Future-Proofing Your Research Practice
The insight struck Neha during an otherwise routine vendor presentation about research automation. Her team had gathered to evaluate a new AI-powered survey platform, and she was initially skeptical. However, midway through the demonstration, her colleague Wei, who leads the data science team, leaned over and whispered, "This isn't replacing your job—it's removing the parts of your job you hate doing." As the presenter demonstrated how the system automated repetitive analysis, freeing up more time for strategic interpretation, Neha realized Wei was right. The tedious aspects of research—data cleaning, basic correlation analysis, standard reporting—were being automated. Meanwhile, the truly valuable elements—contextual understanding, strategic application, creative problem framing—remained uniquely human domains. That afternoon transformed Neha's understanding of research's future: not a competition between human and machine capabilities, but a partnership where each contributes its strengths. Neha understood that researchers who will thrive are those who embrace technological advancement and redefine their value in a world where data collection and basic analysis become increasingly automated.
Introduction: The Research Revolution
Market research finds itself in a transformational period—caught between established methodologies developed for a more stable, slower-moving consumer landscape and the demands of business environments characterized by unprecedented change velocity. This transformation represents what the Marketing Research Association has termed "the great research recalibration."
The integration of artificial intelligence, automation, and behavioral sciences into research practice is not simply adding new tools to the traditional research toolkit but fundamentally reshaping what research is and how it delivers value. Research from McKinsey indicates that organizations with future-oriented research capabilities respond to market changes 43% faster and demonstrate 28% higher marketing ROI compared to organizations with traditional research frameworks.
As Jay Baer, founder of Convince & Convert, observes: "The future of research is not about finding answers faster but about asking better questions sooner. Speed without direction is just acceleration toward irrelevance."
1. Skillsets Needed
Hybrid Capabilities Beyond Traditional Research
Tomorrow's researchers require multidisciplinary expertise:
- Data science fundamentals and statistical fluency
- Behavioral economics and decision science understanding
- Business strategy integration capabilities
- Technology evaluation and implementation expertise
Consumer packaged goods leader Procter & Gamble has redesigned its researcher career path to require "insight triad" skills—research methodology expertise, data science capabilities, and business strategy understanding—recognizing that single-discipline researchers increasingly struggle to deliver comprehensive value.
From Research Execution to Research Architecture
The evolution from tactician to strategist:
- Research system design rather than project execution
- Continuous intelligence frameworks versus discrete studies
- Multi-method integration capabilities
- Automated and human method orchestration
Global media agency Mindshare has reorganized its insights function from project-based teams to "intelligence architects" who design interconnected research systems that combine continuous automated data collection with strategic human-led inquiry.
Human-AI Collaboration Skills
Developing capabilities for the augmented research era:
- AI-augmented analysis and interpretation
- Human oversight of machine learning systems
- Ethical AI governance in research applications
- Complementary task allocation between human and machine
Technology company Microsoft maintains a dedicated "augmented research team" that specializes in developing collaborative workflows between AI systems and human researchers, reportedly reducing research execution time by 40% while improving strategic impact of findings.
2. Keeping Up With Trends
Methodological Innovation Frameworks
Systematic approaches to research evolution:
- Emerging methodology evaluation protocols
- Test-and-learn programs for new approaches
- Cross-industry methodology scanning
- Academic-commercial research partnerships
Financial services firm Goldman Sachs operates a "research innovation lab" that systematically evaluates emerging research methodologies, testing over 30 novel approaches annually and integrating approximately 15% into standard research practices.
Cross-Disciplinary Knowledge Networks
Expanding research horizons through diverse inputs:
- Behavioral science research integration
- Data science community engagement
- Decision theory and cognitive psychology application
- Complex systems thinking incorporation
Entertainment company Netflix maintains an active "cross-pollination program" that rotates researchers through diverse disciplines including data science, content strategy, and behavioral economics to develop what they call "methodological bilingualism."
