Cluster Analysis for Consumer Segmentation
Neeraj vividly recalls the puzzled expression on the CMO's face when reviewing the latest product launch results. Despite strong overall performance, the campaign had resonated brilliantly with some consumer groups while completely missing others. "But I thought we understood our target audience," she remarked, gesturing toward their traditional demographic segmentation report. That evening, Neeraj reached out to his former graduate school professor, who specialized in marketing analytics, for insight. "You're treating your market as one homogeneous group when it's actually several distinct clusters with different needs," she explained. "Demographics tell you who people are, but not how they think or what they value." This conversation ignited Neeraj's journey into cluster analysis—a methodological approach that fundamentally transformed their understanding of consumers and ultimately increased campaign effectiveness by 47%.
Introduction: Beyond Demographic Boxes
In today's complex marketplace, traditional demographic segmentation alone proves increasingly inadequate for capturing the nuanced reality of consumer behavior. The 45-year-old suburban father and the 42-year-old urban professional might share an age bracket and income level, yet hold radically different values, preferences, and purchasing patterns.
Cluster analysis addresses this limitation by identifying natural groupings within consumer data based on similarities across multiple dimensions. Rather than forcing consumers into predetermined categories, this approach lets patterns emerge organically from the data, revealing segments that often cut across conventional demographic lines.
Research from the Journal of Consumer Research demonstrates that segmentation strategies based on cluster analysis outperform demographic segmentation by 32% in conversion rates and 27% in customer lifetime value. As markets fragment and personalization becomes the norm, the ability to identify and understand these natural consumer groupings has evolved from competitive advantage to strategic necessity.
1. Input Variables: The Foundation of Meaningful Clusters
The quality of cluster analysis outputs depends entirely on selecting appropriate input variables—the dimensions along which consumer similarities will be measured.
Behavioral Variables
Behavioral Variables capture what consumers actually do:
- Purchase history patterns
- Product usage frequency
- Channel preferences
- Price sensitivity behaviors
- Loyalty program engagement
The supermarket chain Tesco revolutionized its marketing by clustering consumers based primarily on shopping basket composition, discovering seven distinct shopper types that transcended demographic categories and led to a 21% increase in promotion effectiveness.
Attitudinal Variables
Attitudinal Variables reflect how consumers think:
- Category involvement levels
- Brand perception measures
- Need states and motivations
- Value orientation (price vs. quality vs. convenience)
- Risk tolerance
Insurance provider USAA implemented attitude-based clustering that identified a previously unrecognized segment of "security planners"—consumers highly receptive to bundled protection products regardless of demographic profile.
Psychographic Variables
Psychographic Variables delve into lifestyle patterns:
- Activities and interests
- Values and beliefs
- Life stage considerations
- Personal aspirations
- Media consumption habits
When athletic apparel brand Lululemon applied psychographic clustering, they identified a substantial "wellness-focused but competition-averse" segment that led to the development of their highly successful casual athleisure line.
2. Interpreting Clusters: From Statistics to Strategy
The true value of cluster analysis emerges when statistical groupings transform into actionable consumer portraits that drive marketing strategy.
Profiling and Naming
Profiling and Naming brings clusters to life:
- Cross-tabulate clusters with descriptive variables
- Identify statistically significant differentiators
- Develop memorable, meaningful cluster names
- Create rich consumer personas for each segment
After clustering users based on app interaction patterns, Spotify developed five distinct listener personas, including "Discovery Enthusiasts" and "Background Listeners," allowing highly targeted feature development and communication strategies.
Size and Value Assessment
Size and Value Assessment determines strategic priorities:
- Calculate the current market share within each cluster
- Analyze profitability potential by segment
- Assess growth trajectory for each group
- Identify underserved high-value clusters
When hotel group Hilton applied cluster valuation to its customer base, they discovered their third-largest segment generated nearly twice the profit per customer as their largest segment, reshaping resource allocation across their portfolio.
