AI in Marketing Research: What's Real, What's Hype?
Neeraj was grabbing coffee with his former colleague Alex last week when their conversation turned to Alex's recent marketing research project. Tasked with evaluating AI tools for his department, Alex's frustration was evident. "Everyone keeps throwing around AI capabilities like they're magic," he sighed, "but when we implemented three different platforms, none delivered what vendors promised." Neeraj found Alex's experience deeply resonant, having observed numerous marketing teams pursuing AI solutions without a clear understanding of their actual capabilities and limitations. That afternoon conversation crystallized for Neeraj the urgent need for marketers to differentiate between AI reality and the pervasive hype dominating industry discussions.
Introduction: The AI Promise in Marketing Research
Marketing research has undergone profound transformation as artificial intelligence technologies have matured from experimental concepts to operational tools. According to the Marketing Research Society, 78% of enterprise-level organizations now incorporate some form of AI in their research methodologies, yet only 23% report achieving their expected ROI from these investments. This disconnect stems largely from misaligned expectations about what current AI technologies can realistically accomplish in research contexts.
As marketing research continues its technological evolution, professionals must develop nuanced understanding of AI's actual capabilities, appropriate applications, and ethical boundaries. This examination seeks to clarify where AI truly delivers value in marketing research and where traditional approaches remain superior.
1. Current Capabilities
The present state of AI in marketing research centers on four primary functional areas where the technology has demonstrated measurable value:
a) Natural Language Processing for Unstructured Data
Today's NLP algorithms can analyze vast quantities of textual data with remarkable efficacy:
- Sentiment analysis with contextual understanding across multiple languages
- Thematic categorization identifying emergent topics without predefined taxonomies
- Emotional intensity measurement beyond basic positive/negative classification
Consumer goods company Unilever deployed NLP analysis across 1.2 million customer service interactions, revealing previously undetected product performance issues that traditional research had missed. The system identified subtle language patterns indicating dissatisfaction before consumers themselves articulated specific complaints.
b) Computer Vision Applications
Visual AI analyzes images and video with increasing sophistication:
- Facial coding for emotional response measurement during advertising exposure
- In-store behavior tracking and purchase pattern identification
- Visual content effectiveness prediction based on historical engagement patterns
Retailer Target utilized computer vision analysis to evaluate customer navigation patterns in redesigned store layouts, identifying that specific visual merchandising elements created 23% higher engagement but 12% lower conversion—insights impossible to capture through traditional observational studies.
c) Predictive Analytics Implementation
Predictive capabilities represent AI's most mature research application:
- Purchase propensity modeling with multi-variable inputs
- Churn prediction based on behavioral indicators
- Price sensitivity forecasting with dynamic adjustment capabilities
Financial services provider American Express implemented AI-driven predictive models that identified customer attrition signals 14 months before actual churn—a 267% improvement over previous statistical methods—enabling preventative intervention strategies.
d) Research Process Automation
Operational efficiencies through automation deliver tangible benefits:
- Survey design optimization and question refinement
- Sampling bias identification and correction
- Initial data cleaning and preparation
Marketing agency Dentsu reported 41% reduction in research preparation time after implementing AI-assisted processes, allowing researchers to focus on strategic analysis rather than administrative tasks.
2. Generative vs. Predictive Uses
The distinction between generative and predictive AI applications represents a crucial consideration for marketing researchers:
a) Predictive Applications
Predictive AI excels at identifying patterns within existing data to forecast outcomes:
- Consumer behavior prediction based on historical patterns
- Market trend forecasting with probabilistic confidence intervals
- Advertising response modeling using multi-variable inputs
Telecommunications company Verizon successfully deployed predictive models to anticipate customer upgrade timing with 84% accuracy, allowing precisely timed marketing interventions.
b) Generative Applications
Generative AI creates new content or concepts based on training data:
- Survey question generation and refinement
- Hypothetical consumer persona development
- Initial creative concept ideation
However, generative applications often suffer from hallucination problems—creating plausible but factually inaccurate outputs that can mislead research efforts. Media company NBCUniversal abandoned an AI-generated audience segmentation project after discovering the system had created convincing but entirely fabricated customer profiles.
c) Hybrid Approaches
The most successful AI implementations combine generative ideation with human oversight:
- AI-suggested research hypotheses refined by human experts
- Machine-generated initial survey drafts with researcher editing
- Automated analysis with human interpretation and contextualization
E-commerce platform Shopify developed a hybrid approach where AI systems generate initial research designs that human researchers then modify, reporting 62% faster research initiation while maintaining quality standards.
