Structural Equation Modeling in Brand Research
Neha still remembers the stunned silence in the boardroom when she presented the brand equity analysis to the executive team. "You're telling me our premium pricing strategy is actually hurting brand loyalty rather than signaling quality?" asked the CEO, visibly troubled. Just moments earlier, Neha had walked them through a structural equation model that revealed an unexpected relationship: while their luxury positioning strengthened perceived quality, it simultaneously weakened emotional connection among core customers. The traditional market research they had relied on had completely missed this relationship because it couldn't distinguish direct from indirect effects. "This is why we've been losing repeat purchases despite quality scores improving," the CMO whispered, realization dawning. That day marked a fundamental shift in how the company understood its brand—transitioning from isolated metrics to a holistic model of interconnected brand dimensions that captured the complex reality of consumer psychology.
Introduction
Brand equity research has evolved dramatically from simple awareness and preference measures to sophisticated models capable of capturing the complex, multidimensional relationships between brand attributes and consumer behavior. At the forefront of this methodological revolution stands Structural Equation Modeling (SEM), a powerful statistical technique that allows marketers to model relationships between variables that cannot be directly observed—such as brand trust, emotional connection, and perceived value. The Marketing Science Institute reports that companies implementing SEM-based brand tracking demonstrate 28% greater accuracy in predicting market share movements than those using traditional methods. As the digital landscape fragments consumer attention and complicates the path to purchase, SEM provides the sophisticated analytical framework needed to understand brand dynamics in increasingly complex markets.
Latent vs. Observed Variables in Brand Frameworks
The foundation of SEM's power in brand research lies in its ability to work with latent variables—theoretical constructs that cannot be directly measured but must be inferred from observable indicators. This distinction is crucial for brand researchers dealing with abstract concepts like brand equity.
The challenge lies in operationalizing these abstract brand constructs. Keller's Customer-Based Brand Equity model theorizes brand resonance as the pinnacle of brand building, but this concept cannot be directly observed. Through SEM, researchers at Interbrand developed measurement models using 6-8 observable indicators (survey items) for each latent construct like brand resonance, allowing for statistical validation of these theoretical relationships.
Nike's brand team demonstrated the practical application of this approach by developing a measurement model for "athletic authenticity"—a crucial brand dimension for their category. Rather than using a single question asking about authenticity (which would be unreliable), they created a latent variable measured through observable indicators including perceived athlete endorsement credibility, technical innovation history, and performance focus. This measurement model explained 42% more variance in purchase intent than single-item measures.
LVMH's luxury brand portfolio management uses what they call "latent segmentation SEM," where latent class analysis identifies consumer segments with different mental models of luxury, and separate structural models are estimated for each segment. This revealed that for traditional luxury consumers, craftsmanship drives willingness to pay, while for emerging luxury consumers, social signaling value is the dominant factor.
Model Fit Indices and Validation Approaches
The validity of SEM-based brand insights depends entirely on how well the specified model fits the observed data. Brand researchers must navigate a complex landscape of fit indices to assess model adequacy.
Comparative Fit Index (CFI), Tucker-Lewis Index (TLI), and Root Mean Square Error of Approximation (RMSEA) form the core assessment toolkit. Unilever's global brand tracking framework requires models to achieve minimal thresholds across multiple indices (CFI/TLI > 0.95, RMSEA < 0.06) before insights are considered actionable, preventing overinterpretation of misspecified models.
Cross-validation addresses the risk of capitalizing on chance relationships in the data. Amazon's brand research team employs k-fold cross-validation, where the model is estimated on training data and validated on held-out samples. Their research revealed that 31% of initially significant brand relationships failed cross-validation tests, highlighting the danger of building strategy on untested models.
Longitudinal invariance testing ensures that brand measurement remains consistent over time. Procter & Gamble's brand equity tracking requires measurement invariance tests before interpreting trend data, after discovering that what consumers understood as "innovation" for their beauty brands had fundamentally shifted over a three-year period, invalidating direct comparisons of scores.
The modification index trap presents a significant hazard, as researchers may be tempted to add parameters simply to improve fit statistics. IBM's brand analytics team implements a strict "theory-first" modification policy, requiring any model adjustments to have theoretical justification beyond statistical improvement, preventing the development of overfit models that fail to generalize.
Real Brand Equity Examples and Applications
The theoretical power of SEM translates into practical brand management applications across diverse industries and brand challenges.
In automotive brand research, Toyota employed SEM to disentangle the impact of their 2010 recall crisis on different brand dimensions. While observed metrics showed uniform damage, their structural model revealed that perceived quality suffered direct damage, while brand trust was affected indirectly through quality perceptions. This insight led to a recovery strategy that first rebuilt quality perceptions through transparent manufacturing changes before addressing trust directly, accelerating their market recovery by an estimated 8 months.
Mastercard's brand team used multi-group SEM to identify which brand elements transferred effectively across cultural contexts. Their analysis revealed that while functional associations transferred relatively intact across markets, emotional associations showed significant cross-cultural variation in structure. This led to a global brand architecture with consistent functional positioning but culturally-adapted emotional selling propositions.
Samsung's transformation from value brand to innovation leader was guided by a longitudinal SEM that tracked how investments in specific product attributes (camera quality, design aesthetics) translated through perceived innovation to overall brand strength. This model identified a crucial 18-month lag between product innovation and brand perception shifts, preventing premature strategy adjustments during their repositioning journey.
Conclusion
As brand ecosystems grow increasingly complex in the digital age, SEM offers marketers a methodological framework capable of matching this complexity. The technique's ability to model unobservable brand constructs, test theoretical relationships, and quantify indirect effects provides insight depth impossible with simpler analytical approaches.
The integration of SEM with big data and artificial intelligence represents the frontier of brand research methodology. Brands like Spotify are now combining traditional survey-based SEM with behavioral data streams, creating hybrid models that link psychological brand perceptions with observed digital behaviors at unprecedented scale.
