The Role of Hypotheses in Marketing Research
Neha vividly remembers the pivotal moment that transformed her approach to marketing research. She was sitting across from Raj, the brilliant but intimidating CMO of a global consumer electronics company. She had just proudly presented their comprehensive research plan for a new product launch—covering every conceivable consumer dimension with impressive methodological rigor. Raj listened patiently, then asked a simple question: "What are your hypotheses?" The room fell silent. Neha realized they had designed an elaborate research program without clearly articulating what they believed to be true and were specifically testing. "Without hypotheses," Raj explained, "you're not conducting research—you're just collecting data." That conversation fundamentally reshaped Neha's understanding of what makes research truly valuable: the courage to make explicit predictions and systematically test them against reality.
Introduction: The Hypothesis Imperative
At the heart of effective marketing research lies a powerful but often underutilized tool: the hypothesis. More than simply a formal research component, hypotheses represent the bridge between business intuition and empirical validation. They transform vague curiosities into testable propositions and provide the structural framework that elevates data collection from mere observation to strategic insight.
The digital transformation of marketing has dramatically expanded our capacity to collect and analyze consumer data. Paradoxically, this data abundance has made hypothesis-driven research more crucial than ever. In environments characterized by information overload, clear hypotheses provide the focus necessary to extract meaningful insights from the noise. As marketing thought leader Byron Sharp observes, "Without hypotheses, researchers become mere data collectors rather than strategic partners in decision-making."
1. How to Formulate a Hypothesis
The development of effective marketing hypotheses represents both an art and a science, requiring disciplined thinking and creative insight.
Hypothesis Structure
A well-formed marketing hypothesis contains specific elements:
- A clear prediction about a relationship between variables
- Observable and measurable components
- Explicit articulation of expected outcomes
- Sufficient specificity to be genuinely falsifiable
According to research from the Marketing Science Institute, effective hypotheses take the form: "If [specific change/condition], then [specific outcome] because [theoretical rationale]." This structure forces precise thinking about both the expected relationship and the underlying logic.
Hypothesis Sources
Strong marketing hypotheses emerge from multiple origins:
- Prior research findings suggesting relationships worthy of validation
- Theoretical marketing frameworks proposing causal connections
- Observed patterns in existing customer data requiring confirmation
- Business intuition and experience meriting systematic testing
- Competitive analysis suggesting market response mechanisms
McKinsey's Consumer Insights practice notes that the most productive hypotheses often emerge at the intersection of data-driven observation and experienced marketing intuition.
Hypothesis Development Process
Sophisticated marketing organizations follow structured processes for hypothesis development:
- Begin with business objectives and key decisions to be informed
- Identify critical knowledge gaps impacting those decisions
- Formulate preliminary hypotheses addressing those gaps
- Refine hypotheses through cross-functional input
- Prioritize hypotheses based on decision impact and testability
Research by the Advertising Research Foundation indicates that organizations with formal hypothesis development processes demonstrate 37% higher research ROI compared to those without structured approaches.
Digital Era Hypothesis Formation
Modern hypothesis development leverages new capabilities:
- Machine learning-assisted pattern identification suggesting potential relationships
- Advanced analytics revealing unexpected correlations requiring causal validation
- A/B testing platforms enabling rapid preliminary hypothesis evaluation
- Natural language processing of customer feedback generating relationship theories
- Behavioral data mining uncovering potential causal mechanisms
Despite these technological advances, Harvard Business School research demonstrates that hypothesis quality still depends fundamentally on human judgment in interpreting patterns and formulating testable relationships.
2. Null vs. Alternative Hypotheses
The formal structure of hypothesis testing requires understanding the distinctive roles of null and alternative hypotheses in marketing research.
Null Hypothesis Characteristics
The null hypothesis (H₀) serves as the statistical starting point:
- States no effect or no relationship exists between variables
- Represents the status quo or default position
- Functions as what the researcher seeks to disprove
- Provides the statistical benchmark for significance testing
For example, a null hypothesis might state: "There is no difference in purchase intent between customers exposed to messaging variant A versus messaging variant B."
Alternative Hypothesis Characteristics
The alternative hypothesis (Hₐ or H₁) represents the researcher's actual expectation:
- Specifies the relationship or effect the researcher predicts exists
- States what will be accepted if the null is rejected
- Can be directional (predicting the nature of the relationship) or non-directional
- Forms the basis for research design decisions
A corresponding alternative hypothesis might state: "Customers exposed to messaging variant A will demonstrate higher purchase intent than those exposed to variant B."
