Common Biases in Consumer Research & How to Avoid Them
The revelation struck Neeraj during a routine research review meeting. While presenting findings from the quarterly brand tracking study to the executive team, the skeptical CFO raised a concern. "These brand perception scores seem suspiciously high compared to our actual market performance," he observed. That evening, Neeraj revisited their methodology and made an uncomfortable discovery: the survey design had inadvertently suggested what constituted "good" answers, and the sampling systematically excluded less enthusiastic customer segments. Despite their rigorous analysis, the research foundation was marred by fundamental biases, leading them to perceive what they wanted to see rather than confronting market reality. This humbling experience transformed Neeraj’s approach to consumer research, highlighting how even experienced researchers can fall prey to insidious biases that distort decision-critical insights.
Introduction: The Hidden Influence of Research Bias
Research bias represents one of the most persistent and damaging challenges in marketing research. Even methodologically sophisticated studies can produce misleading results when underlying biases remain unaddressed. According to the Marketing Research Association, approximately 83% of professional researchers acknowledge having identified significant biases in their work after publication, often too late to inform the decisions their research was meant to guide.
The consequences extend beyond academic concerns into material business impact. Analysis from the Journal of Marketing reveals companies making decisions based on biased research experience 23% higher product failure rates and 31% less effective campaign performance compared to organizations employing bias-mitigation techniques.
As Professor Robert Cialdini, renowned influence researcher, observes, "The most dangerous biases aren't found in consumers but in how we study them—these methodological blind spots shape everything we think we know about our markets."
1. Social Desirability and Priming Effects
Social desirability bias—respondents answering in ways they believe will be viewed favorably—and priming effects—subtle cues influencing response patterns—represent pervasive challenges requiring systematic mitigation.
Recognizing Social Pressure in Research Contexts
Social desirability manifests differently across research domains:
- Brand perception research where respondents overstate premium brand preference
- Sustainability studies where environmental concern is artificially elevated
- Health behavior research showing exaggerated compliance with recommendations
- Financial studies where spending is understated and saving overstated
Advanced researchers counter these effects through indirect questioning techniques, projective methods, and behavioral validation approaches that circumvent respondents' self-presentation concerns.
Implicit Association Measurement
Leading organizations increasingly supplement explicit questions with implicit association techniques:
- Response latency measurement revealing unconscious associations
- Gamified assessment tools reducing deliberate response control
- Neuroscience-inspired approaches capturing automatic responses
- Scenario-based evaluations removing direct self-reference
Nike's consumer insights team pioneered combining explicit brand attribute ratings with implicit association testing, revealing a 34% gap between stated and unconscious brand perceptions that dramatically reshaped their messaging strategy.
Design-Level Priming Control
Sophisticated research operations implement systematic priming controls:
- Question sequence randomization preventing order effects
- Neutral language development protocols
- Brand masking techniques where appropriate
- Counter-balanced design frameworks
Research by the Advertising Research Foundation found implementing comprehensive priming controls resulted in customer preference data with 27% greater predictive accuracy for actual purchasing behavior.
2. Recall and Sampling Bias
Memory limitations and non-representative sampling create foundational distortions that undermine research validity regardless of analytical sophistication.
Memory Reconstruction vs. Recall
Human memory operates through reconstruction rather than retrieval, creating systematic distortions:
- Telescoping effects compressing or expanding timelines
- Post-experience rationalization altering remembered motivations
- Peak-end effects overweighting emotional high points and conclusions
- Consistency bias aligning remembered behavior with current attitudes
Forward-thinking researchers address these limitations through experience sampling methods, passive measurement techniques, and contextual triggers that capture insights closer to the experience moment.
Representative Sampling Beyond Demographics
Traditional demographic balancing addresses only the most obvious sampling biases:
- Digital behavior patterns creating participation propensity differences
- Psychographic factors influencing research engagement
- Category involvement variations affecting who participates
- Response style tendencies skewing who completes studies
Procter & Gamble revolutionized their sampling approach by incorporating digital behavior patterns and category engagement metrics alongside demographics, improving predictive validity of research outcomes by 41% for new product introductions.