Call to Action
For marketing leaders seeking to elevate their brand research capabilities:
- Invest in developing technical capacity for advanced modeling techniques through training or strategic hiring
- Review existing brand tracking programs to identify opportunities for deeper structural analysis
- Partner with academic researchers to incorporate latest methodological innovations
- Develop integrated data systems that combine survey and behavioral data for more comprehensive models
- Create learning programs to help senior decision-makers interpret and act on complex model results
The brands that thrive in tomorrow's marketplace will be those that build the analytical infrastructure to understand not just what consumers think about their brand, but why they think it and how these perceptions connect to create holistic brand experiences.
Featured Blogs
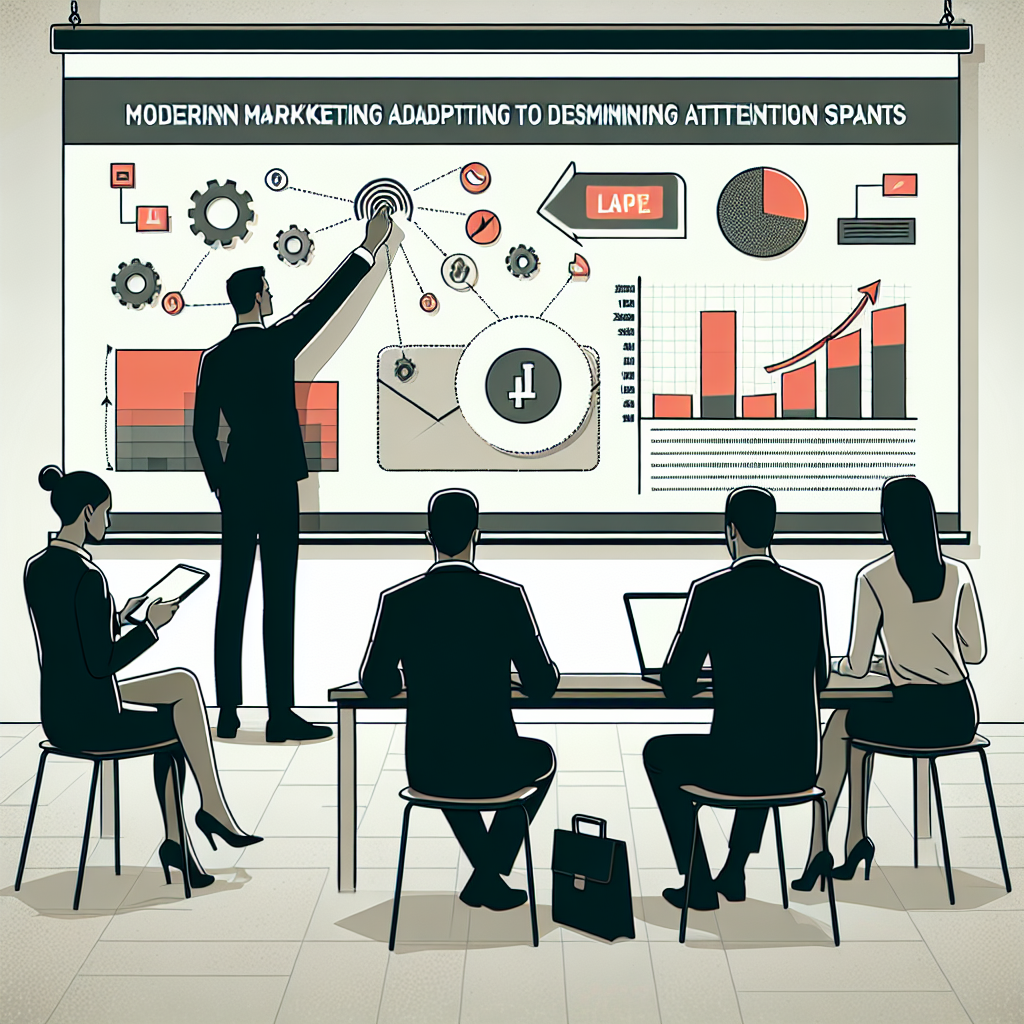
How the Attention Recession Is Changing Marketing
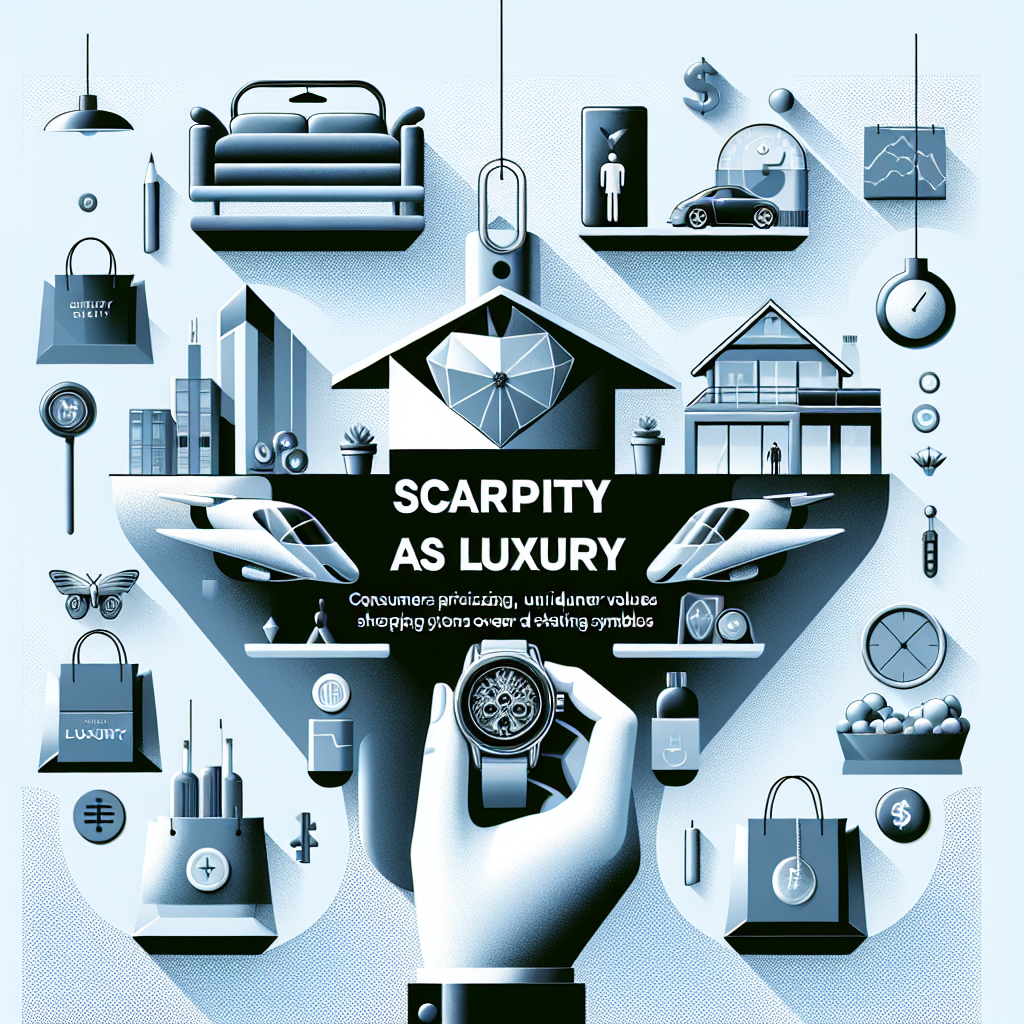
The New Luxury Why Consumers Now Value Scarcity Over Status
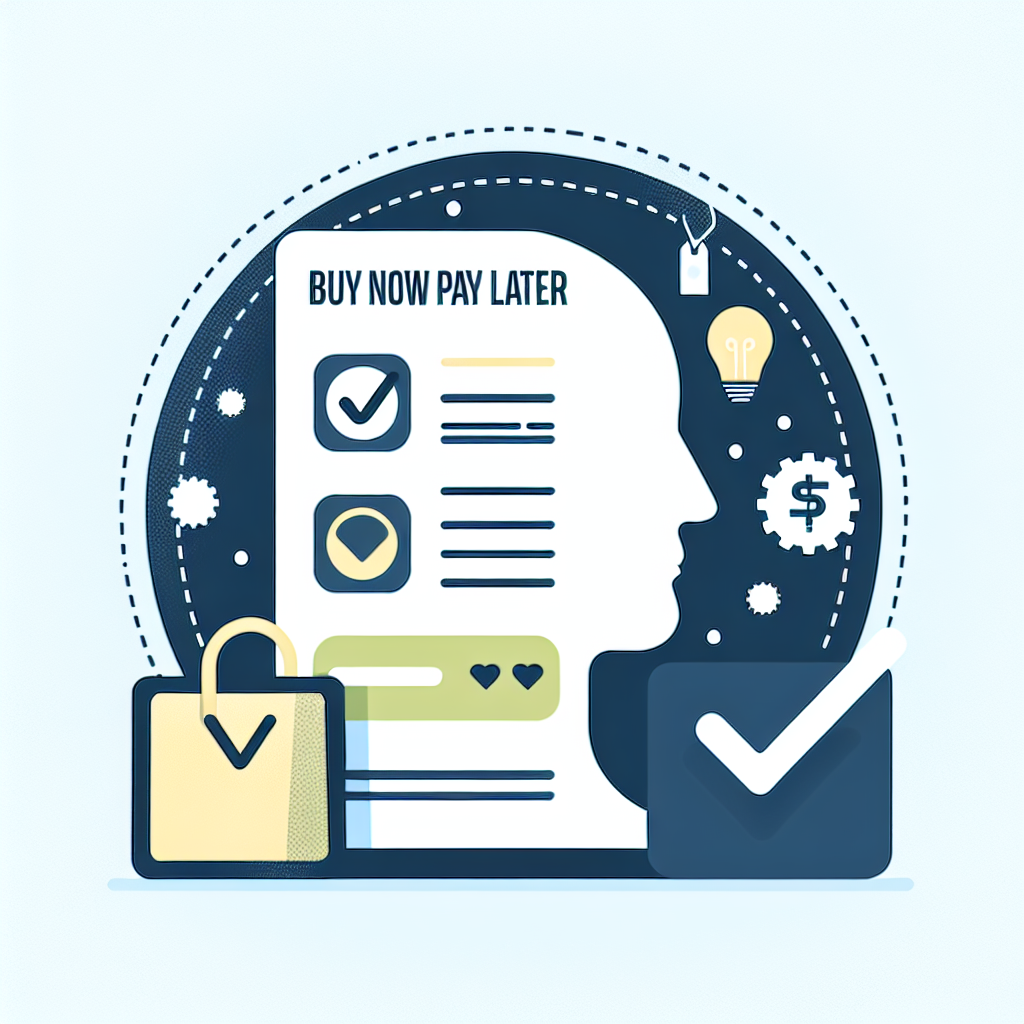
The Psychology Behind Buy Now Pay later