Strategic Implications of Hypothesis Types
The relationship between these hypothesis types carries important strategic considerations:
- Null hypothesis rejection represents the standard of evidence for action
- Type I errors (falsely rejecting the null) can lead to implementing ineffective strategies
- Type II errors (falsely accepting the null) can mean missing valuable opportunities
- Statistical power (ability to reject a false null) directly impacts decision confidence
According to research published in the Journal of Marketing Research, marketing organizations frequently underestimate the business cost of Type II errors, leading to conservative testing approaches that miss significant opportunities.
Practical Application in Marketing Contexts
In applied marketing research, these formal concepts translate into practical approaches:
- Setting explicit thresholds for what constitutes meaningful differences (not just statistically significant ones)
- Establishing clear decision rules linked to hypothesis outcomes
- Balancing risk tolerance between false positives and false negatives based on decision consequences
- Using one-tailed tests when direction is predicted and important to decision-making
The most sophisticated marketing organizations establish different evidence standards based on decision reversibility, implementation cost, and opportunity value.
3. Hypothesis Testing Techniques
Translating hypotheses into actionable insights requires appropriate testing methodologies aligned with hypothesis types.
Experimental Designs
True experiments provide the strongest causal evidence:
- Randomized controlled trials with treatment and control groups
- A/B/n testing with random assignment and isolated variables
- Multivariate testing for complex hypothesis evaluation
- Field experiments balancing control with real-world validity
Digital platforms have dramatically expanded experimental capabilities, with companies like Amazon reportedly running over 10,000 controlled experiments annually to test specific hypotheses about customer behavior.
Quasi-Experimental Approaches
When full experimental control isn't possible:
- Natural experiments leveraging external events
- Regression discontinuity designs
- Propensity score matching
- Instrumental variable analysis
- Difference-in-differences methods
These approaches, while less definitive than true experiments, provide stronger evidence than purely observational methods.
Statistical Analysis Frameworks
Different hypotheses require appropriate analytical methods:
- T-tests and ANOVA for mean difference hypotheses
- Chi-square tests for categorical relationship hypotheses
- Regression analysis for relationship strength hypotheses
- Time series analysis for trend and pattern hypotheses
- Structural equation modeling for complex causal hypotheses
The Marketing Accountability Standards Board emphasizes that analysis method selection should directly align with hypothesis structure rather than defaulting to familiar techniques.
Digital-Era Testing Innovations
Contemporary testing approaches leverage advanced capabilities:
- Machine learning-enhanced causal inference
- Algorithmic experimental design optimization
- Automated multivariate testing platforms
- Bayesian updating for continuous hypothesis refinement
- Synthetic control methods for counterfactual analysis
Despite technological advances, the Journal of Marketing Analytics notes that hypothesis testing fundamentals remain unchanged—only the efficiency and sophistication of implementation have evolved.
Hypothesis Testing Process Flow
Effective hypothesis testing follows a structured sequence:
- Establish clear acceptance/rejection criteria before testing
- Design research methodology specifically aligned with hypothesis structure
- Collect data with sample size determined by required statistical power
- Analyze results against predetermined significance thresholds
- Draw conclusions about hypothesis support or rejection
- Translate statistical outcomes into business implications
Organizations with formalized hypothesis testing processes demonstrate significantly higher rates of research utilization in decision-making compared to those with ad hoc approaches.
Conclusion: Hypotheses as Strategic Assets
In the increasingly data-saturated marketing environment, hypotheses serve not merely as technical research components but as valuable strategic assets. They transform nebulous questions into testable propositions, focus research resources on decision-critical uncertainties, and provide the essential structure that converts data into actionable insight.
As marketing continues its digital transformation, the fundamental importance of hypothesis-driven research only increases. While technology enables more sophisticated testing approaches and broader data collection, the intellectual discipline of articulating clear, testable relationships remains the foundation of research that drives genuine business impact.
The organizations achieving superior marketing performance in complex environments are those that treat hypothesis development with the same strategic importance as they do data collection and analysis—recognizing that research value ultimately depends not on how much we measure, but on how well we formulate and test what we believe.
