Choice Modelling in Consumer Research
The projector illuminated the last slide of the presentation as an uncomfortable silence settled over the room. Neeraj's colleague had just pitched their recommendation for the company's new product line, which was based on traditional consumer preference surveys. The Chief Product Officer leaned forward, skepticism evident in his voice. "I'm not convinced," he said. "Last quarter, customers told us they wanted eco-friendly packaging, but sales data shows nobody's paying the premium. How do we know this isn't the same disconnect?"
Feeling his pulse quicken, Neeraj opened his laptop. "May I show you something different?" he asked, pulling up the choice modeling study prepared as a backup. Unlike the survey data, this study demonstrated how consumers made actual trade-offs between features, price points, and sustainability options. "When forced to choose between competing priorities, here's what they actually select," Neeraj explained, revealing that sustainability was indeed important to consumers—but only at a 5% premium, rather than the 15% the company had implemented. Two months later, after adjusting their strategy based on the choice model, sales had increased by 23%. That day fundamentally changed how Neeraj approached consumer research, shifting from merely asking people what they want to closely observing how they make choices.
Introduction
Understanding consumer decision-making has evolved dramatically beyond simply asking consumers what they want. Choice modeling has emerged as a sophisticated methodology that replicates real-world decision contexts, forcing respondents to make trade-offs that reveal their genuine preferences. The difference is profound: while traditional research might indicate 70% of consumers value sustainability, choice modeling might reveal only 30% will choose sustainable options when faced with a 10% price premium. According to research from the Journal of Marketing, choice modeling demonstrates up to 3.7 times greater predictive accuracy than direct preference measurement techniques. As digital marketplaces multiply consumer options and complicate decision journeys, choice modeling provides the analytical framework necessary to understand how consumers navigate increasingly complex purchase decisions.
Stated vs. Revealed Preferences in Marketing Research
The distinction between what consumers say (stated preferences) and what they do (revealed preferences) represents one of marketing's most persistent challenges. Choice modeling bridges this gap by structuring research to more closely mirror actual marketplace decisions.
Stated preference methods often suffer from social desirability bias, hypothetical bias, and strategic response bias. When Unilever investigated environmentally friendly packaging, direct questioning showed 74% of consumers prioritized sustainability. However, their parallel choice modeling study revealed only 26% would select eco-friendly options when presented with realistic price differences, helping them avoid a costly misinvestment in premium sustainable packaging.
Revealed preference studies examine actual market behavior but face limitations in testing new concepts or isolating specific attributes. Delta Airlines combined credit card purchase data (revealed preferences) with choice modeling (stated preferences) to create what they termed "calibrated choice models," where stated preference data was mathematically adjusted based on observed behavior patterns. This hybrid approach improved their new route demand forecasting accuracy by 41%.
Best Buy developed an innovative application they called "shadow preference tracking," where in-store shoppers were invited to participate in choice modeling immediately after making purchases. This allowed direct comparison between their actual purchases and choice model predictions, creating continuous improvement in model accuracy and revealing systematic biases in how consumers projected their preferences.
Building Effective Choice Sets for Meaningful Insights
The design of choice sets—the specific options presented to research participants—determines the quality and usefulness of choice modeling results. This requires careful consideration of attributes, levels, and experimental design.
Attribute selection must balance comprehensiveness with cognitive manageability. When Amazon redesigned their Prime subscription offerings, they initially identified 17 potential attributes but used factor analysis to consolidate these into six distinct dimensions that consumers evaluated independently. This streamlined approach improved model fit by 27% compared to more complex alternatives.
Level selection critically impacts results, as ranges must be wide enough to observe preference thresholds but realistic enough to maintain engagement. Marriott's hospitality research team developed what they call "adaptive level selection," where initial choice sets help identify individual price sensitivity, which then informs personalized attribute levels in subsequent choices. This approach reduced hypothetical bias by 34% compared to fixed designs.
Experimental design efficiency determines statistical power and insight quality. Starbucks implemented D-optimal design techniques that reduced required sample sizes by 64% while maintaining statistical precision. This enabled them to test seasonal offerings across multiple market segments with far greater efficiency than traditional design approaches.
Nestlé addressed the challenge of too many potential attributes by implementing a two-stage choice modeling approach: first identifying the most important attribute categories through a simpler model, then conducting detailed choice experiments within those categories. This hierarchical approach made previously unwieldy product development decisions manageable while preserving decision realism.
Predictive Accuracy and Business Implementation
The ultimate value of choice modeling lies in its predictive accuracy and actionability for business decisions. Leading companies have developed sophisticated approaches to validate and implement choice modeling insights.
Predictive validation requires comparing model predictions against actual market outcomes. Microsoft's Surface product team implements what they call "limited release validation," where choice model predictions are tested against sales data from limited market introductions before full-scale launches. This identified a systematic overestimation of innovation value by 14%, allowing for prediction calibration that has improved forecast accuracy by 32%.
Competitor response modeling addresses the reality that market choices occur in competitive contexts. Samsung Mobile's choice modeling framework incorporates game theory elements to simulate how competitors might adjust their offerings in response to new product introductions. This dynamic approach improved market share predictions by 26% compared to static models that assumed fixed competitor offerings.
Price elasticity estimation represents one of choice modeling's most valuable applications. Coca-Cola's beverage portfolio management uses choice-based conjoint analysis to estimate cross-price elasticities between their own products and competitors. This "elasticity mapping" identified previously unknown substitution patterns, preventing cannibalization that internal forecasts had missed.
L'Oréal's beauty product division developed what they term "segment-specific choice modeling," where separate models are estimated for different consumer segments rather than relying on a single model with segment covariates. This approach improved new product forecasting accuracy by 47% by capturing fundamentally different decision rules across consumer types.
Conclusion
As consumer decision journeys grow increasingly complex in the digital age, choice modeling provides a methodological framework capable of capturing the multidimensional trade-offs that characterize real-world choices. The technique's ability to isolate the influence of specific attributes, quantify willingness to pay, and simulate market scenarios offers unprecedented insight into consumer decision-making.
