Using AI to Personalize Customer Touchpoints
I was having dinner with my college roommate who recently joined the digital team at a major retailer. As we caught up, she shared a story that illustrated how dramatically AI has transformed customer experience. "Last month, we had a customer service issue that would have been a disaster in the past," she explained. "A shipment of anniversary gifts was delayed nationwide due to weather. Our AI system identified affected customers, analyzed their purchase history and preferences, then automatically generated personalized apology messages with compensatory offers tailored to each customer's interests." What impressed me wasn't just the operational efficiency, but how the system made decisions a thoughtful human would make—considering the emotional context of an anniversary purchase versus routine shopping. The technology wasn't just processing data; it was interpreting human meaning behind the transactions.
Introduction: The Evolution of AI-Driven Personalization
Customer experience personalization has progressed through distinct evolutionary stages: from basic demographic targeting to rules-based segmentation, from behavioral triggers to predictive modeling, and now to the frontier of artificial intelligence that can interpret context, anticipate needs, and generate appropriate responses across the entire customer journey. This evolution represents not just technological advancement but a fundamental shift in how organizations understand and respond to customer behavior.
Research from the Northwestern Kellogg School of Management indicates that AI-driven personalization increases customer engagement by 40% compared to rules-based approaches, while MIT Sloan's analysis reveals a 38% improvement in conversion rates and 30% higher customer lifetime value. However, these metrics only begin to capture the transformative potential of systems that continuously learn from customer interactions while respecting privacy boundaries.
1. Contextual Understanding Through Advanced AI
Modern AI systems develop rich understanding of customer context beyond explicit data.
a) Multi-Dimensional Customer Modeling
Advanced AI systems incorporate:
- Longitudinal pattern recognition across touchpoints and time
- Emotional state inference from interaction signals
- Intent modeling beyond explicit search or navigation cues
- Relationship strength assessment based on engagement patterns
Example: Financial services provider American Express implemented an AI system called "Customer Intelligence Platform" that synthesizes transaction history, service interactions, digital engagement patterns, and external economic factors to create holistic customer understanding. The system detects subtle changes in spending patterns that might indicate life events, financial concerns, or evolving needs, triggering appropriate engagement. This approach increased preemptive service interventions by 58% and relevant offer acceptance by 41%.
b) Natural Language Understanding for Sentiment Analysis
Sophisticated language processing enables:
- Emotional tonality detection in customer communications
- Urgency and priority assessment from linguistic patterns
- Satisfaction prediction from communication subtleties
- Personality trait inference from communication style
Example: Telecommunications company T-Mobile deployed natural language understanding AI across customer service channels to analyze not just what customers say but how they express themselves. The system detects frustration signals in chat interactions and adjusts response strategies accordingly—sometimes offering more technical detail, sometimes streamlining to faster resolution. This capability reduced escalation rates by 37% and improved first-contact resolution by 28%.
2. Predictive Personalization Across Touchpoints
AI enables forward-looking personalization rather than reactive responses.
a) Anticipatory Experience Design
Advanced predictive systems enable:
- Next-need anticipation based on behavior patterns
- Proactive issue prevention through early signal detection
- Journey stage prediction and appropriate content staging
- Life event anticipation and relevant offer preparation
Example: Banking group HSBC implemented an "Anticipatory Banking" AI system that analyzes transaction patterns, life stage indicators, and external factors to predict upcoming financial needs. When the system detects patterns suggesting an impending home purchase, it prepares personalized mortgage information and makes it prominently accessible through the customer's preferred channels. This approach has increased product adoption by 31% and strengthened primary banking relationships by 24%.
b) Dynamic Journey Orchestration
Sophisticated orchestration systems deliver:
- Real-time journey adaptation based on detected signals
- Cross-channel experience coordination through central intelligence
- Touchpoint sequencing optimization based on receptivity patterns
- Timing adjustments based on engagement propensity modeling
Example: Retailer Target developed an AI-powered "Journey Orchestrator" that coordinates personalization across email, app, website, and in-store experiences. Rather than treating each channel as a separate personalization opportunity, the system maintains consistent but complementary messaging across touchpoints while optimizing delivery timing based on individual engagement patterns. This approach increased multi-channel engagement by 46% and improved conversion rates by 32%.