Call to Action
For marketing professionals seeking to elevate their research impact through hypothesis-driven approaches:
- Implement hypothesis workshops before designing major research initiatives
- Create hypothesis libraries documenting tests and outcomes for organizational learning
- Develop hypothesis quality criteria for research proposal evaluation
- Establish hypothesis-to-decision mapping to ensure research relevance
- Invest in training that builds hypothesis development skills across marketing teams.
Featured Blogs
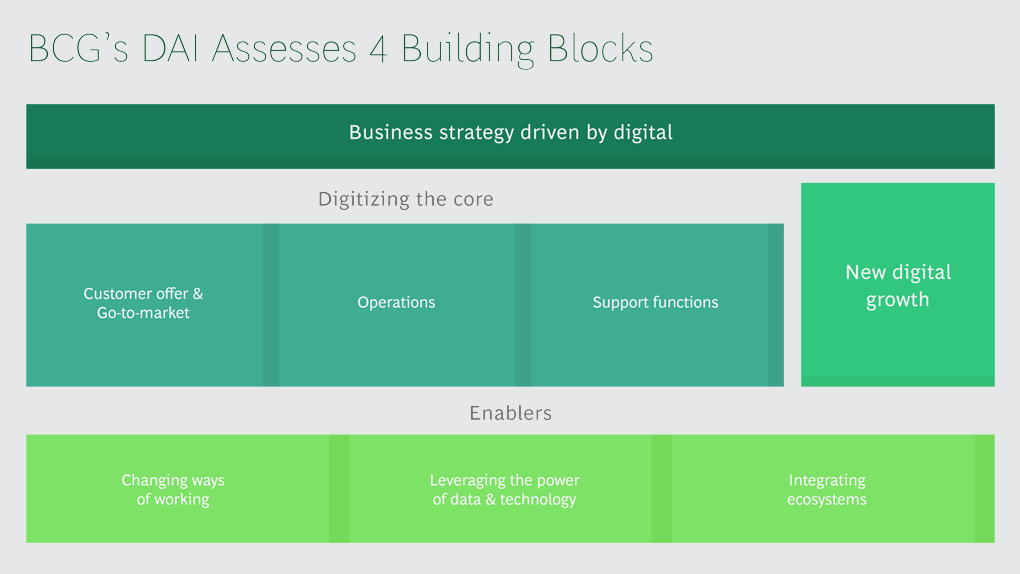
