Passive Data Collection in Consumer Behavior
The insight came to Neha during a routine fieldwork observation last summer. As she shadowed a participant in a traditional ethnographic shopping study, she noticed the participant frequently checking a fitness tracker. Curious about its influence on the participant's shopping decisions, Neha inquired further. The participant's response was eye-opening: "My app tracks where I walk in stores, how long I browse, even my heart rate when I consider expensive purchases—and I've given three different companies permission to use that data." This casual conversation highlighted the profound methodological shift occurring in marketing research. While significant resources were dedicated to observing behavior, devices were already passively collecting incredibly rich behavioral data with the participant's explicit consent. This moment prompted Neha to reevaluate fundamental assumptions about studying consumer behavior and the ethical boundaries of emerging methodological approaches.
Introduction: The Passive Collection Revolution
Marketing research methodology has traditionally relied heavily on participants' conscious engagement—whether responding to surveys, participating in interviews, or knowingly being observed in ethnographic studies. This paradigm is being rapidly transformed by the emergence of passive data collection methodologies that continuously gather behavioral information without requiring active participant engagement beyond initial consent.
According to the Journal of Marketing Analytics, passive data collection methodologies now account for 47% of all consumer behavior data utilized by Fortune 500 marketers, compared to just 12% five years ago. This shift reflects both technological capabilities and a fundamental reconsideration of research validity—as stated behaviors often diverge significantly from actual behaviors, with the MSI (Marketing Science Institute) estimating that stated preferences explain only 21% of variance in actual purchase behavior.
This examination explores the methodological implications, applications, and ethical considerations surrounding the integration of passive data collection into modern marketing research practice.
1. Mobile Sensors, Browsing Data
The technological infrastructure enabling passive behavioral data collection continues rapid evolution:
a) Mobile Device Sensory Data
Smartphones and wearables generate unprecedented behavioral insights:
- Location tracking revealing physical movement patterns
- Accelerometer data capturing handling and activity levels
- Proximity sensors identifying environmental contexts
- Biometric measurements indicating emotional responses
Retailer Target partnered with a mobile research panel collecting opt-in location data, identifying that 23% of customers who claimed to "comparison shop extensively" actually visited zero competitor locations before major purchases.
b) Digital Journey Tracking
Online behavioral tracking provides comprehensive digital visibility:
- Click patterns revealing decision processes
- Dwell time indicating interest and consideration depth
- Search behavior exposing information gathering strategies
- Cart abandonment patterns highlighting purchase barriers
Financial services provider Chase implemented passive journey tracking with permission-based screen recording, discovering that prospective credit card customers spent three times longer reviewing fee structures than interest rates, despite stating the opposite in surveys.
c) Cross-Platform Integration
Connecting behaviors across physical and digital environments:
- Online-to-offline journey mapping
- Cross-device behavior tracking
- Multichannel purchase pathway analysis
- Environmental context correlation with digital behavior
Sports apparel brand Nike developed an opt-in research program integrating mobile location data with digital browsing history, revealing that 78% of eventual in-store purchasers conducted specific product research within two hours of store visits—a phenomenon invisible to traditional research methods.
d) Internet of Things Applications
Connected devices create new passive data streams:
- Smart speaker interaction patterns
- Connected appliance usage behavior
- Automotive telematics revealing travel patterns
- Smart TV viewership and engagement metrics
Consumer packaged goods company Procter & Gamble utilized smart packaging sensors in an opt-in research program, identifying that consumers used their products in entirely different patterns than reported in diaries or surveys—applying products at different times and in different quantities.
