Using Factor Analysis to Simplify Consumer Data
Last spring, Neha sat across from the VP of Marketing at a global beauty brand as the VP pushed away a 120-page brand perception report, visibly frustrated. "We asked consumers about 45 different brand attributes," she sighed, "but I still can't tell what's really driving their decisions or how we compare to competitors on dimensions that actually matter." The team had fallen into a common trap—mistaking more data for better insights. The following week, Neha introduced her to factor analysis, a statistical approach that would transform their overwhelming attribute list into a manageable set of meaningful dimensions. Six months later, the brand's repositioning campaign, built around three core factors identified by the analysis, had reversed a two-year market share decline. Tracking studies showed significant improvements in precisely the perceptual areas that mattered most.
Introduction: Finding Order in Complexity
In the era of big data, marketers face a paradoxical challenge: we collect increasingly granular consumer information but struggle to extract meaningful patterns from the overwhelming complexity. Factor analysis addresses this challenge by identifying the underlying structure within large datasets, revealing the hidden dimensions that truly drive consumer perceptions and behavior.
This analytical approach operates on a fundamental insight: many seemingly separate variables actually measure aspects of the same underlying construct. By identifying these latent factors, marketers can reduce dozens of individual metrics to a handful of meaningful dimensions without sacrificing explanatory power.
Research from the Marketing Accountability Standards Board demonstrates that brands using factor analysis to simplify their measurement frameworks achieve 28% higher marketing ROI and 34% better cross-functional alignment than those relying on ungrouped metric sets. As marketing data continues to proliferate, the ability to distill complexity into actionable simplicity has become a critical competitive advantage.
1. Principal Components vs. Factor Analysis
Though often confused, principal components analysis (PCA) and factor analysis represent distinct approaches to dimensionality reduction, each with specific marketing applications.
Principal Components Analysis
creates maximal mathematical efficiency:
- Focused on accounting for total variance in the dataset
- Components are mathematical combinations of original variables
- Primarily a data reduction technique
- Each successive component explains less variance
- All variables contribute to all components to some degree
When Walmart applied PCA to its massive consumer purchase dataset, they reduced 200+ category purchase variables to 12 components that captured 83% of the variation in shopping behavior, enabling more efficient customer targeting.
Factor Analysis
seeks underlying explanatory constructs:
- Focused on explaining correlations between variables
- Factors represent hypothesized latent constructs
- Primarily an explanatory technique
- Variables typically load strongly on only a few factors
- Rotation methods enhance interpretability
Hotel chain Marriott employed factor analysis on guest satisfaction metrics and discovered three underlying factors—"service excellence," "physical comfort," and "frictionless experience"—that explained 78% of variation in overall satisfaction scores, leading to targeted operational improvements.
Choosing the Right Approach
depends on your objective:
- Use PCA when pure data reduction efficiency is the goal
- Choose factor analysis when seeking meaningful psychological constructs
- Consider PCA as a first step before factor analysis with very large datasets
- Exploratory factor analysis identifies structures inductively
- Confirmatory factor analysis tests hypothesized structures
Financial services giant American Express strategically combines approaches, using PCA for initial data exploration before applying factor analysis to develop their renowned "customer engagement index" that guides relationship management.
2. Use Cases: Brand Image and Psychographics
Factor analysis proves particularly valuable in two critical marketing domains where multiple measures often obscure simpler underlying structures.
Brand Image Simplification
clarifies perception patterns:
- Reduces extensive attribute lists to core dimensions
- Reveals how attributes cluster perceptually
- Identifies the underlying factors driving brand preference
- Enables more meaningful competitive mapping
- Simplifies tracking and communication
When Procter & Gamble applied factor analysis to laundry detergent brand perceptions, they discovered that 28 measured attributes collapsed into just four factors—"cleaning power," "gentleness," "fragrance experience," and "environmental responsibility"—simplifying both strategic focus and messaging architecture.
