Correlation vs. Causation in Marketing Studies
During an industry conference last quarter, Neeraj found himself in a heated discussion with a marketing director who proudly announced their latest insight: "We discovered that customers who engage with our email newsletters spend 40% more than non-engaged customers, so we're tripling our email marketing budget." When Neeraj asked whether engaged customers spend more because of the emails or if big spenders simply tend to open more emails, the director's confident expression faltered. This exchange underscored one of the most persistent challenges in marketing research—distinguishing between correlation and causation—a distinction that can mean the difference between strategic insight and expensive misdirection.
Introduction: The Correlation-Causation Challenge
The correlation-causation distinction represents perhaps the most common analytical error in marketing decision-making. While identifying correlations (when two metrics move together) is relatively straightforward, establishing causation (when one factor directly influences another) requires significantly more methodological rigor. Yet this distinction underpins every effective marketing strategy.
Research from the Journal of Marketing Analytics indicates that approximately 62% of marketing studies conflate correlation with causation in their conclusions. Meanwhile, a survey by the Marketing Management Analytics Association found that 71% of marketing leaders acknowledged making significant budget allocations based on correlational evidence without establishing causality.
As marketing strategist Avinash Kaushik observes, "Correlation gives you hints about where to look; causation tells you where to act." Understanding this distinction transforms marketing from informed guesswork into strategic science.
1. Examples Where Confusion Occurs
Several common scenarios repeatedly trap marketers in correlation-causation confusion:
- Channel attribution analysis: Assuming the last touchpoint caused the conversion when it may simply correlate with customer decision timing
- Social media engagement: Interpreting high engagement metrics as causing sales lifts when engaged customers might already be predisposed to purchase
- Brand tracking studies: Concluding that awareness campaigns directly drove sales increases that may have resulted from concurrent product improvements or competitive factors
Tech company Spotify initially attributed increased subscription conversions to their podcast investment based on strong correlational data—subscribers consumed more podcasts than non-subscribers. However, careful experimentation revealed that podcast listeners were already higher-value customers before podcast exposure. This insight shifted their strategy from general podcast acquisition to targeted content development for specific customer journey stages.
2. Tools to Establish Causality
Marketing researchers employ several methods to move beyond correlation to causation:
- Randomized controlled experiments: The gold standard, where customers are randomly assigned to treatment and control groups
- Natural experiments: Leveraging external changes (e.g., regional rollouts) to create comparison opportunities
- Quasi-experimental designs: Including difference-in-differences analysis, regression discontinuity, and instrumental variables
- Granger causality testing: Statistical tests examining whether past values of one variable predict future values of another
Global CPG company Unilever developed what they call "Causal Marketing Maps" that combine controlled experiments with advanced statistical modeling. This framework enabled them to distinguish between correlation and causation in their digital marketing efforts, ultimately reducing wasted advertising spend by 29% while maintaining sales performance.
3. Visualizing Relationships
Effective visualization techniques help communicate the distinction between correlation and causation:
- Directed acyclic graphs (DAGs): Visual representations of causal relationships showing direct and indirect influences
- Confounding factor matrices: Mapping potential hidden variables influencing both the presumed cause and effect
- Time-series decomposition: Visualizing relationship patterns over time to identify leading versus lagging indicators
E-commerce platform Shopify developed a visualization system called "Causal Canvas" that maps correlations alongside confirmed causal relationships. This tool helps their merchant partners distinguish metrics they should monitor from those they should actively influence, resulting in 26% more effective budget allocations according to their internal studies.
Conclusion: From Correlation to Causation
The shift from correlational thinking to causal understanding represents a crucial evolution in marketing maturity. Organizations that master this distinction make more effective resource allocations, avoid costly strategic errors, and build competitive advantage through truly actionable insights.
As marketing environments grow increasingly complex with more channels, touchpoints, and data sources, the ability to distinguish correlation from causation becomes not just a methodological nicety but a fundamental business skill. The future belongs to marketing organizations that combine methodological rigor with business intuition—transforming the correlation-causation challenge from a conceptual hurdle into a strategic asset.
Call to Action
Transform your organization's approach to causality through these practical steps:
- Audit existing marketing assumptions to identify where correlational thinking may be driving decisions
- Develop a tiered evidence framework that explicitly distinguishes correlational findings from causal conclusions
- Implement controlled experimentation protocols, even at small scales, to test key marketing assumptions
- Invest in analytical talent with specific training in causal inference methodologies
- Create visualization standards that clearly communicate the difference between observed correlations and established causal relationships
The pursuit of causality isn't merely academic—it's the foundation of truly effective marketing in an increasingly complex business landscape.