2. Consent and Ethical Use
The ethical implementation of passive methodologies requires careful consideration:
a) Informed Consent Frameworks
Ethical collection demands transparency and control:
- Clear communication of data types being collected
- Specific disclosure of research purposes
- Granular permission options for different data streams
- Straightforward withdrawal mechanisms
E-commerce platform Etsy pioneered a tiered consent model for their passive data program, allowing participants to selectively permit different tracking types—resulting in 83% accepting basic browsing tracking but only 41% allowing emotional response monitoring.
b) Anonymization and Security Protocols
Responsible data handling practices protect participant privacy:
- Immediate personal identifier removal
- Aggregate-only analysis where appropriate
- End-to-end encryption for sensitive information
- Strict time limitations on data retention
Technology company Microsoft implemented a "privacy by design" research framework requiring all passive data to undergo three-stage anonymization before researcher access, with systems automatically deleting identifiable elements.
c) Value Exchange Considerations
Ethical programs provide meaningful participant benefits:
- Fair compensation relative to data sensitivity
- Personal insight provision from collected data
- Service improvements directly benefiting participants
- Transparency regarding commercial applications
Media company NBCUniversal created a "data dividend" model for their passive viewing panel, providing participants both monetary compensation and personalized viewing insights based on their own data, establishing what the ARF (Advertising Research Foundation) recognized as an industry-leading ethical framework.
3. Triangulation with Stated Data
Methodological integration maximizes research validity:
a) Multi-Method Research Designs
Combined approaches overcome individual methodology limitations:
- Sequential designs with passive data informing qualitative exploration
- Concurrent tracking validating self-reported behaviors
- Longitudinal integration revealing behavior evolution over time
Media entertainment company Netflix implemented a research program combining passive viewing data with periodic in-depth interviews, discovering that participants systematically misremembered viewing duration by an average of 31% and entirely forgot watching specific content types despite significant time investment.
b) Behavioral-Attitudinal Integration
Connecting what consumers do with why they do it:
- Passive behavioral patterns triggering attitudinal inquiry
- Stated preferences contextualized by actual behaviors
- Unconscious behaviors revealed through passive tracking
- Attitudinal measurement calibrating behavioral interpretation
Automotive manufacturer Toyota developed a research framework integrating passive vehicle usage data with targeted micro-surveys, revealing that drivers who claimed "safety features" as primary purchase motivators rarely engaged with those features in actual driving—fundamentally reshaping their value proposition messaging.
c) Algorithmic Insight Generation
Advanced analytics extracting meaning from behavioral data:
- Pattern recognition identifying behavioral segments
- Anomaly detection revealing unexpected consumer actions
- Predictive modeling forecasting future behaviors
- Attribution analysis connecting behaviors to outcomes
Retail banking institution HSBC implemented machine learning analysis of passively collected mobile banking data, identifying seven distinct behavioral segments invisible to traditional research—including a high-value segment of "night-time financial planners" who showed distinctive product needs despite demographic similarity to other segments.
Conclusion: The Behavioral Data Future
The integration of passive data collection methodologies into marketing research practice represents not merely a technological evolution but a fundamental reconceptualization of consumer understanding. By capturing actual behaviors rather than stated intentions, these approaches provide unprecedented insight into the often unconscious or unarticulated aspects of consumer decision-making.
The most sophisticated research organizations now operate what might be called "behavioral insight systems"—ecosystems of passive data collection, traditional research methods, and advanced analytics that together provide a comprehensive understanding impossible through any single methodology. These systems acknowledge both the limitations of traditional approaches in capturing actual behavior and the contextual limitations of passive data in explaining underlying motivations.
As passive methodologies continue developing, the most successful organizations will be those that establish both technological capabilities and ethical frameworks ensuring these powerful approaches benefit both businesses and consumers through respectful, transparent practices.
Call to Action
For marketing researchers seeking to responsibly integrate passive methodologies:
- Develop comprehensive ethical frameworks establishing clear boundaries for data collection and use
- Create transparent consent processes that truly inform participants rather than merely securing legal compliance
- Invest in secure technical infrastructure specifically designed for sensitive behavioral data
- Establish systematic integration between passive collection and traditional methodological approaches
- Implement participant value exchange models ensuring fair compensation for valuable behavioral data
The future belongs to organizations that leverage passive data's unprecedented behavioral insights while maintaining the ethical standards and research expertise necessary to transform raw behavioral data into meaningful consumer understanding.