Psychographic Framework Development
structures consumer mindsets:
- Transforms lengthy attitudinal batteries into manageable dimensions
- Identifies fundamental value orientations
- Creates more meaningful segmentation inputs
- Reveals lifestyle patterns from behavioral data
- Enables cross-category psychological profiling
Automotive manufacturer Toyota used factor analysis to develop its "Innovation Orientation Index" by reducing 35 technology attitude statements to three core dimensions—"early adoption drive," "practicality focus," and "status consciousness"—that now guide product feature development and communication strategy.
Data Integration Applications
synthesize multiple sources:
- Combines variables across research studies
- Integrates attitudinal and behavioral metrics
- Aligns customer and employee measurement frameworks
- Creates unified indices from disparate measures
- Enables holistic organizational scorecards
When Netflix applied factor analysis across viewing behaviors, content ratings, and survey responses, they identified seven content engagement factors that now guide their recommendation algorithm and original content development strategy.
3. Deciding the Number of Factors
Perhaps the most crucial decision in factor analysis is determining how many factors to retain—balancing parsimony against explanatory power.
Statistical Criteria
provide objective guidance:
- Kaiser criterion retains factors with eigenvalues above 1.0
- Scree plot analysis identifies natural breaks in explained variance
- Parallel analysis compares results to random data
- Minimum percentage of variance explained (typically 60-70%)
- Statistical fit measures in confirmatory analysis
When Unilever conducted factor analysis on their global brand health metrics, parallel analysis indicated five factors would be optimal—two fewer than the seven dimensions in their existing framework, leading to a more streamlined but equally explanatory measurement system.
Practical Considerations
ensure usability:
- Interpretability of the factor structure
- Face validity with domain experts
- Alignment with existing theoretical frameworks
- Managerial usefulness and actionability
- Stability across samples and time periods
Starbucks initially identified six factors in their customer experience analysis but consolidated to four when testing revealed the last two factors weren't consistently actionable at the store level, creating a more pragmatic framework without sacrificing key insights.
Rotation Methods
enhance interpretability:
- Varimax rotation maximizes distinctiveness between factors
- Oblique rotations allow for correlated factors
- Pattern matrix shows relationships between variables and factors
- Structure matrix accounts for factor intercorrelations
- Factor scoring enables individual consumer profiles
When retailer Target applied different rotation methods to their shopper motivation analysis, oblique rotation revealed meaningful correlations between "convenience" and "value-seeking" factors that had appeared independent in earlier analyses, fundamentally reshaping their understanding of shopping missions.
Call to Action
To leverage factor analysis effectively in your marketing research:
- Start with clear conceptual hypotheses about potential underlying dimensions
- Collect diverse indicators for each suspected construct rather than redundant measures
- Apply both statistical criteria and practical judgment when determining factor structure
- Test the stability of your solution across different consumer segments
- Translate statistical factors into compelling marketing narratives that drive strategic action
Thinking back to that frustrated VP of Marketing, factor analysis didn't just simplify their data—it transformed their entire approach to brand management. By focusing resources on the three dimensions that truly mattered to consumers, they created more coherent communications, more targeted product development, and more aligned organizational objectives. In an increasingly complex marketing environment, the ability to identify the signal within the noise isn't just an analytical skill—it's a strategic imperative for modern brands seeking meaningful consumer connections.