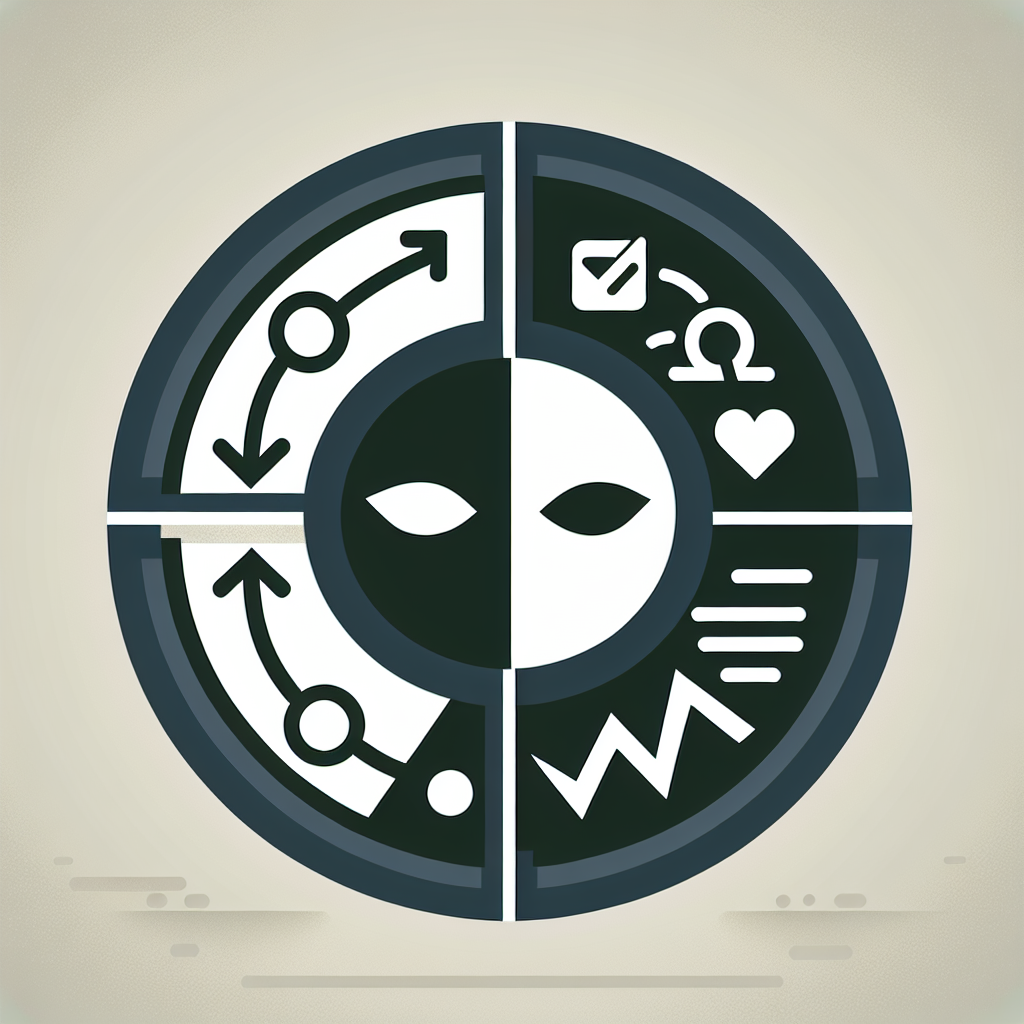
The Role of Dark Patterns in Digital Marketing and Ethical Concerns
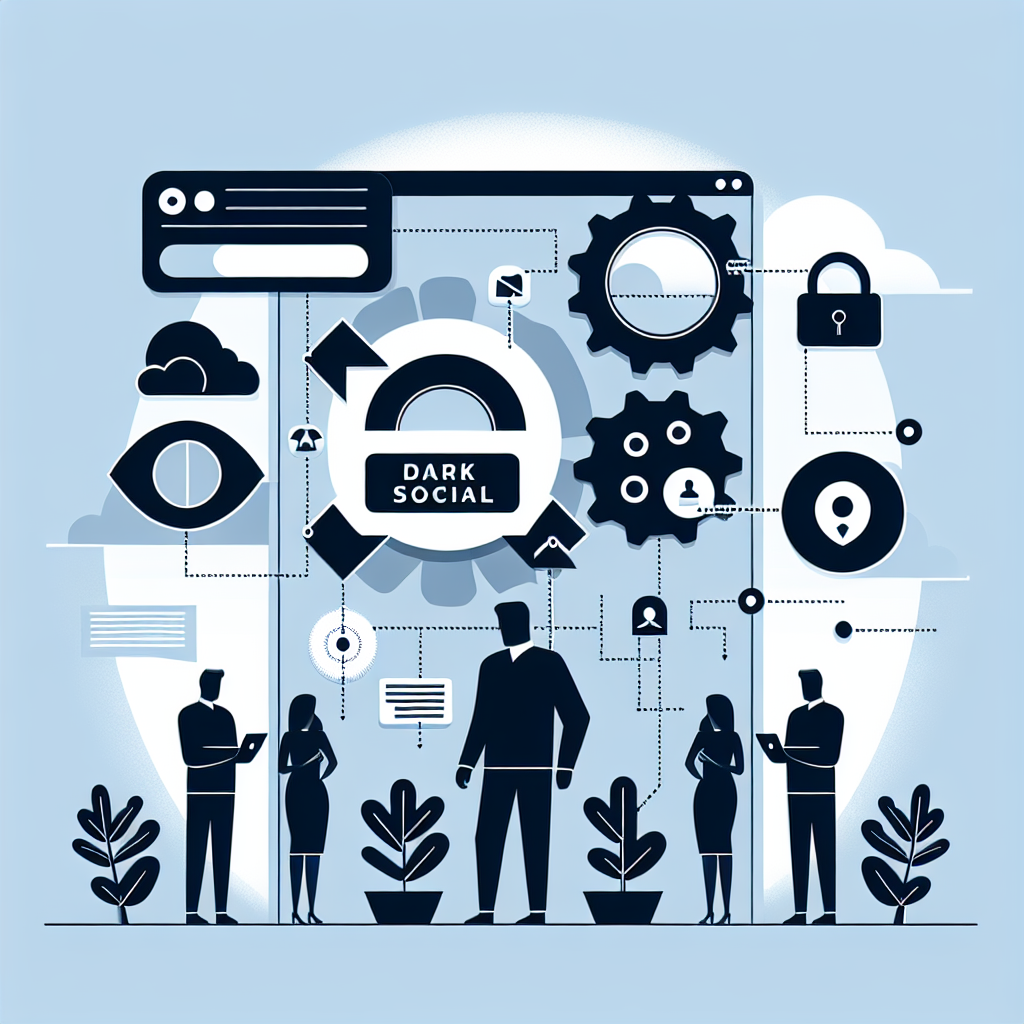
The Rise of Dark Social and Its Impact on Marketing Measurement
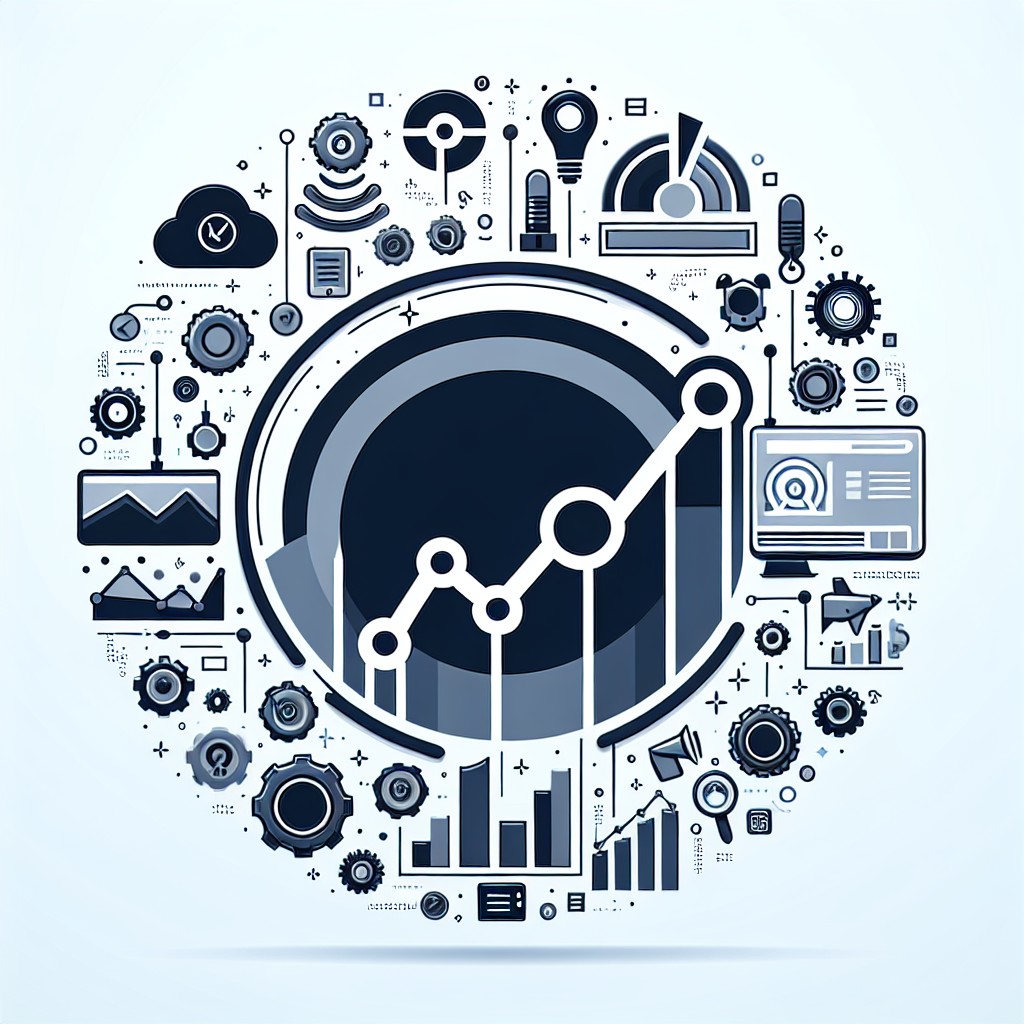
