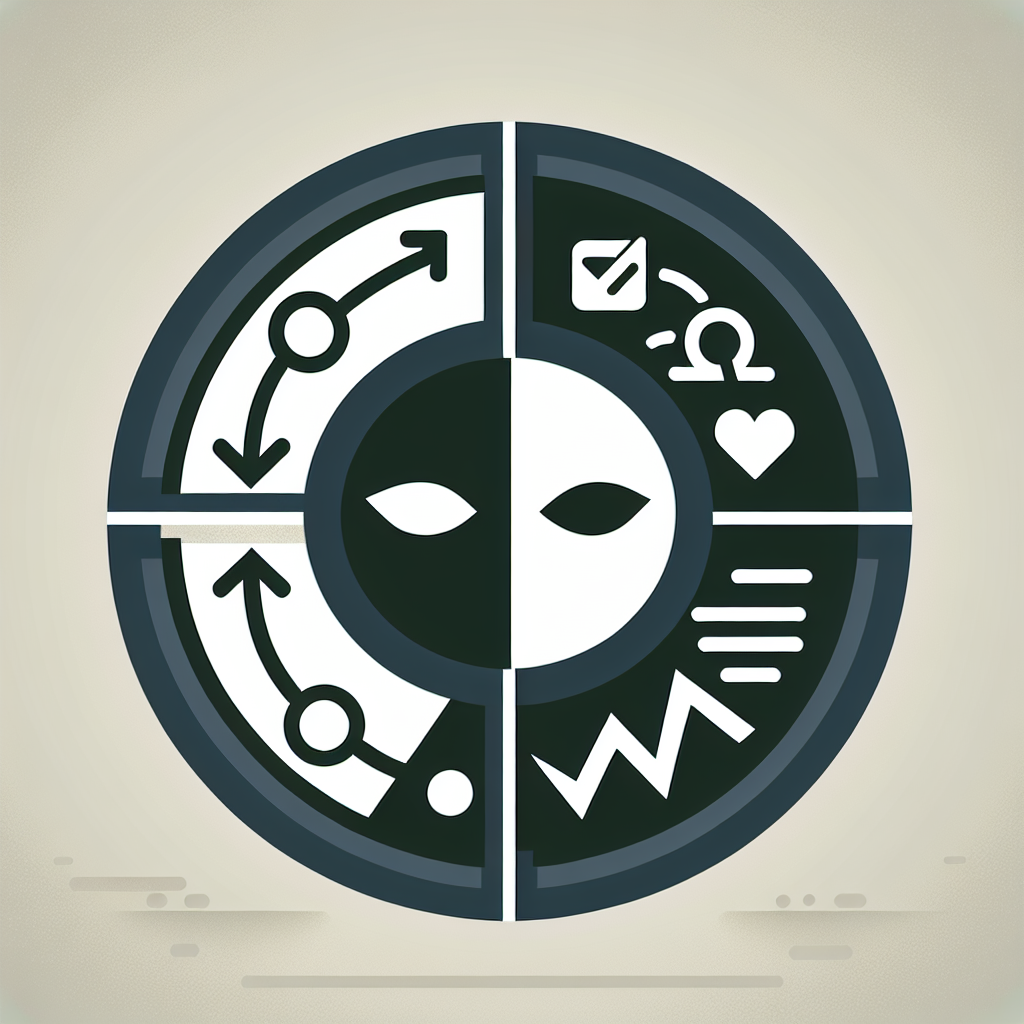
The Role of Dark Patterns in Digital Marketing and Ethical Concerns