Technology Landscape Monitoring
Staying ahead of research technology evolution:
- Research automation platform assessment
- AI and machine learning application evaluation
- Virtual and augmented reality research potential
- Behavioral measurement technology adoption
Automotive manufacturer Toyota employs a dedicated "research technology radar" team that systematically evaluates emerging technologies, maintaining a three-year technology adoption roadmap that is updated quarterly based on new capabilities and organizational needs.
3. Human + AI Partnerships
Complementary Capability Frameworks
Defining optimal human-AI research division of labor:
- Pattern recognition and analysis automation
- Human hypothesis generation and interpretation
- Combined qualitative depth and quantitative scale
- Augmented researcher workflows and tools
Telecommunications leader Verizon has developed a "complementary intelligence model" that explicitly defines which research tasks are assigned to AI systems (pattern detection, linguistic analysis, basic reporting) and which remain human domains (cultural context, strategic application, ethical consideration).
Ethical AI Integration
Ensuring responsible AI application in research:
- Algorithmic bias detection and mitigation
- Transparency in AI-assisted insights
- Ethical boundaries in automated research
- Human oversight and accountability frameworks
Professional services firm Deloitte maintains an "ethical AI in research" framework that requires all automated research systems to meet specific transparency, fairness, and explainability standards before implementation.
Continuous Learning Systems
Building ever-improving research capabilities:
- Human-supervised machine learning
- Knowledge management and institutional memory
- Cross-method validation frameworks
- Insight synthesis across methodologies and data sources
E-commerce leader Amazon has implemented what they call "research memory systems" that not only store past research findings but actively connect them to new insights, creating an ever-expanding knowledge network that reportedly improves insight quality by 23% compared to isolated studies.
Augmented Researcher Tools
Enhancing human capabilities through technology:
- Natural language processing for qualitative analysis
- Automated pattern recognition and anomaly detection
- Predictive analytics for hypothesis generation
- Real-time insight distribution systems
Consumer insights firm Kantar has developed a suite of "researcher amplification tools" that automate routine analysis tasks while enhancing researchers' ability to identify patterns across disparate data sources, reportedly increasing researcher productivity by 35%.
Conclusion: The Collaborative Future of Research
As technology continues transforming the research landscape, the future belongs not to organizations that simply adopt new tools but to those that fundamentally reimagine the relationship between human insight and technological capability. The most effective research functions are evolving from project executors to strategic intelligence systems—combining technological scale with uniquely human capabilities for context, creativity, and strategic application.
The research leaders who will thrive are developing what might be called "collaborative intelligence"—the ability to seamlessly integrate human and machine capabilities into systems greater than either could achieve alone. As the pace of market change continues accelerating, this integration offers the only viable path to research that delivers both the speed and depth modern organizations require.
Call to Action
For research leaders looking to build future-ready capabilities:
- Invest in developing hybrid researcher skill sets that span methodology, data science, and business strategy
- Create systematic approaches to evaluating and adopting emerging research technologies
- Develop explicit frameworks for optimal human-AI task allocation and collaboration
- Build continuous learning systems that connect insights across methodologies and time periods
- Foster research cultures that embrace technological augmentation rather than fearing automation
The future belongs not to those with the largest research budgets or the most advanced technologies, but to those who most effectively combine technological scale with human insight—creating research practices capable of delivering both speed and depth in an increasingly complex and rapidly changing market environment.