Featured Blogs
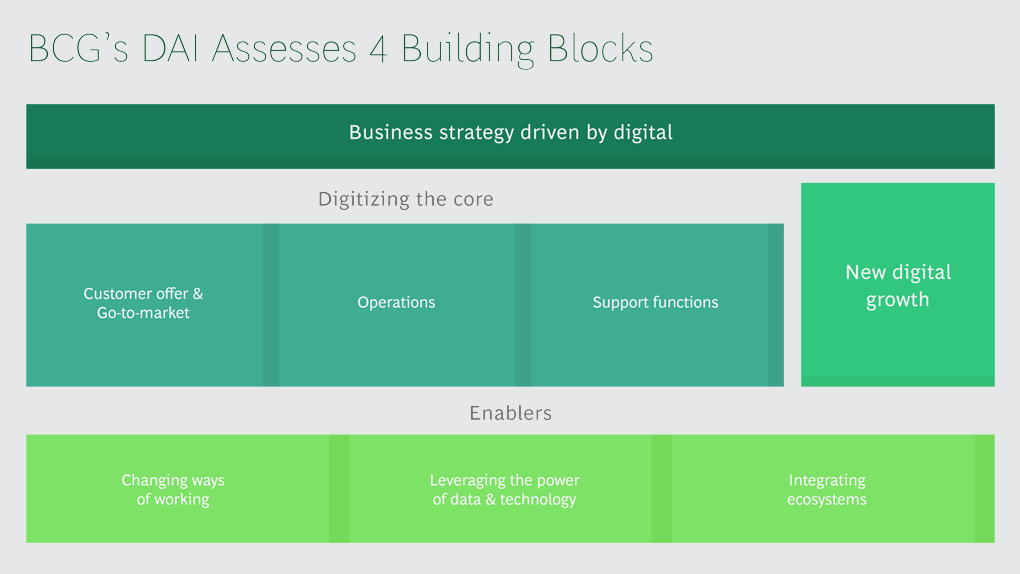
BCG Digital Acceleration Index
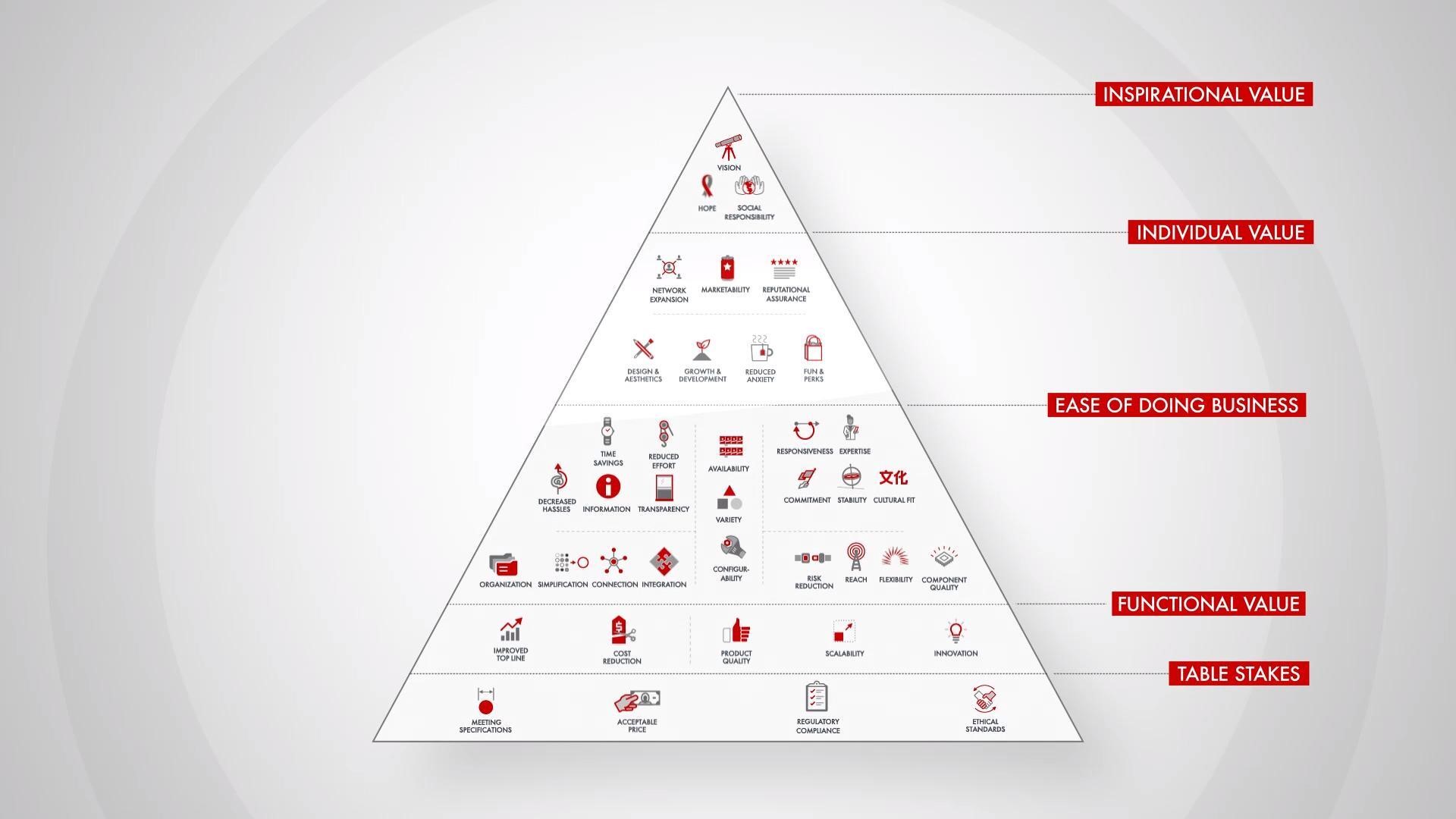