Featured Blogs
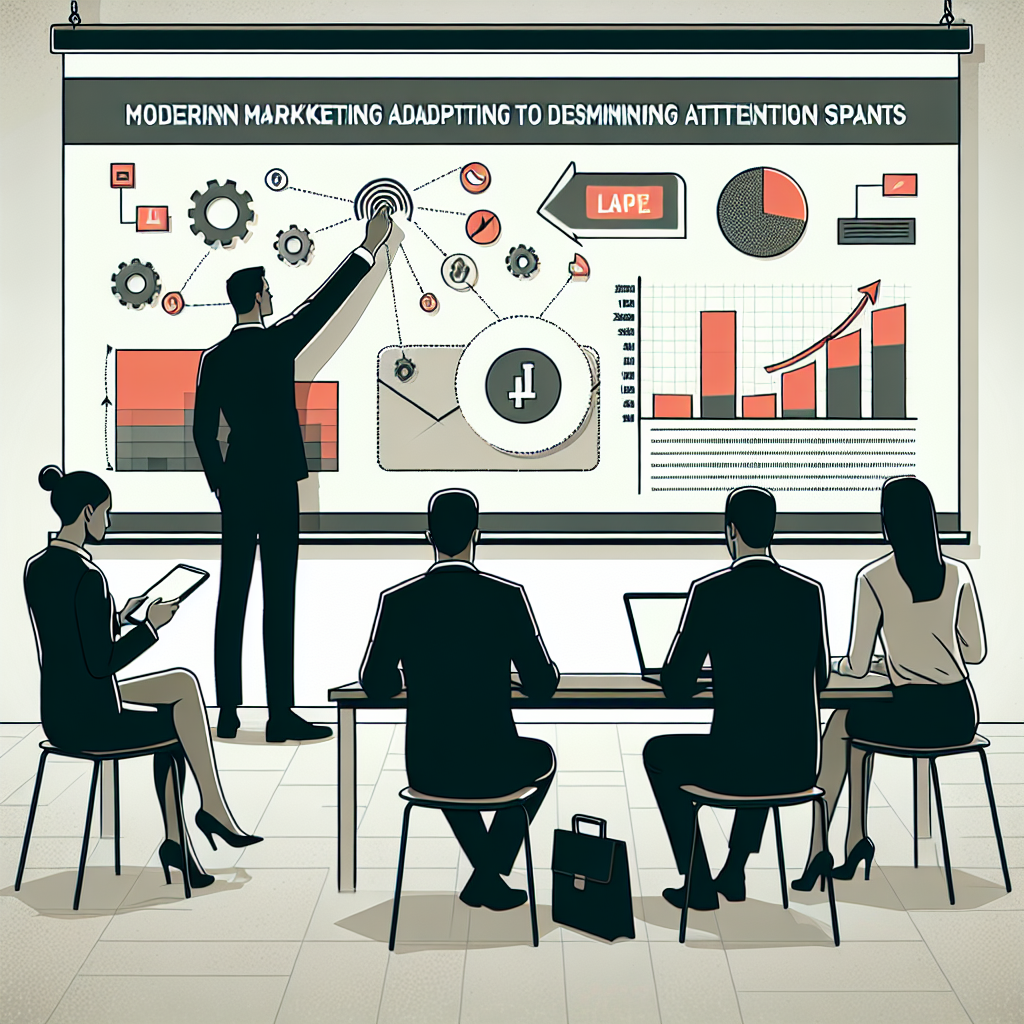
How the Attention Recession Is Changing Marketing
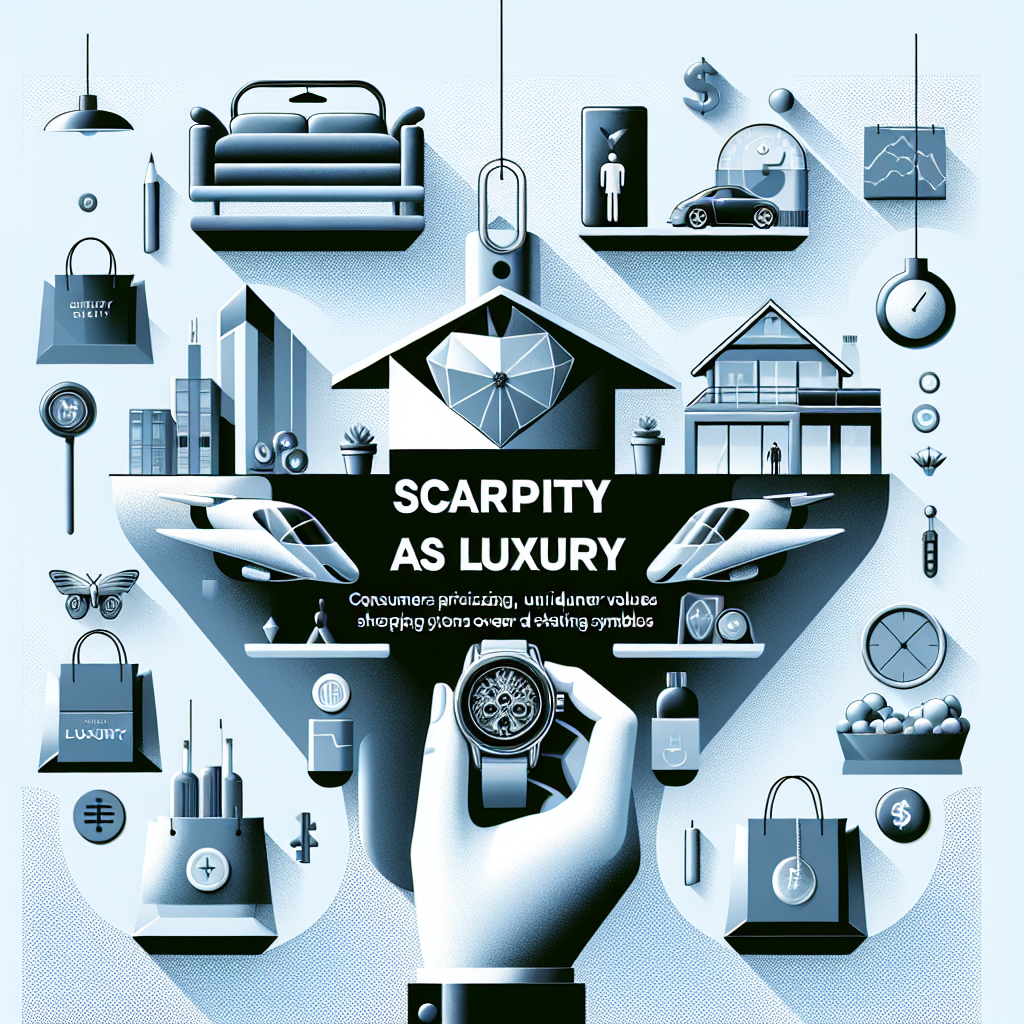