Non-Response Analysis
Leading research operations analyze who doesn't participate as rigorously as who does:
- Systematic follow-up with non-respondents
- Passive data comparison between respondents and non-respondents
- Propensity modeling to identify and correct participation bias
- Sensitivity analysis estimating impact of missing voices
Hospitality leader Marriott implemented comprehensive non-response analysis for their guest satisfaction research, revealing that their most dissatisfied customers were 72% less likely to complete surveys, significantly distorting satisfaction metrics.
3. Researcher Influence and Confirmation Bias
Researcher expectations and preferences subtly shape every aspect of the research process, creating compounding distortions that can fundamentally mislead decision-makers.
Observer Effects in Qualitative Research
Researcher presence inevitably influences participant behavior:
- Status dynamics between researchers and participants
- Non-verbal cues signaling "correct" responses
- Cultural alignment affecting communication patterns
- Environment effects from artificial research settings
Progressive research operations implement structured reflexivity protocols, third-party moderation, and environment naturalization techniques to minimize these distortions.
Hypothesis-Confirming Analysis
Analysis approaches frequently privilege confirmation over disconfirmation:
- Selective data filtering reinforcing existing beliefs
- Statistical fishing revealing spurious patterns
- Asymmetric skepticism toward unexpected findings
- Post-hoc rationalization of contradictory results
Microsoft's marketing sciences team addresses these tendencies through adversarial analysis protocols where separate teams analyze the same data with competing hypotheses, reducing confirmatory analysis bias by 58% in A/B test interpretation.
Organizational Pressure and Motivated Reasoning
Organizational context creates powerful incentives affecting research interpretation:
- Executive expectations constraining acceptable conclusions
- Previous investments creating sunk cost effects
- Team consensus generating conformity pressure
- Career implications of delivering unwelcome findings
Research operations at innovation-driven organizations like Apple and Google implement structural safeguards including insight review committees with methodological expertise, standardized validity assessment frameworks, and organizational separation between research and implementation teams.
Conclusion: Building a Bias-Resistant Research Practice
As marketing research tools become increasingly sophisticated, addressing fundamental biases becomes more critical than ever. Advanced analytics cannot compensate for biased inputs, and AI-powered research tools risk amplifying rather than reducing human biases without proper design.
The future belongs to research operations that integrate bias mitigation throughout the research lifecycle rather than attempting to correct for biases during analysis. Organizations implementing comprehensive bias management frameworks report not only more accurate insights but also 37% higher research ROI and 29% greater stakeholder confidence in research-based decisions.
Next-generation approaches include adversarial research designs specifically intended to challenge organizational assumptions, machine learning bias detection systems, and cross-functional research validation protocols that leverage diverse perspectives to identify potential blind spots.
Call to Action
For research professionals seeking to minimize bias in consumer insights:
- Audit existing research methodologies for bias vulnerabilities
- Implement structured bias mitigation protocols across the research lifecycle
- Develop methodological triangulation approaches to validate key insights
- Create organizational mechanisms separating research findings from implementation pressure
- Build systematic reflexivity practices that acknowledge researcher influence
In today's complex decision environment, the most valuable research isn't simply methodologically sophisticated or statistically significant—it's fundamentally honest about human limitations and organizational pressures that shape what we see and what we miss in consumer behavior.