The integration of choice modeling with digital behavioral data and artificial intelligence represents the frontier of consumer research methodology. Leading brands are now combining traditional choice modeling with digital footprint analysis, creating hybrid models that connect stated preferences with observed digital behaviors at scale.
Call to Action
For marketing leaders seeking to elevate their consumer research capabilities:
- Audit existing consumer insight methodologies to identify where choice modeling could address current limitations
- Develop proof-of-concept choice modeling studies for high-priority product or service decisions
- Invest in technical capacity building through specialized training or strategic hiring
- Create validation frameworks that systematically compare choice model predictions with market outcomes
- Implement "insights translation" processes that convert complex model outputs into actionable business recommendations
Tomorrow's market leaders will be organizations that move beyond asking consumers what they want to understanding how they choose, using choice modeling to bridge the persistent gap between what consumers say and what they do.
Featured Blogs
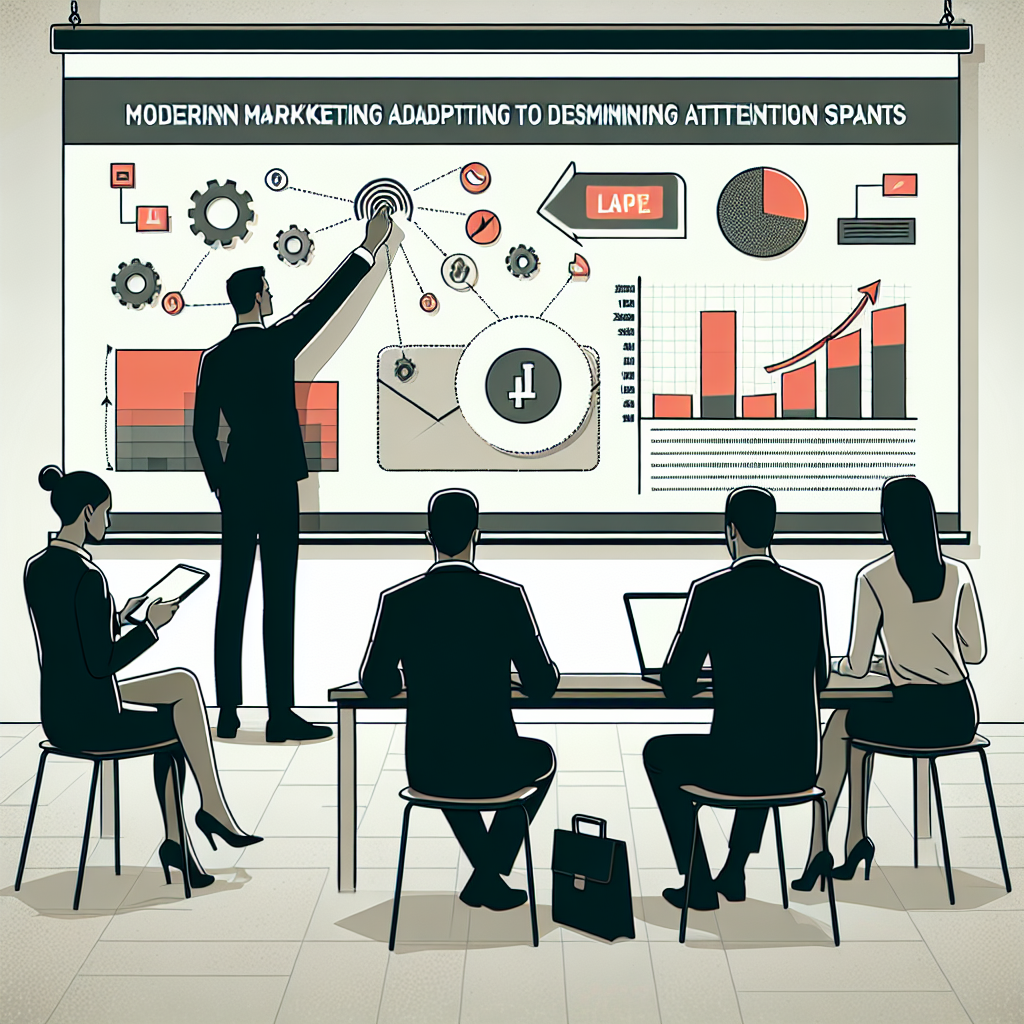
How the Attention Recession Is Changing Marketing
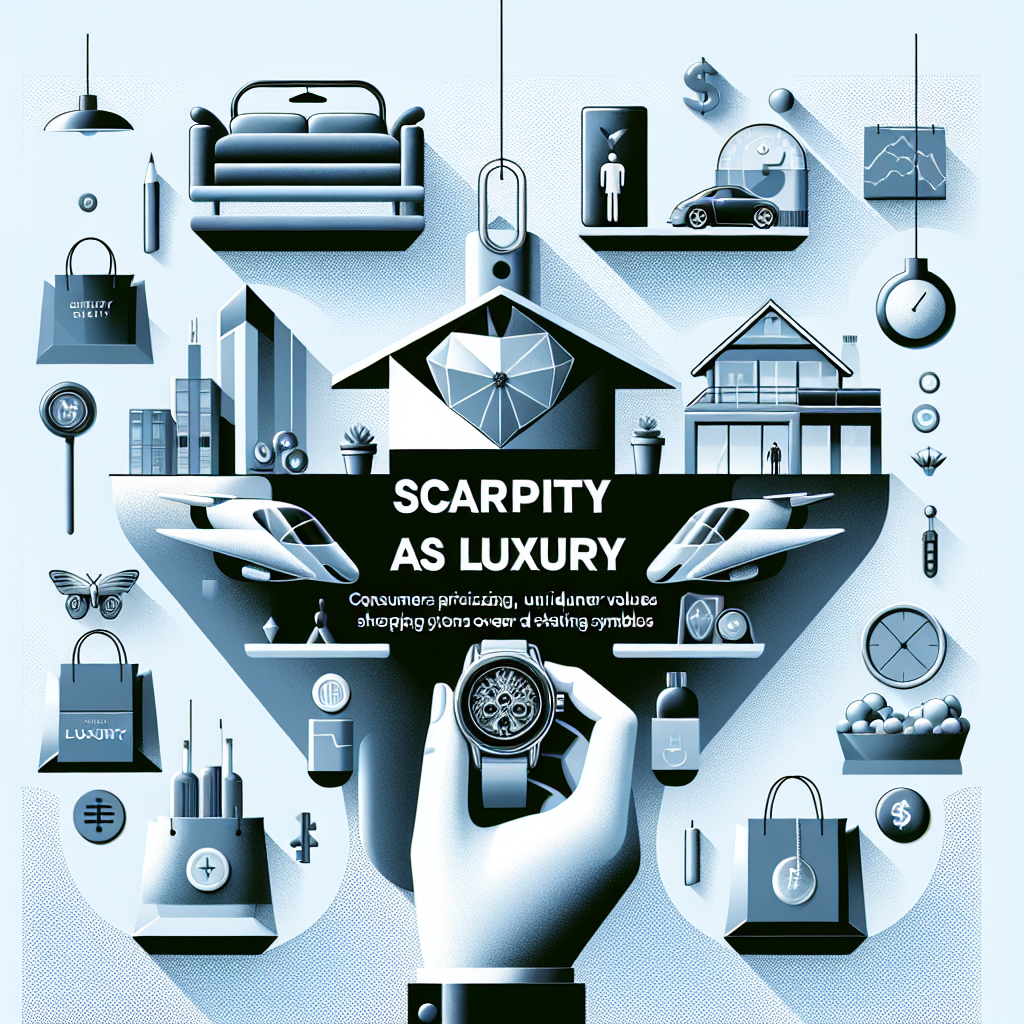