The New Luxury Why Consumers Now Value Scarcity Over Status
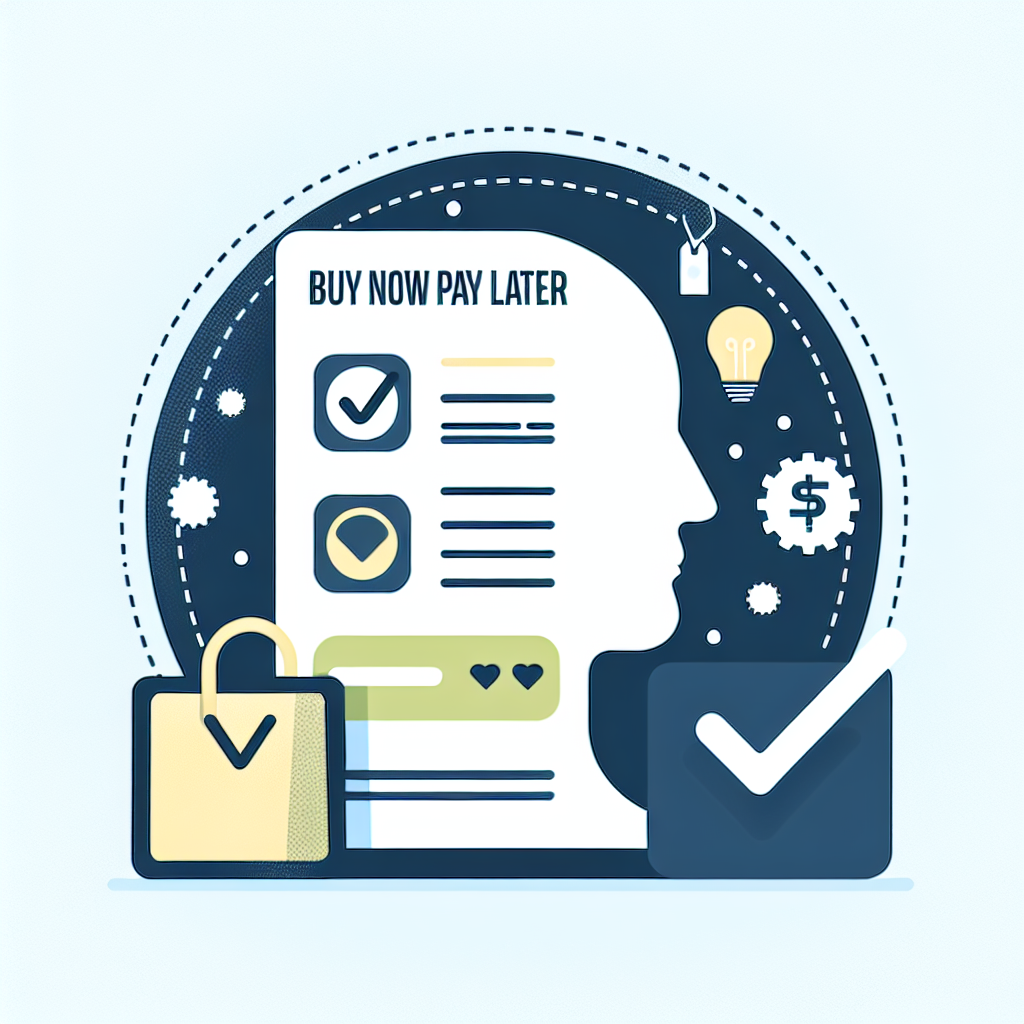
The Psychology Behind Buy Now Pay later
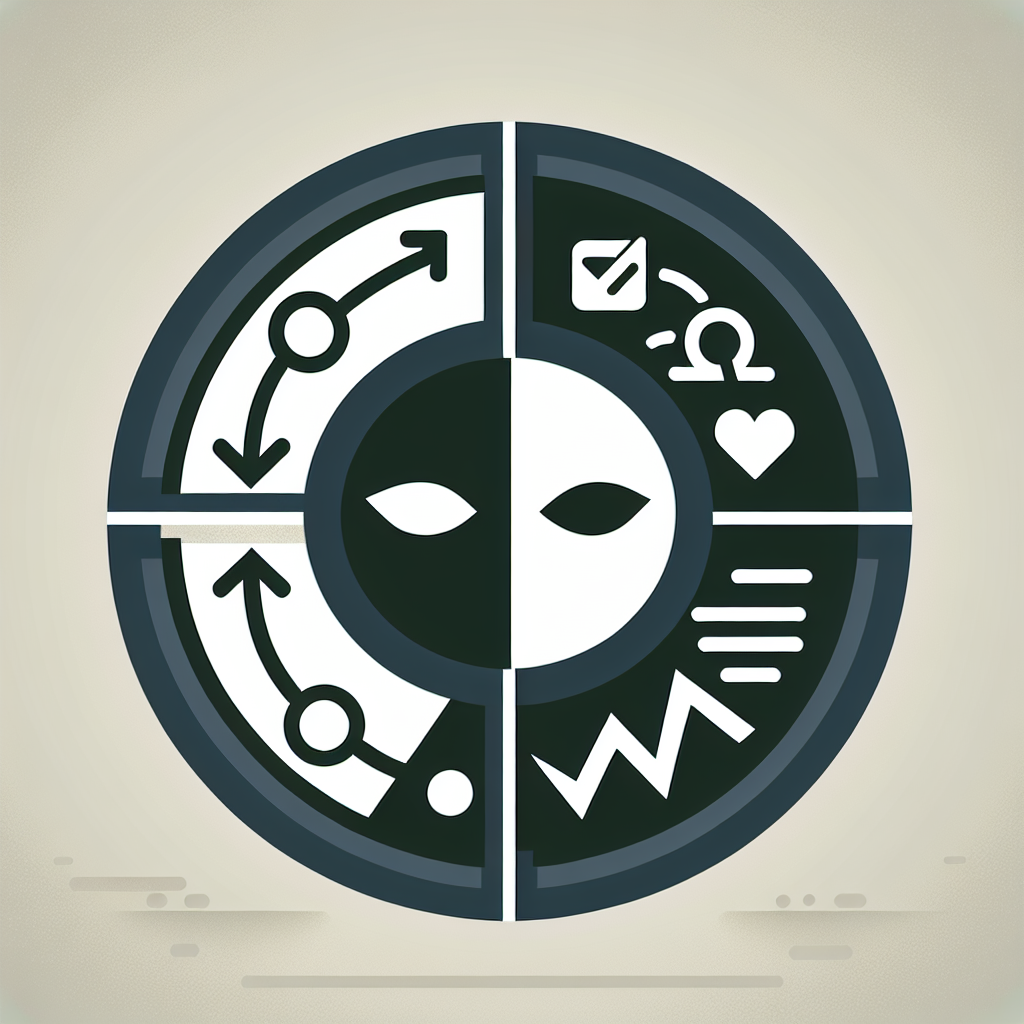