Featured Blogs
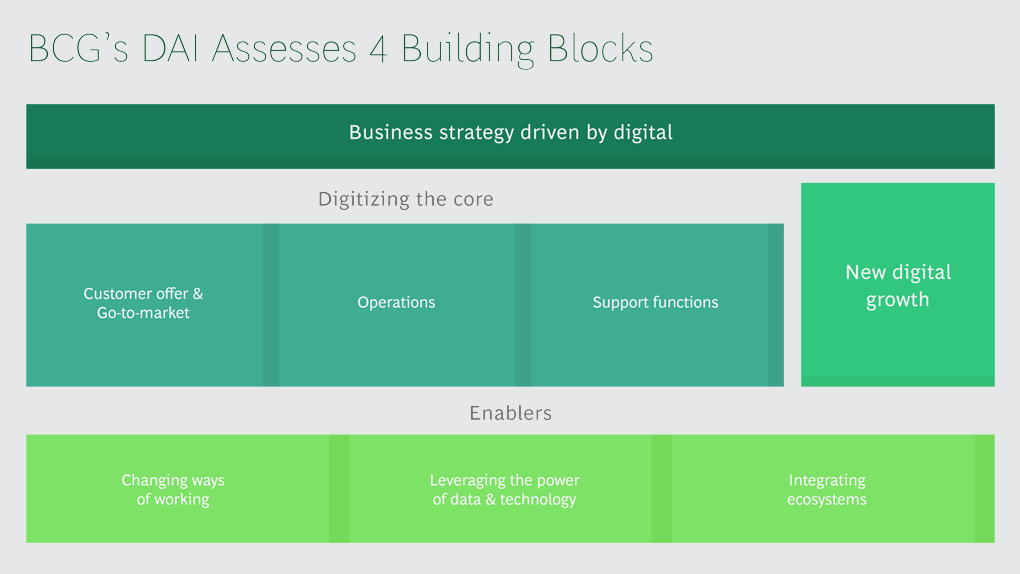
BCG Digital Acceleration Index
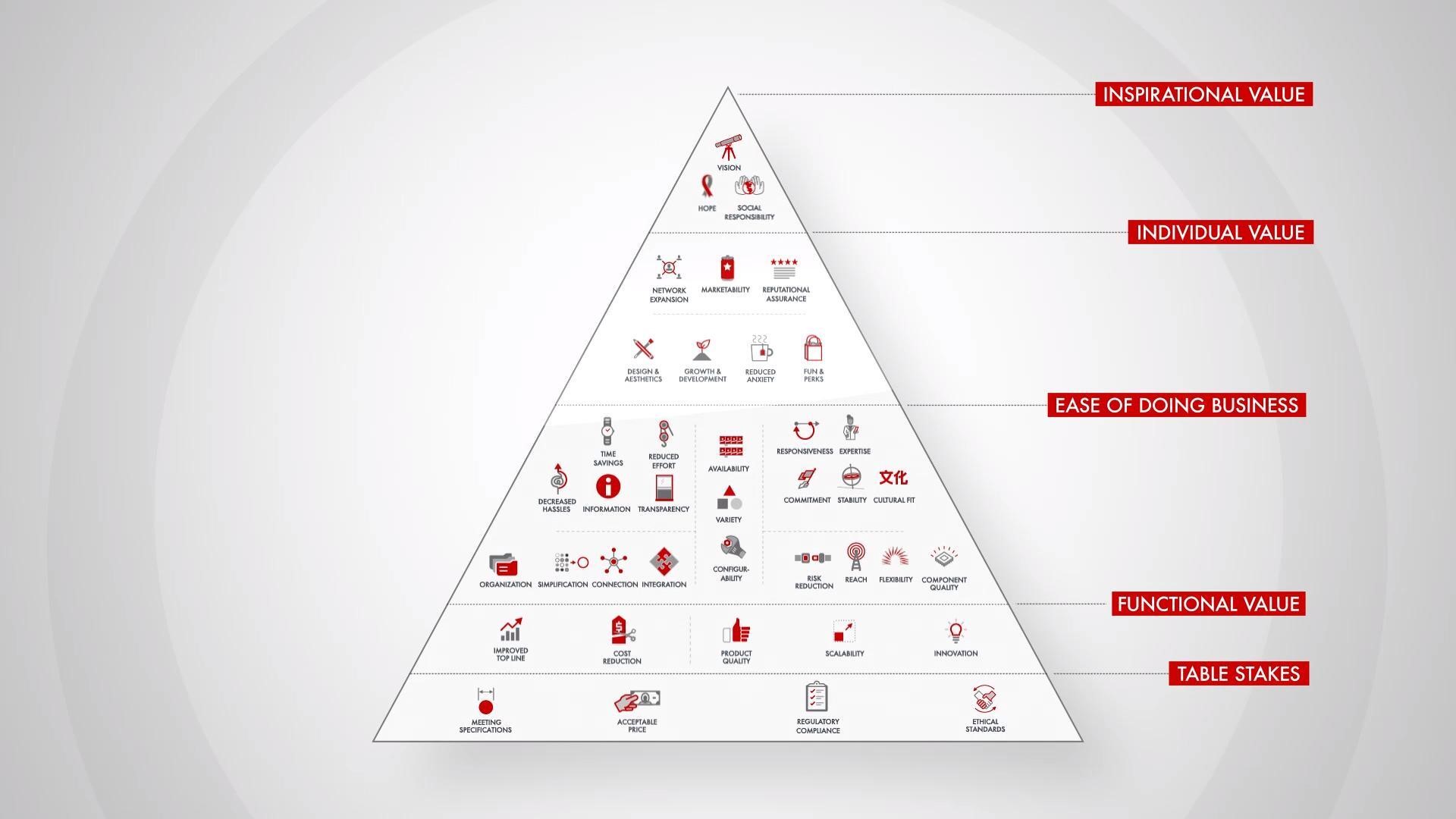