The New Luxury Why Consumers Now Value Scarcity Over Status
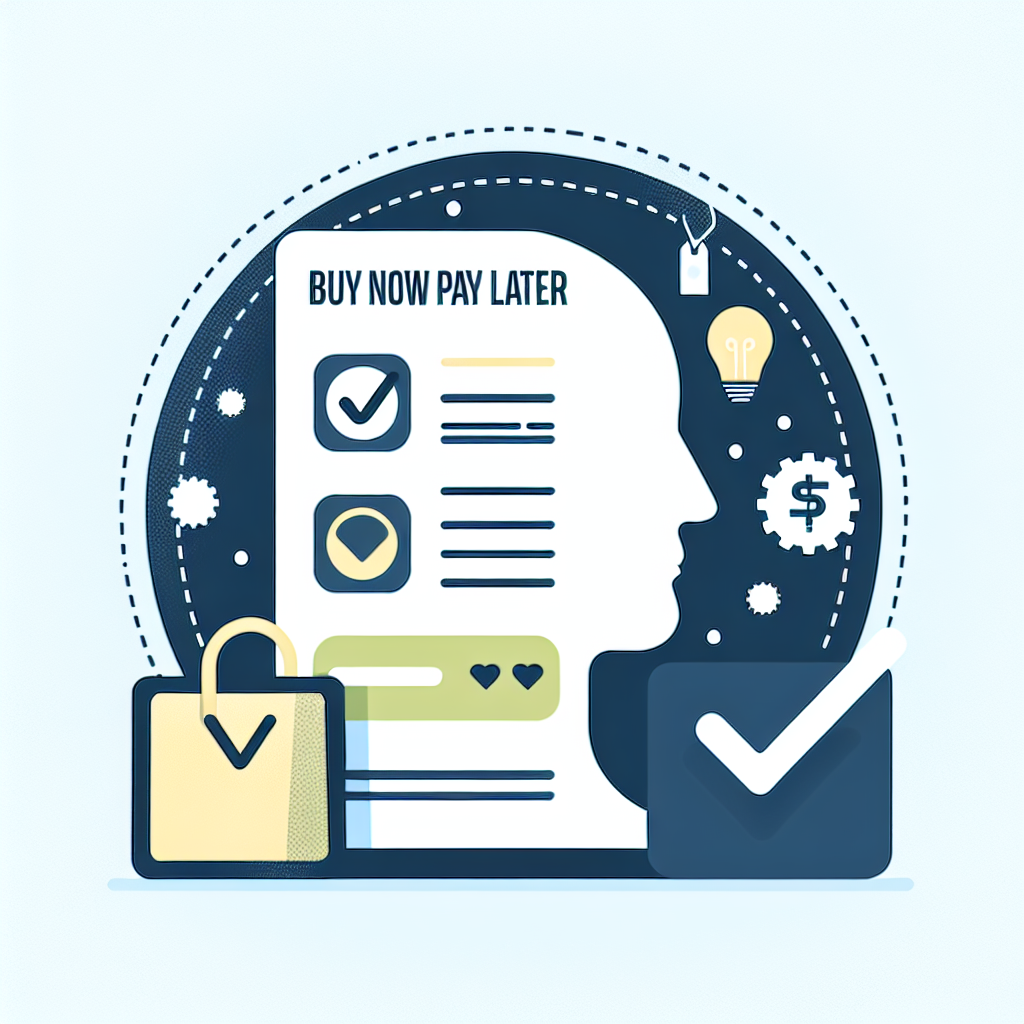
The Psychology Behind Buy Now Pay later
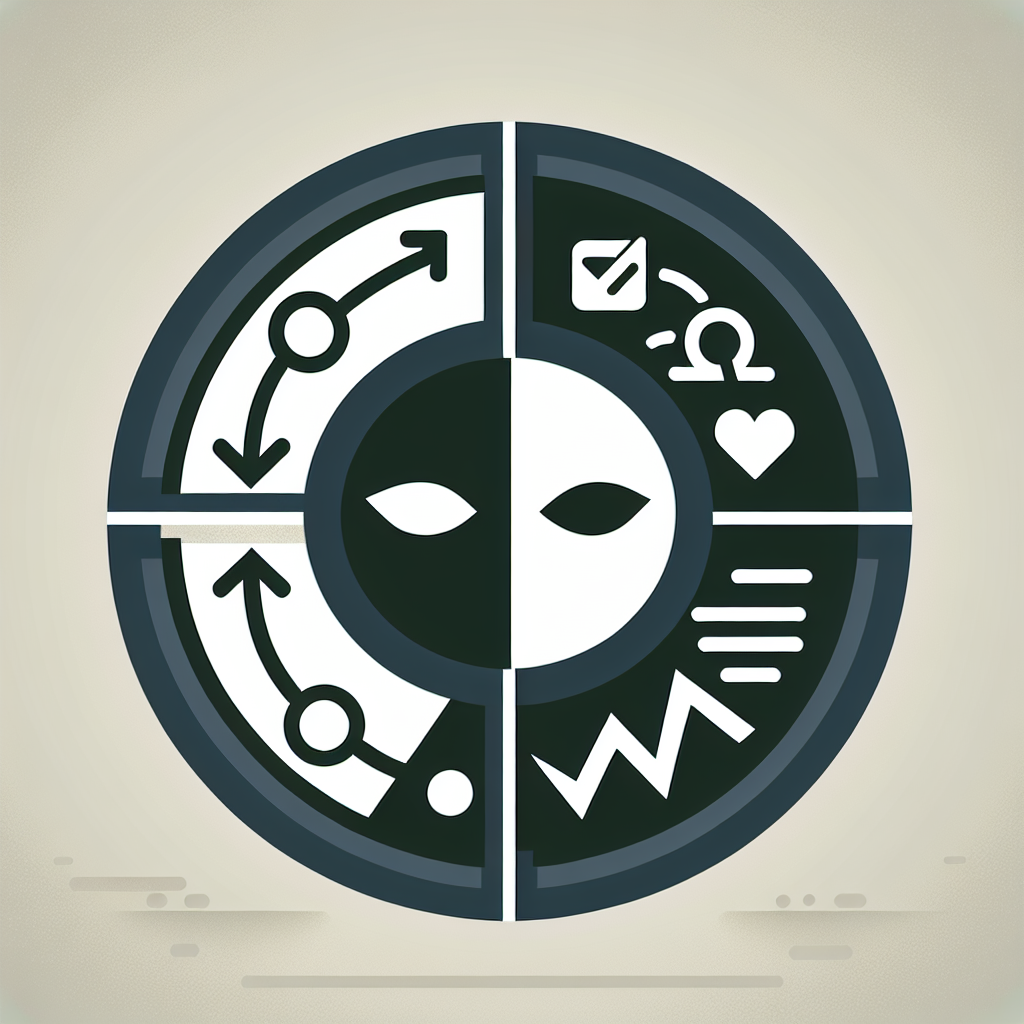
The Role of Dark Patterns in Digital Marketing and Ethical Concerns
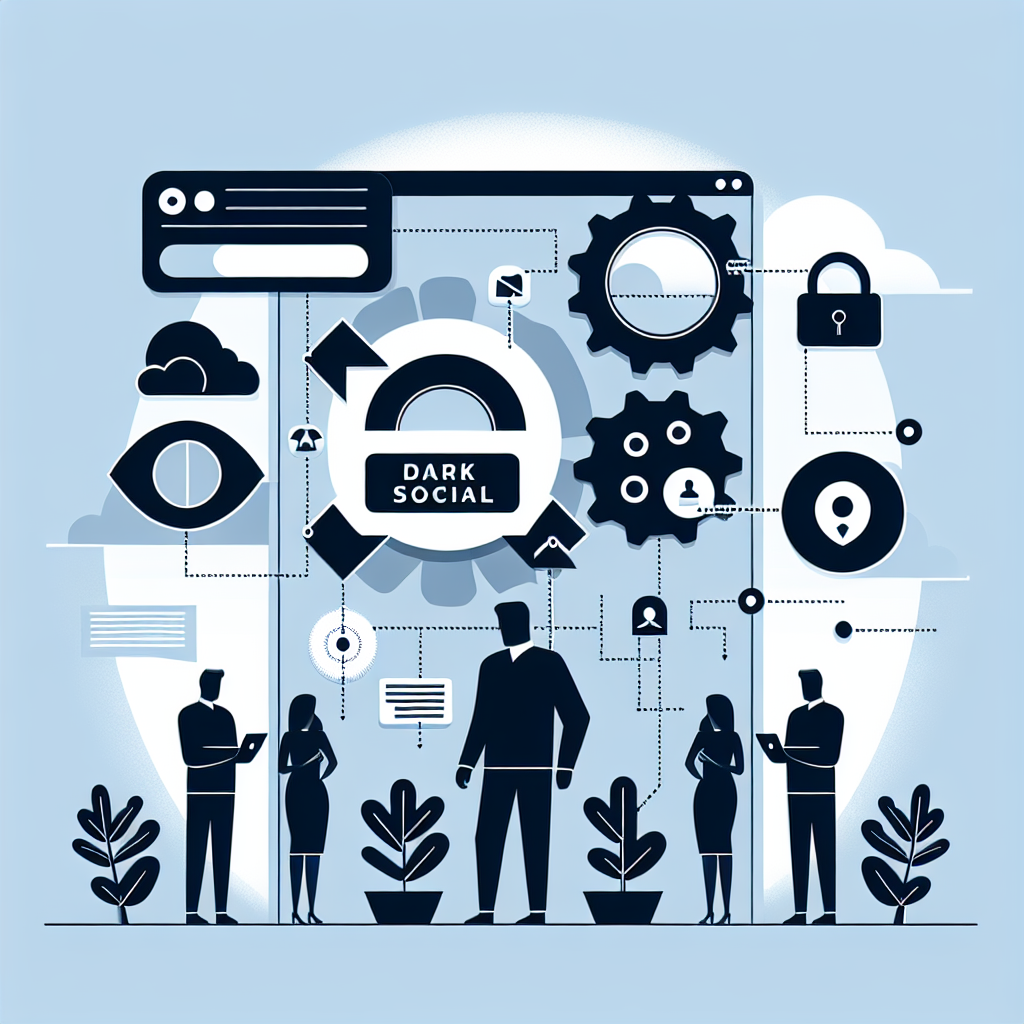
The Rise of Dark Social and Its Impact on Marketing Measurement