The Role of Dark Patterns in Digital Marketing and Ethical Concerns
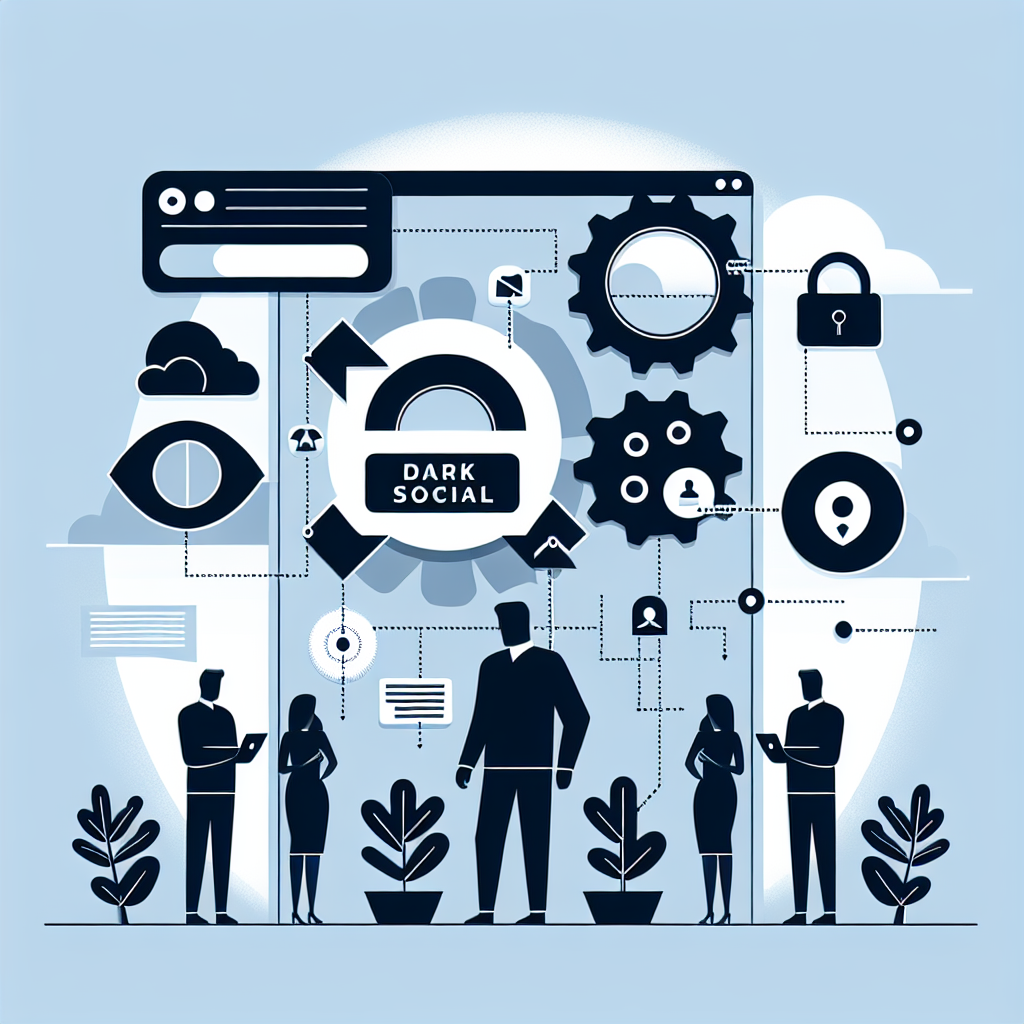
The Rise of Dark Social and Its Impact on Marketing Measurement