BCG Digital Acceleration Index
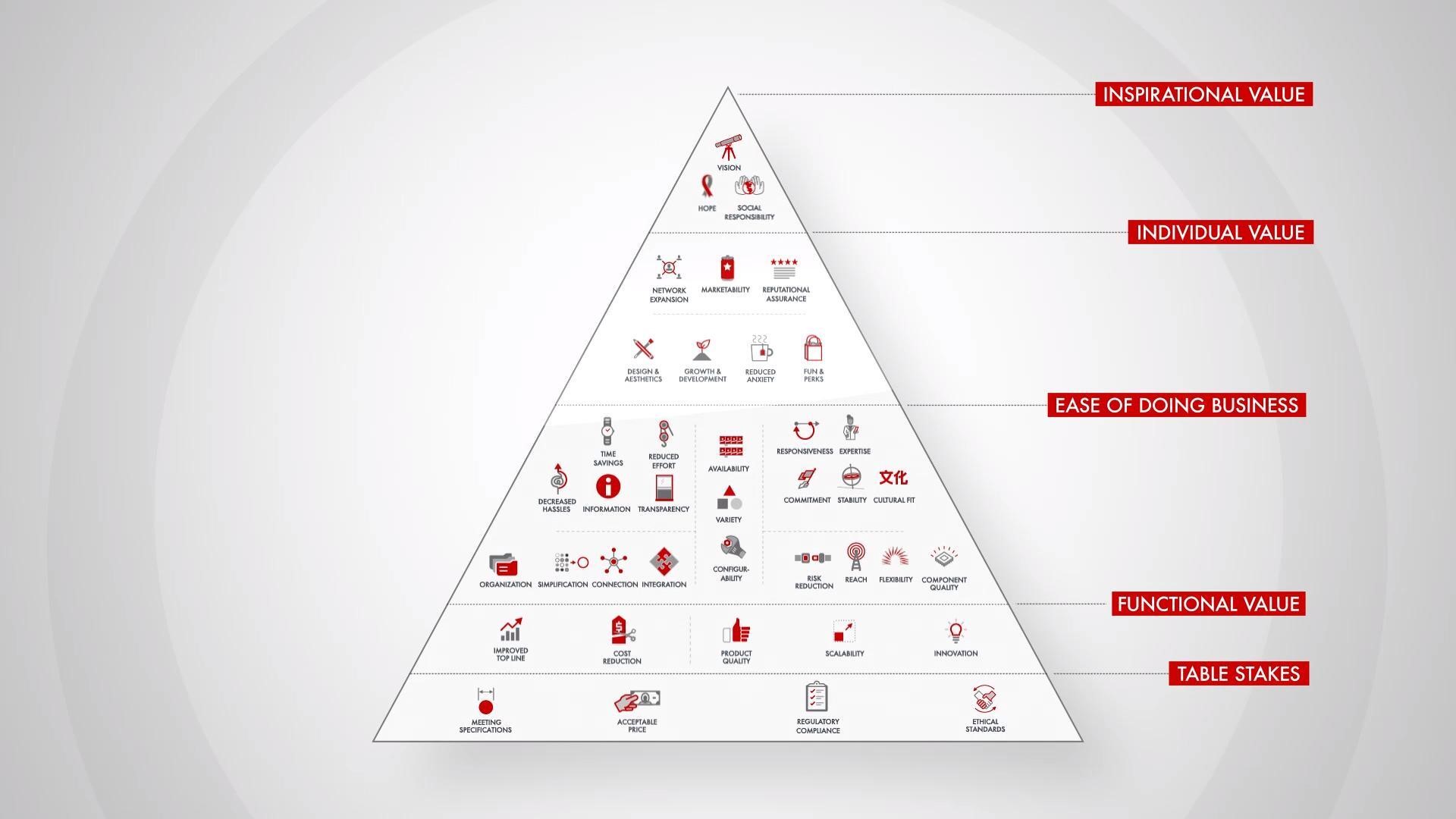
Bain’s Elements of Value Framework
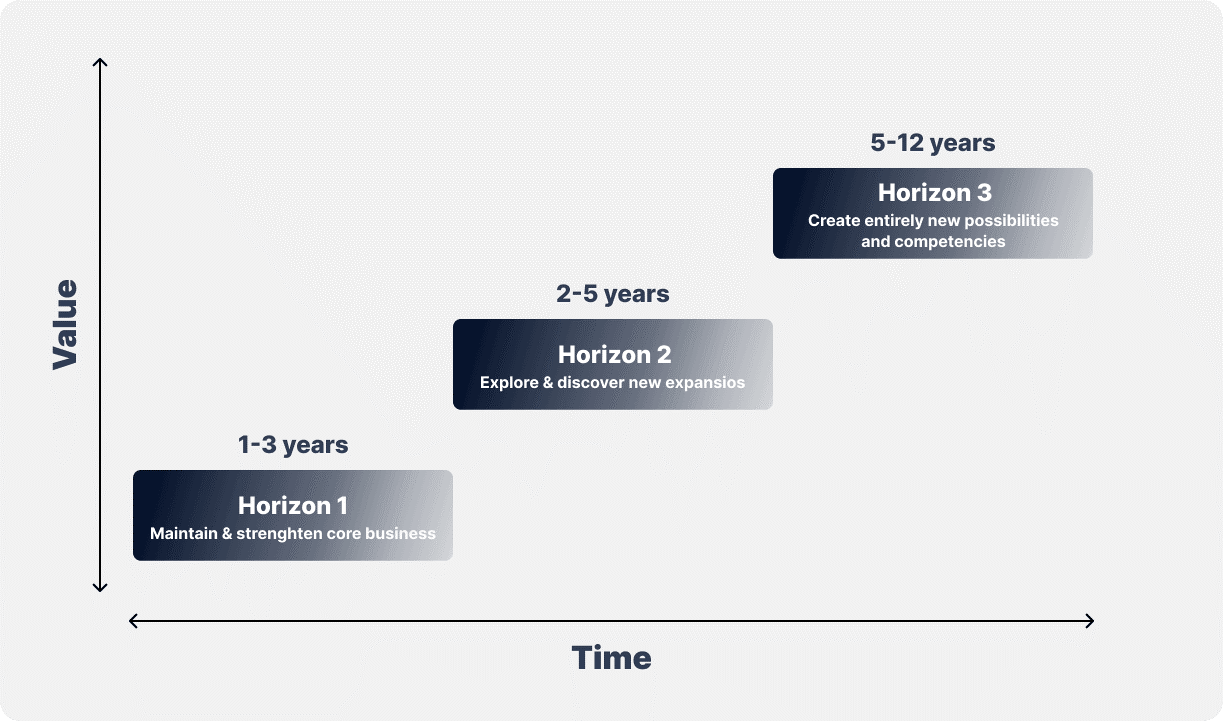
McKinsey Growth Pyramid
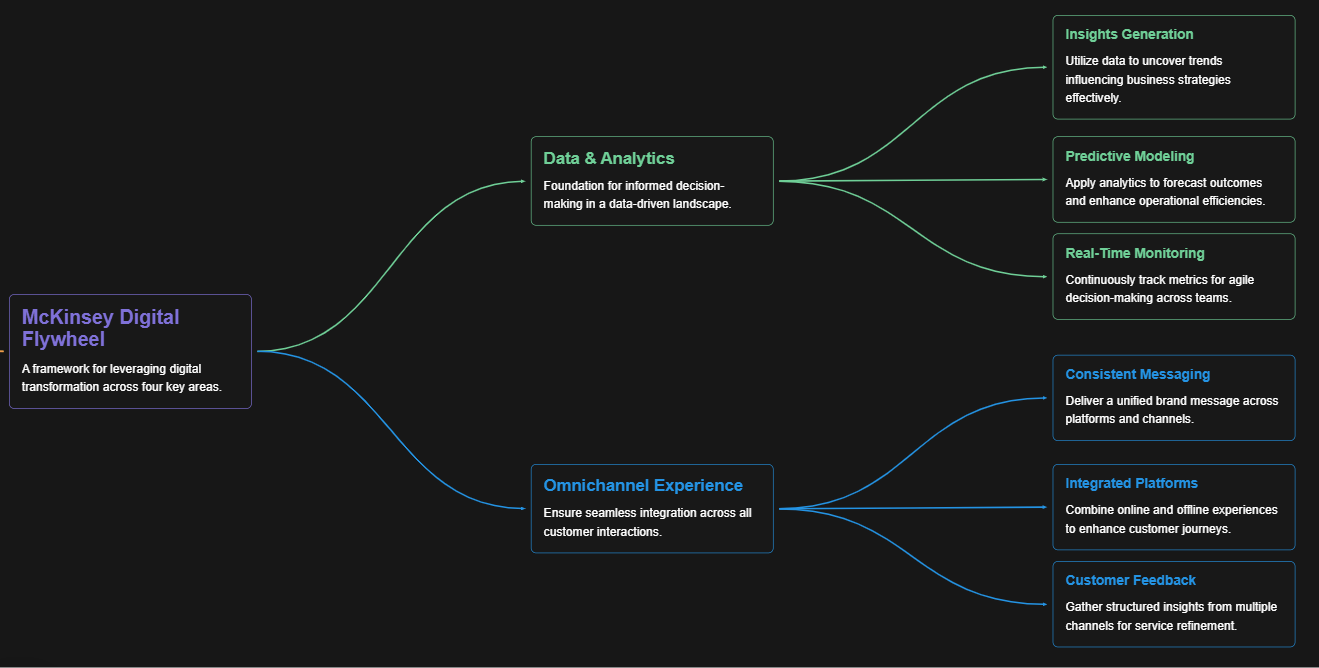