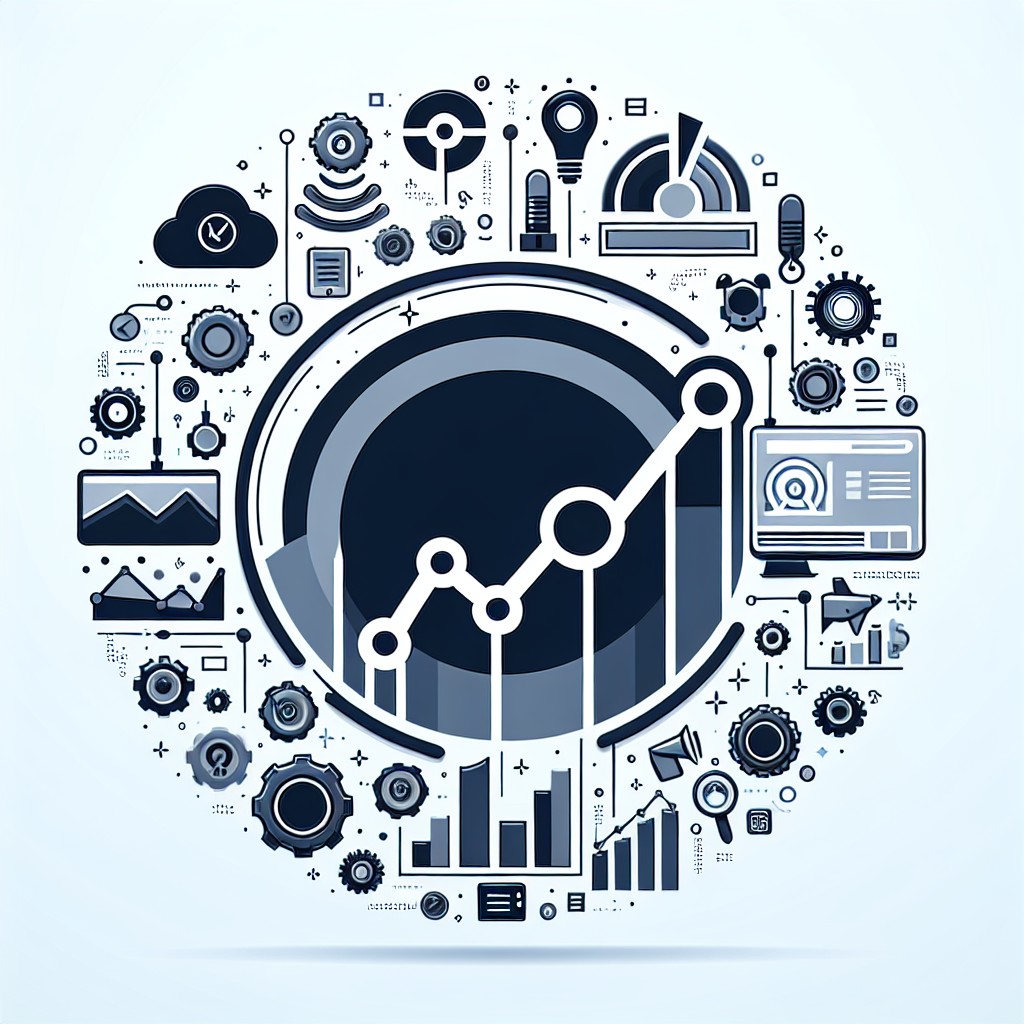
The Future of Retail Media Networks and What Marketers Should Know
Recent Blogs
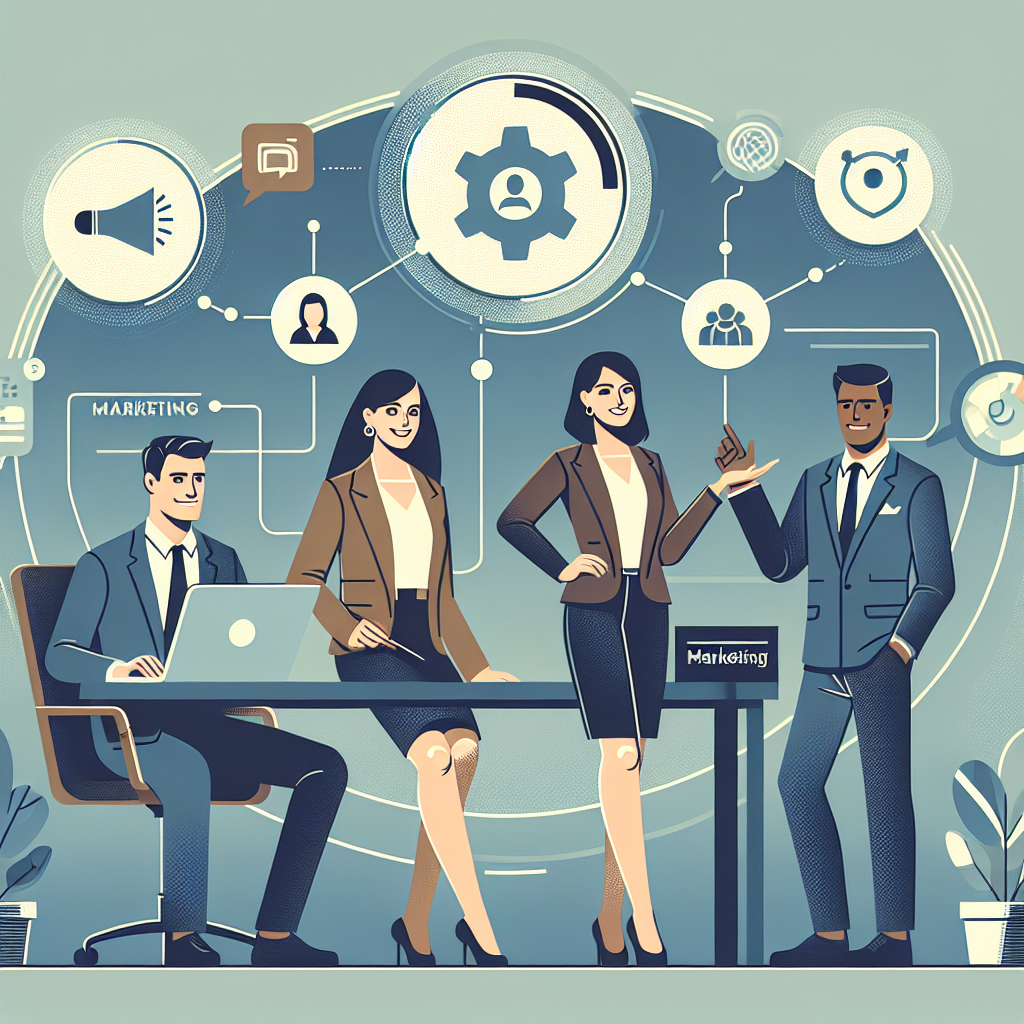
From Service to Experience - Training Teams for the Shift
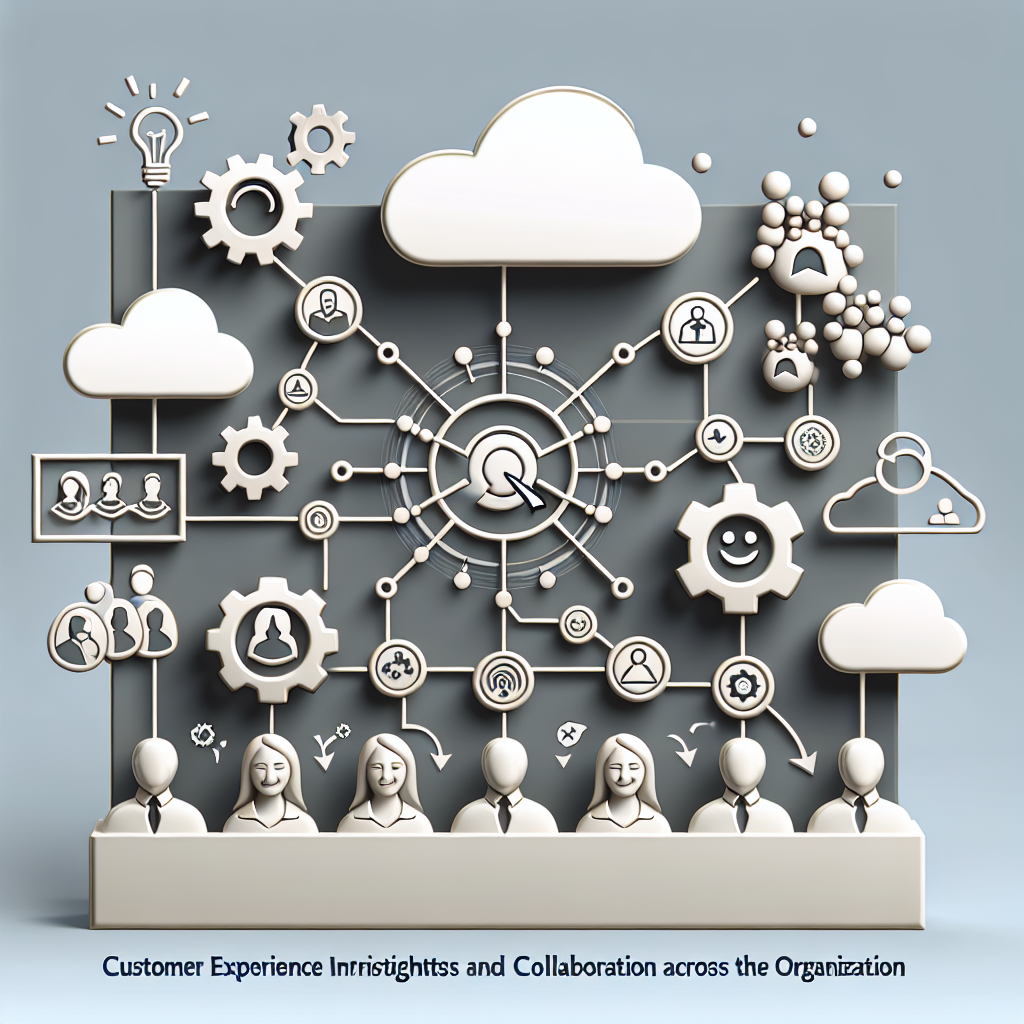
How to Democratize CX Insights Across the Org