3. Ethics and Future
The ethical implications of AI in marketing research demand careful consideration:
a) Transparency Challenges
Black-box algorithms create accountability issues:
- Decision processes that cannot be adequately explained to stakeholders
- Difficulty identifying sources of bias in automated systems
- Challenges in validating AI-generated insights
Major retailer Walmart established an "algorithmic transparency committee" requiring all research AI to provide decision path documentation, slowing implementation but significantly improving long-term reliability.
b) Privacy Considerations
Advanced capabilities raise serious privacy concerns:
- Increasingly invasive data collection possibilities
- Re-identification risks in anonymized datasets
- Consumer discomfort with perceived surveillance
Technology company IBM developed a privacy-preserving AI research platform that analyzes consumer data without raw information leaving secure environments, establishing a potential framework for ethical advancement.
c) Future Directions
Emerging developments suggest promising paths forward:
- Explainable AI systems prioritizing transparency over pure performance
- Federated learning allowing insights without centralizing sensitive data
- Human-AI collaborative frameworks balancing automation with expertise
Research firm Ipsos has pioneered a "human-in-the-loop" framework where AI handles initial data processing while human researchers maintain control over interpretation and recommendations, establishing a potential industry standard for responsible implementation.
Conclusion: Toward Practical AI Integration
The reality of AI in marketing research falls between the hype-driven extremes. While not the autonomous research miracle some vendors promise, AI tools deliver substantial value when applied with clear understanding of their capabilities and limitations. Success requires viewing AI as an enhancement to human expertise rather than a replacement—augmenting researchers' capabilities while maintaining critical thinking and contextual understanding.
