Sampling Methods in Marketing Research
The hotel conference room fell silent as the CMO finished presenting the market segmentation strategy. "Based on our research," she concluded confidently, "we're targeting Segment A exclusively." Neha glanced at her colleague from the research department, who looked uncomfortable. Later, over dinner, her colleague confided what was bothering her. "Their sample was completely biased—they only surveyed existing customers in three urban locations." That conversation has stayed with Neha for years, serving as a stark reminder of how sampling decisions can make or break million-dollar strategies. No matter how sophisticated the analysis becomes, faulty sampling creates a house of cards—impressive but fundamentally unsound.
Introduction: The Foundation of Marketing Truth
Sampling represents the foundation upon which all marketing research insights are built. Even with perfect execution in every other aspect of research, sampling errors can fundamentally undermine the validity of findings and lead to costly strategic missteps. As markets fragment and consumer behavior grows increasingly complex, sampling methodology becomes even more critical to uncovering actionable truths.
The digital transformation has revolutionized sampling approaches—creating unprecedented opportunities for precision while introducing new forms of bias. According to the Marketing Research Association, organizations that modernize their sampling strategies while maintaining methodological rigor show 31% higher ROI on research investments and make more accurate market predictions.
As noted by Professor Byron Sharp in his influential work on marketing science, "The representativeness of your sample determines the boundaries of your knowledge. Sample poorly, and you're not just getting partial insights—you're getting misleading ones."
Probability vs. Non-probability Sampling
Probability sampling employs random selection to give each population member a known, non-zero chance of inclusion. This approach provides statistical validity and enables precise calculation of sampling error. Types include simple random, systematic, stratified, and cluster sampling.
Procter & Gamble's Consumer Pulse program utilizes stratified random sampling to ensure representation across demographic and behavioral segments. This probability-based approach allowed them to identify emerging consumer trends three months earlier than competitors, according to industry benchmarks.
The digital era has both simplified and complicated probability sampling. Online panels with proper recruitment methodologies can approximate probability samples, while API connections to customer databases enable more sophisticated randomization approaches. ESOMAR research indicates companies implementing digital-first probability sampling reduced research timelines by 42% while maintaining statistical validity.
Non-probability sampling selects participants based on availability, judgment, or specific characteristics without random selection. While statistically less rigorous, these methods offer practical advantages in speed, cost, and accessing specialized populations. Types include convenience, purposive, quota, and snowball sampling.
Unilever's rapid innovation teams utilize quota sampling to quickly test product concepts, allowing them to screen ideas three times faster than with probability approaches. For their specialized beauty products, purposive sampling helps target specific skin conditions or usage occasions that random sampling might miss.
The artificial intelligence revolution has particularly enhanced non-probability approaches. Machine learning algorithms now optimize quota samples to better match population characteristics, while natural language processing improves the identification of appropriate participants from digital footprints. Research by the ARF found AI-optimized non-probability samples reduced bias by 36% compared to traditional approaches.
Sample Size and Confidence Levels
Sample size determination balances statistical confidence against practical constraints. Digital technologies have shifted this equation by dramatically reducing the marginal cost of additional responses in many research contexts.
Pharmaceutical company GlaxoSmithKline applies tiered sample sizing—larger samples for strategic decisions (95% confidence, ±2% margin of error) and smaller samples for tactical adjustments (90% confidence, ±5% margin). This approach optimizes research budgets while maintaining appropriate rigor for each decision type.
Sample size requirements vary significantly across methodologies. The Journal of Marketing Research found qualitative approaches like in-depth interviews typically reach saturation at 12-30 participants, while quantitative segmentation studies require 200+ respondents per expected segment to ensure statistical stability.
Technology platforms now enable more sophisticated approaches to sample sizing. Sequential sampling methodologies, popularized by tech companies like Netflix, continually evaluate statistical significance during data collection, stopping exactly when confidence thresholds are met. This approach has reduced average sample sizes by 23% while maintaining statistical power.