3. Generative AI for Experience Creation
The newest frontier uses AI to create rather than just select personalized experiences.
a) Dynamic Content Generation
Advanced generative systems enable:
- Personalized messaging with individual relevance
- Custom visual asset creation based on preferences
- Individualized offer structuring reflecting value perception
- Tailored educational content matching learning styles
Example: E-commerce platform Shopify introduced "Personal Copywriter," an AI system that generates completely individualized product descriptions based on each customer's browsing history, past purchases, and engagement patterns. Rather than selecting from pre-written content variants, the system creates unique descriptions emphasizing features and benefits most relevant to each customer. This capability increased product detail page engagement by 37% and conversion rates by 24%.
b) Adaptive Interface Generation
Sophisticated interface personalization includes:
- Navigation structure optimization for individual usage patterns
- Information hierarchy adaptation based on interest signals
- Visual design adjustment reflecting preference patterns
- Functionality emphasis reflecting task priorities
Example: Streaming service Netflix expanded their recommendation engine to personalize not just content suggestions but the entire interface experience. The "Adaptive UI" system dynamically adjusts category organization, content preview styles, and navigation emphasis based on individual usage patterns and preferences. This approach increased content discovery by 29% and reduced browsing abandonment by 18%.
Conclusion: The Future of AI-Personalized Experiences
As AI systems continue to evolve, we're witnessing the emergence of what futurist Kevin Kelly calls "cognified experiences"—interactions so intelligently personalized they feel like engaging with someone who knows you deeply while respecting your boundaries. The most sophisticated implementations achieve personalization that enhances rather than diminishes human connection, creating what the Harvard Business Review terms "augmented relationships" where technology amplifies rather than replaces human understanding.
The future belongs to organizations that view AI not as a mechanism for extracting more customer value but as a means of creating more customer-centered experiences. As my friend's story illustrates, the true power of AI lies not in operational efficiency but in enabling organizations to respond with human-like understanding at technological scale.
Call to Action
For customer experience leaders implementing AI personalization:
- Develop clear ethical frameworks governing how AI interprets and acts on customer data
- Implement progressive permission models giving customers control over personalization depth
- Create measurement systems that evaluate both immediate performance metrics and relationship quality indicators
- Build cross-functional teams combining data science expertise with human-centered design thinking
- Establish governance ensuring AI systems enhance rather than replace human connection points where emotional intelligence matters most.