Featured Blogs
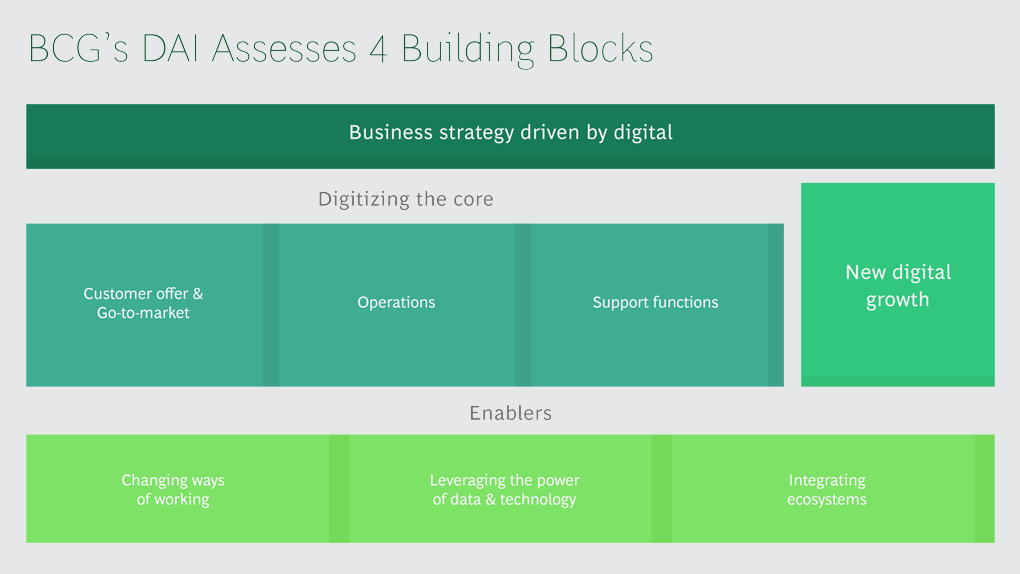
BCG Digital Acceleration Index
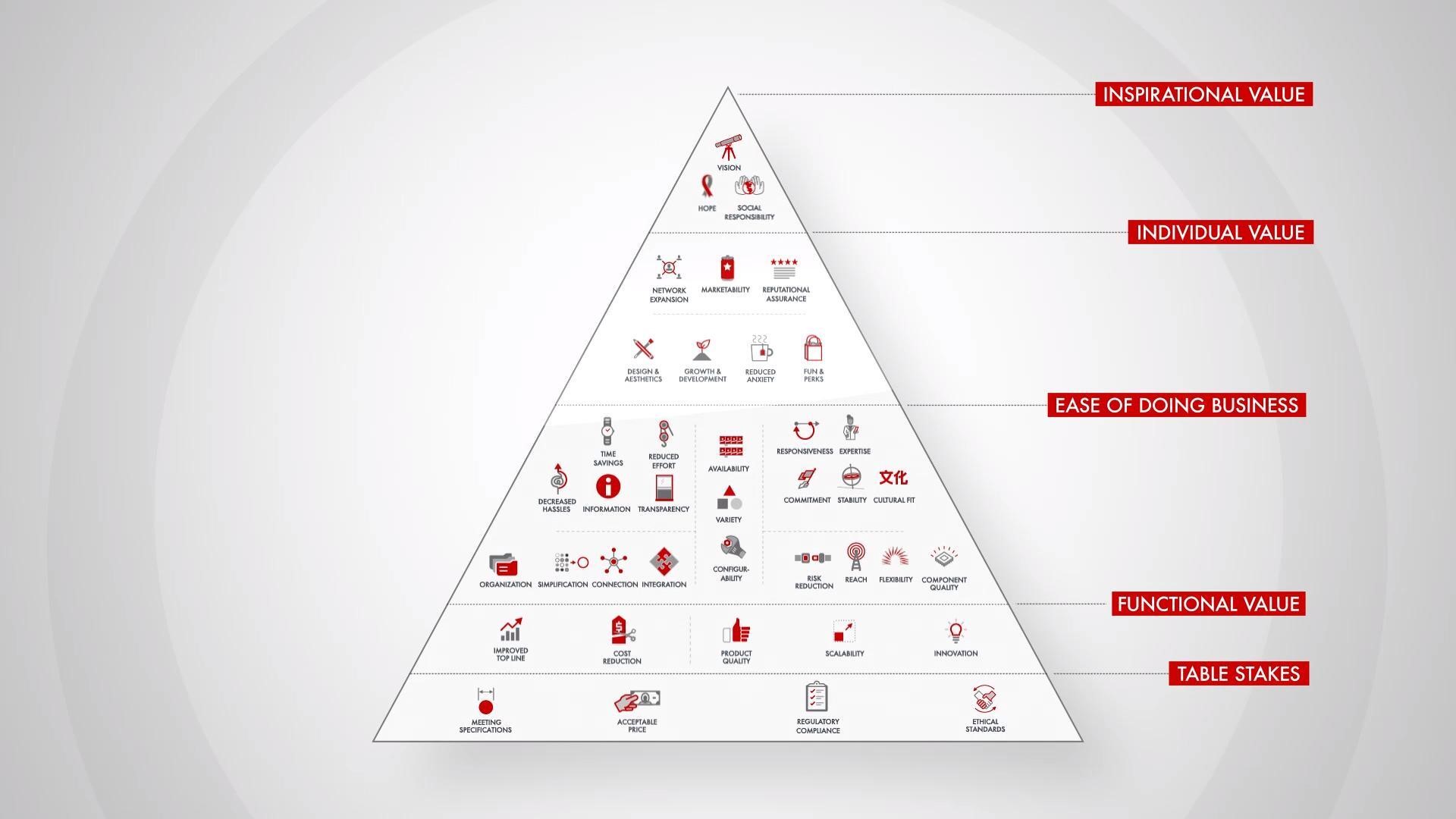