Featured Blogs
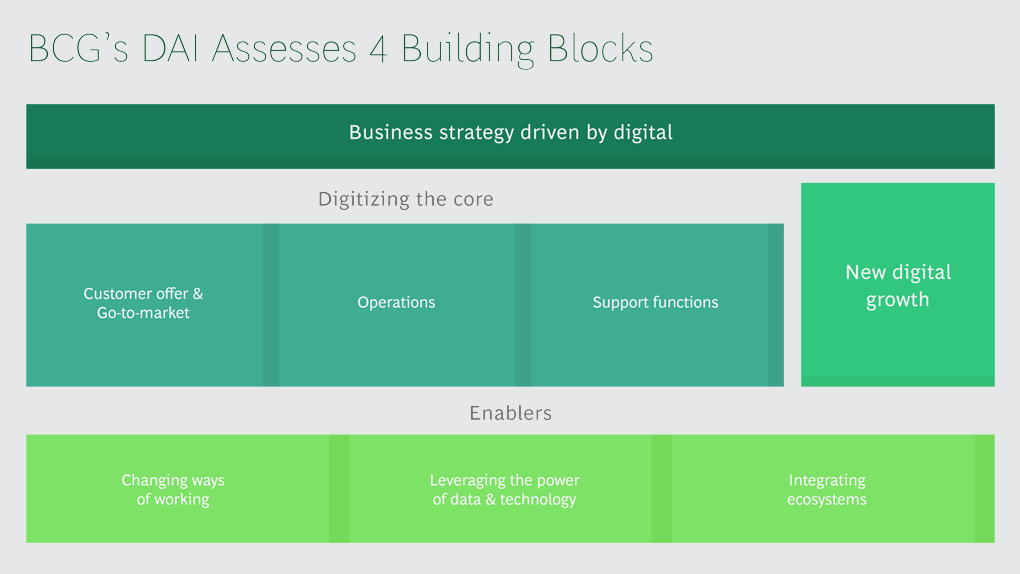
BCG Digital Acceleration Index
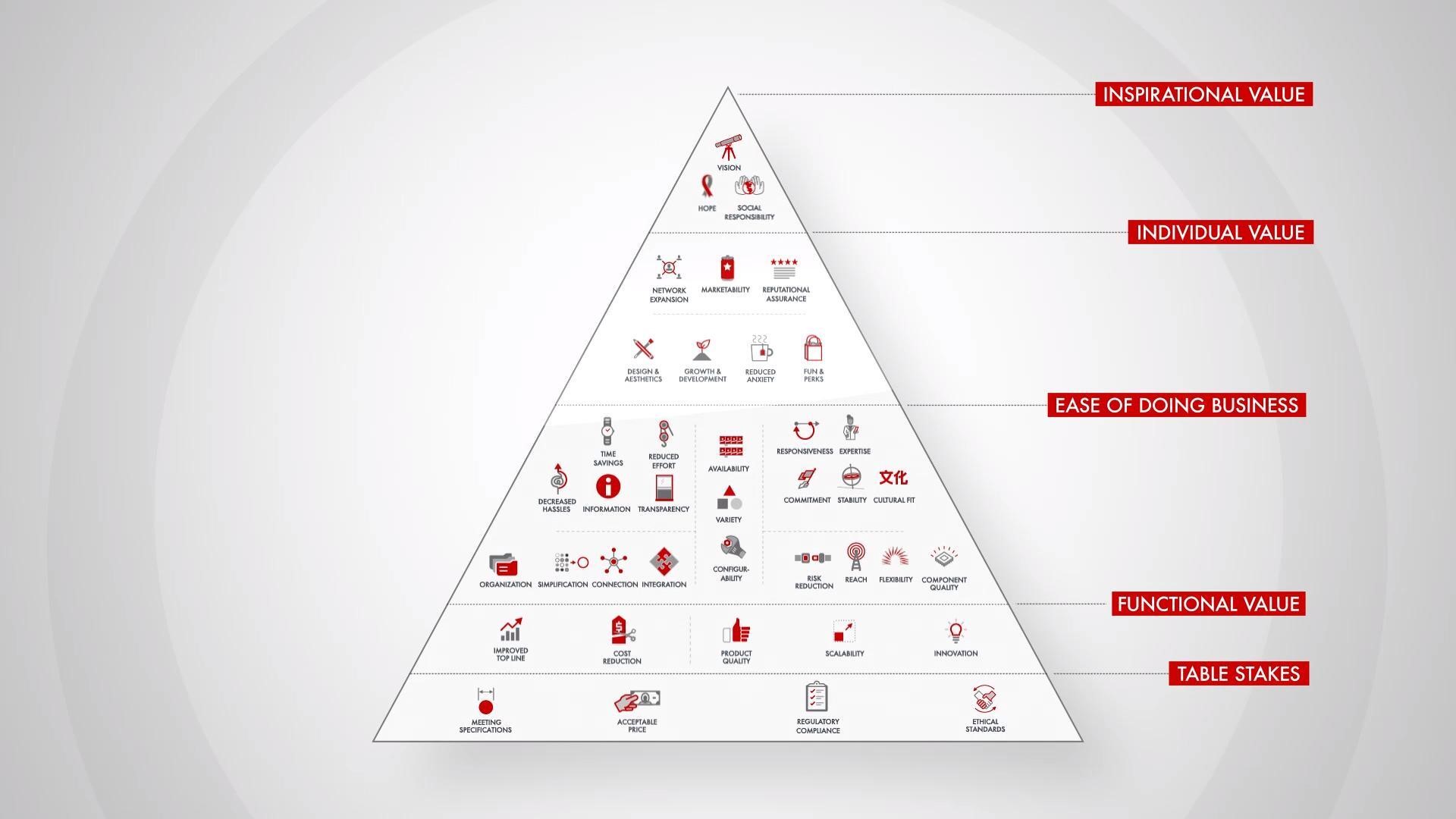