The Future of Retail Media Networks and What Marketers Should Know
Recent Blogs
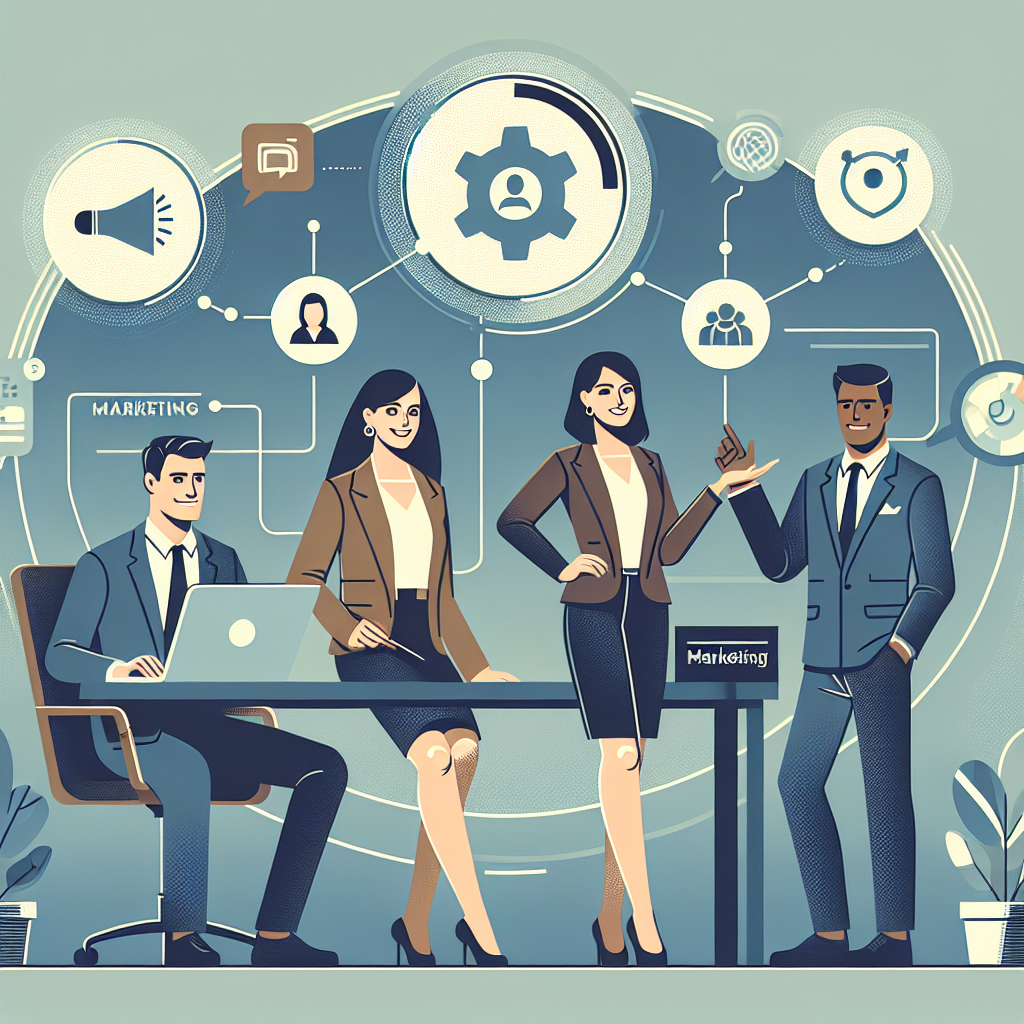
From Service to Experience - Training Teams for the Shift
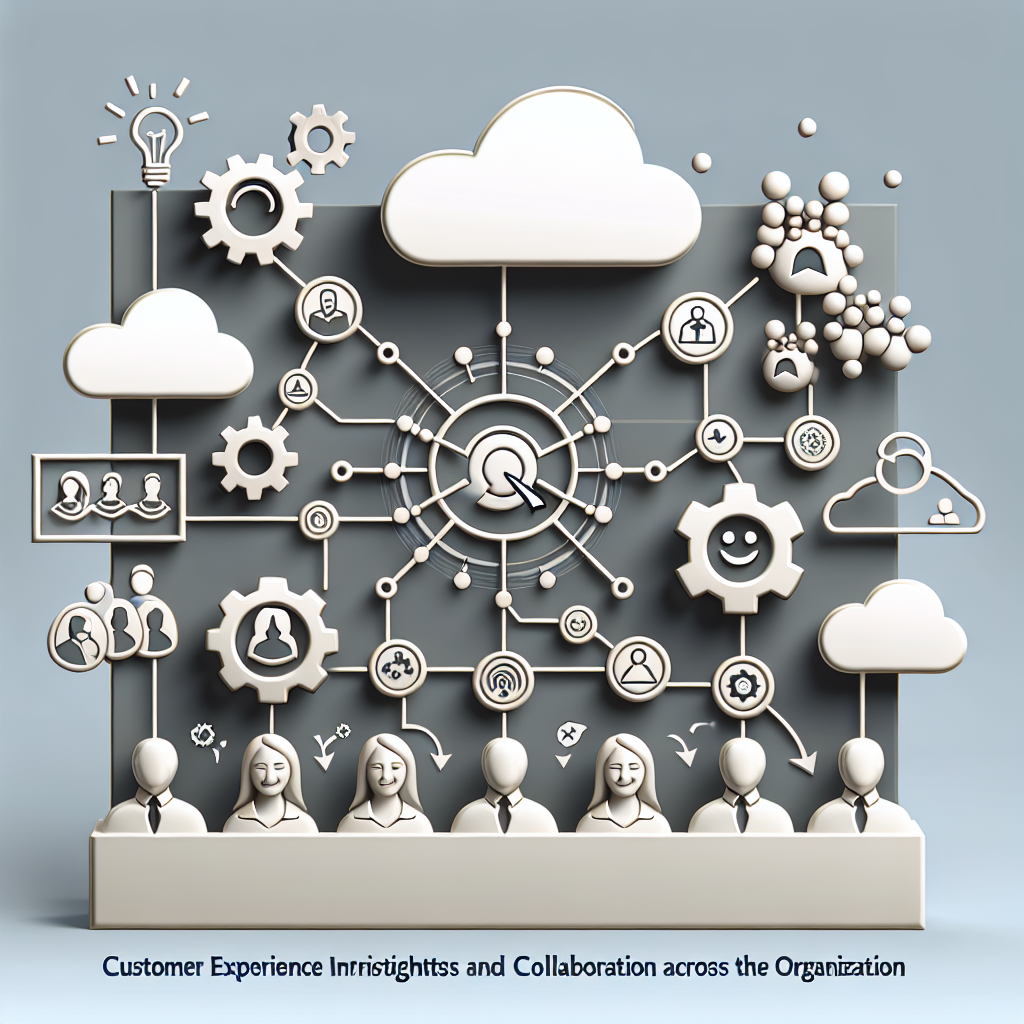
How to Democratize CX Insights Across the Org
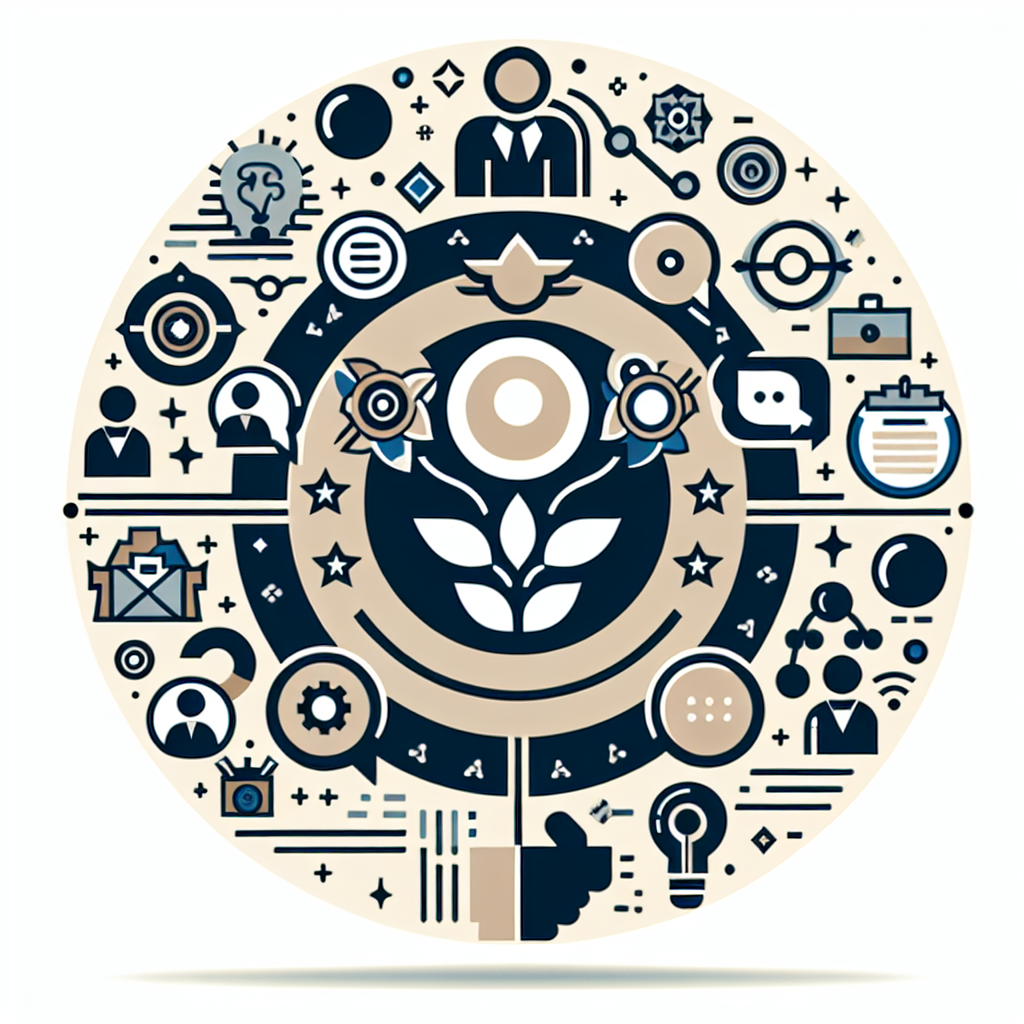