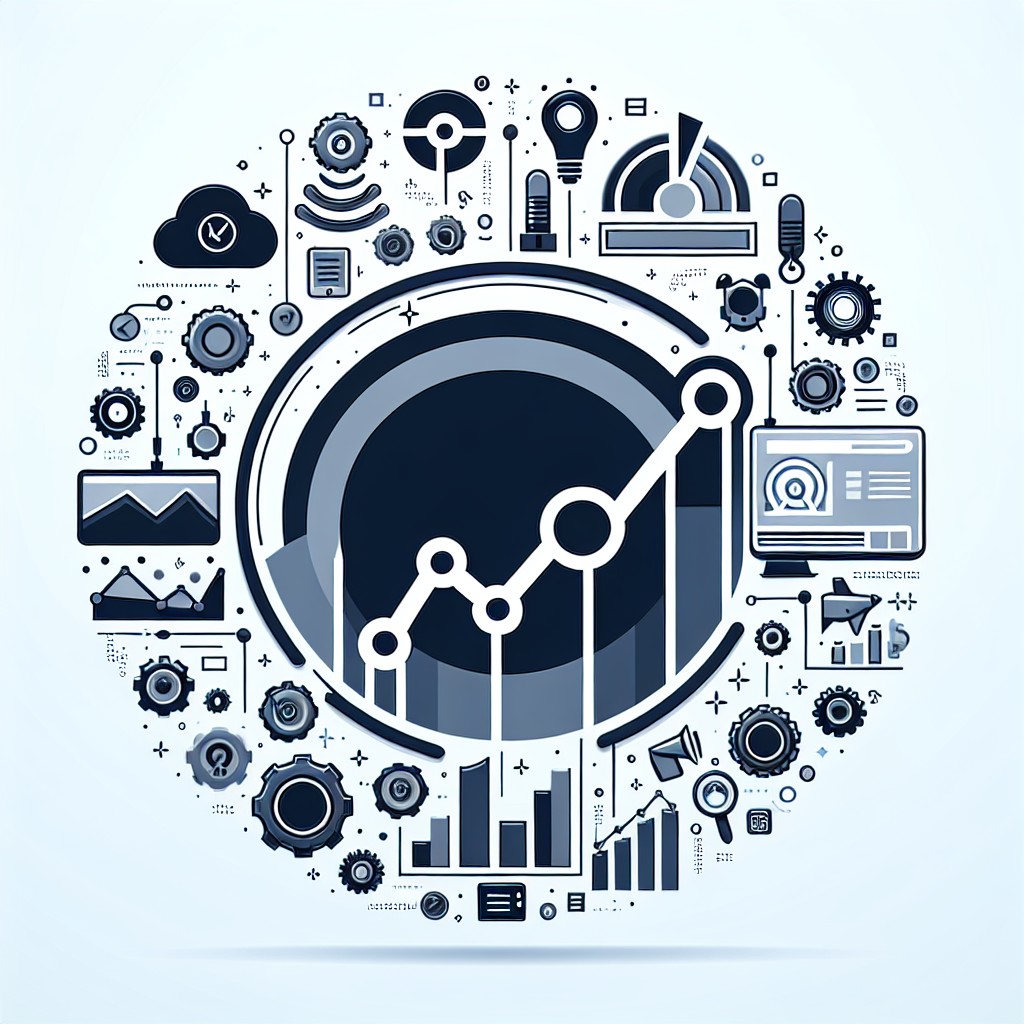
The Future of Retail Media Networks and What Marketers Should Know
Recent Blogs
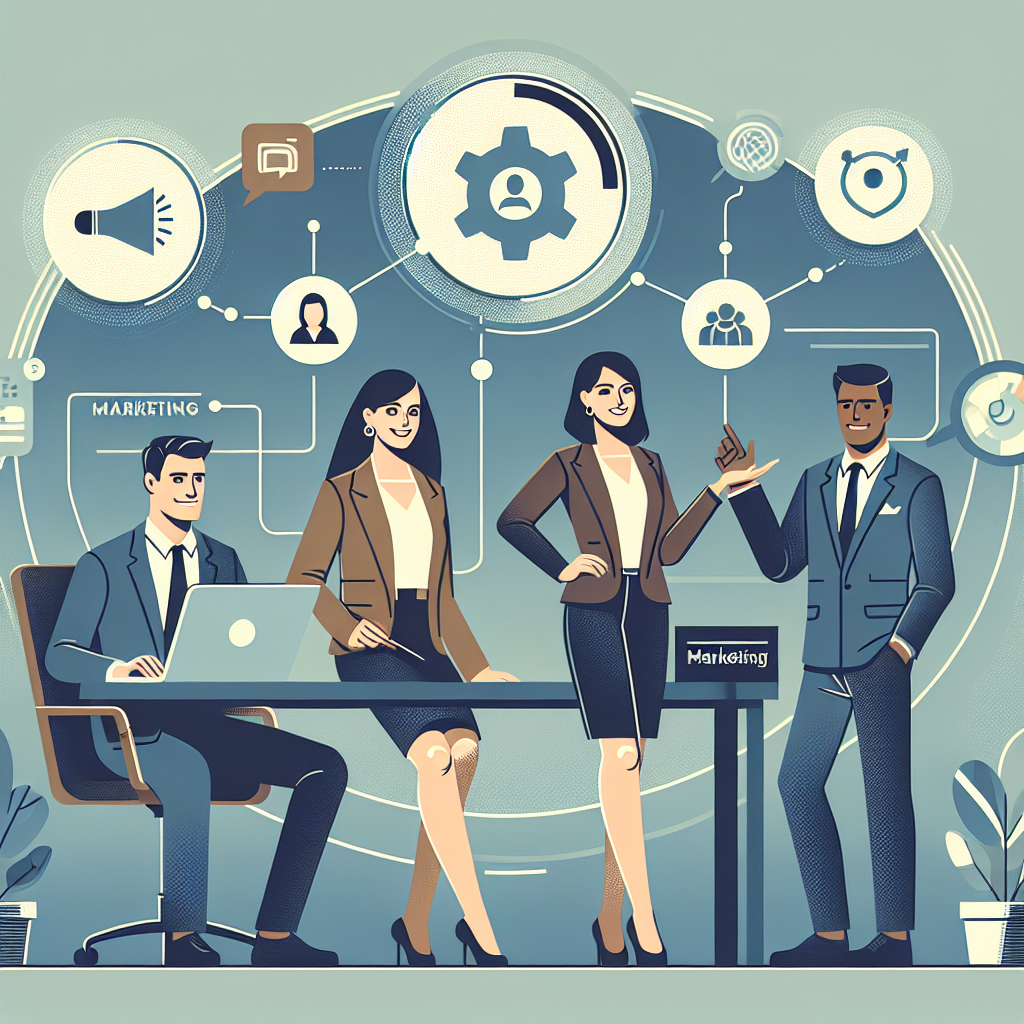