Bain’s Elements of Value Framework
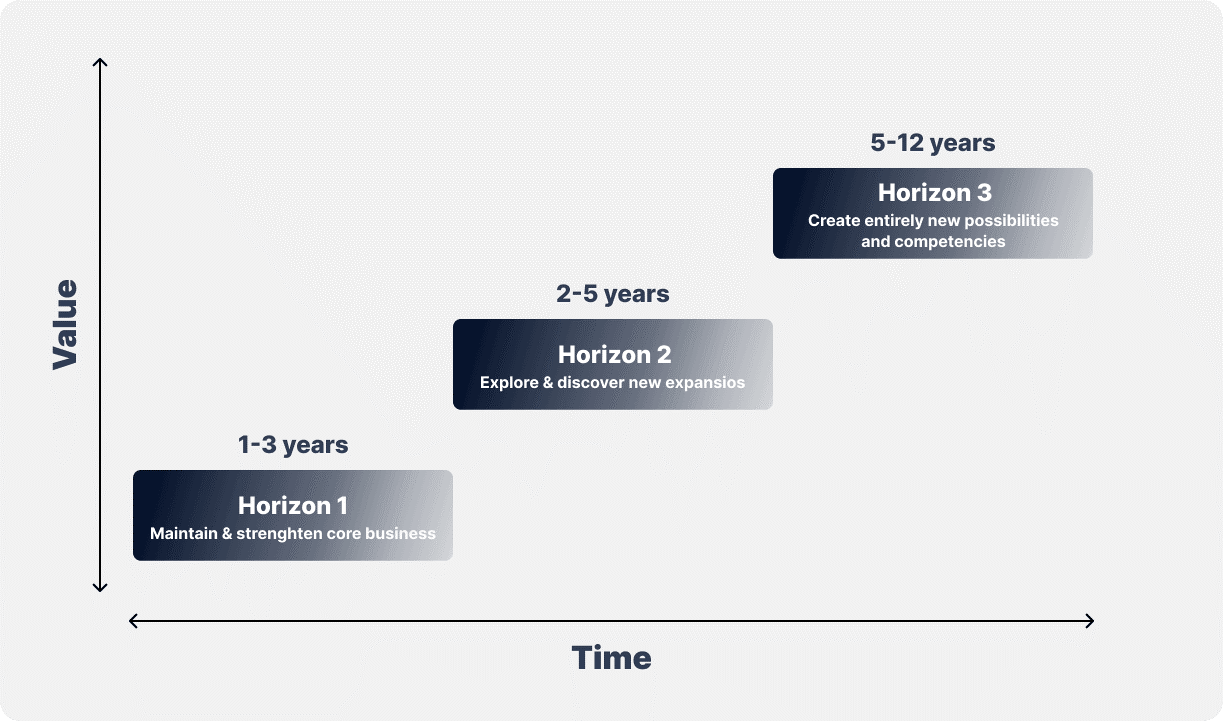
McKinsey Growth Pyramid