Bain’s Elements of Value Framework
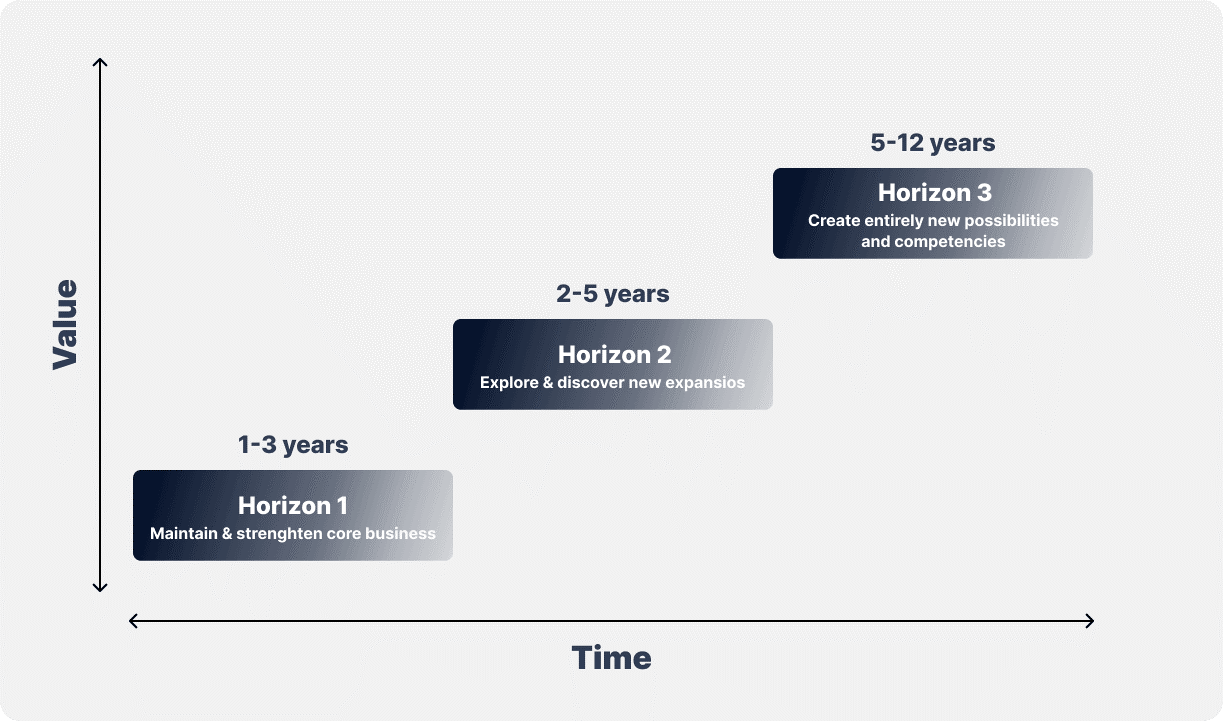
McKinsey Growth Pyramid