From Service to Experience - Training Teams for the Shift
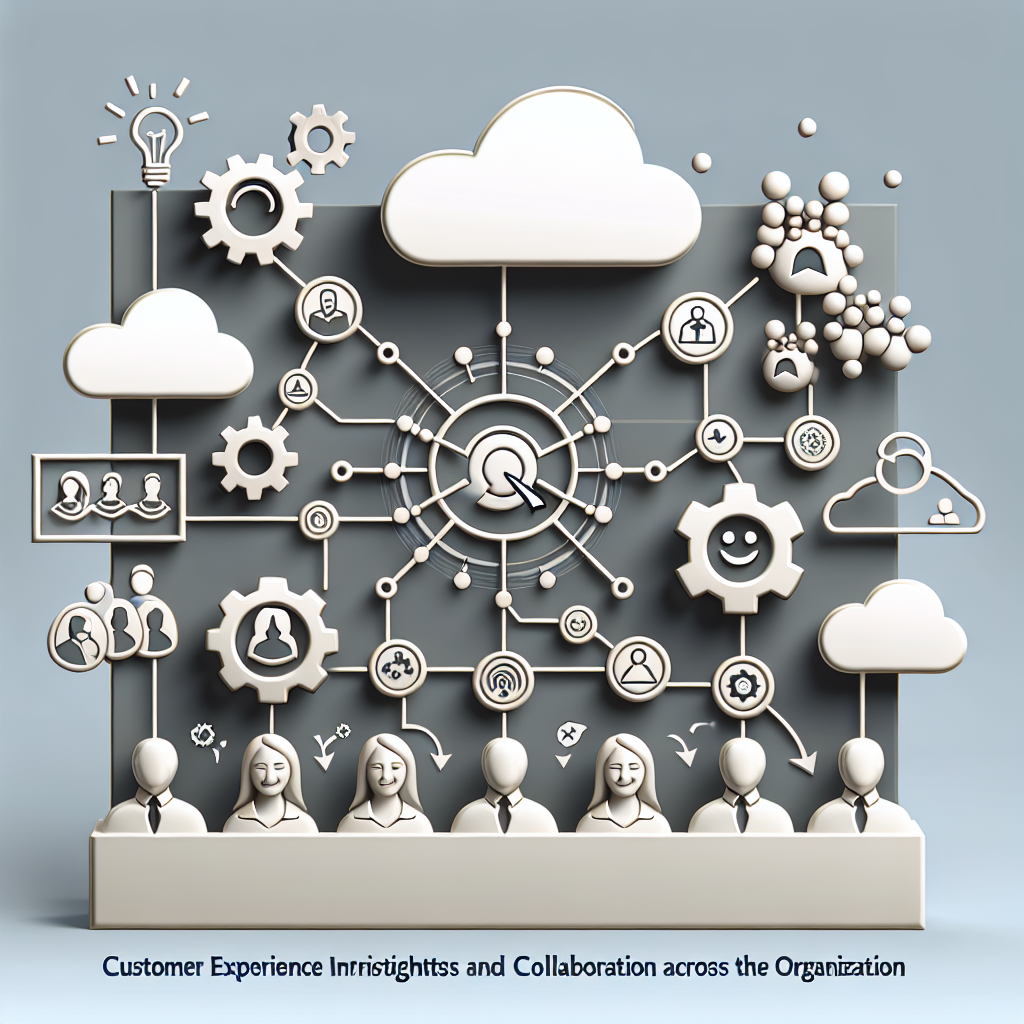
How to Democratize CX Insights Across the Org