CX is Everyone's Job Building a Customer-Centric Culture
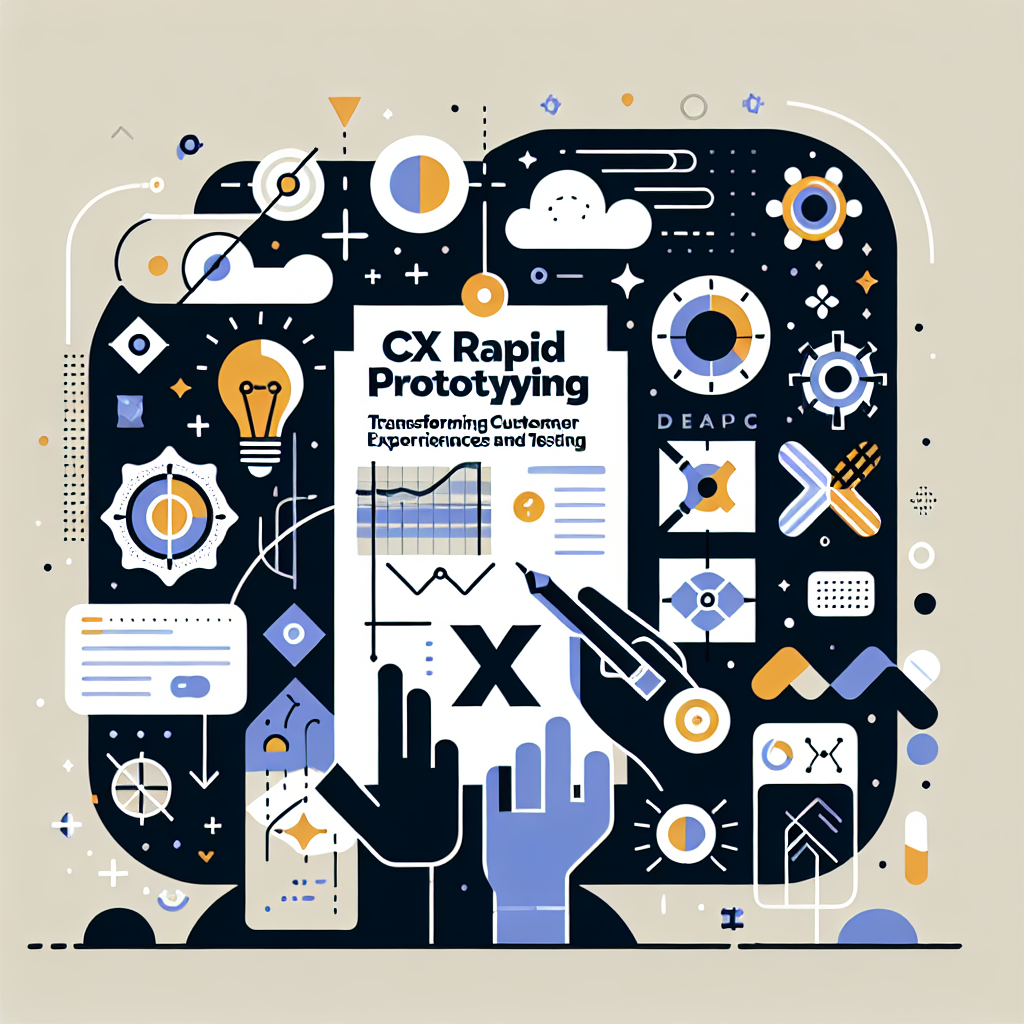
Experimenting with CX Rapid Prototyping for New Journeys
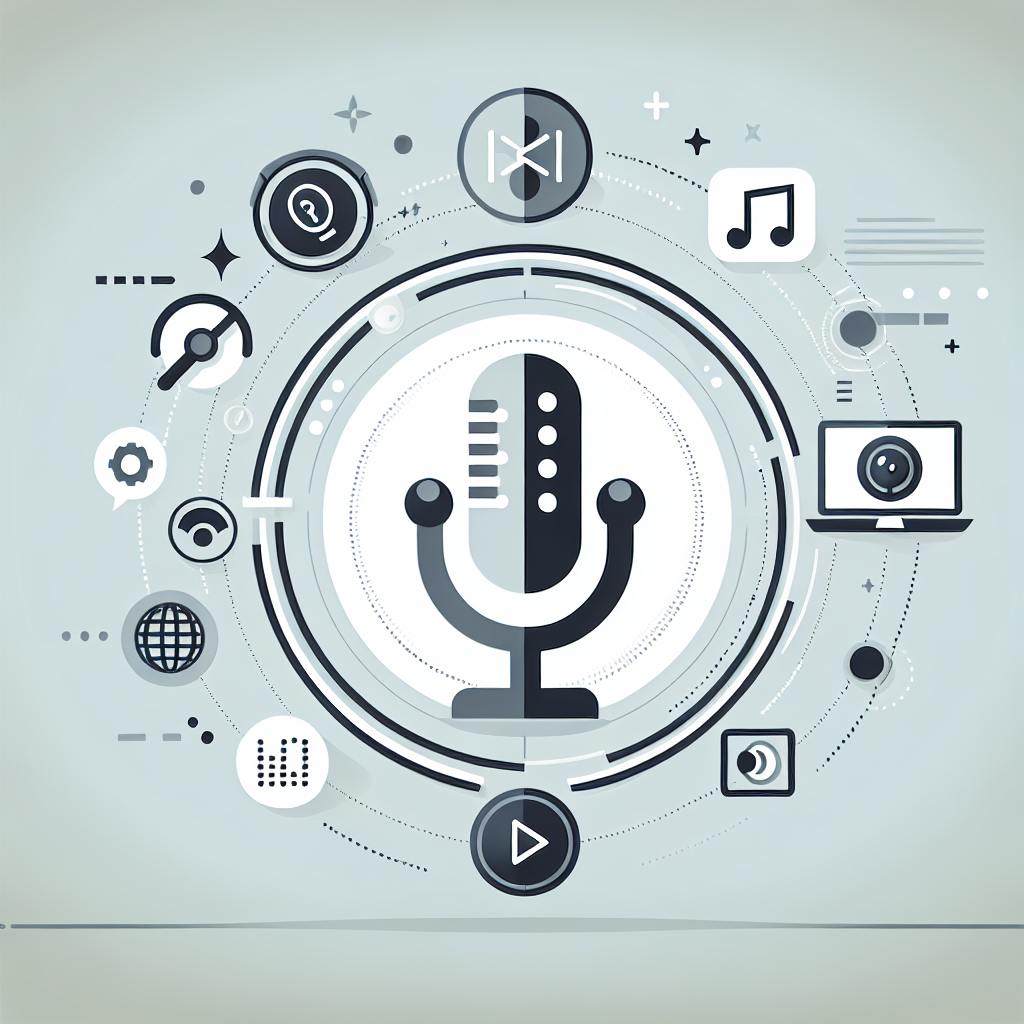
Voice, Video, and Beyond The Multimodal CX Future
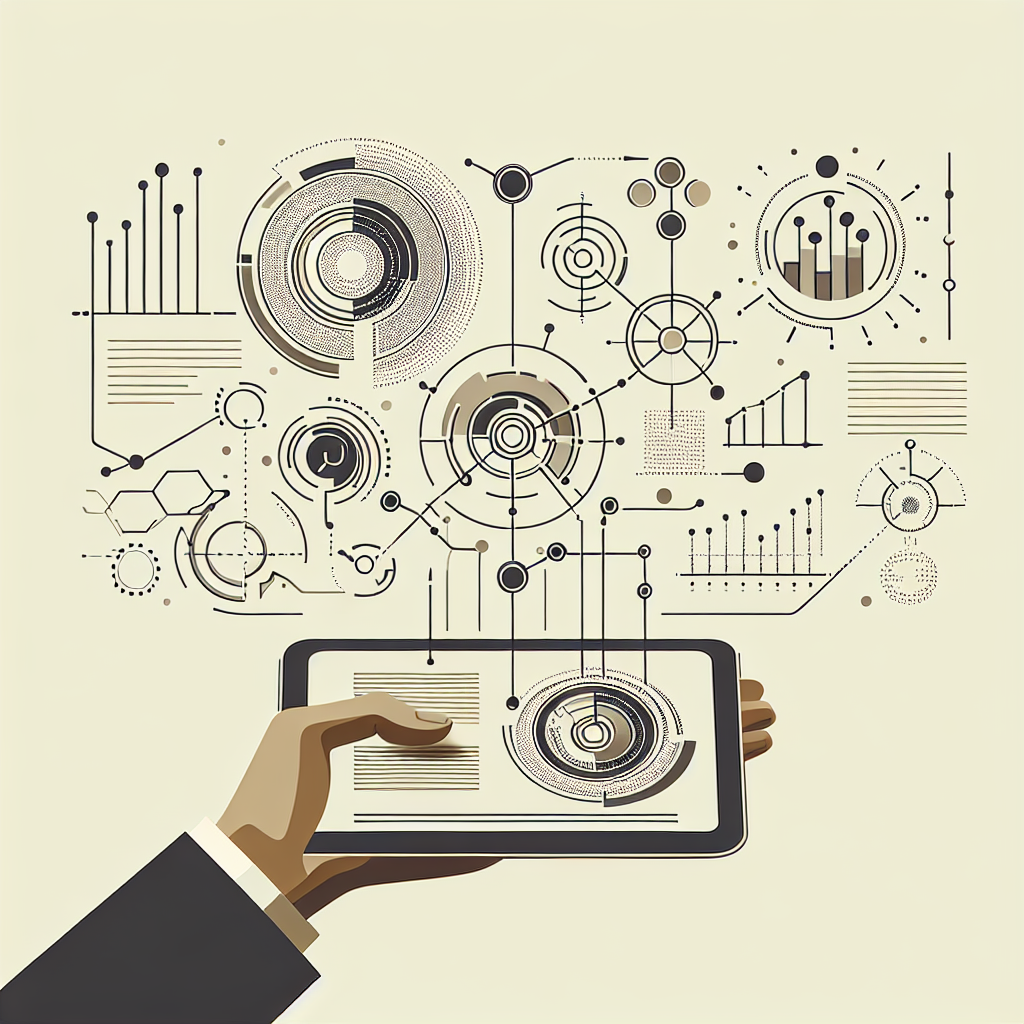