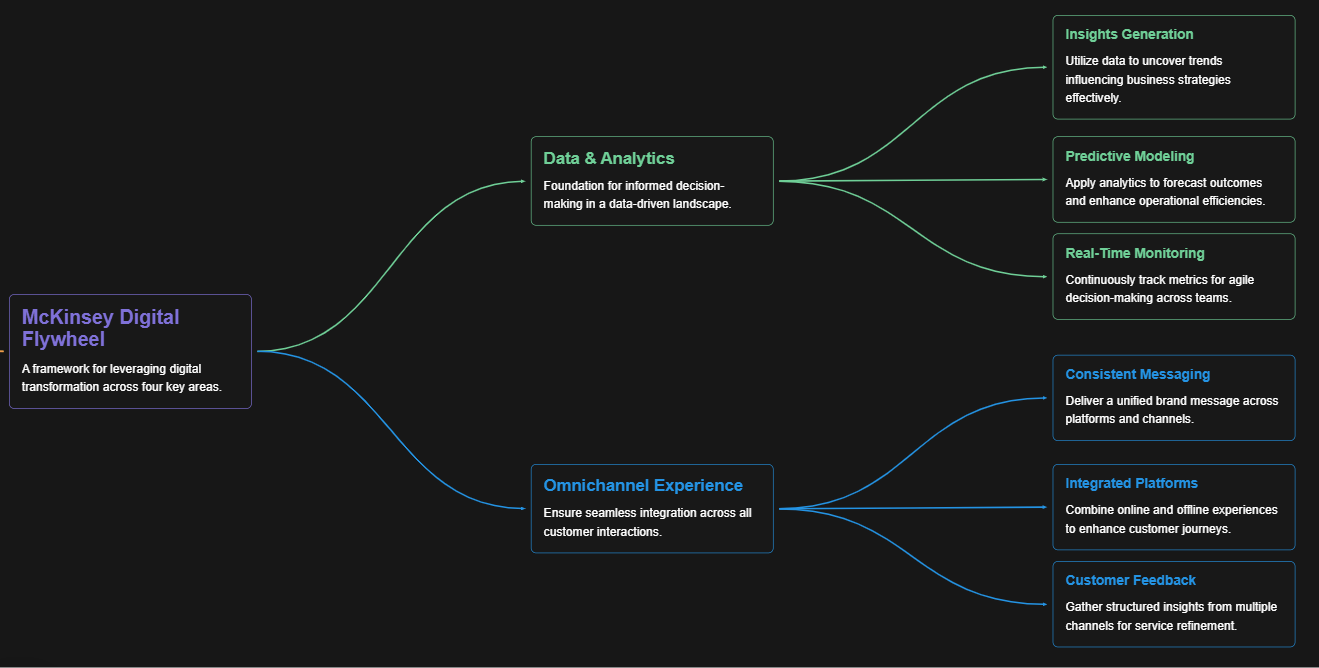
McKinsey Digital Flywheel
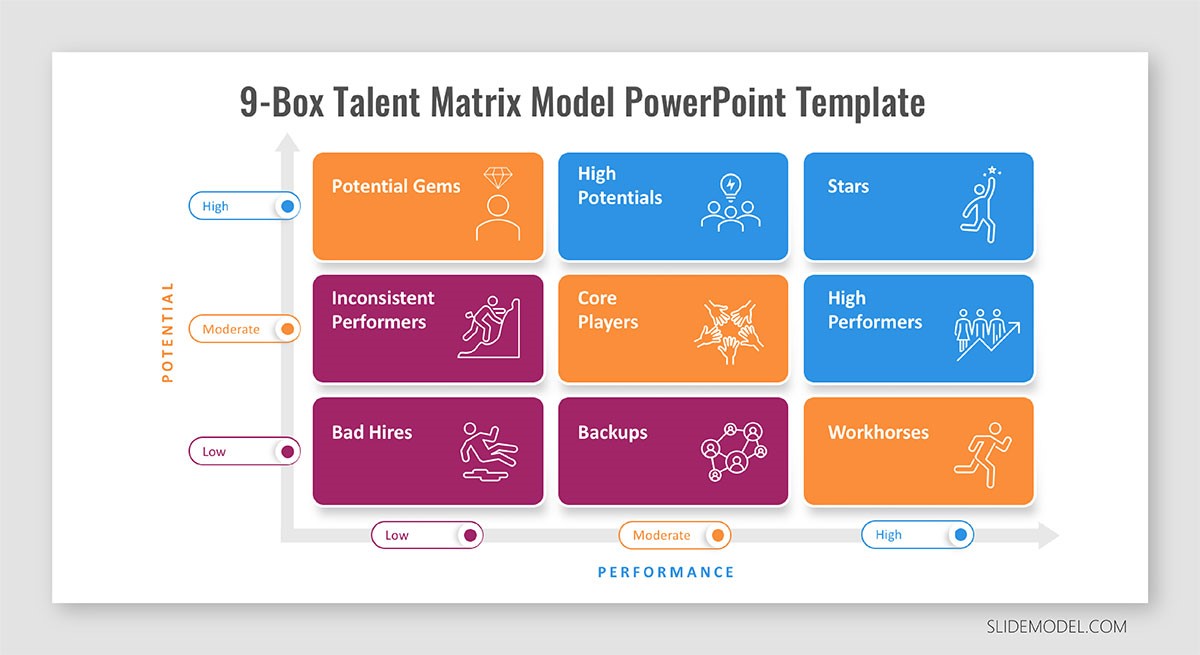