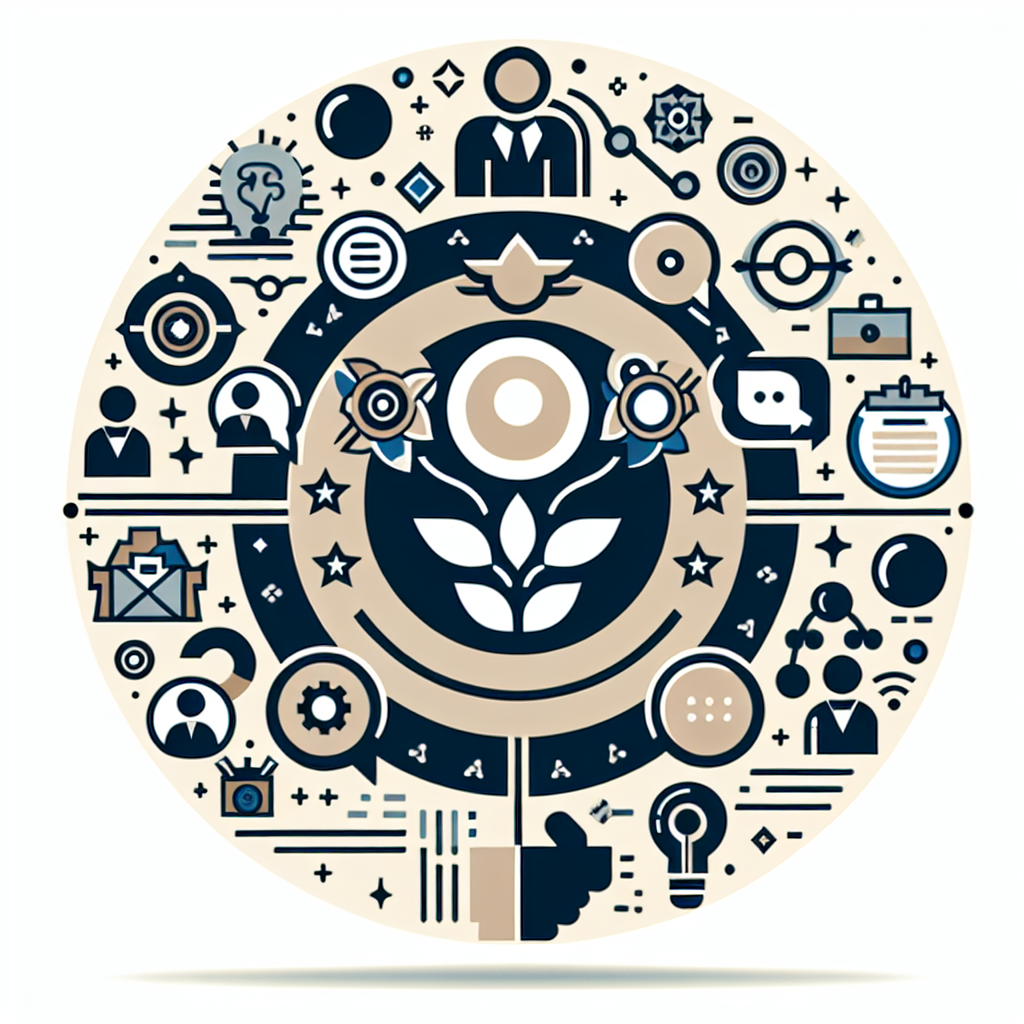
CX is Everyone's Job Building a Customer-Centric Culture
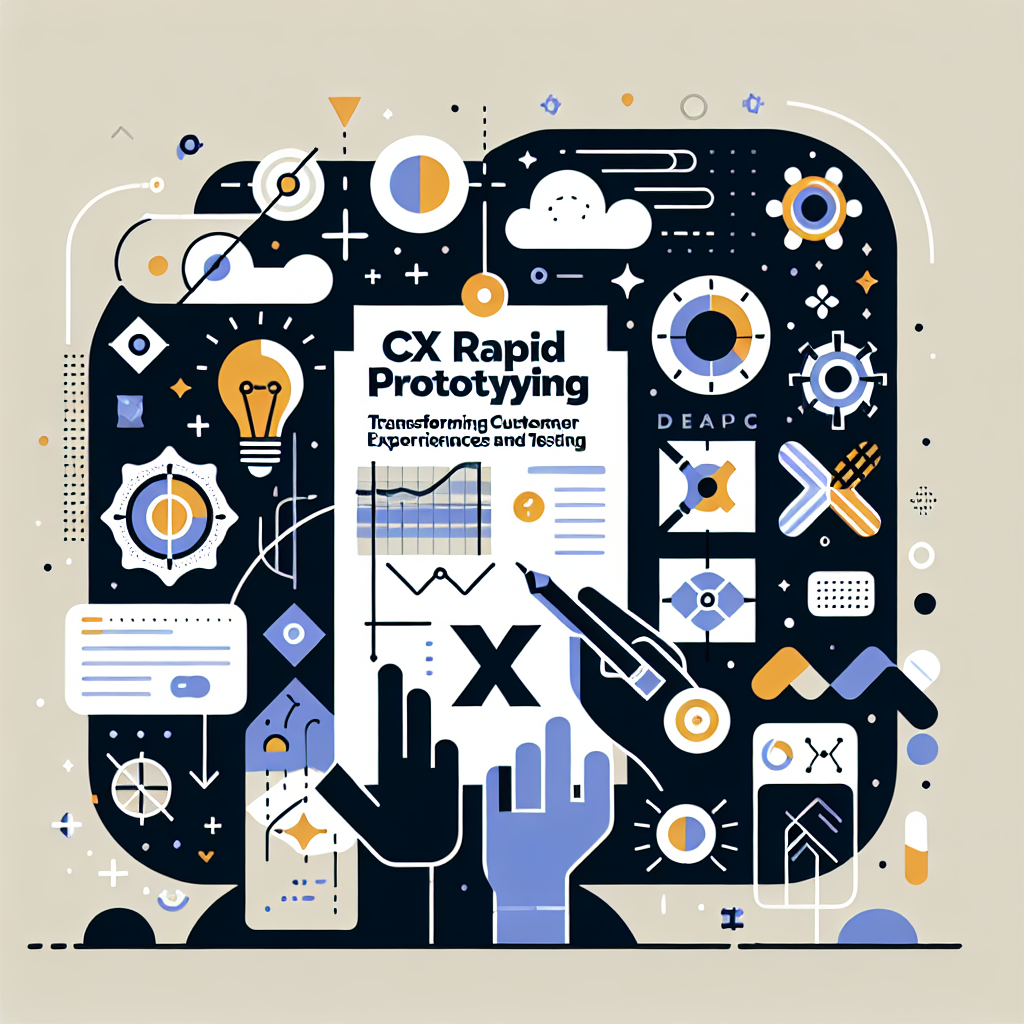
Experimenting with CX Rapid