Strategic Application
Strategic Application connects insights to action:
- Develop segment-specific value propositions
- Optimize marketing mix by cluster
- Align product development with cluster needs
- Design targeted acquisition strategies
Financial services provider American Express used cluster insights to completely redesign its customer journey maps for three distinct cardholder segments, resulting in a 34% increase in engagement and 28% reduction in churn among previously at-risk customers.
3. Pitfalls to Avoid: Ensuring Analytical Integrity
Despite its power, cluster analysis contains methodological traps that can undermine its effectiveness if not properly addressed.
Over-clustering
Over-clustering creates artificial distinctions:
- Statistical indicators like the elbow method and silhouette scores help determine optimal cluster numbers
- More clusters aren't necessarily better
- Each additional cluster should be meaningfully different and actionable
- Segment size must remain practical for separate marketing efforts
When Unilever initially identified eight consumer segments for their personal care division, further validation revealed that three pairs were not meaningfully different in purchasing behavior, leading them to consolidate to five truly distinct clusters.
Variable Selection Biases
Variable Selection Biases distort results:
- Including too many correlated variables overweights certain factors
- Mixing scales without standardization skews outcomes
- Overrepresentation of available data points creates blind spots
- Missing values require careful handling strategies
Netflix discovered their initial content preference clusters were heavily skewed by interface interaction metrics simply because they had more of this data, leading to a rebalanced approach incorporating diverse data sources.