Bain’s Elements of Value Framework
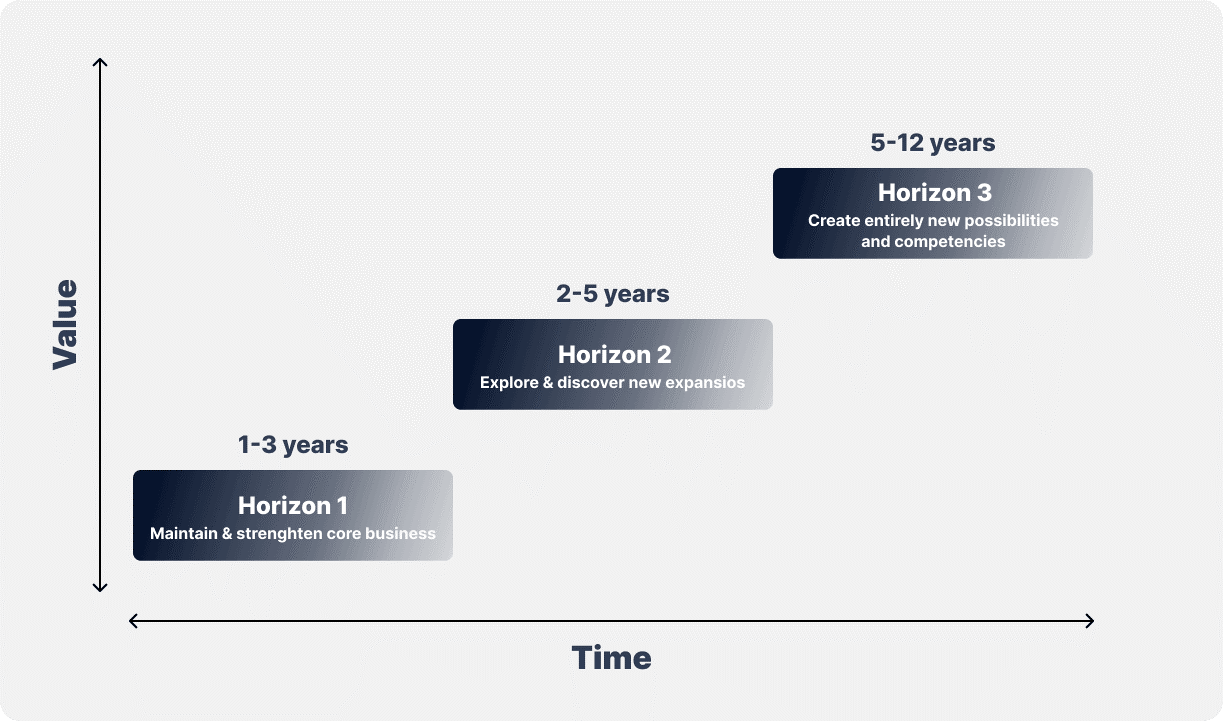
McKinsey Growth Pyramid