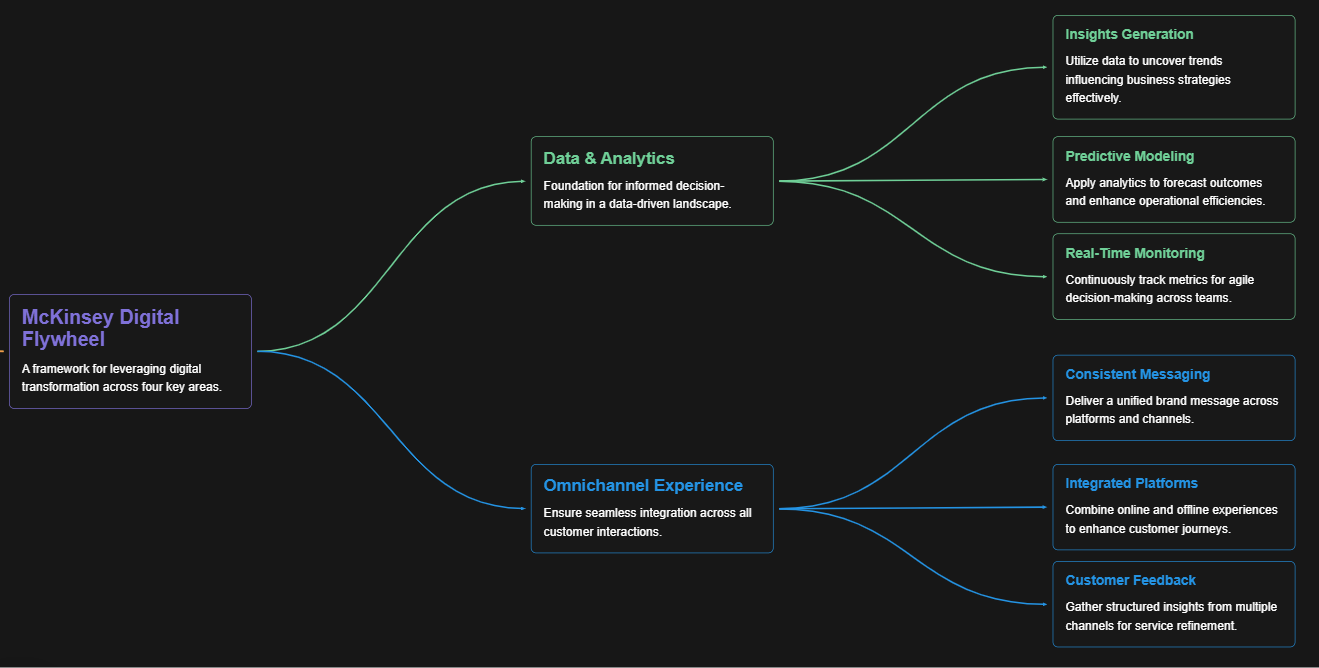
McKinsey Digital Flywheel
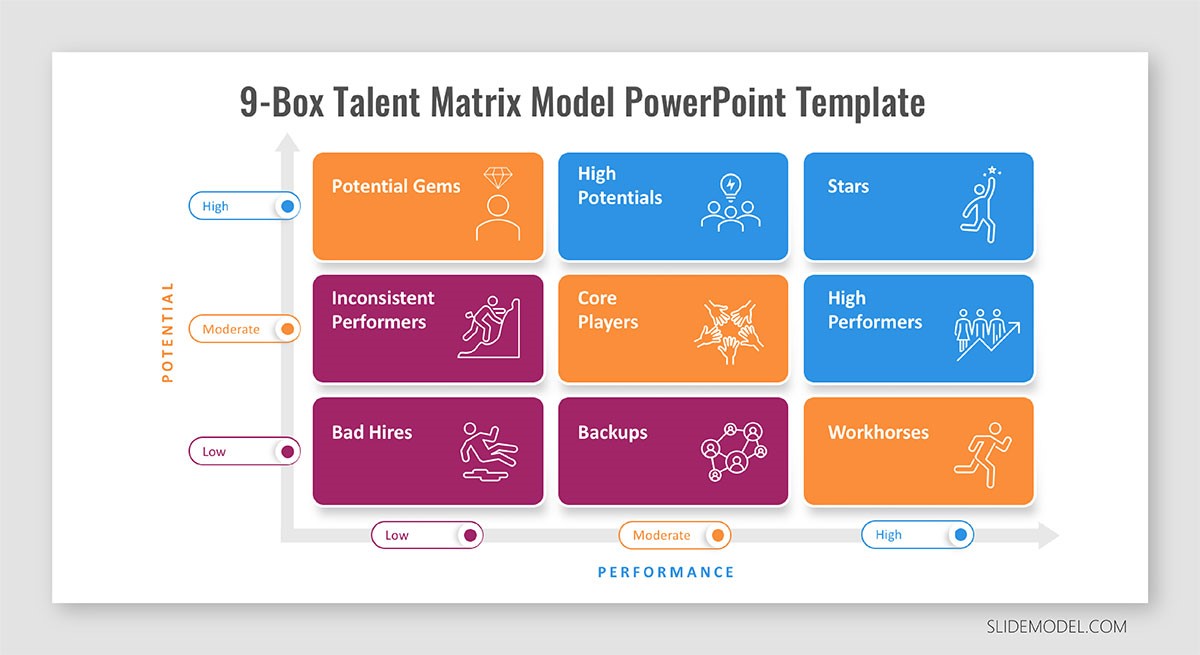