McKinsey Digital Flywheel
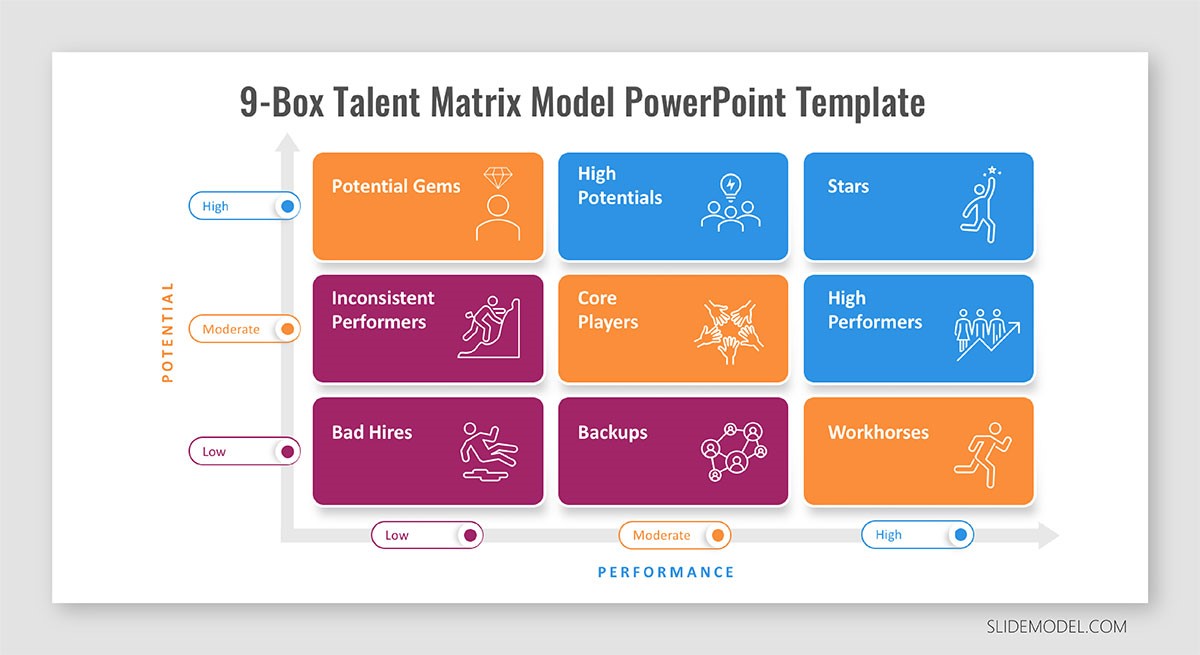
McKinsey 9-Box Talent Matrix
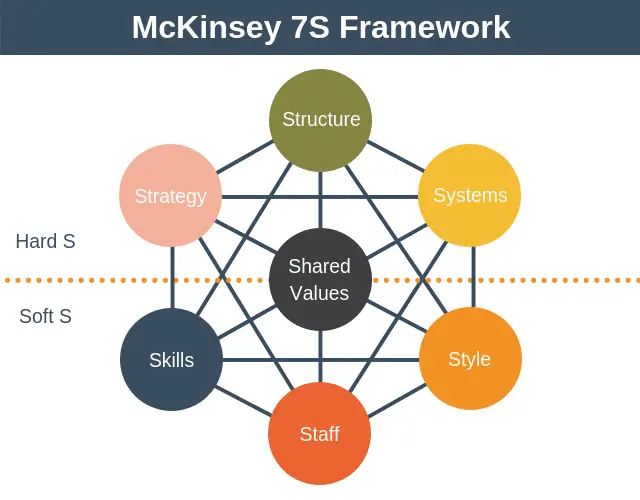
McKinsey 7S Framework
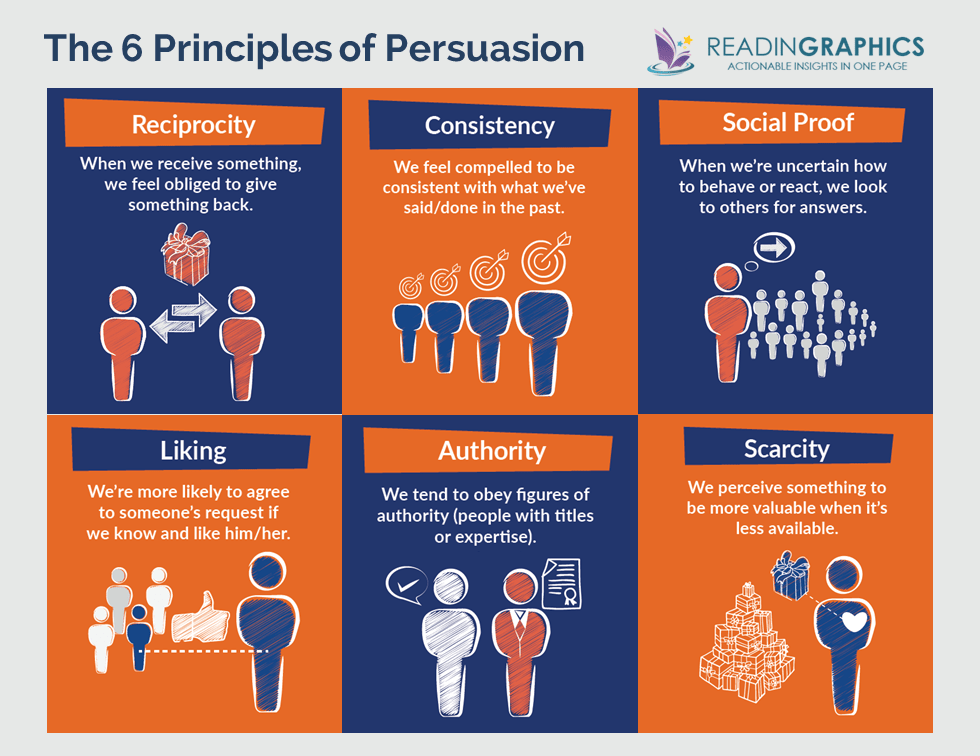