Bain’s Elements of Value Framework
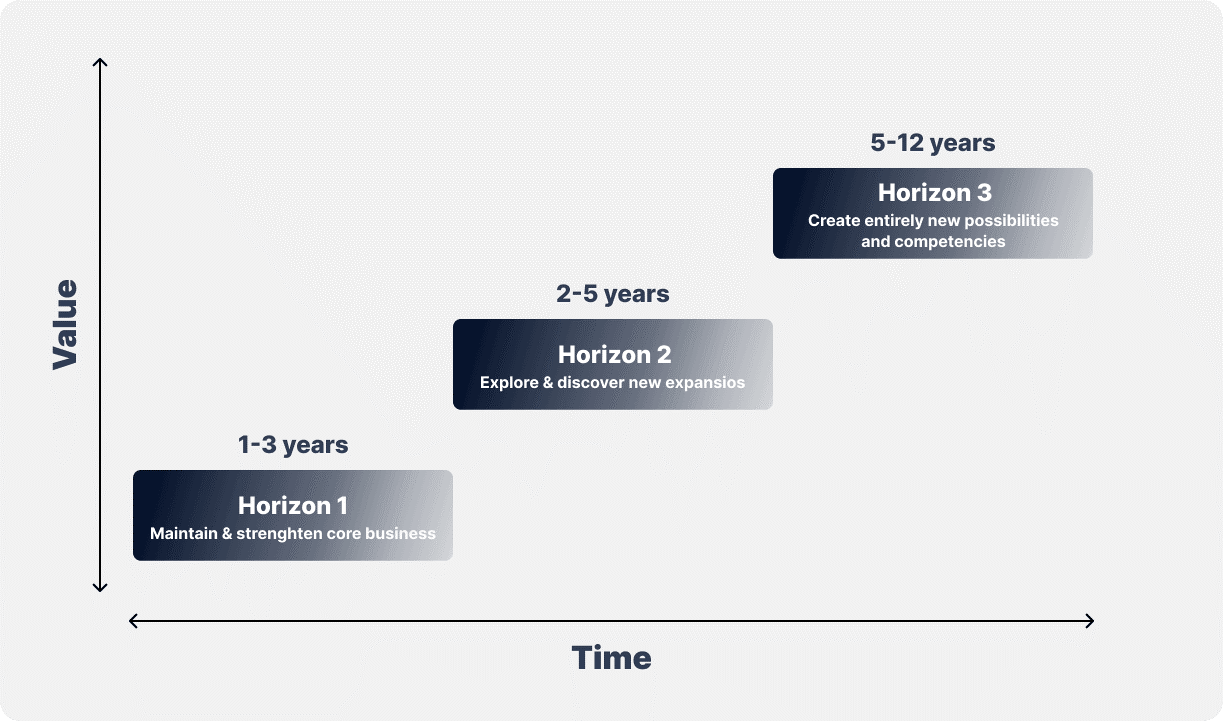
McKinsey Growth Pyramid