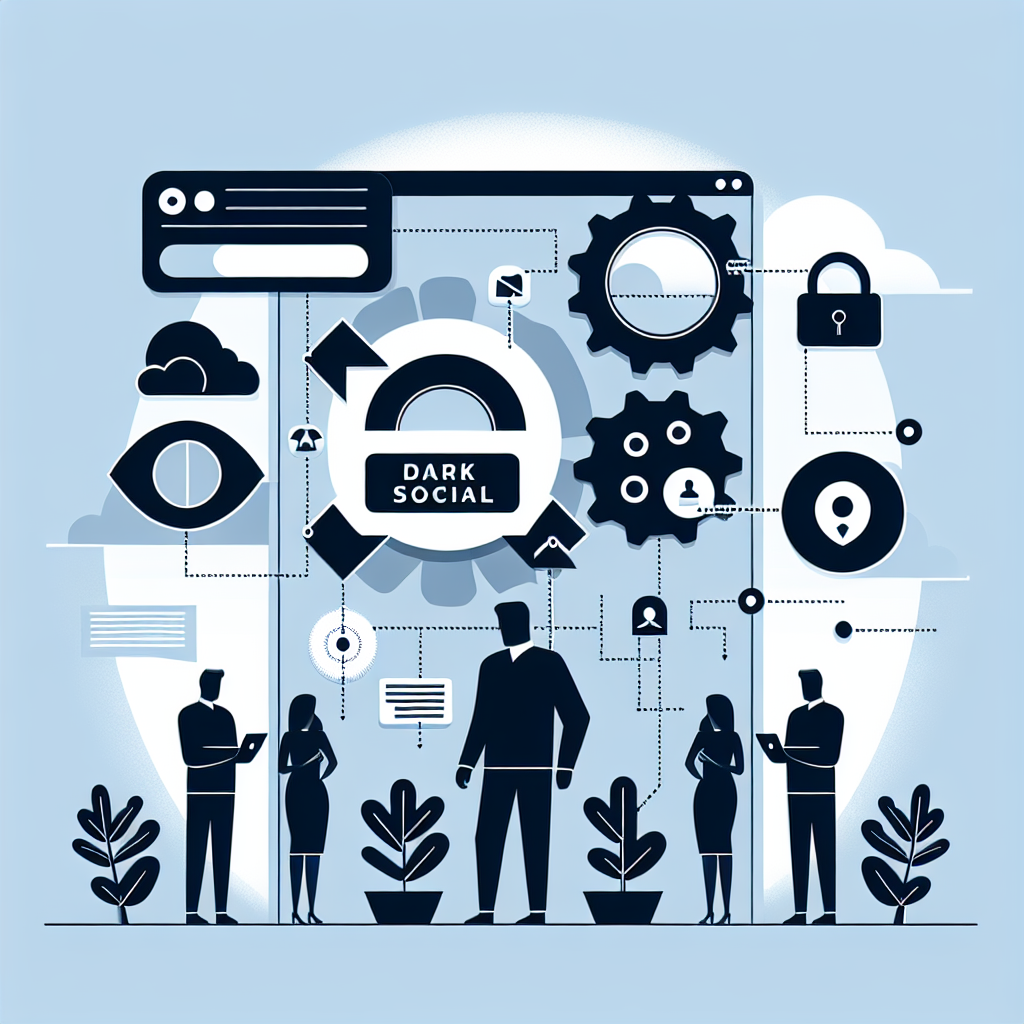
The Rise of Dark Social and Its Impact on Marketing Measurement
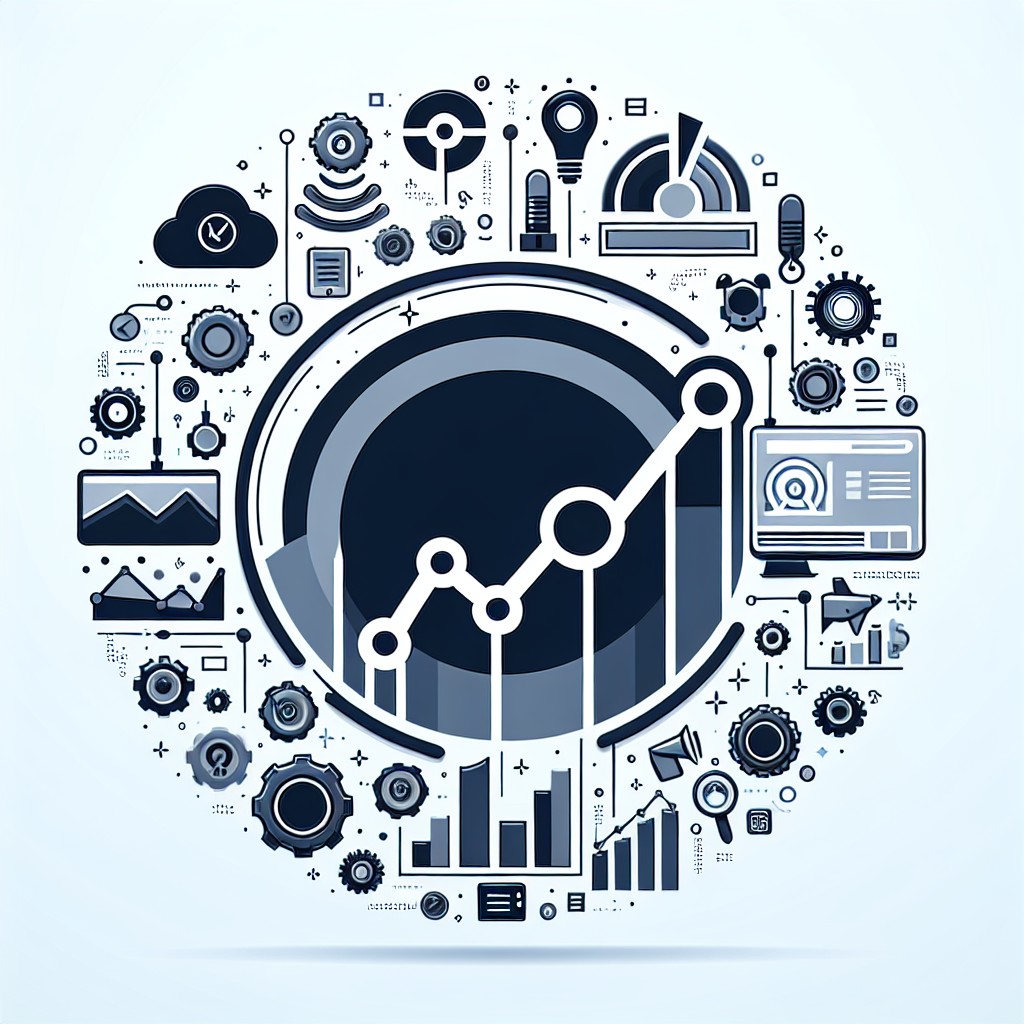