Prototyping for New Journeys
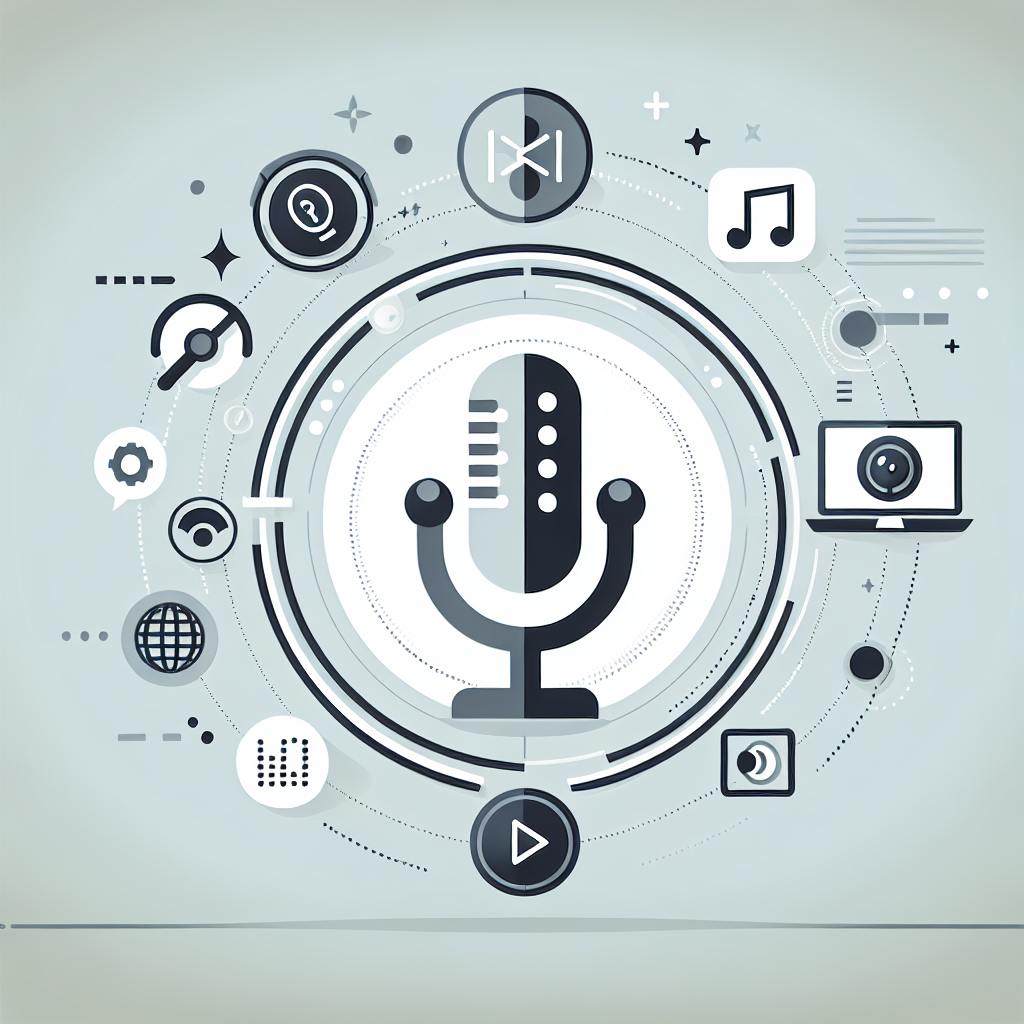
Voice, Video, and Beyond The Multimodal CX Future
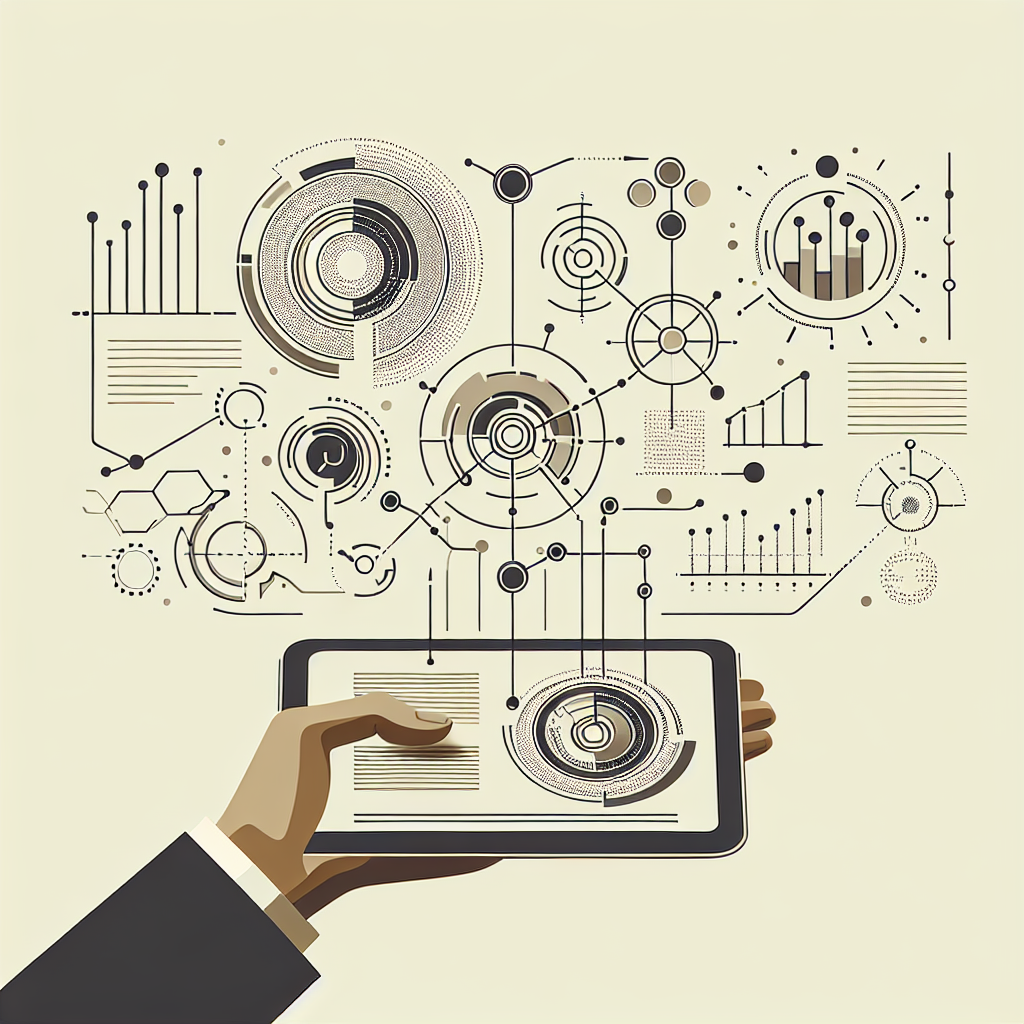