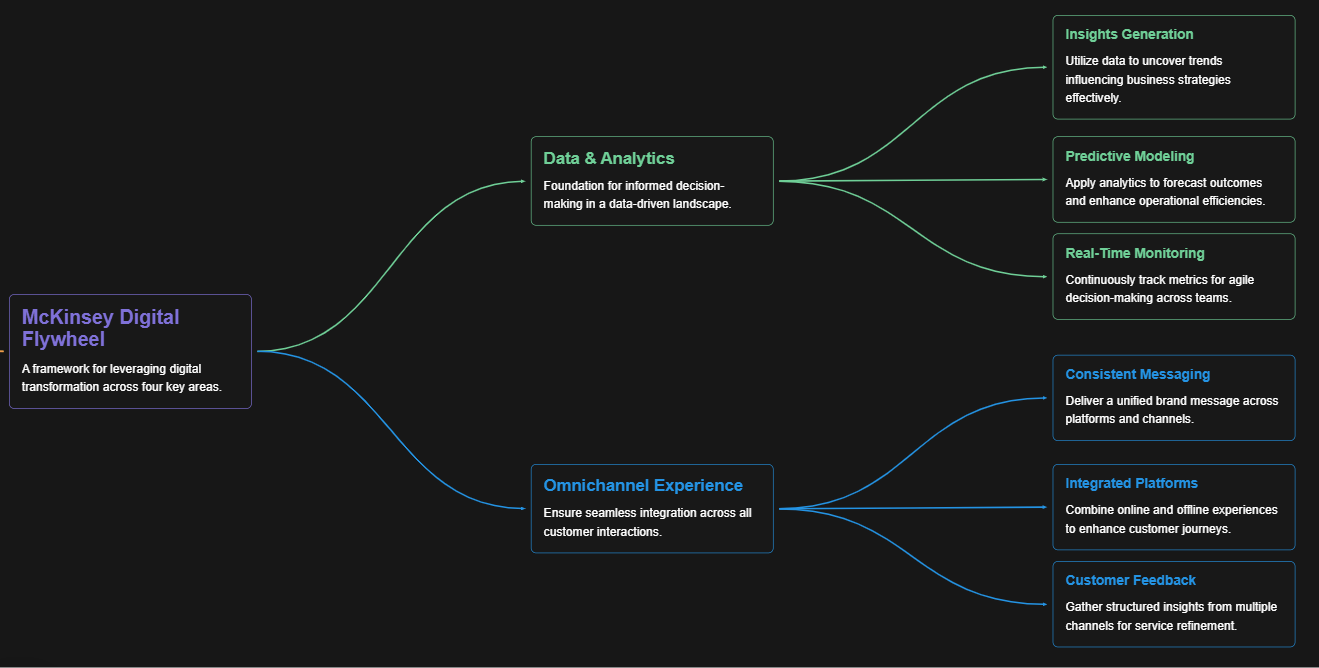
McKinsey Digital Flywheel
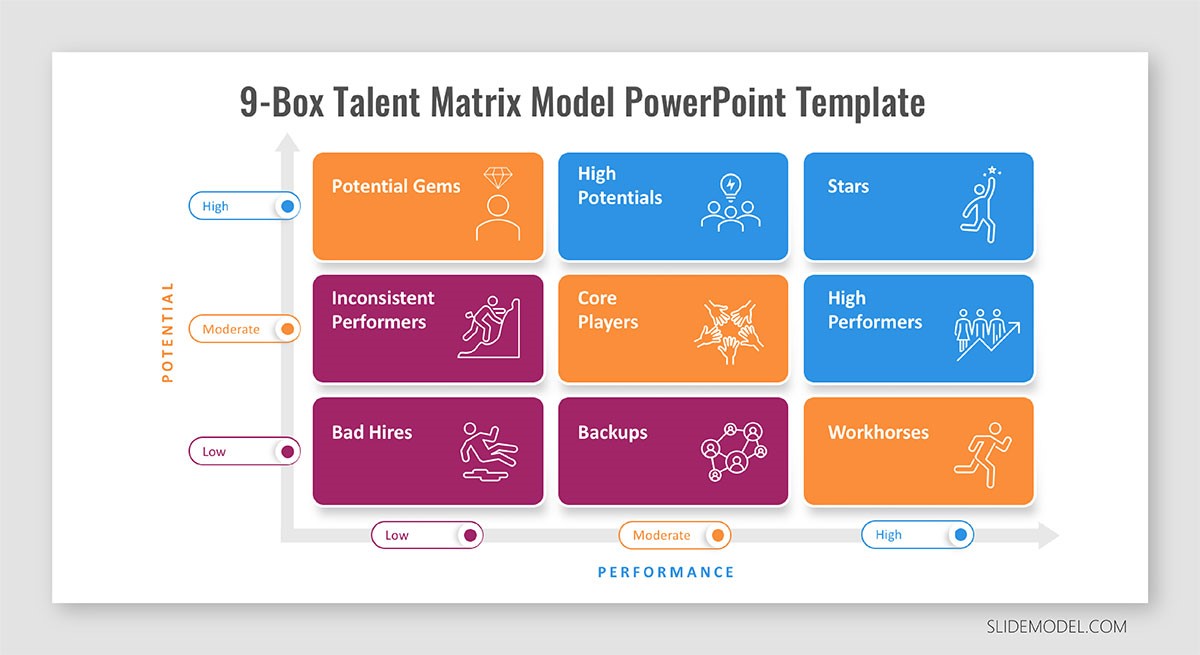