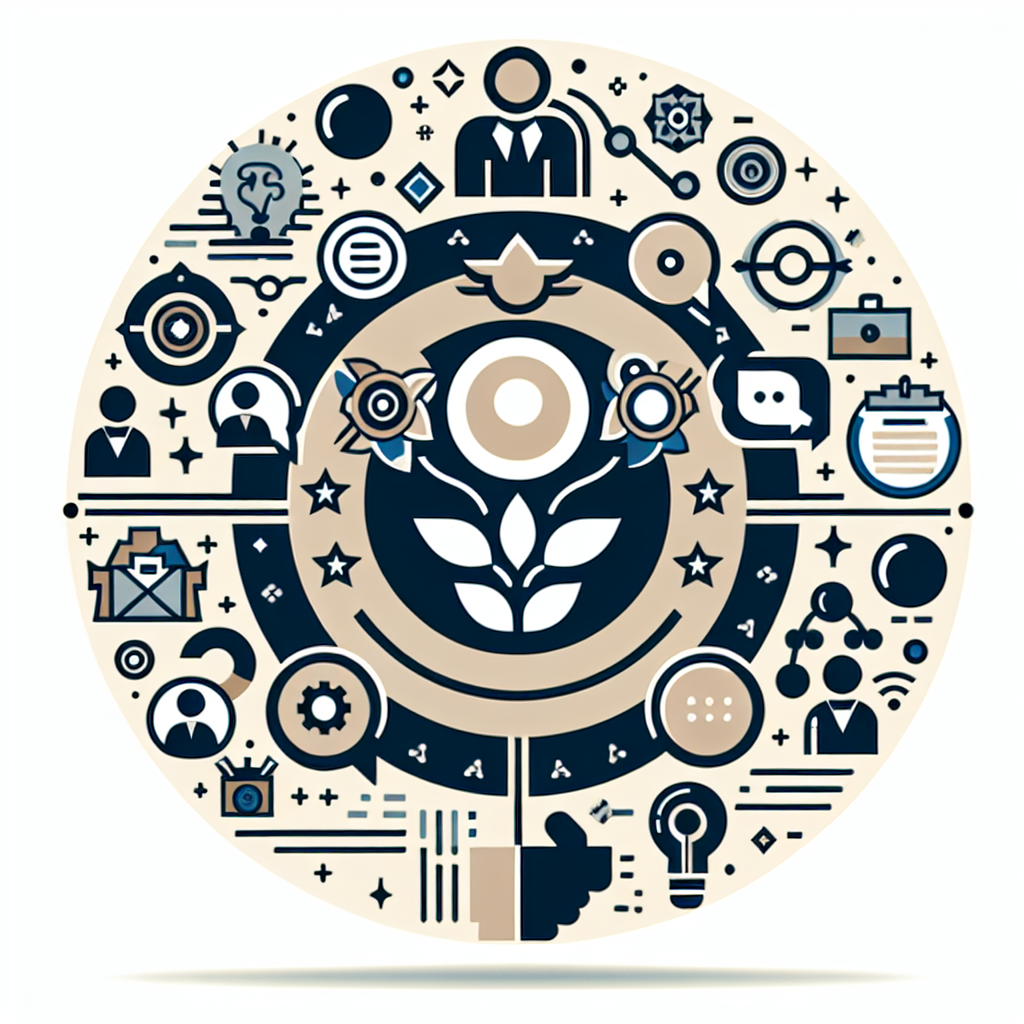
CX is Everyone's Job Building a Customer-Centric Culture
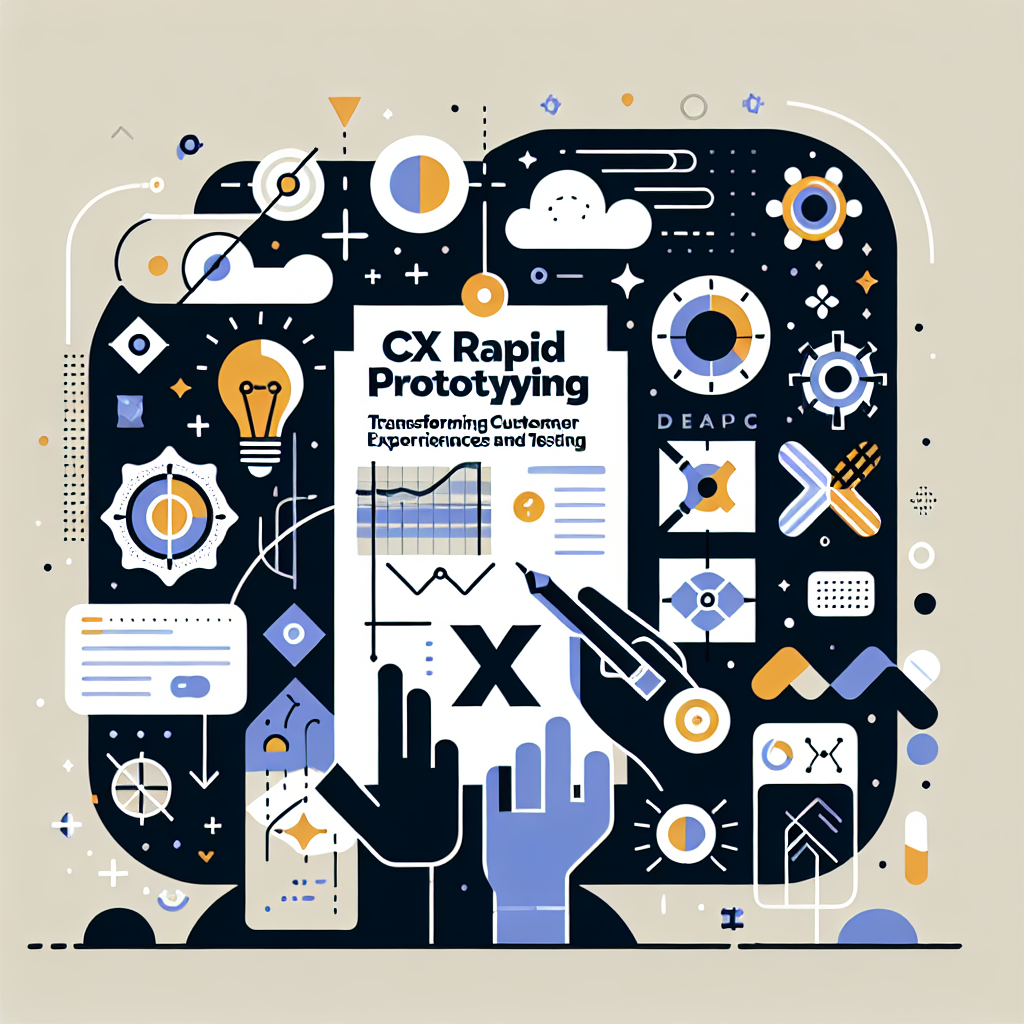
Experimenting with CX Rapid Prototyping for New Journeys
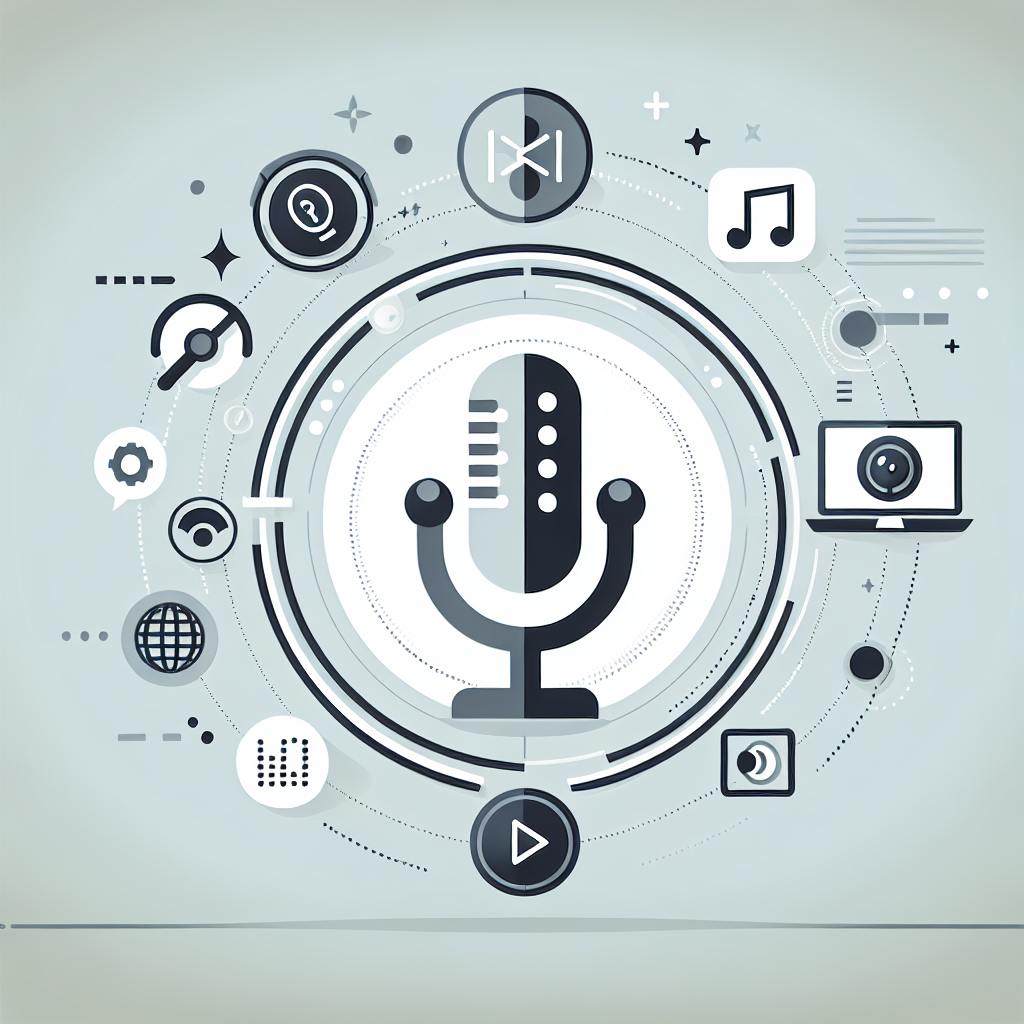
Voice, Video, and Beyond The Multimodal CX Future
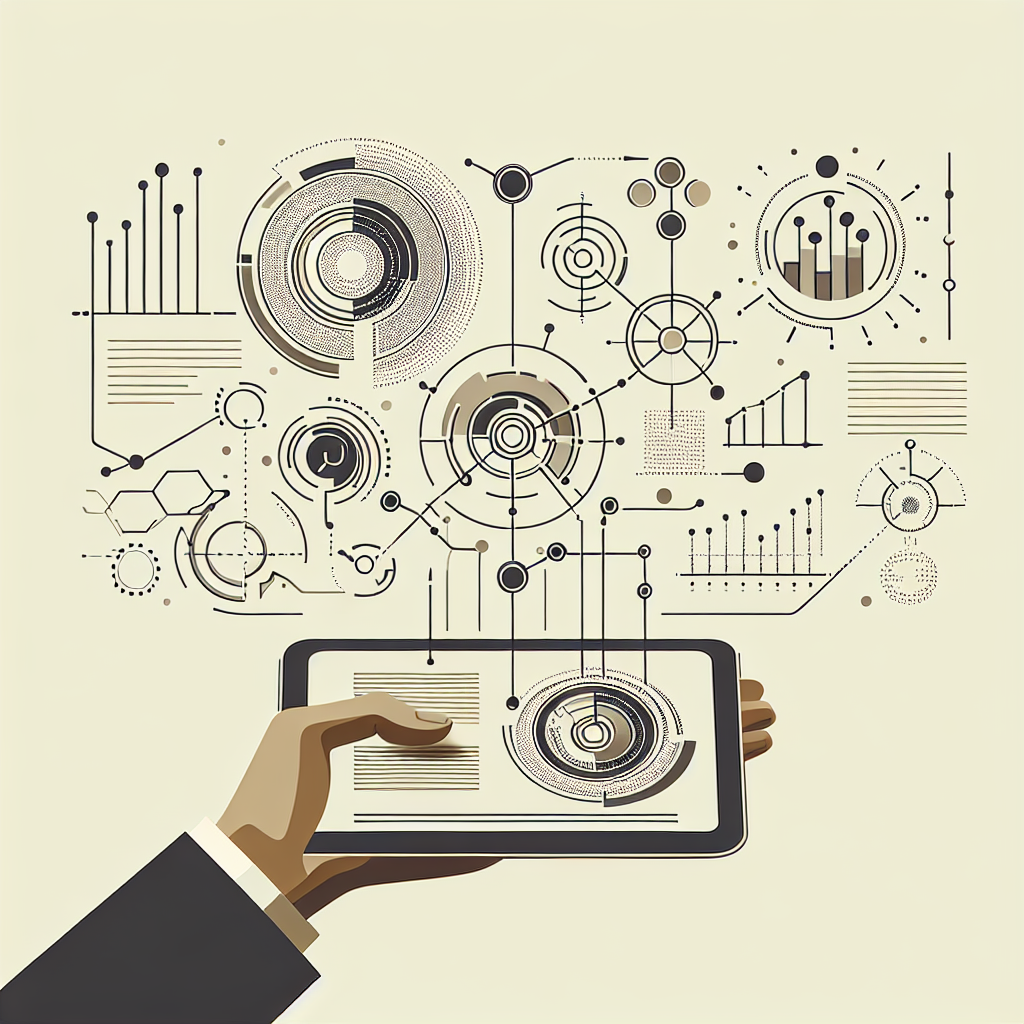