McKinsey 9-Box Talent Matrix
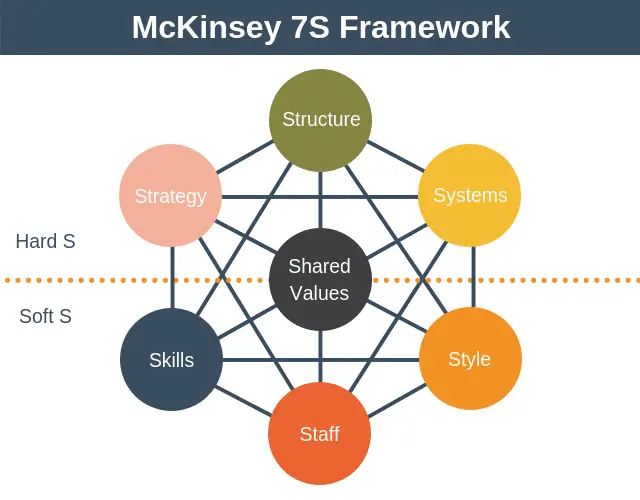
McKinsey 7S Framework
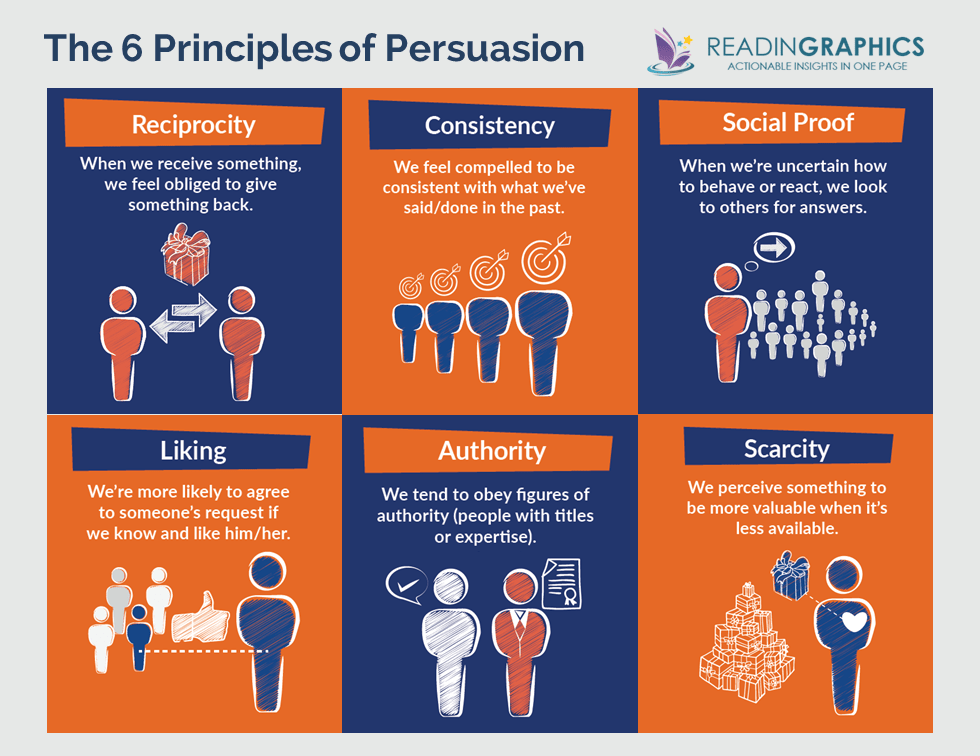
The Psychology of Persuasion in Marketing
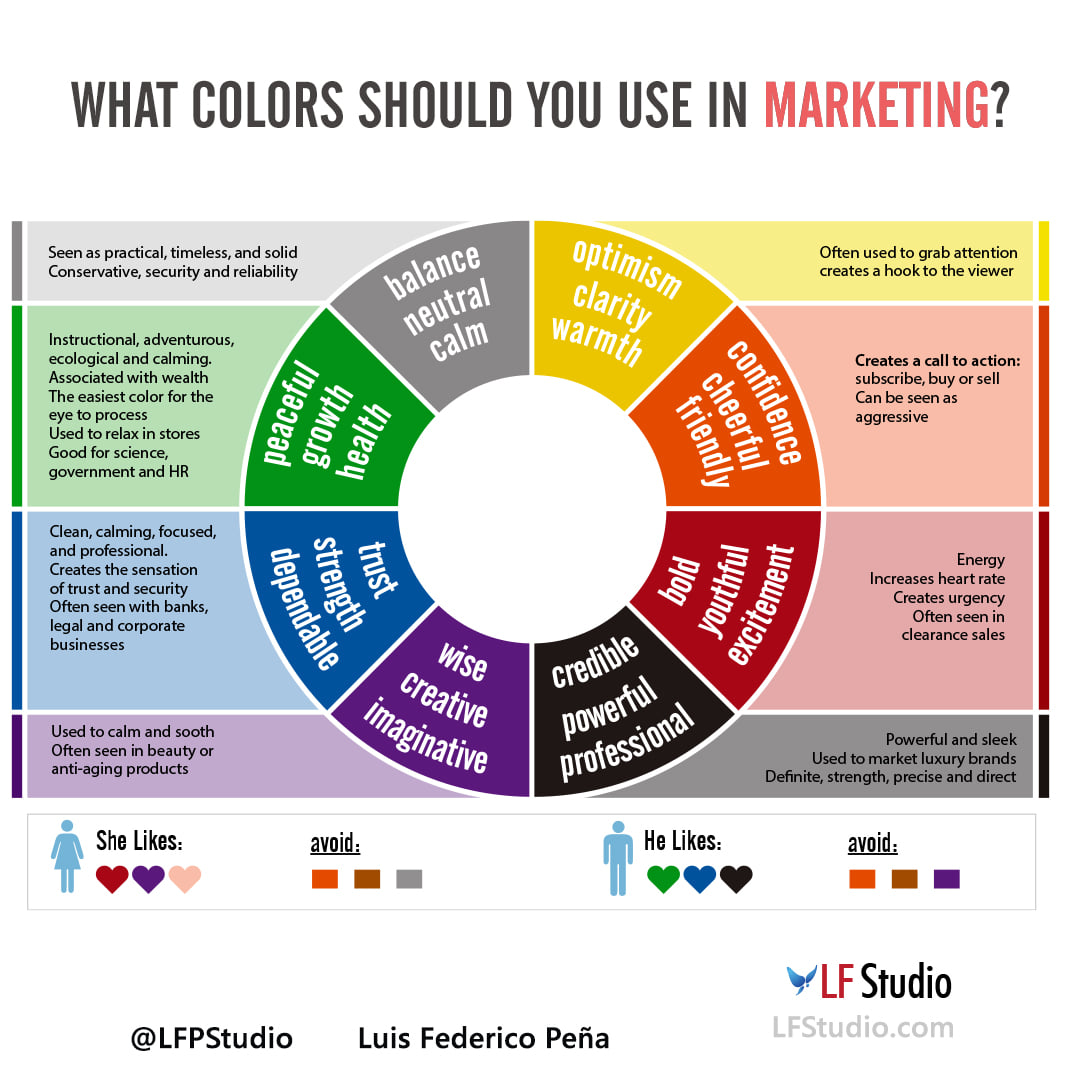