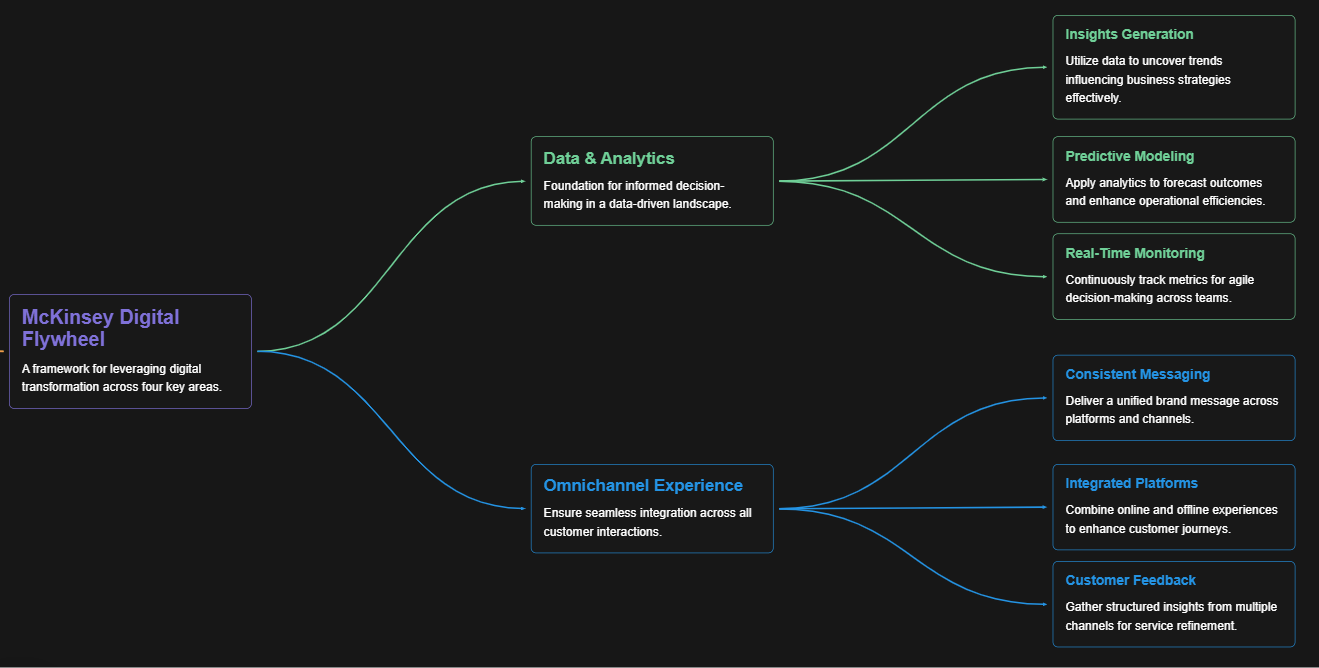
McKinsey Digital Flywheel
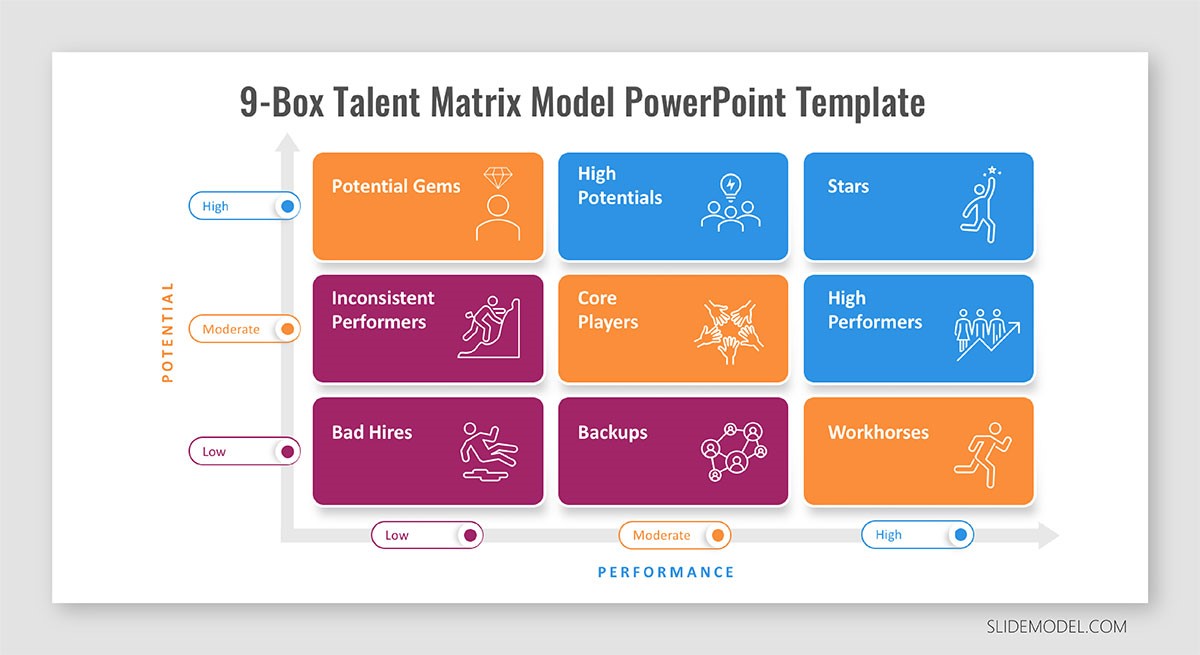