McKinsey 9-Box Talent Matrix
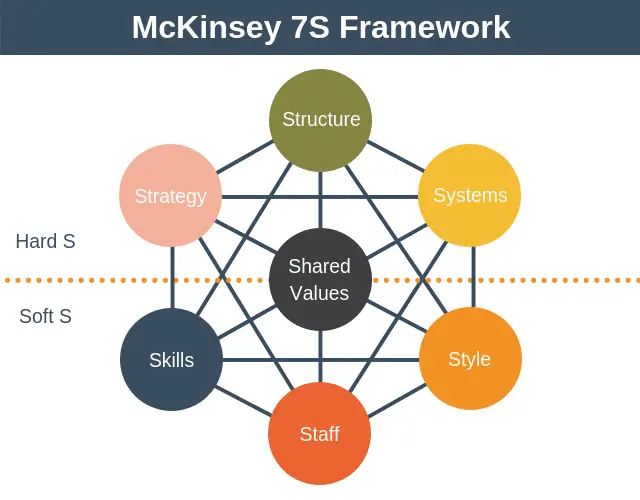
McKinsey 7S Framework
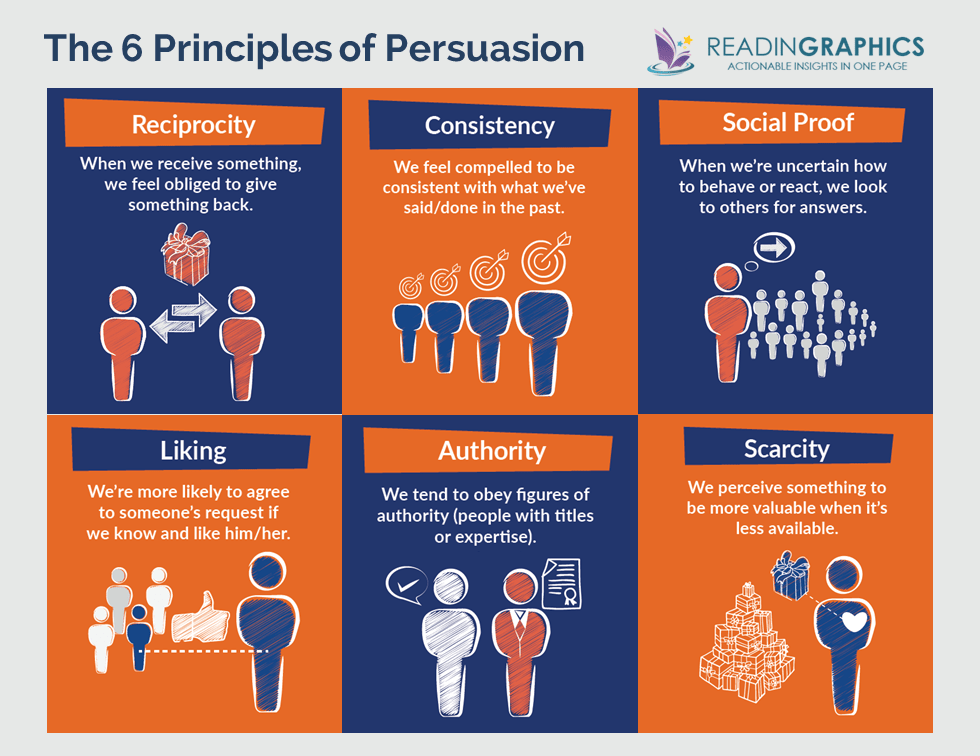
The Psychology of Persuasion in Marketing
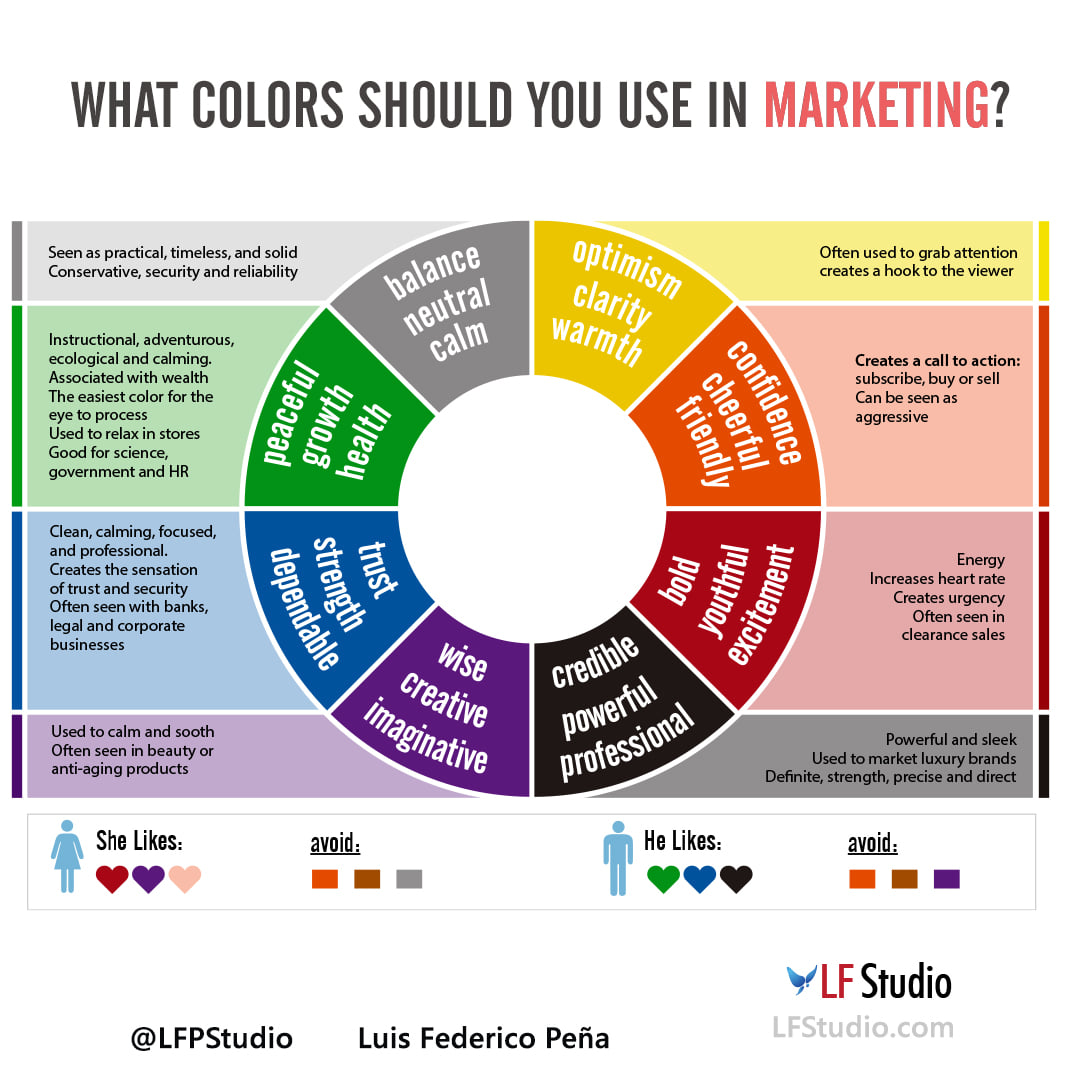