McKinsey 9-Box Talent Matrix
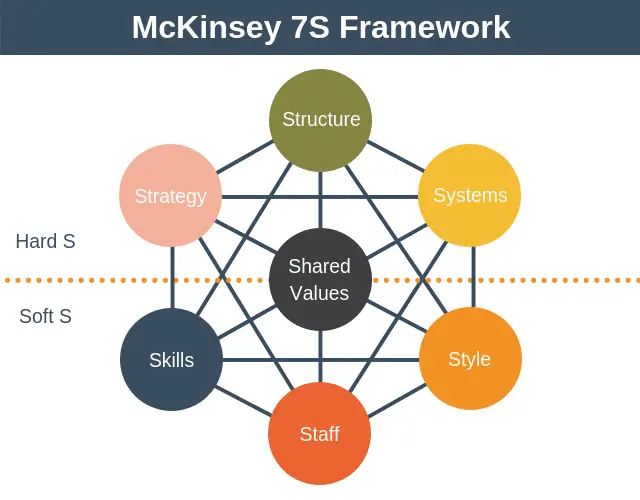
McKinsey 7S Framework
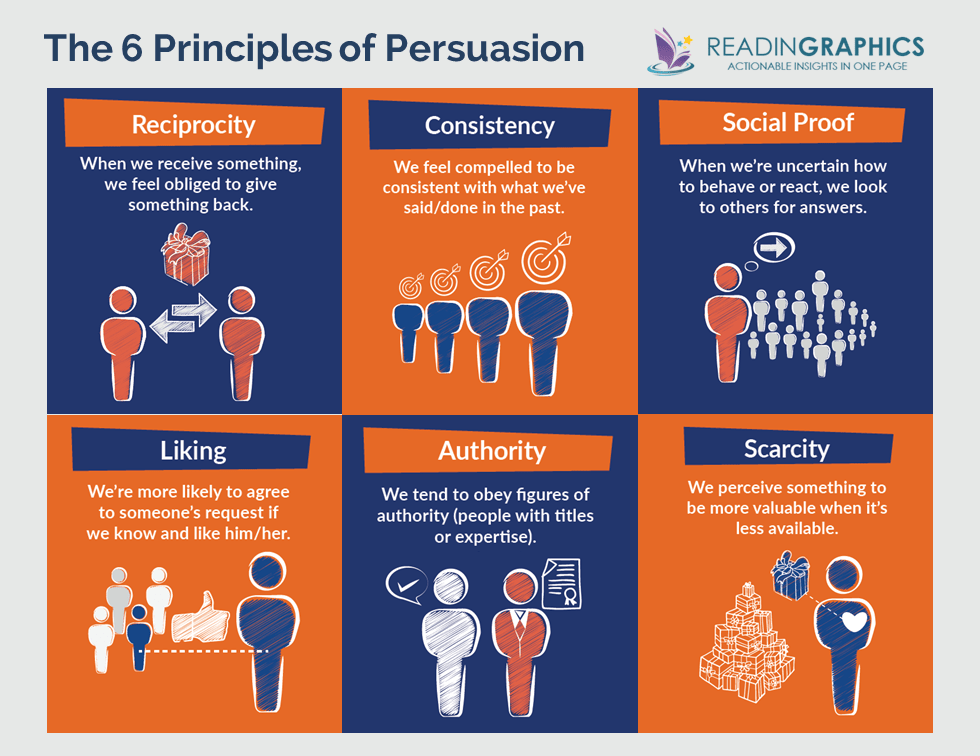
The Psychology of Persuasion in Marketing
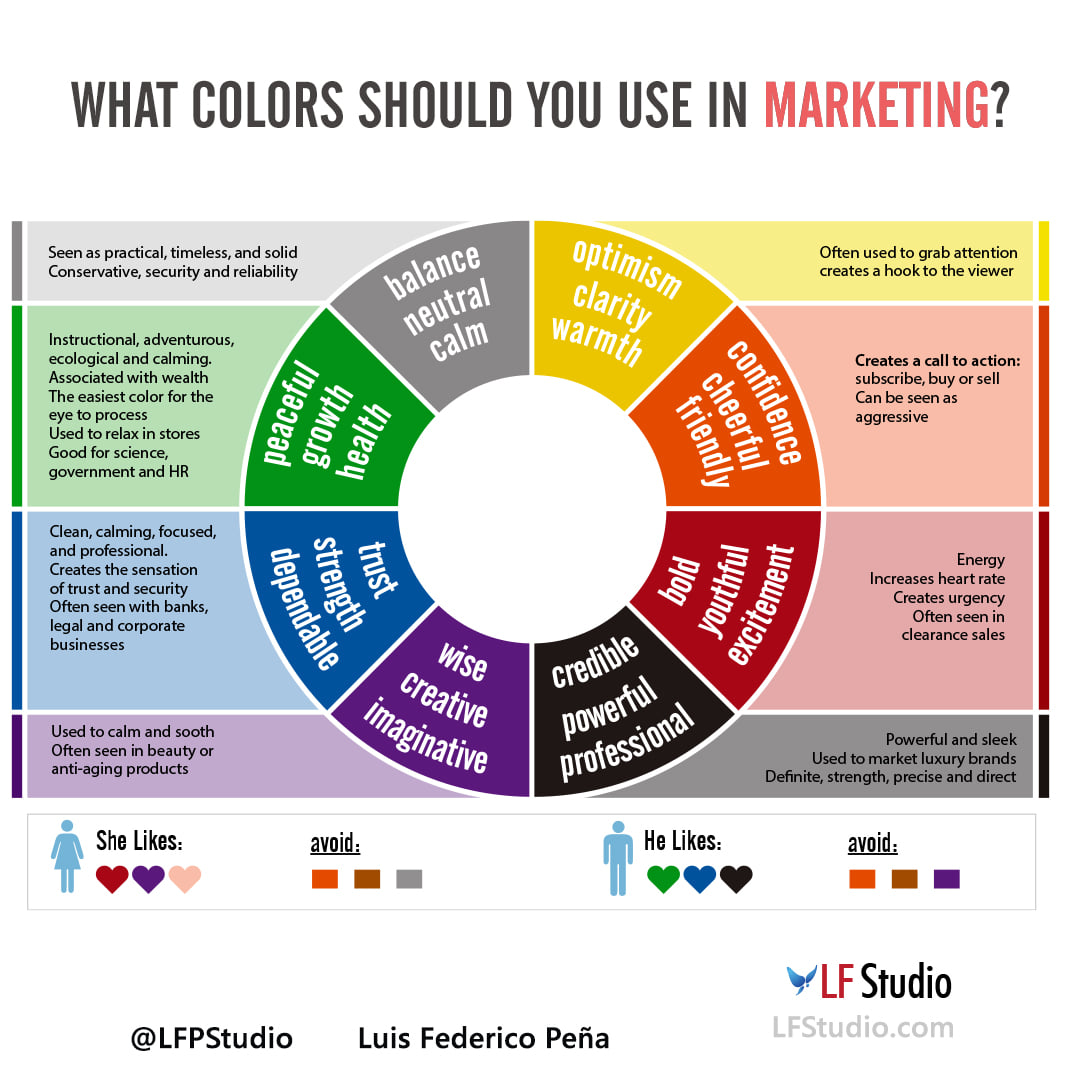