Implementation Disconnect
Implementation Disconnect wastes analytical effort:
- Clusters must connect to operational capabilities
- Segmentation should inform actionable decisions
- All stakeholders need to understand and embrace the framework
- Regular validation ensures continued relevance
When Ford attempted to implement a complex eight-cluster consumer segmentation, dealer confusion and implementation challenges led them to simplify to four primary segments with clear dealership implications, dramatically improving adoption and effectiveness.
Call to Action
To leverage cluster analysis for more effective consumer segmentation:
- Begin by clearly defining the strategic purpose your segmentation will serve
- Collect diverse data spanning behaviors, attitudes, and needs—not just demographics
- Apply both statistical validation and common-sense checks to your cluster solutions
- Create compelling internal narratives around each segment to drive organizational adoption
- Implement a continuous feedback loop to refine segments as your market evolves
Reflecting on that conversation with my professor years ago, I'm struck by how fundamentally cluster analysis changed our marketing approach. What began as a technical solution to a campaign challenge evolved into a company-wide shift in how we conceptualize our consumers. Rather than debating subjective consumer archetypes in planning meetings, we now ground our strategy in data-derived clusters that reveal not just who our consumers are demographically, but how they behave, what they value, and why they choose—insights that continue to drive growth across our entire portfolio.
Featured Blogs
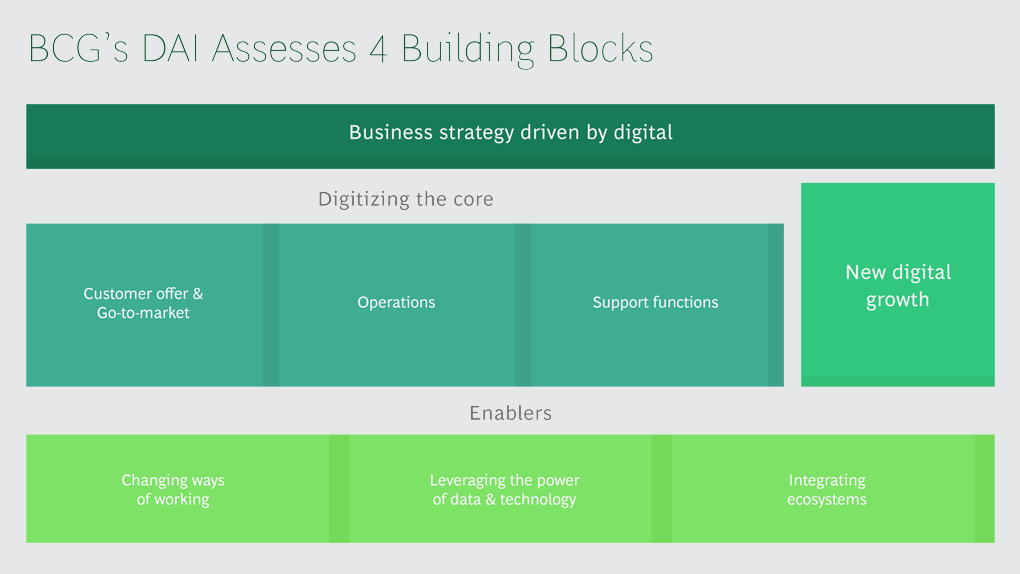