Call to Action
For marketing research professionals navigating this complex landscape:
- Implement systematic evaluation frameworks for AI tools, focusing on measurable outcomes rather than technological novelty
- Develop comprehensive data governance policies before deploying advanced AI systems
- Invest in training programs that develop both technical AI literacy and critical evaluation skills
- Create cross-functional teams combining technical expertise with research methodology knowledge
- Establish ethical review processes for AI applications with potential consumer impact
The future belongs to organizations that harness AI's analytical power while maintaining human wisdom in their research practice—embracing technological advancement without sacrificing the human insight that gives research its ultimate value.
Featured Blogs
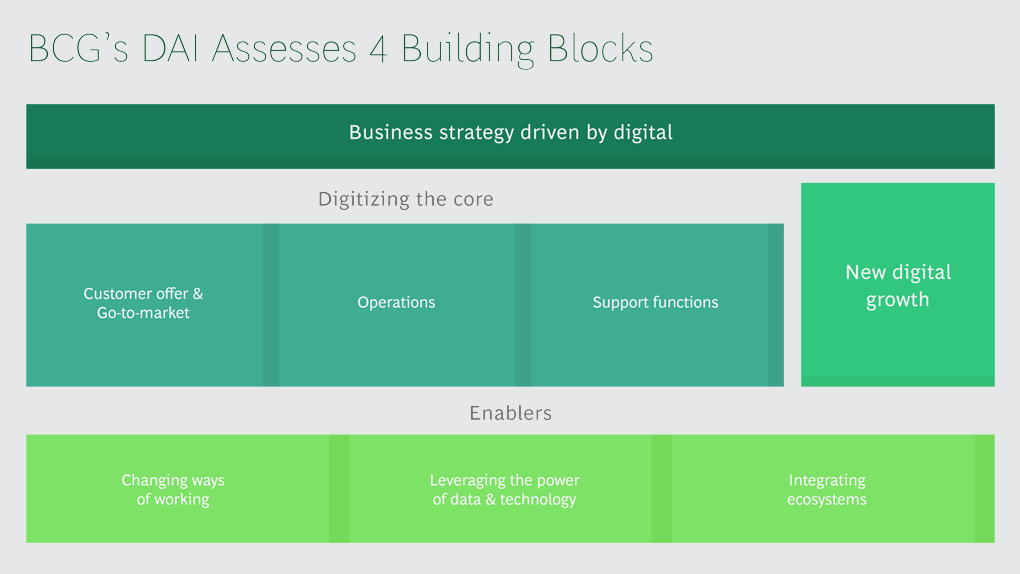
BCG Digital Acceleration Index
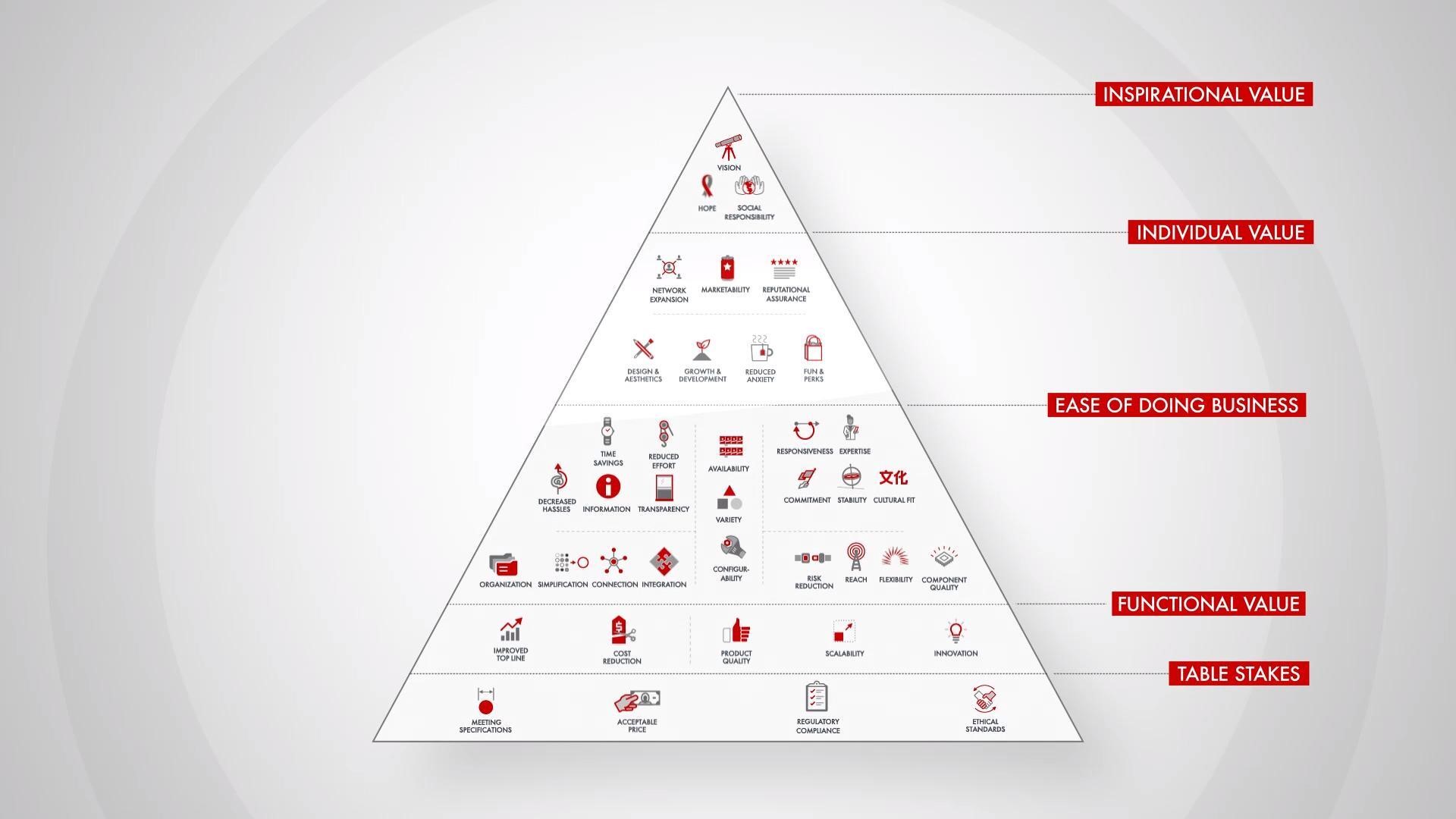