Mobile-first research designs have particularly benefited from adaptive sample sizing. Nike's consumer insight team employs Bayesian sequential analysis that adjusts required sample sizes based on emerging effect strengths, optimizing both speed and statistical confidence.
Bias and Representation
Sampling bias occurs when systematic errors prevent the sample from accurately representing the target population. Digital methodologies have introduced new bias challenges while providing tools to address traditional ones.
Coverage bias has evolved in the digital era. While online access has expanded dramatically, the Journal of Consumer Research notes that 14% of American consumers remain digitally underrepresented. Companies like Nielsen maintain mixed-mode sampling frameworks that combine digital and traditional recruitment to ensure comprehensive representation.
Non-response bias presents growing challenges as participation rates decline. Research by ESOMAR shows average survey response rates have fallen from 20% to 5% over the past decade. Leading organizations combat this through respondent engagement strategies, including gamification, mobile optimization, and value-based incentives. Amazon's customer research program achieved 31% higher response rates by implementing personalized outreach and relevant incentives.
Self-selection bias has been magnified by digital methodologies. Starbucks addresses this by employing passive data collection alongside active research participation, creating calibration metrics that adjust for the enthusiasm bias of their most engaged customers.
The integration of primary research with behavioral data provides new approaches to bias management. McDonald's combines declared survey preferences with actual purchase behavior, creating weighted correction factors that significantly improved promotional forecasting accuracy.