The Influence of Colors on Branding and Marketing Psychology
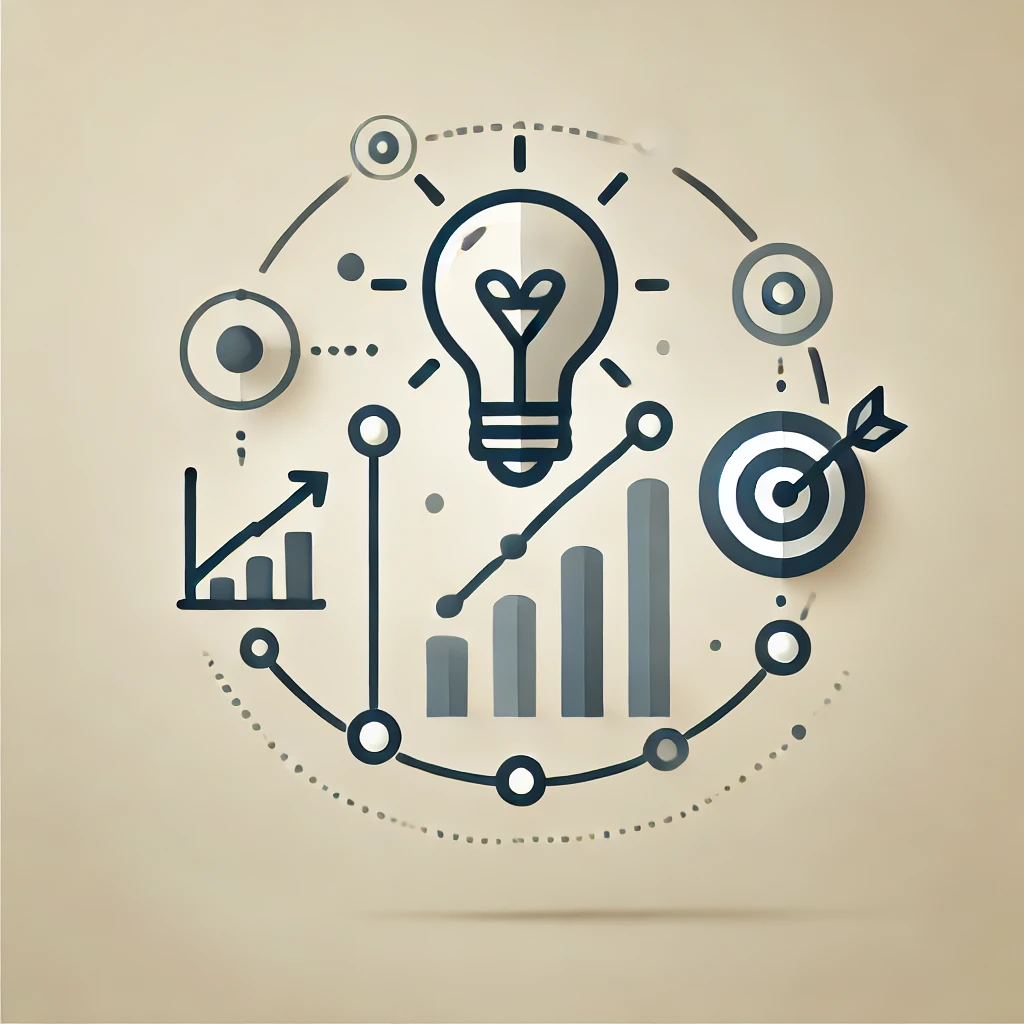