Bain’s Elements of Value Framework
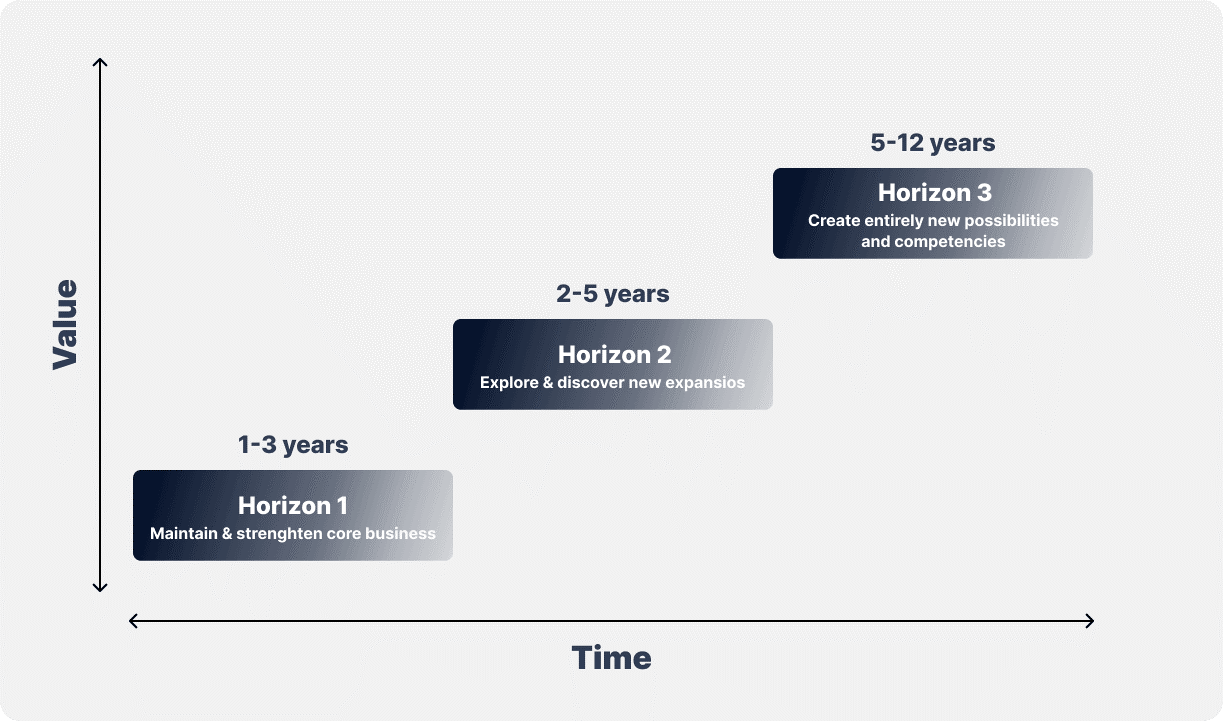
McKinsey Growth Pyramid
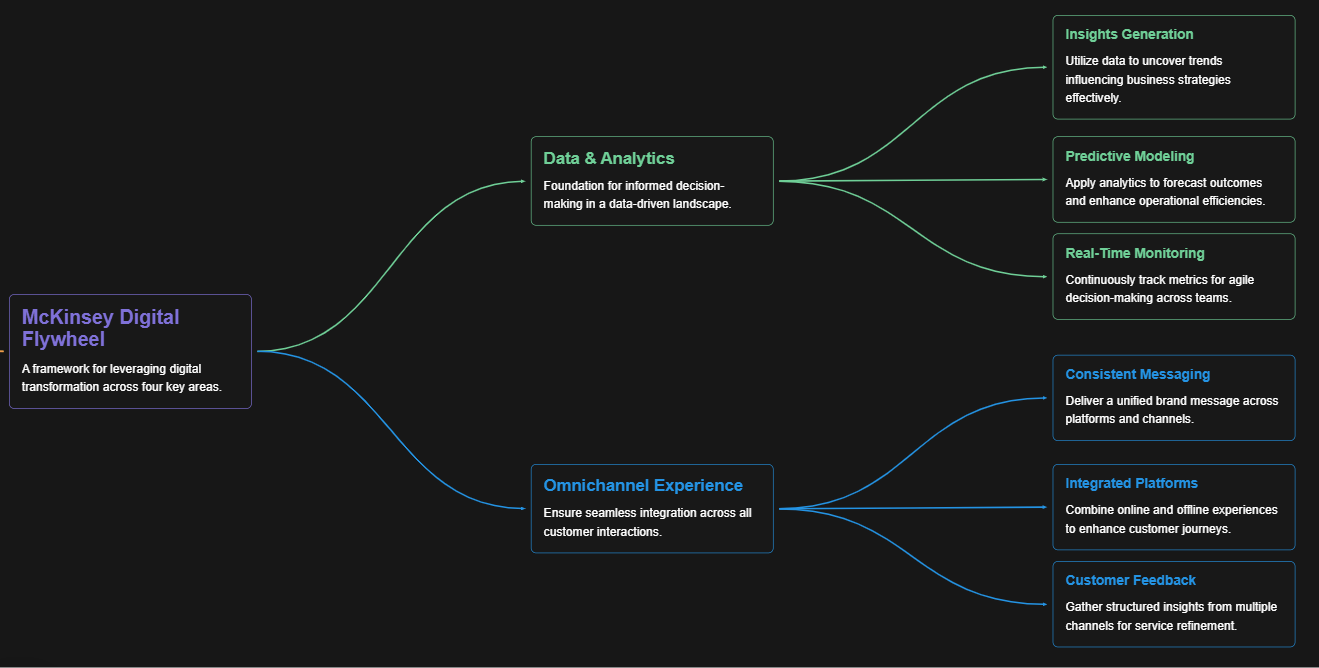
McKinsey Digital Flywheel
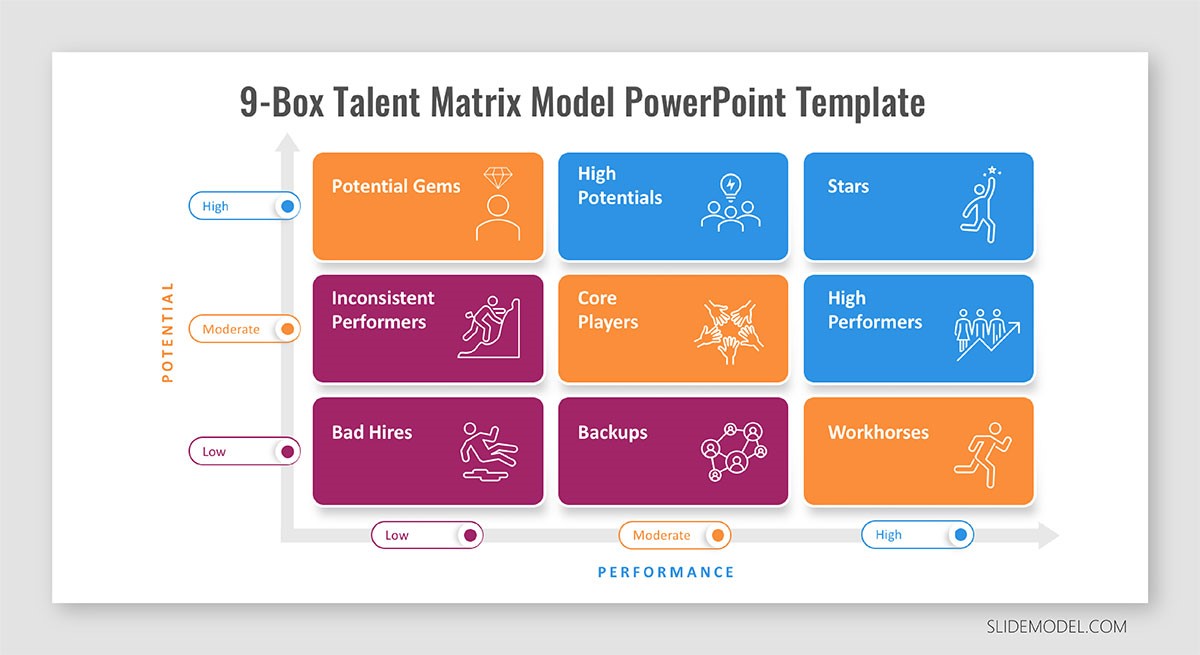
McKinsey 9-Box Talent Matrix
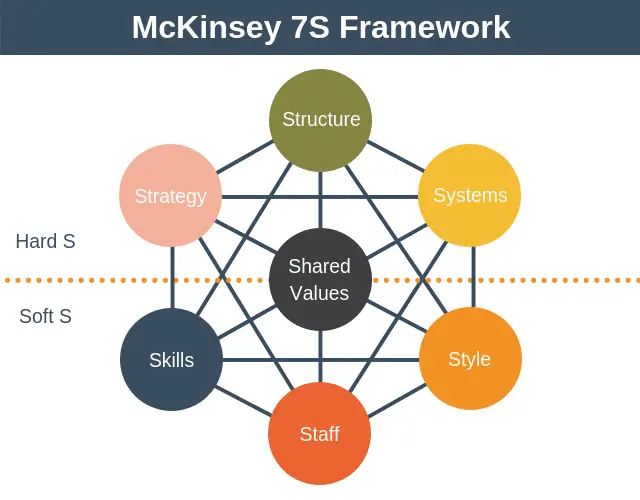
McKinsey 7S Framework
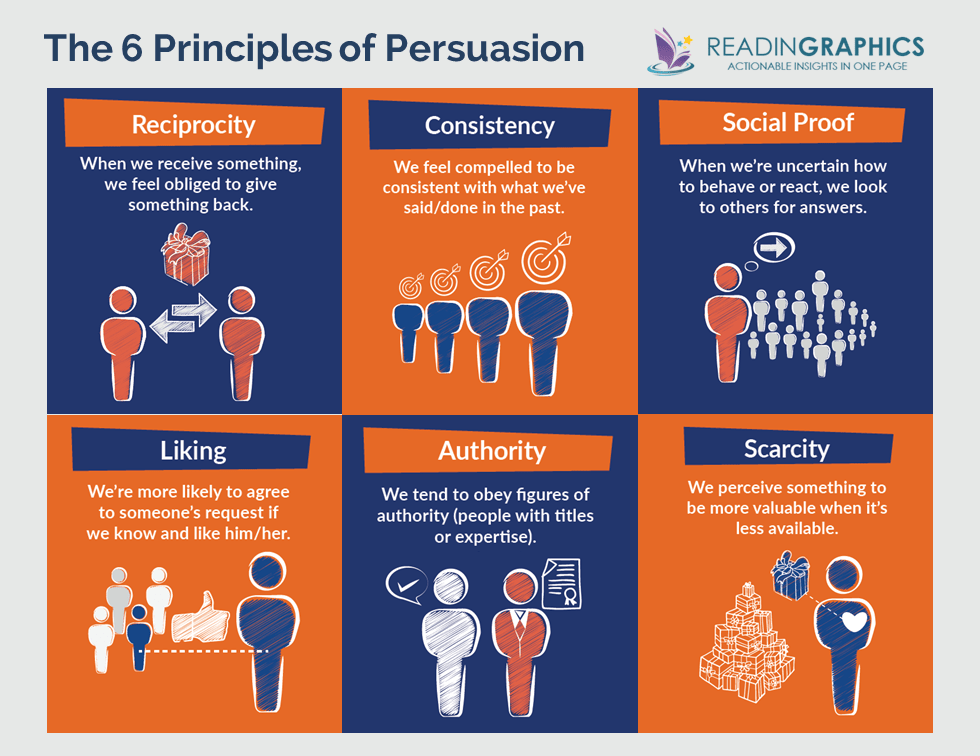
The Psychology of Persuasion in Marketing
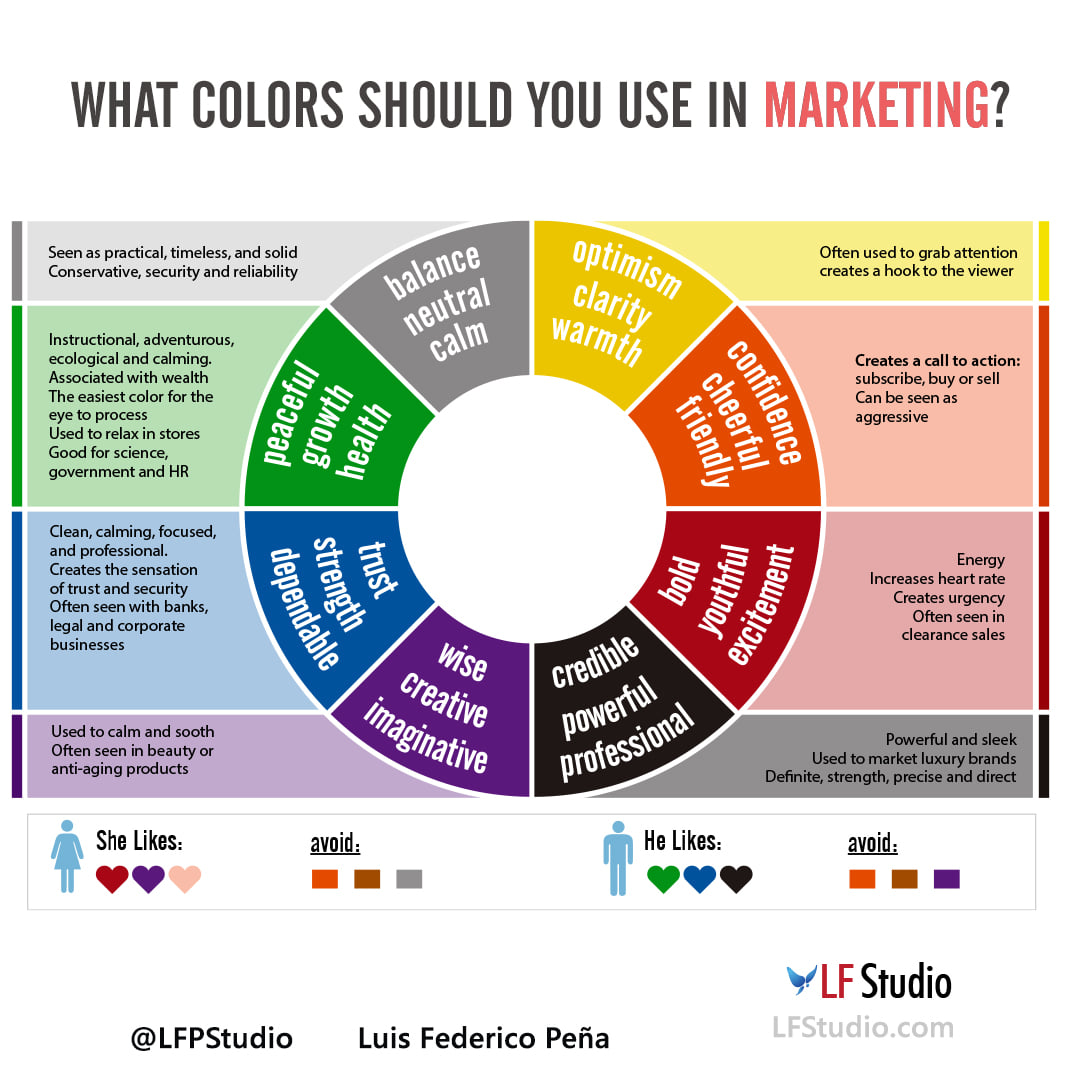
The Influence of Colors on Branding and Marketing Psychology
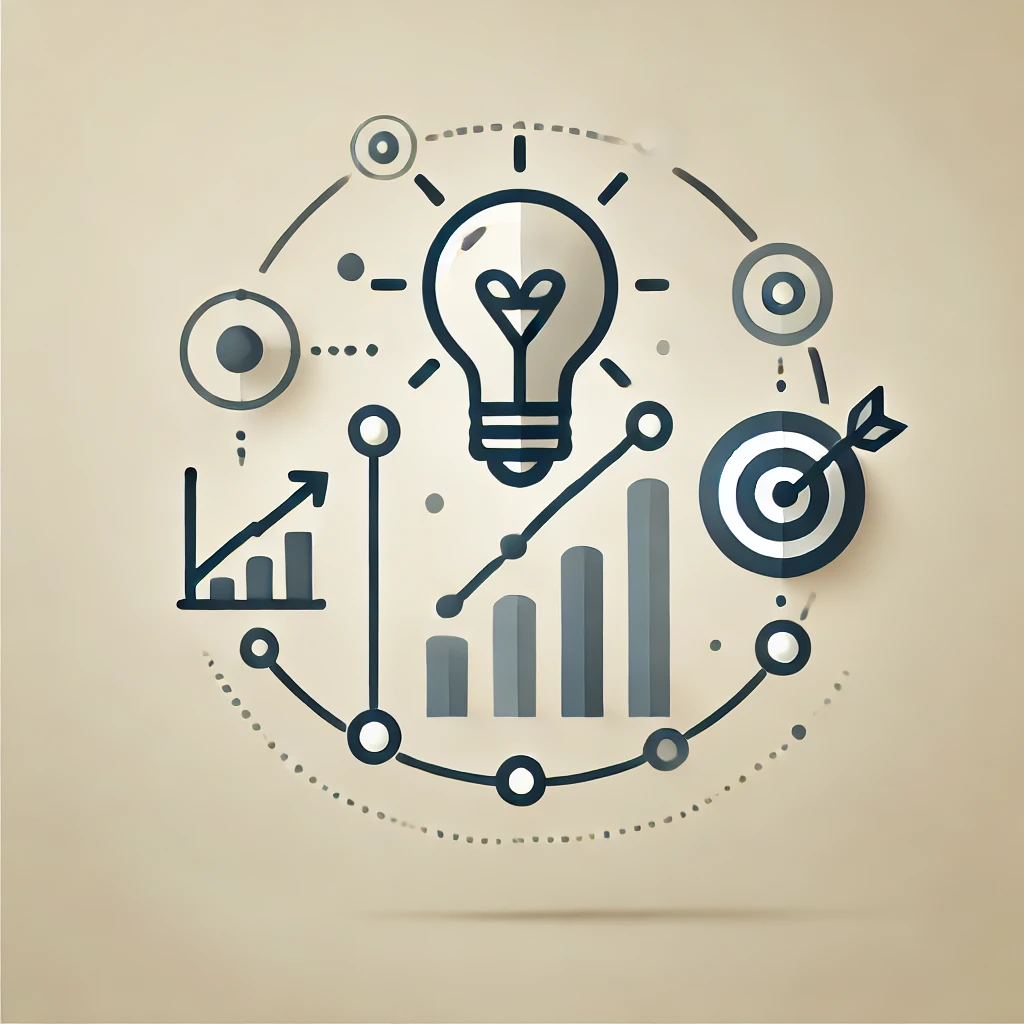