The Influence of Colors on Branding and Marketing Psychology
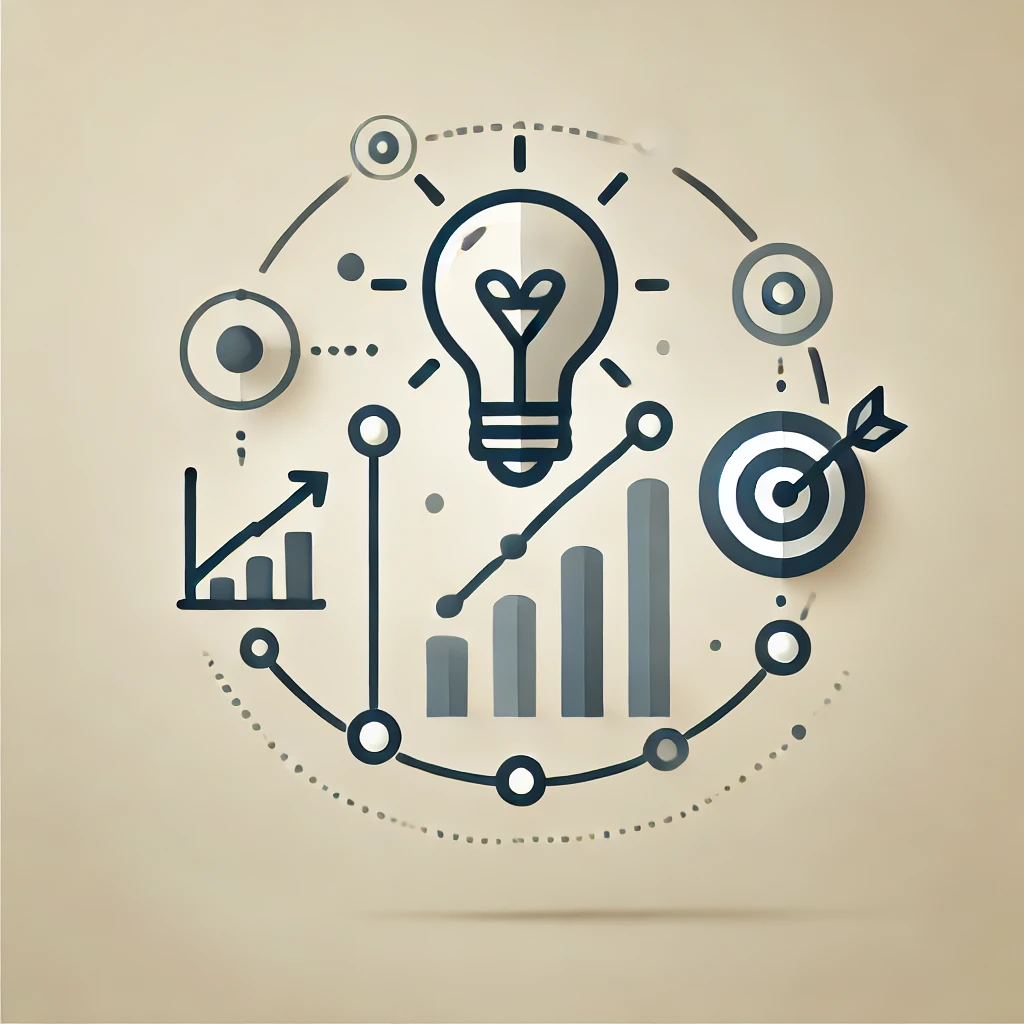