Featured Blogs
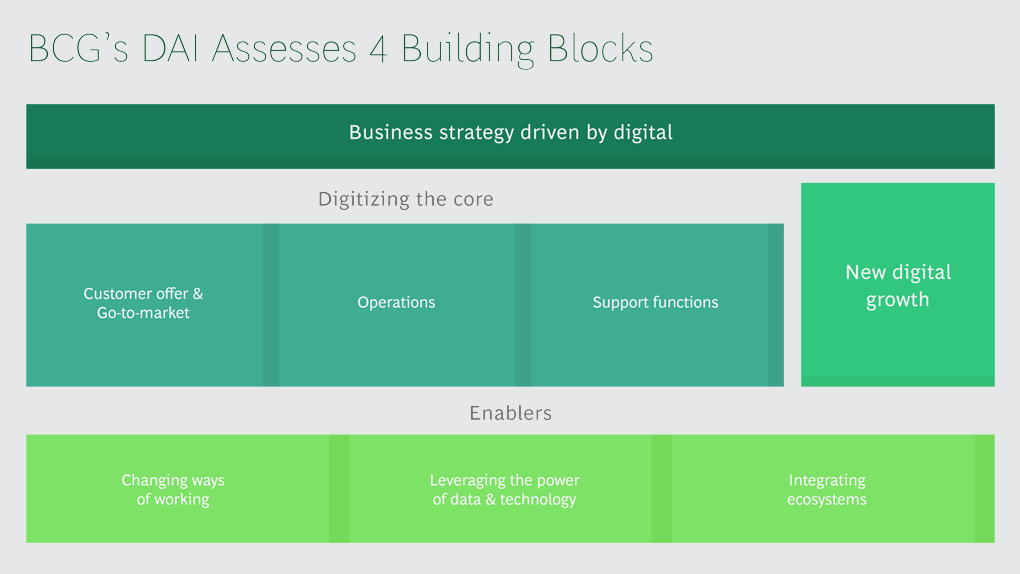
BCG Digital Acceleration Index
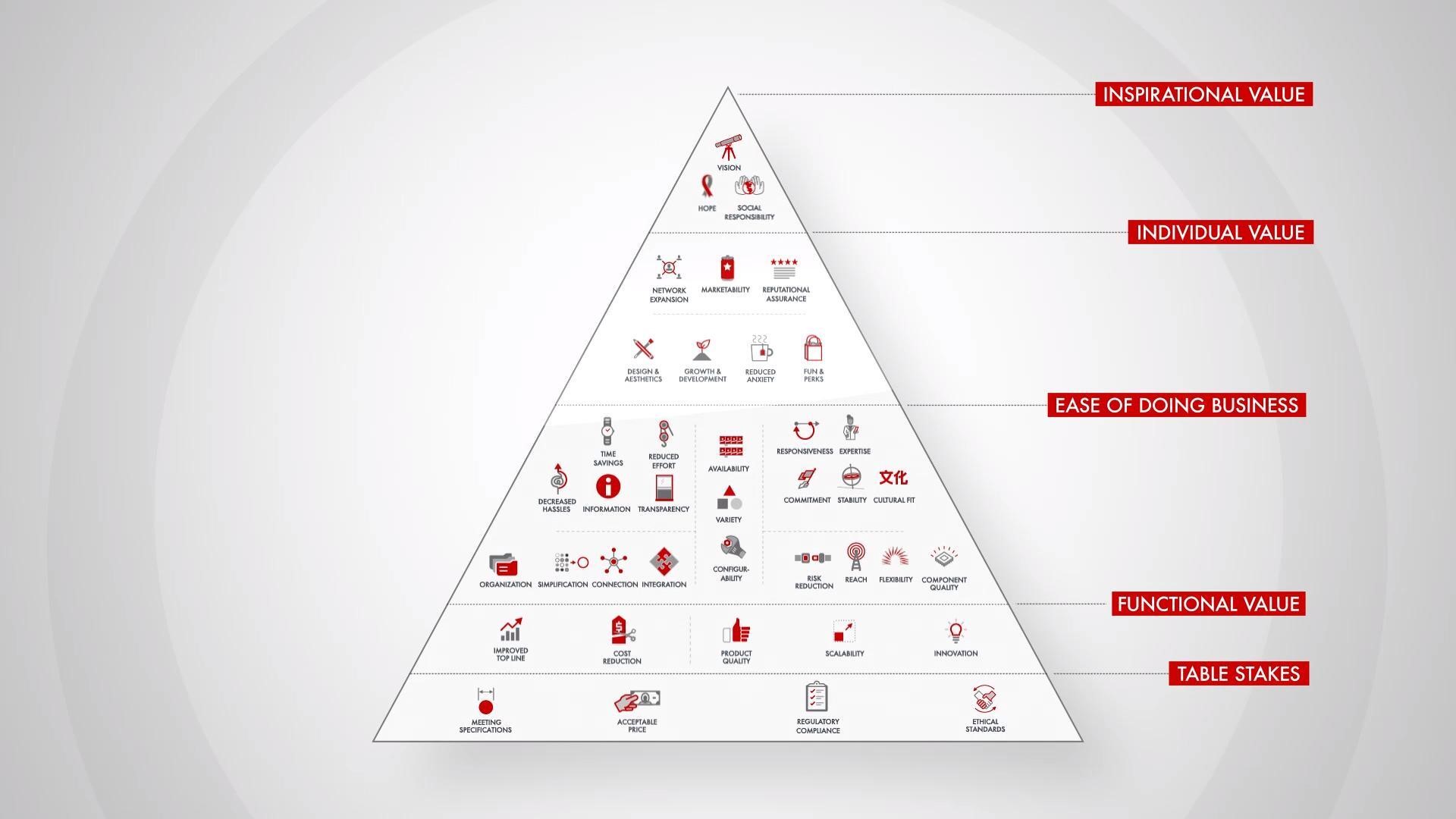