Conclusion: The Strategic Imperative of Sampling Excellence
As marketing environments grow increasingly complex, excellence in sampling methodology becomes a critical strategic capability rather than merely a technical consideration. The most successful organizations build sampling frameworks that evolve with changing market conditions and consumer behaviors.
Artificial intelligence and machine learning will continue transforming sampling approaches. AI-powered representativeness algorithms now continuously monitor sample composition, suggesting real-time adjustments to recruitment. Predictive analytics increasingly help forecast potential biases before they impact results.
Integration across data sources represents the next frontier. Organizations like Coca-Cola now employ unified sampling frameworks that connect consumer research samples with analytics from social media, sales data, and digital behavior—creating a comprehensive view that no single sample could provide.
Call to Action
For marketing leaders committed to building stronger sampling capabilities:
- Audit current sampling methodologies against best practices in your category
- Develop explicit bias identification and mitigation strategies for key research streams
- Invest in sampling technology that enables more sophisticated targeting and weighting
- Create training programs that build sampling literacy across marketing teams
- Establish clear documentation standards for sampling decisions and limitations
The future belongs not to those with merely big data, but to those with representative data—insights built on samples that genuinely reflect the markets they serve. As marketing complexity increases, excellence in sampling methodology becomes not just a research foundation but a sustainable competitive advantage.
Featured Blogs