McKinsey 9-Box Talent Matrix
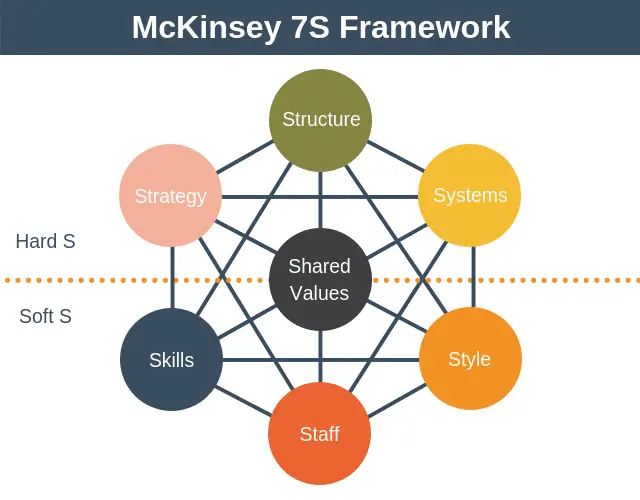
McKinsey 7S Framework
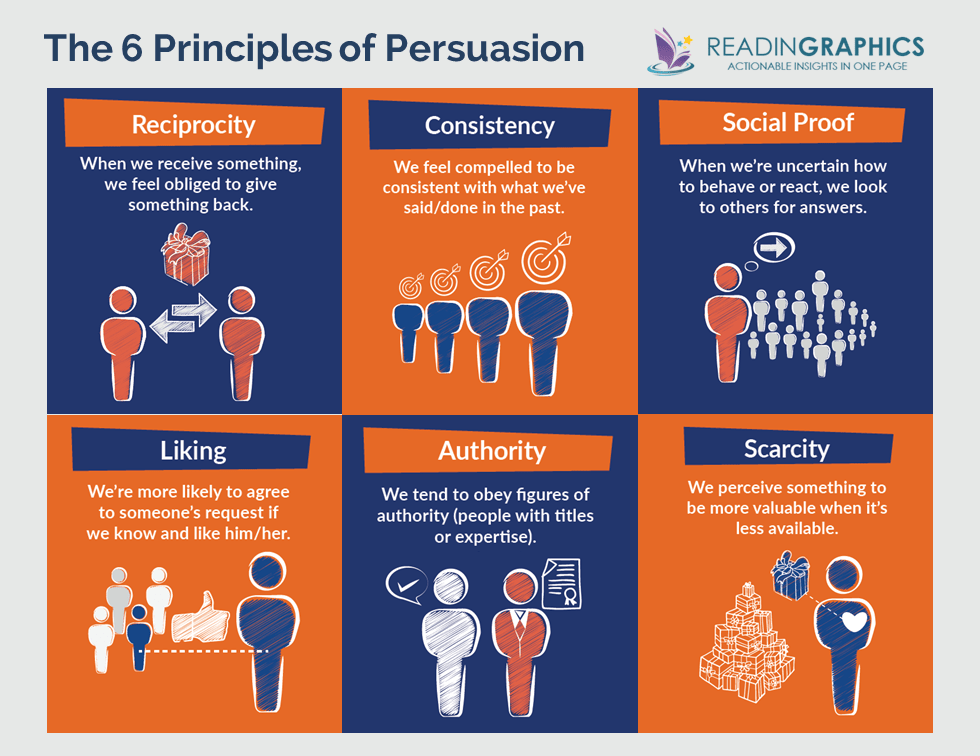
The Psychology of Persuasion in Marketing
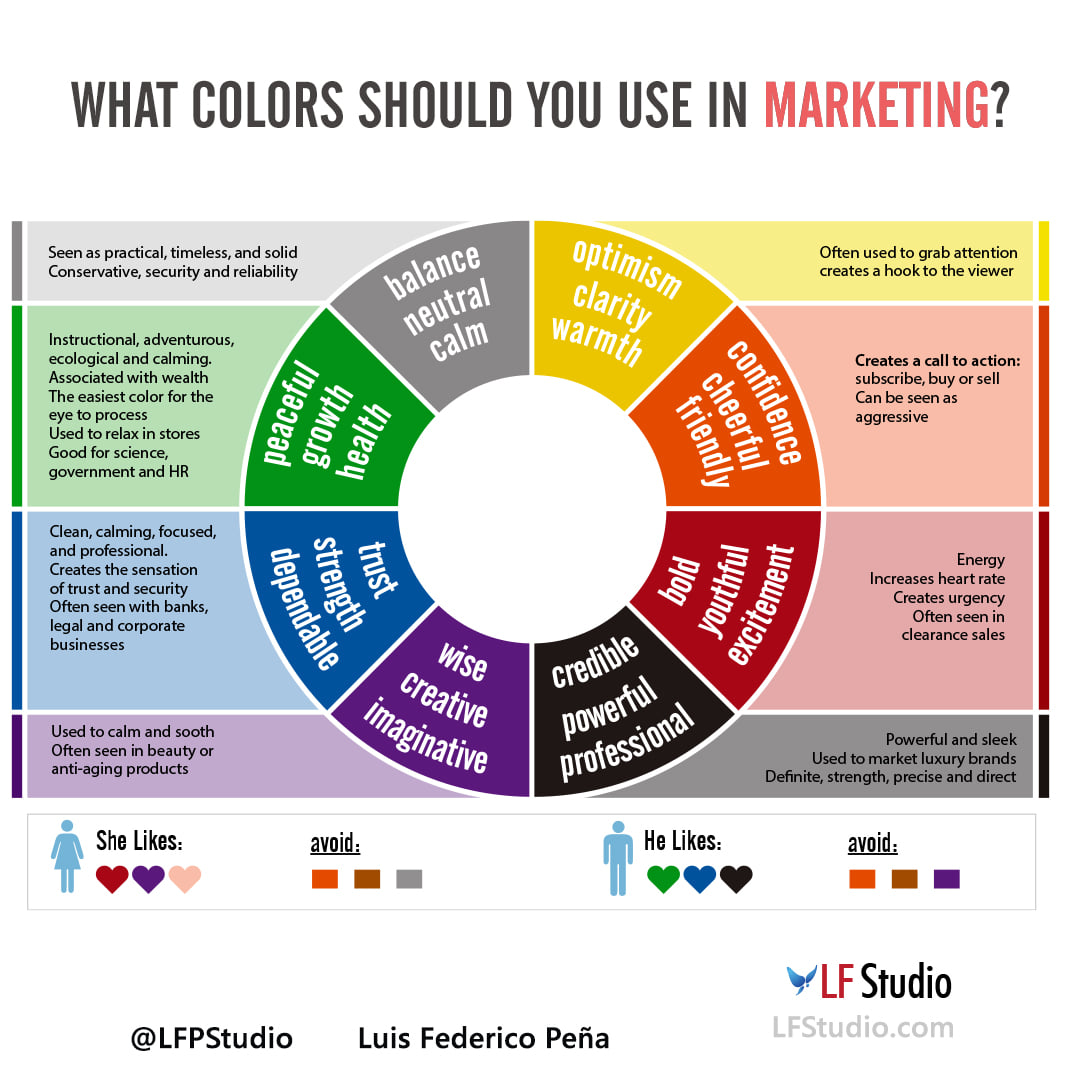