BCG Digital Acceleration Index
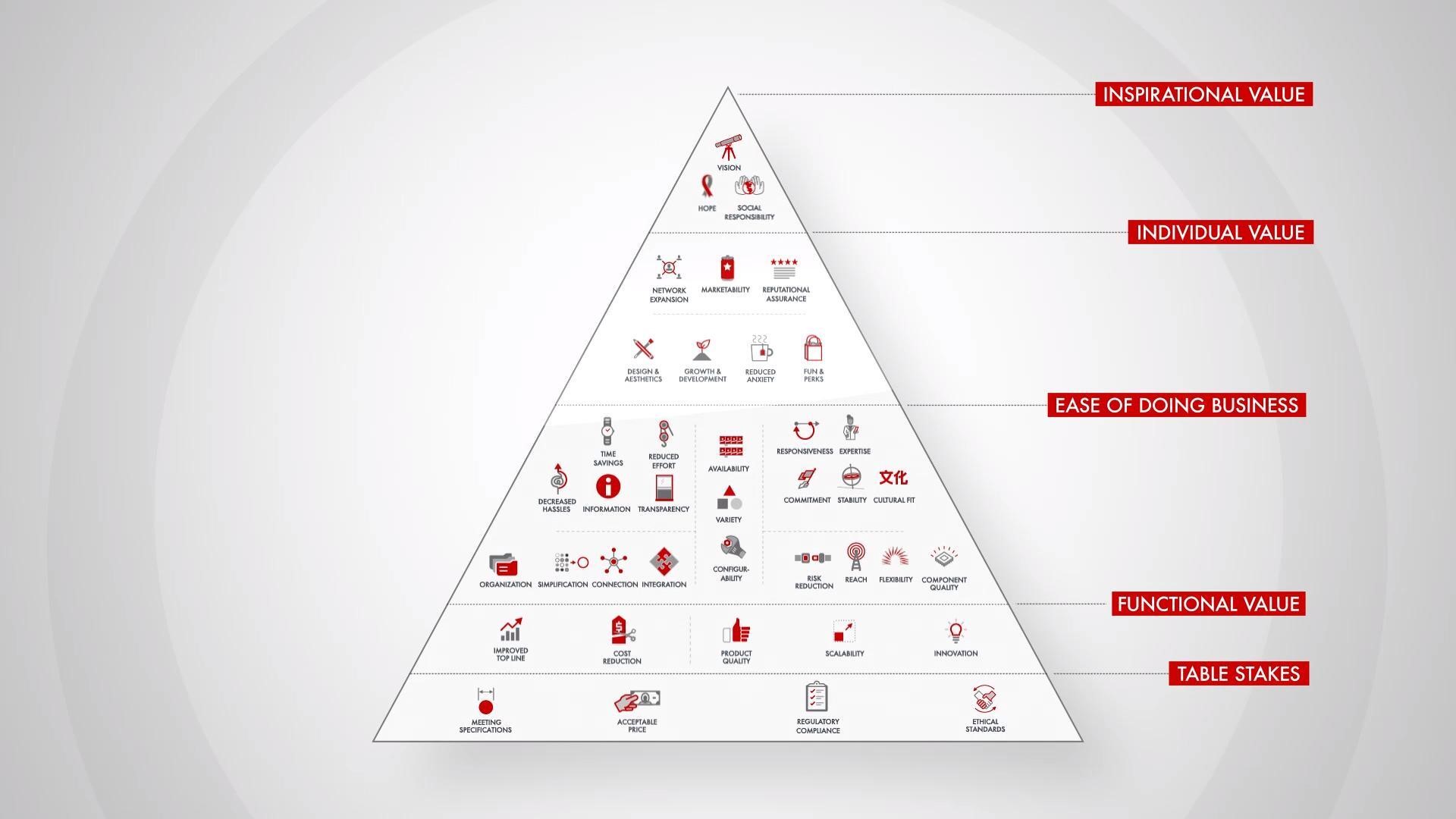
Bain’s Elements of Value Framework
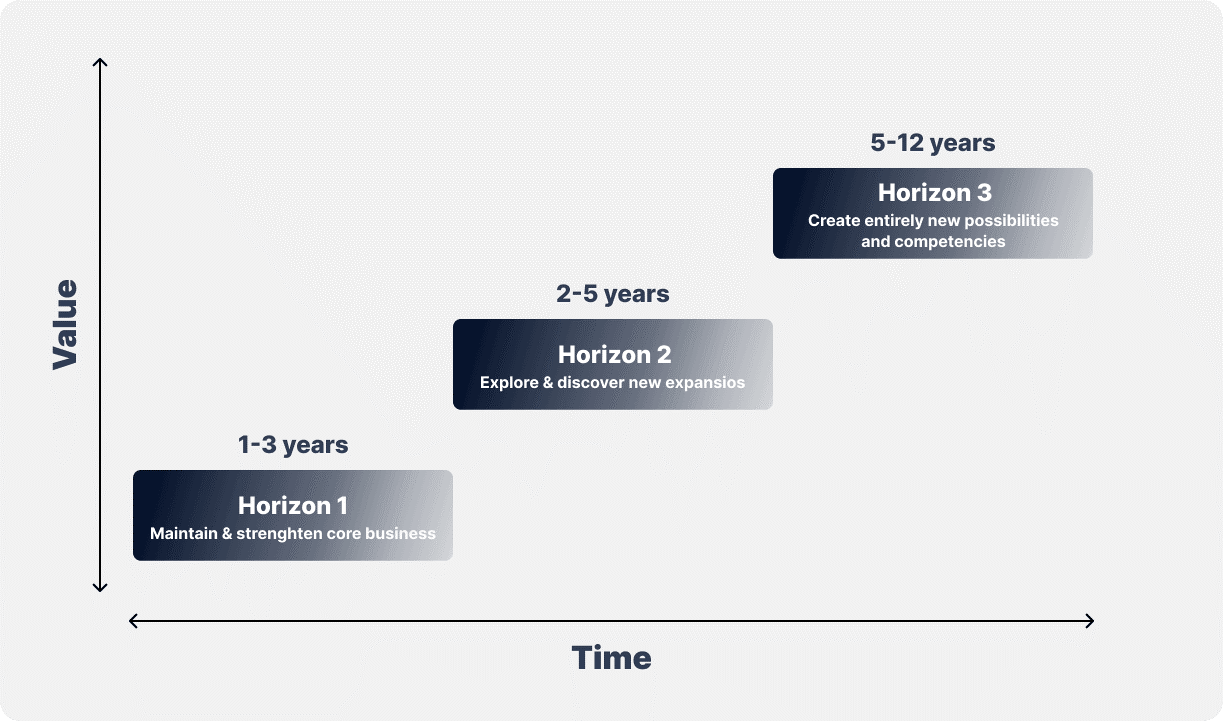
McKinsey Growth Pyramid
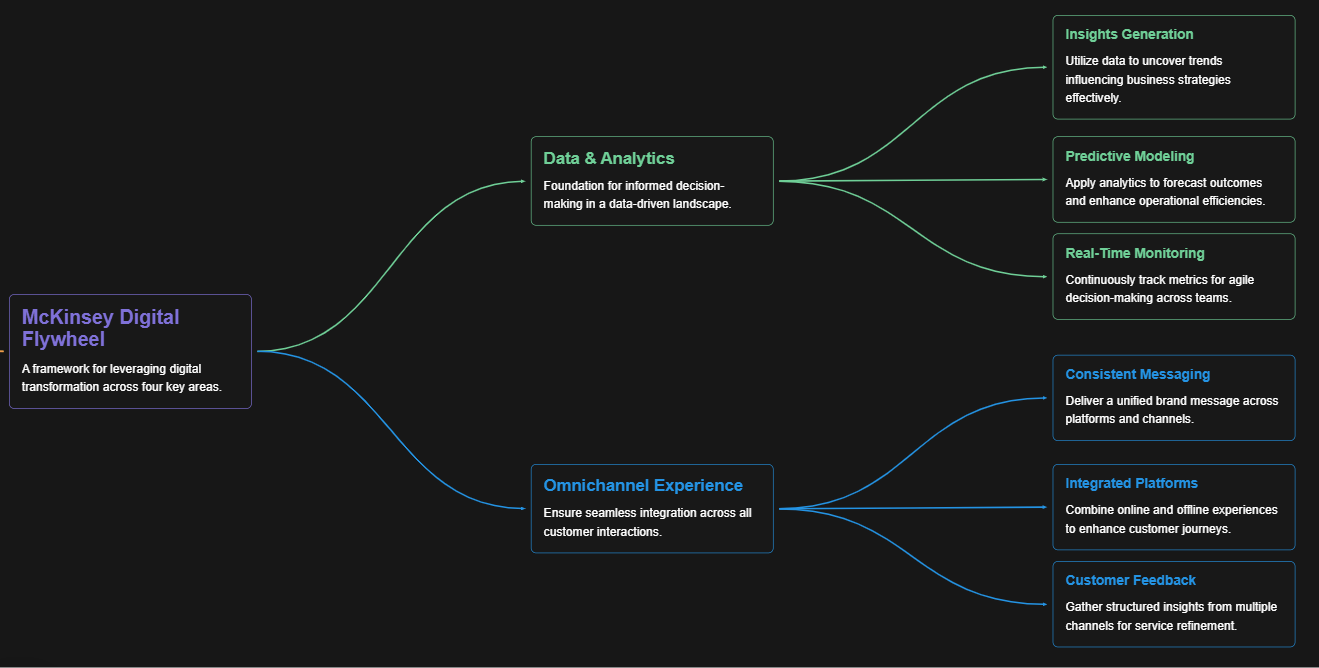
McKinsey Digital Flywheel
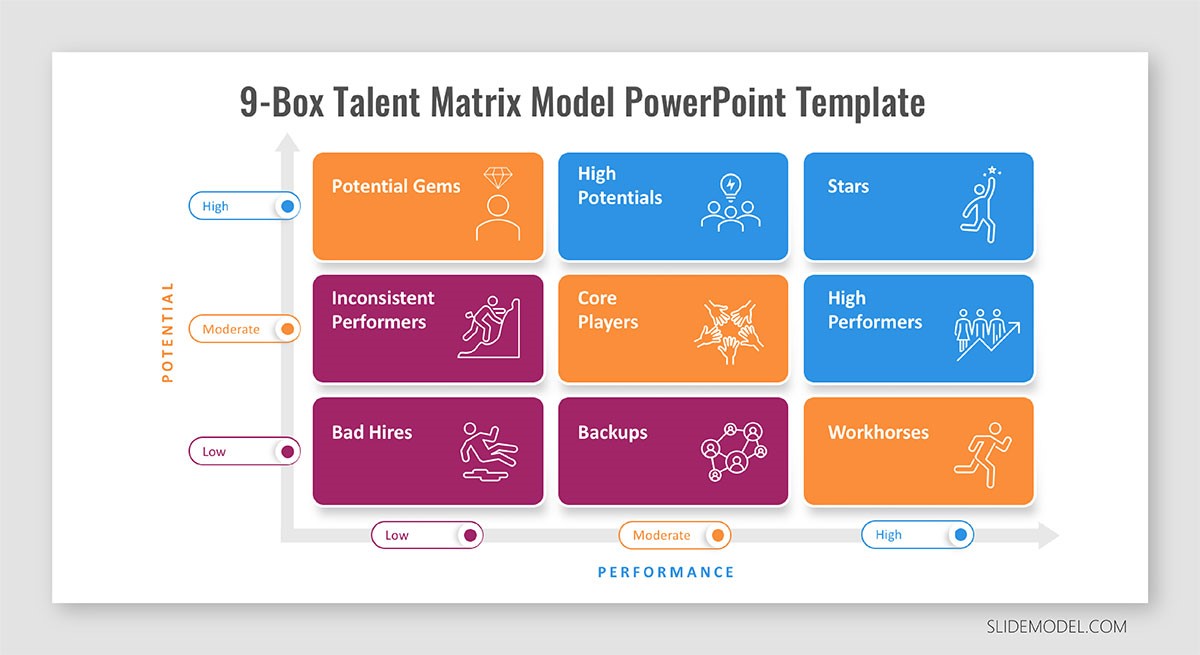
McKinsey 9-Box Talent Matrix
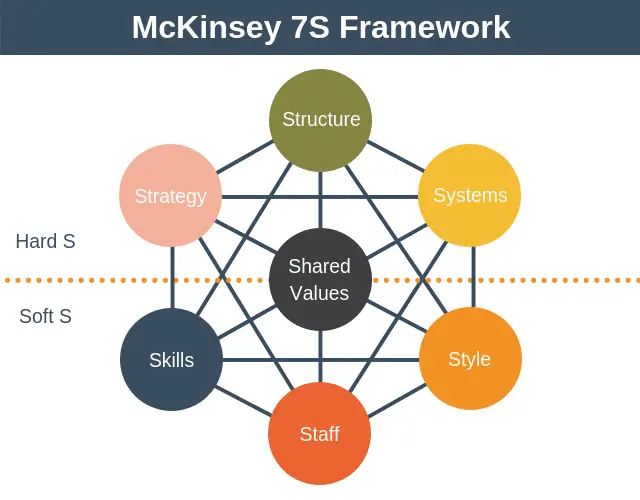
McKinsey 7S Framework
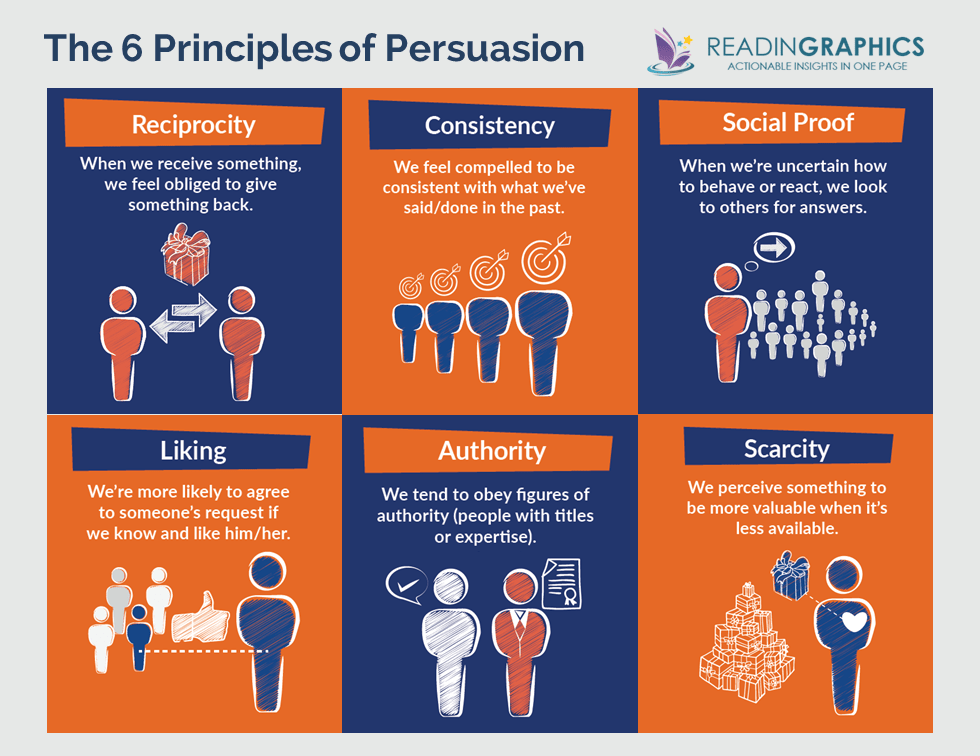
The Psychology of Persuasion in Marketing
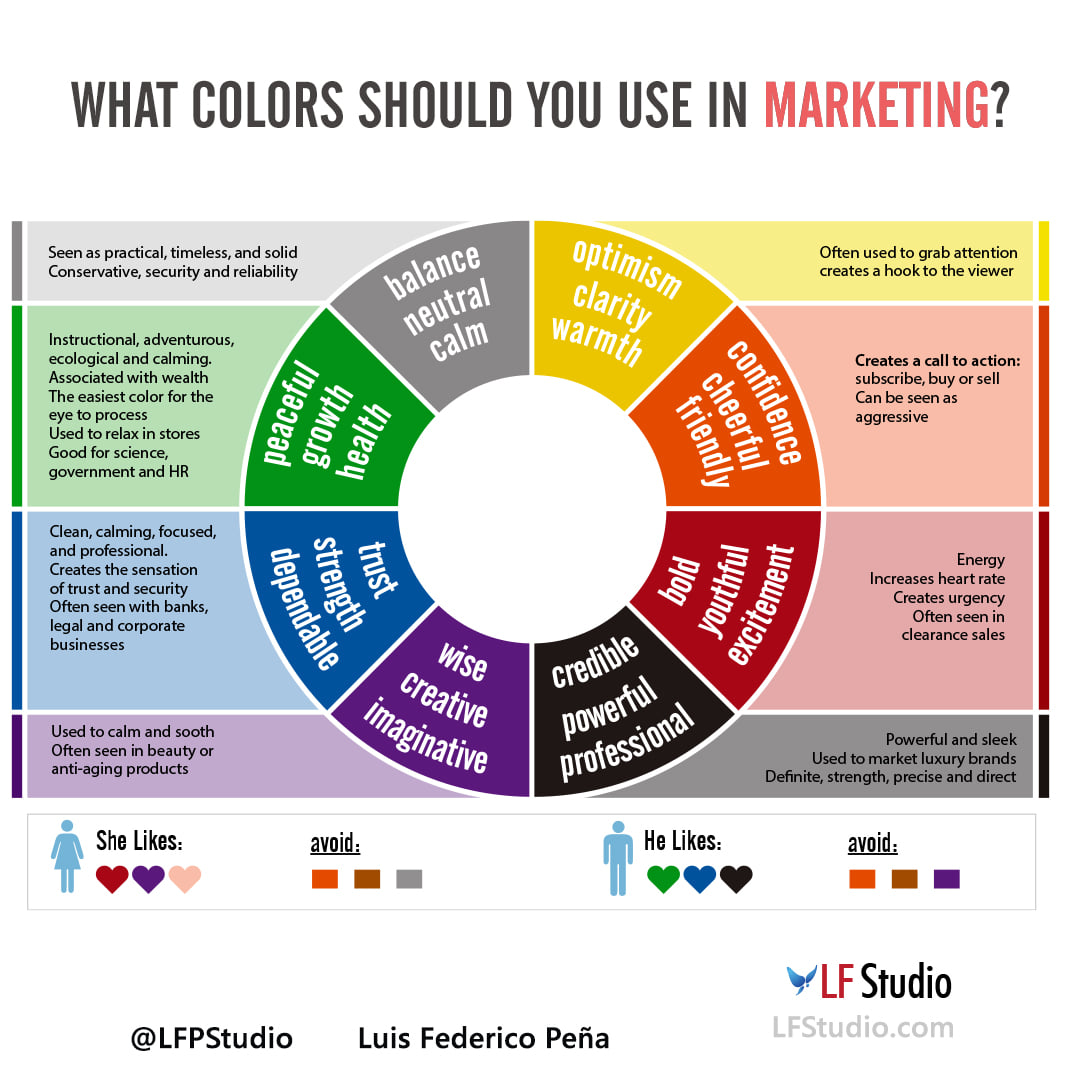
The Influence of Colors on Branding and Marketing Psychology
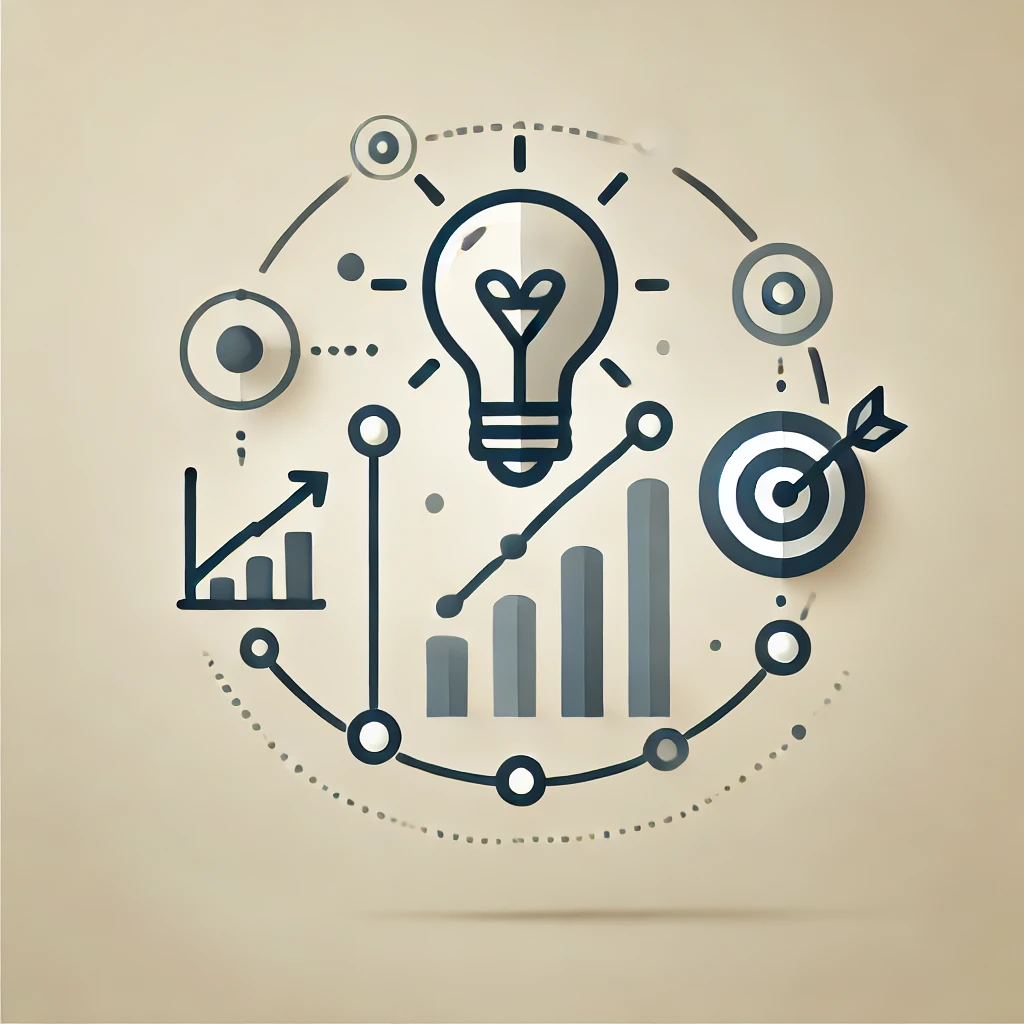