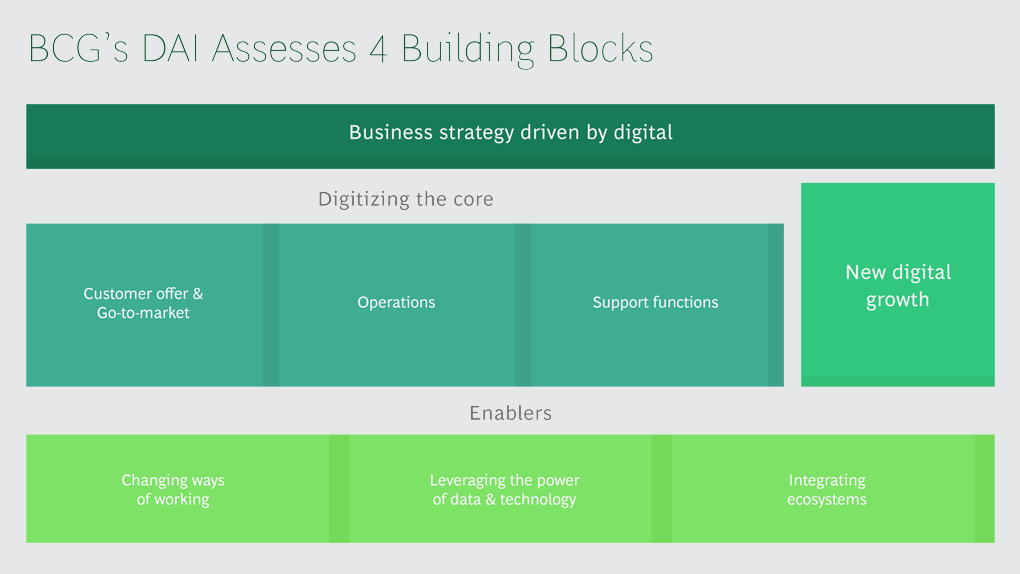
BCG Digital Acceleration Index
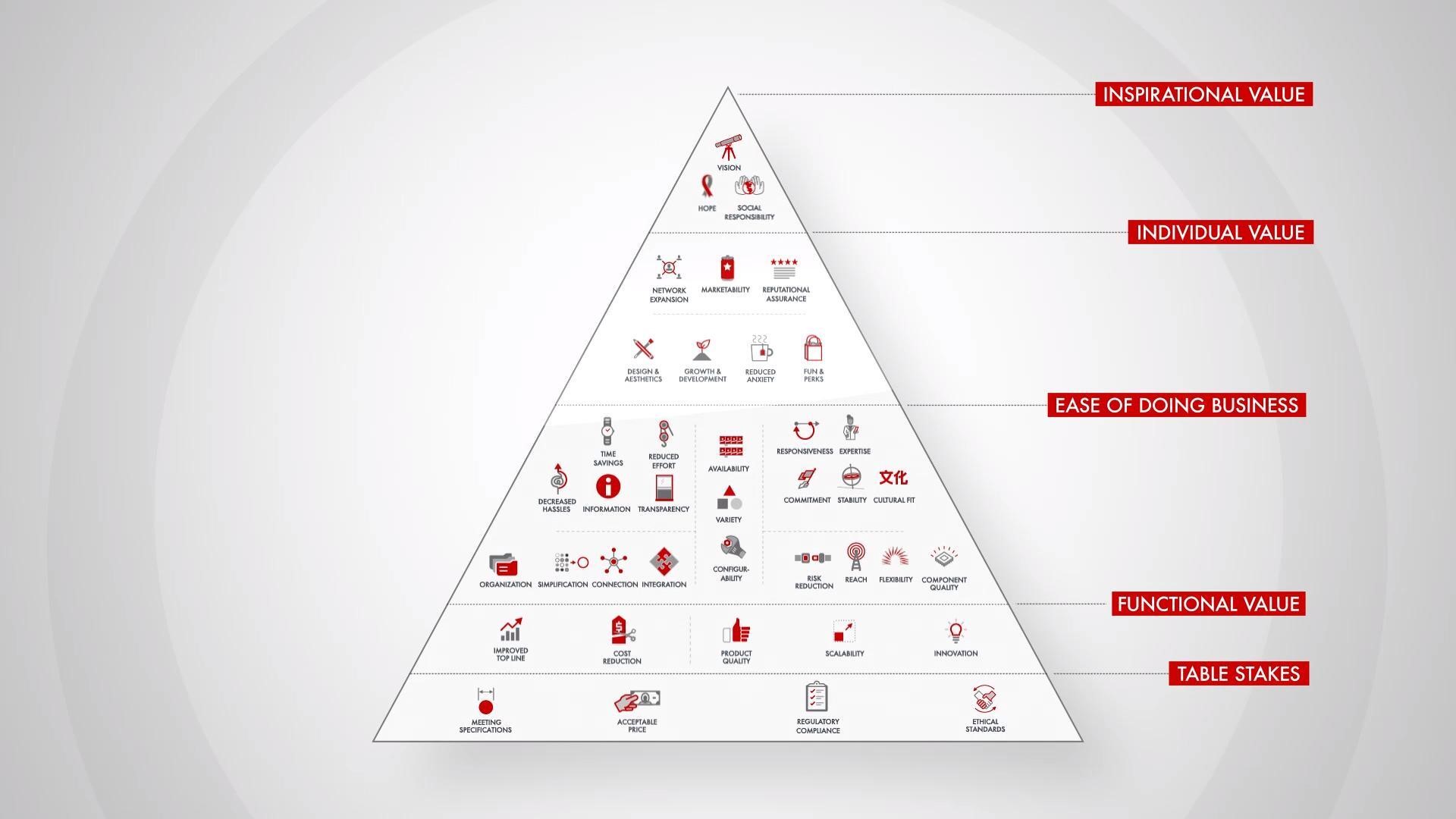
Bain’s Elements of Value Framework
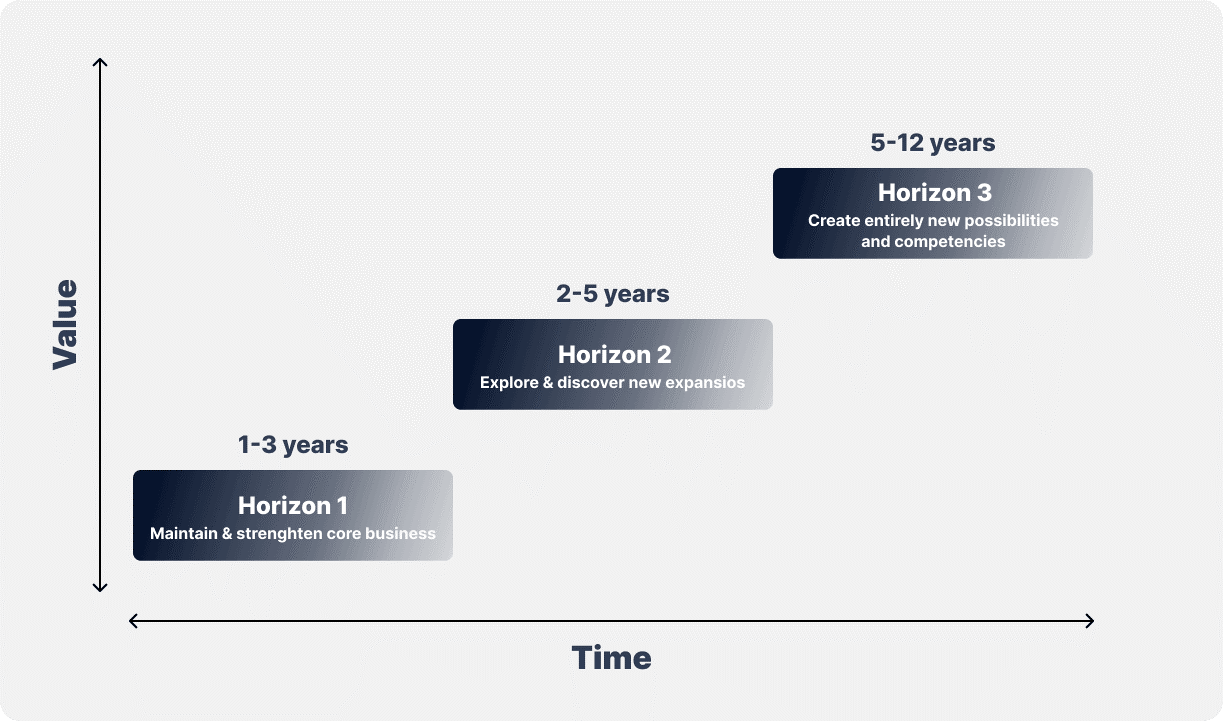
McKinsey Growth Pyramid
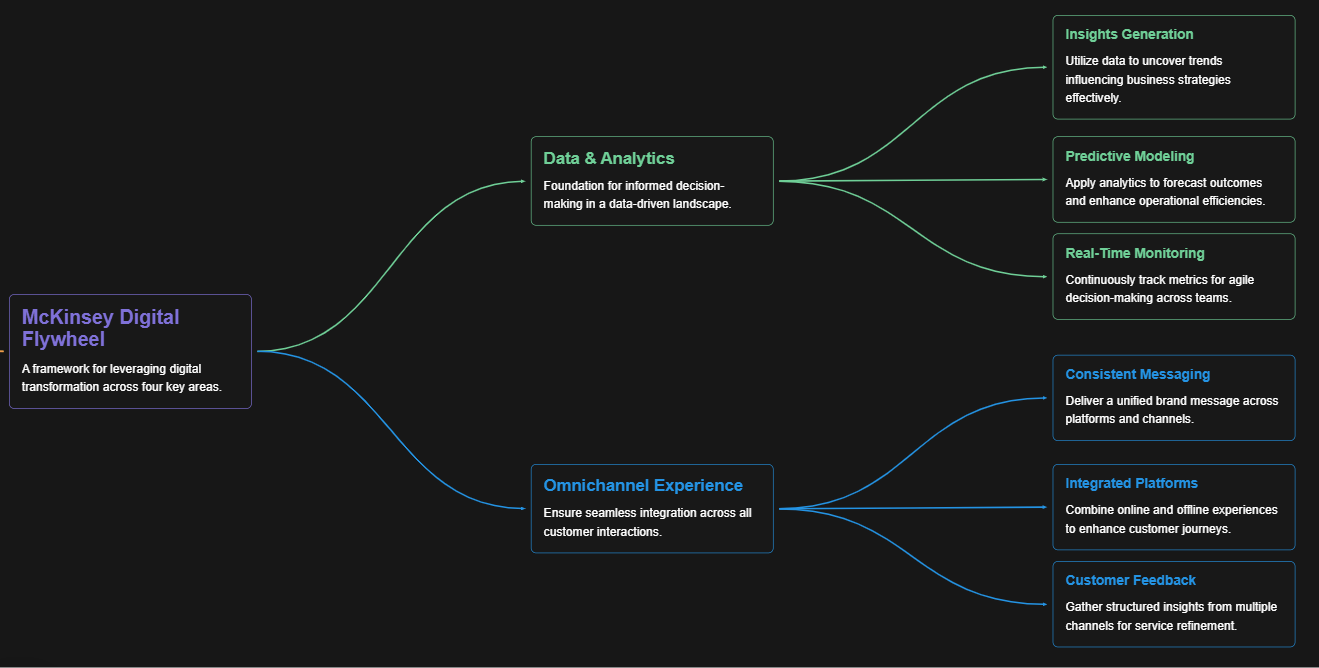
McKinsey Digital Flywheel
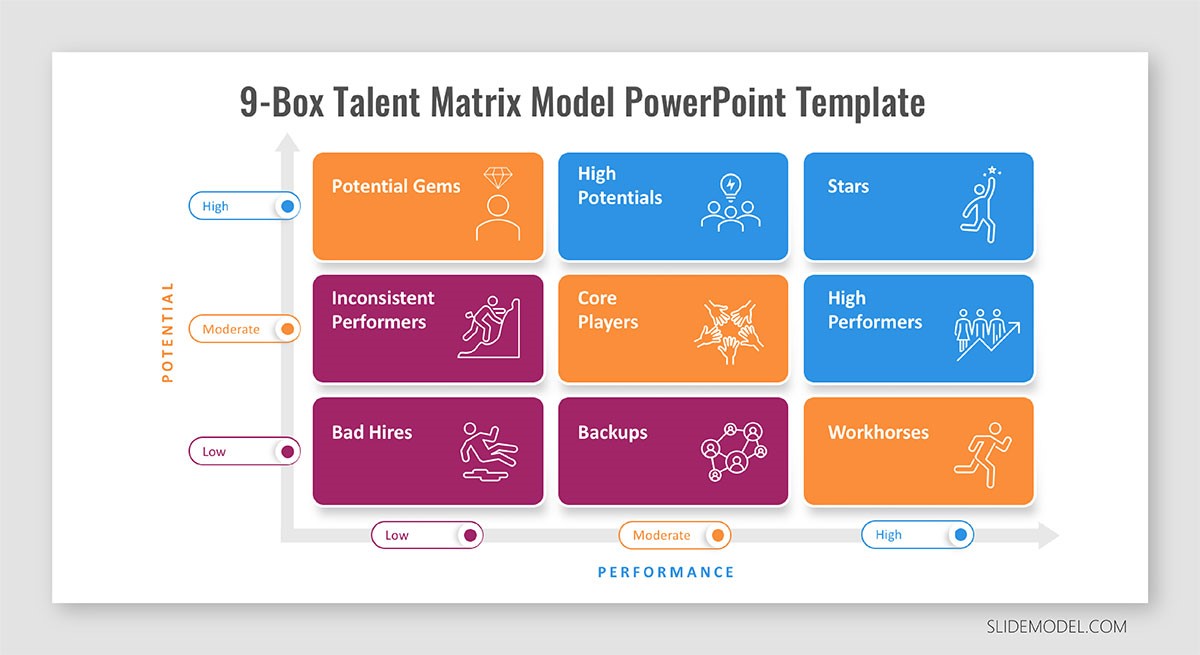
McKinsey 9-Box Talent Matrix
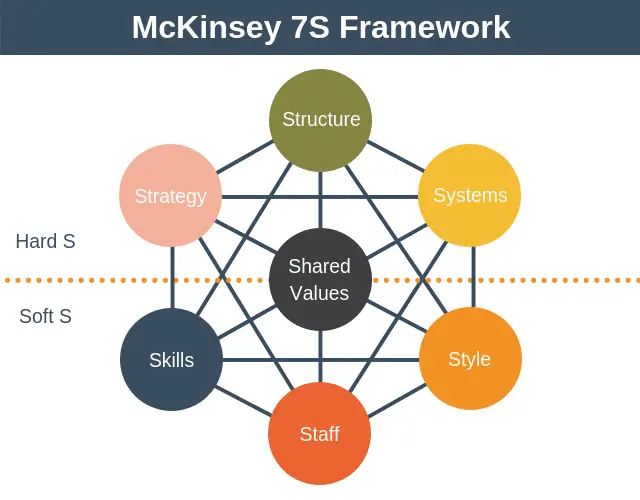
McKinsey 7S Framework
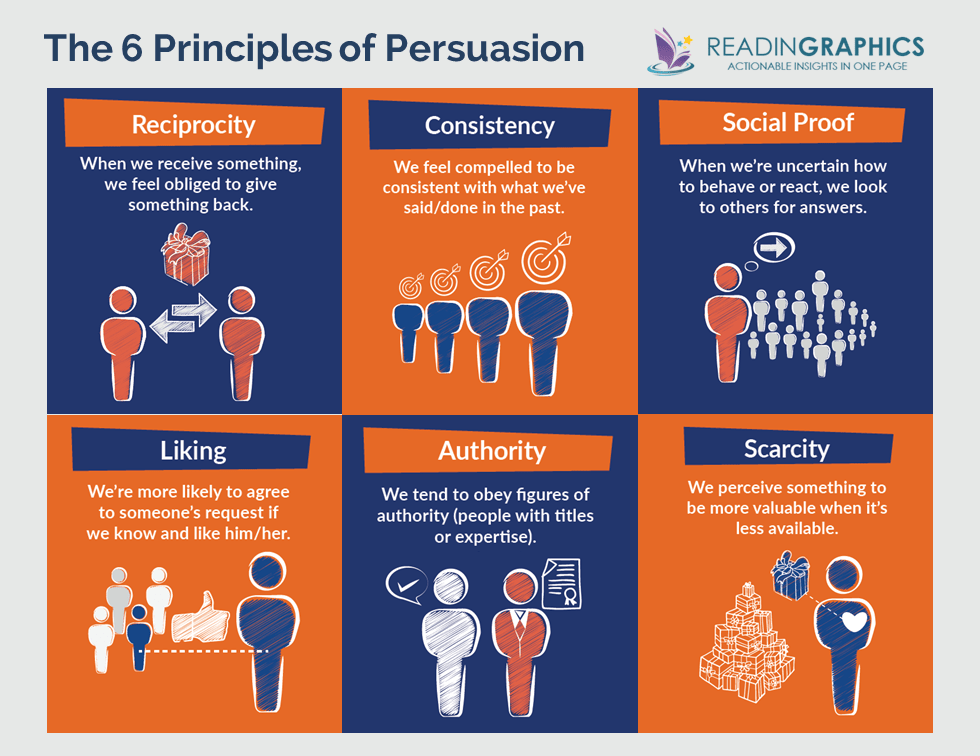
The Psychology of Persuasion in Marketing
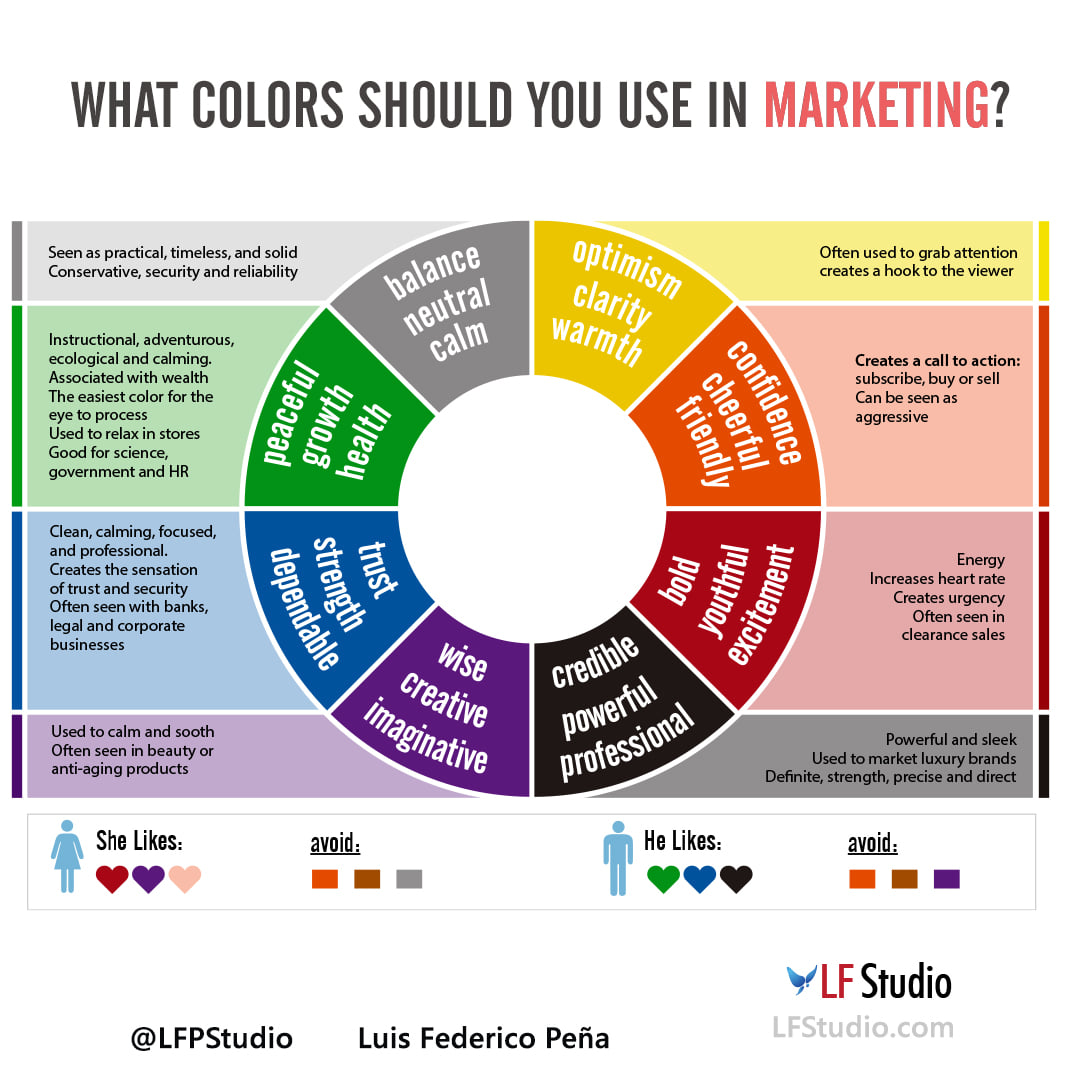
The Influence of Colors on Branding and Marketing Psychology
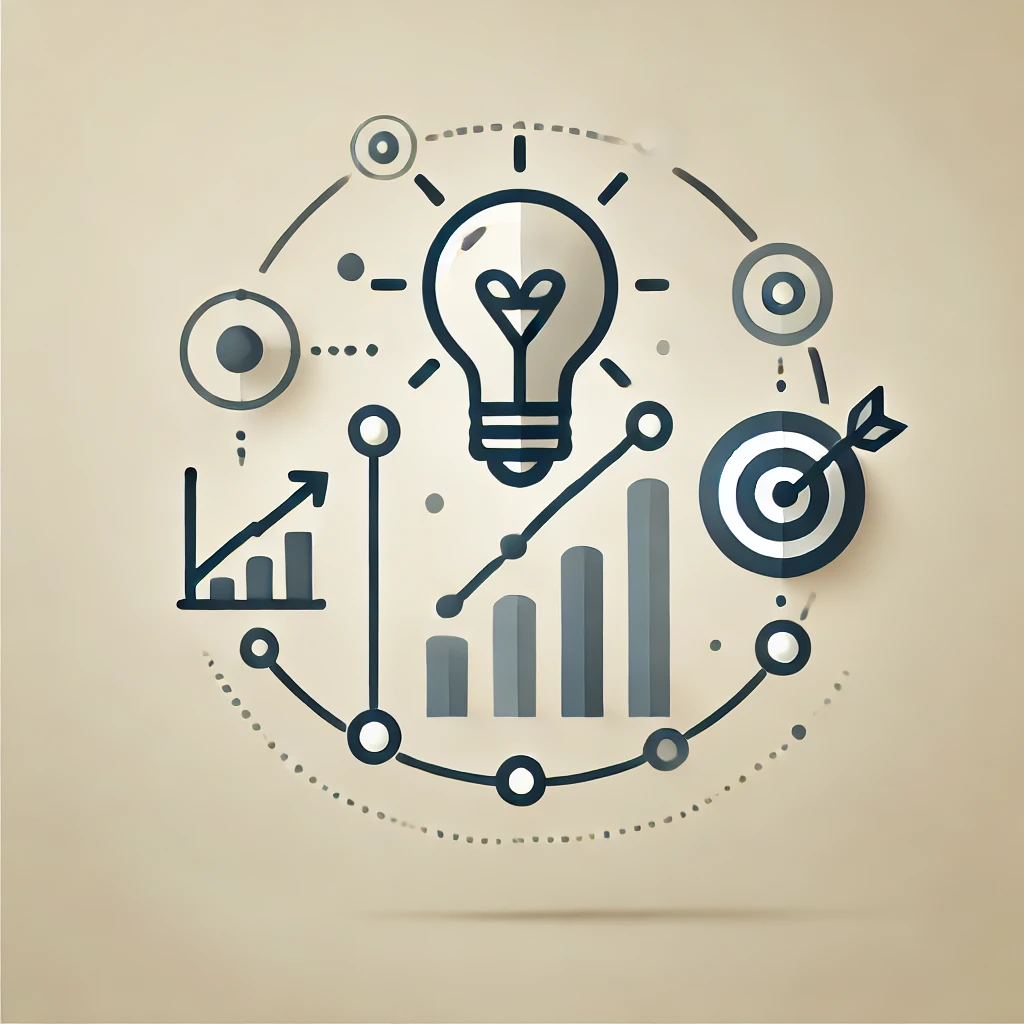