The Psychology of Persuasion in Marketing
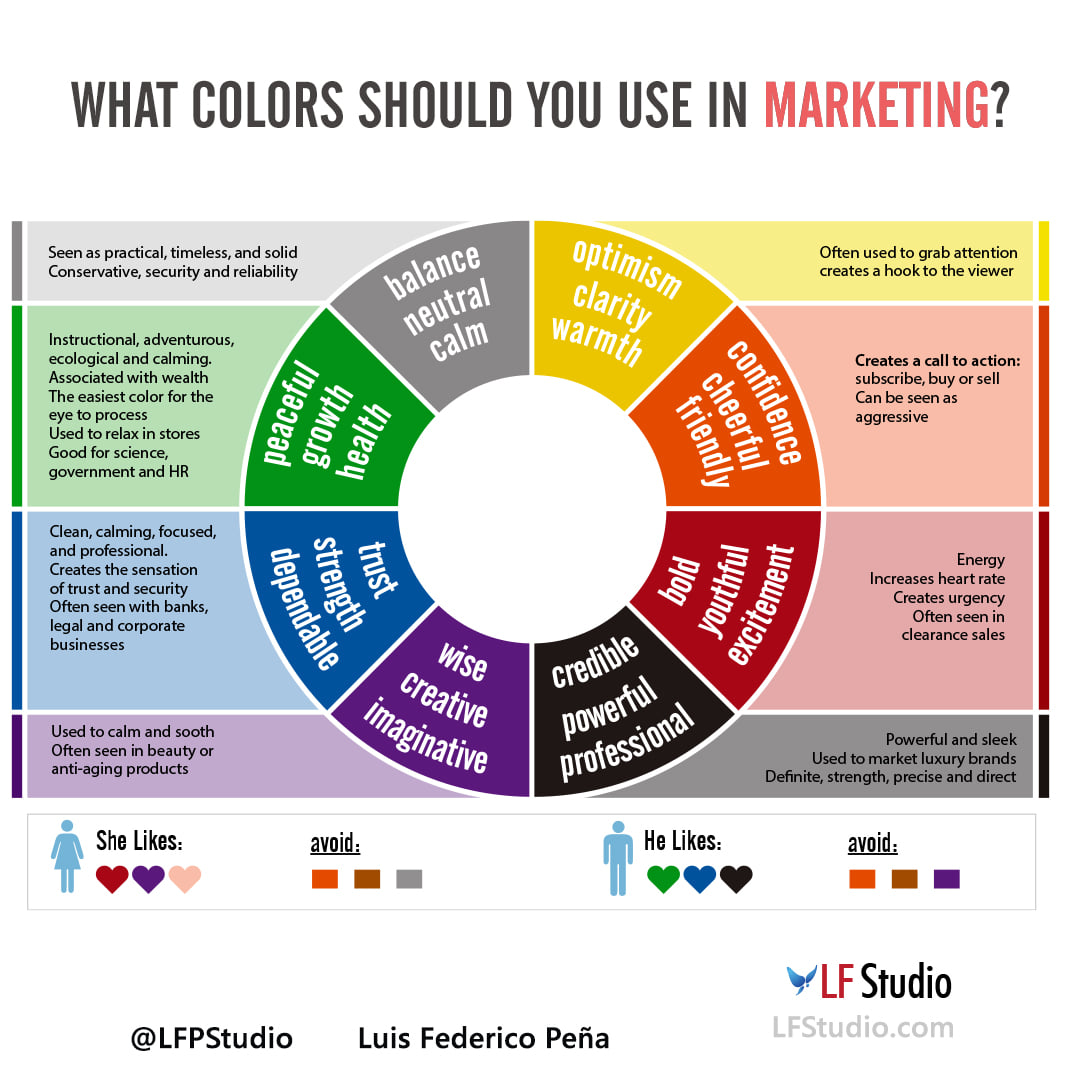
The Influence of Colors on Branding and Marketing Psychology
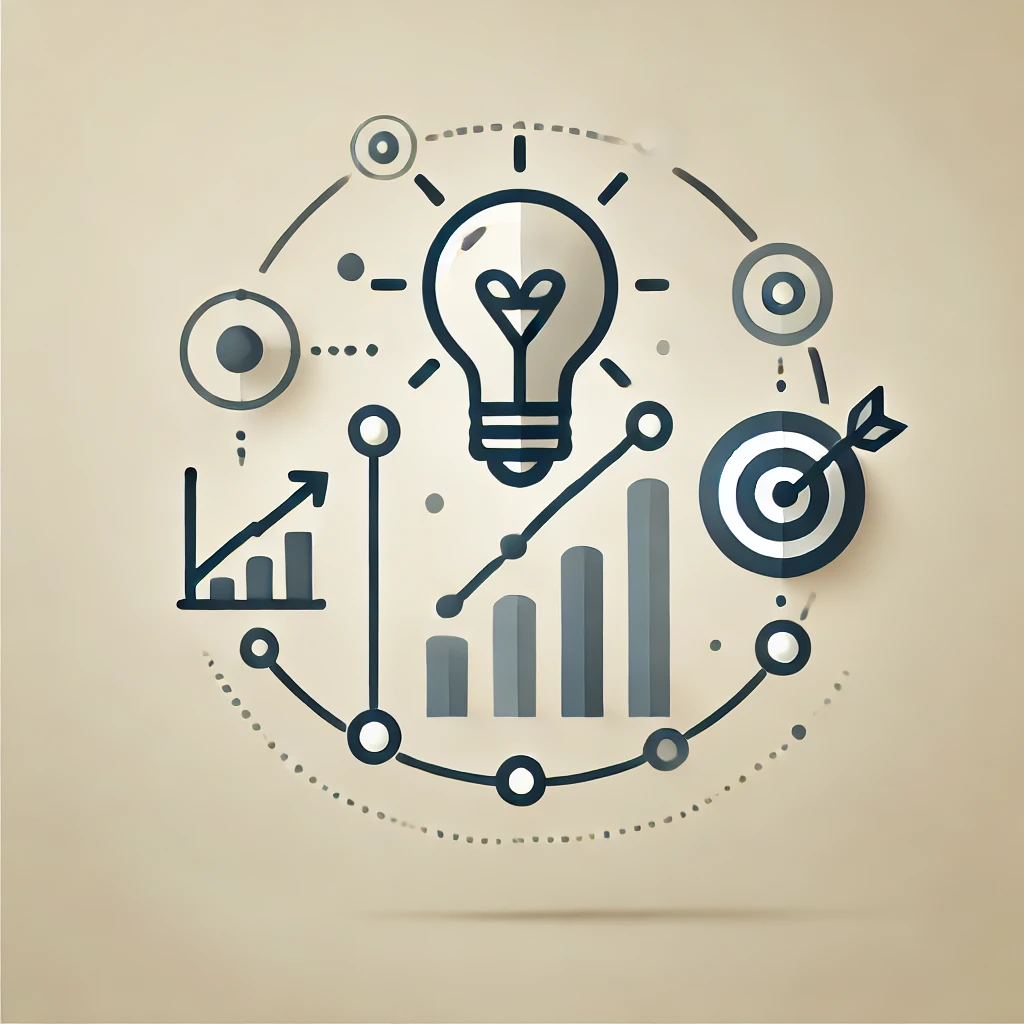