The Influence of Colors on Branding and Marketing Psychology
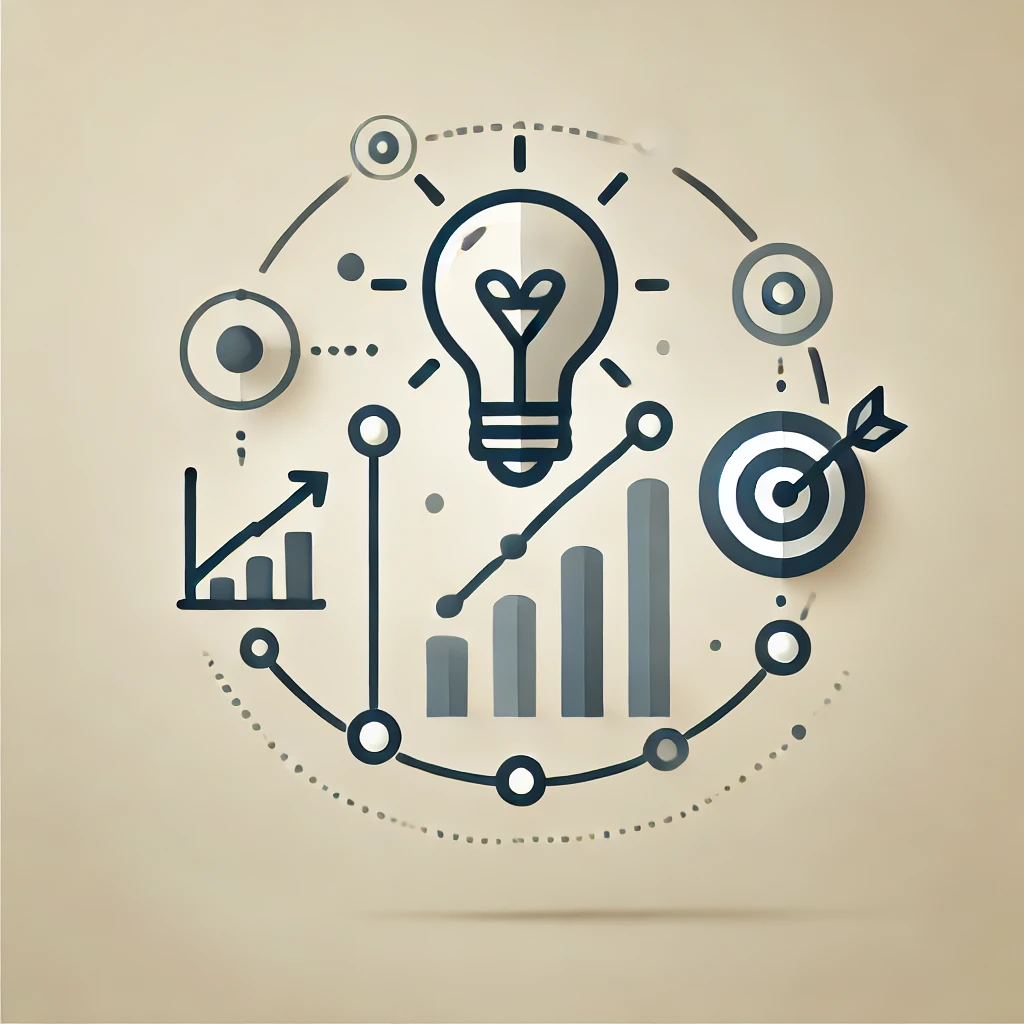