The Influence of Colors on Branding and Marketing Psychology
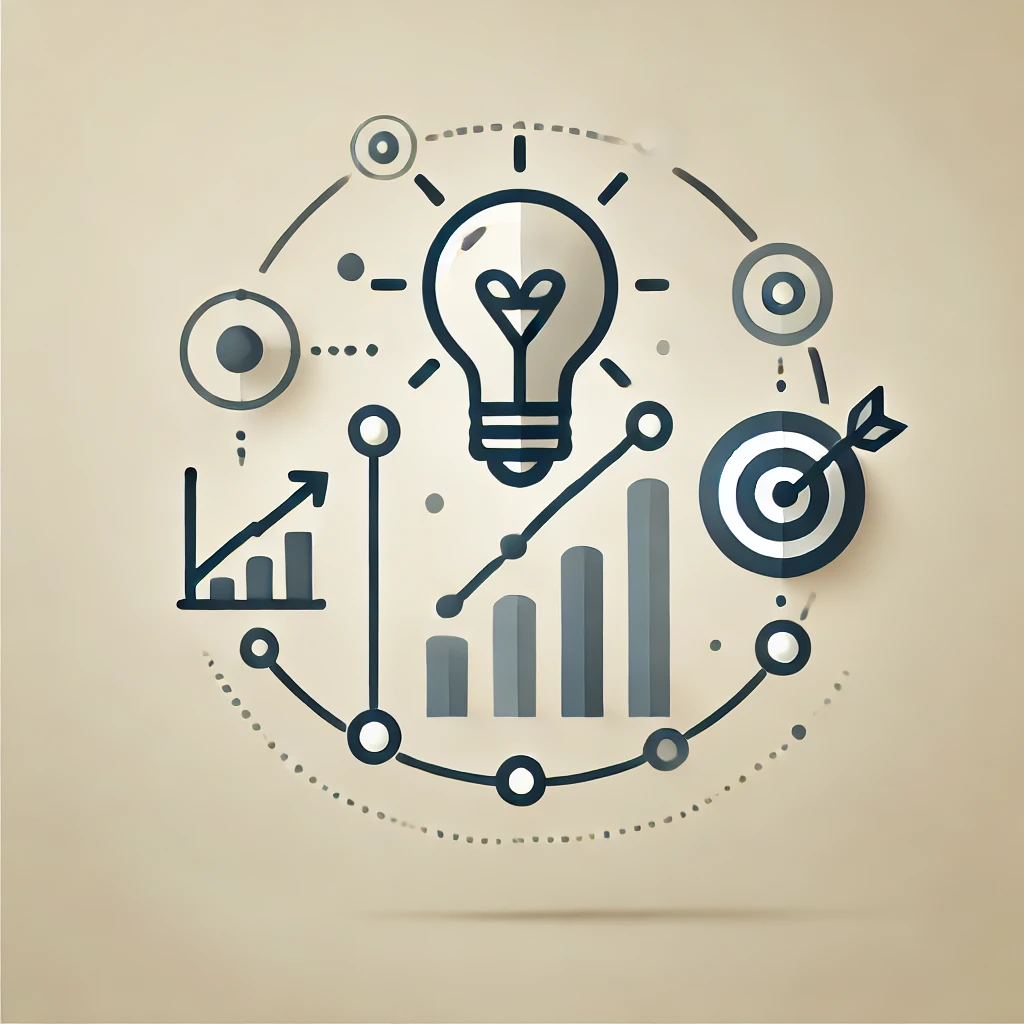