Bain’s Elements of Value Framework
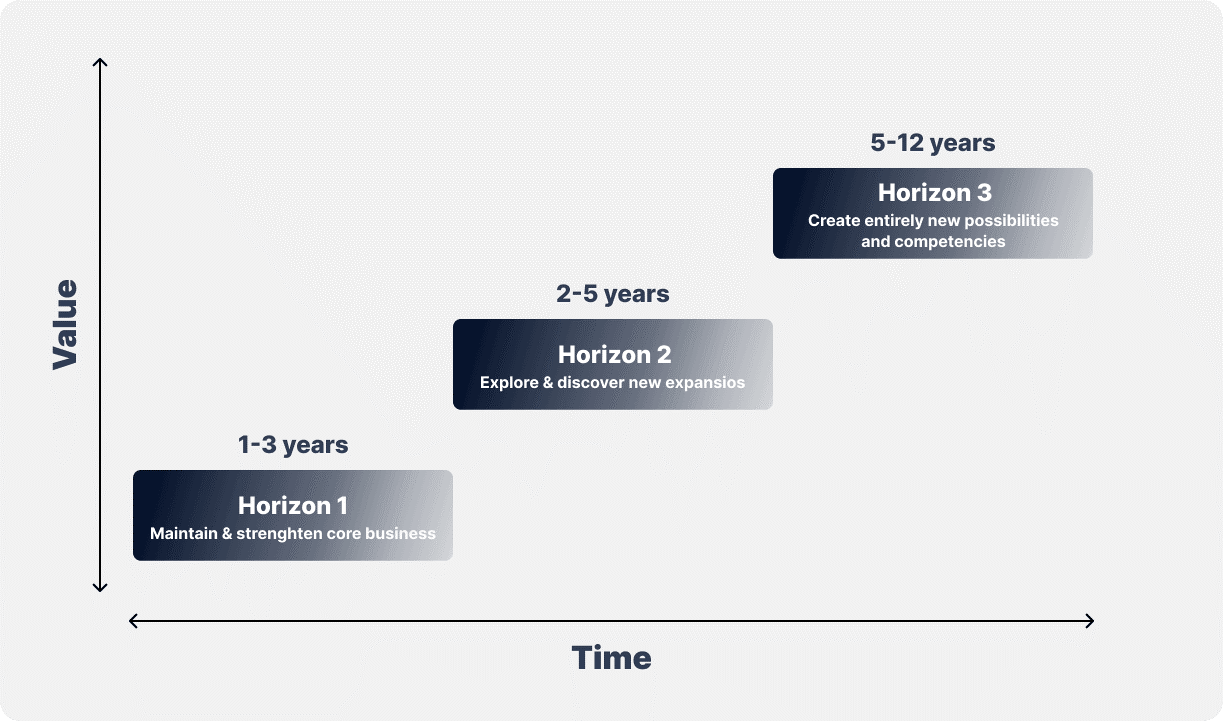
McKinsey Growth Pyramid
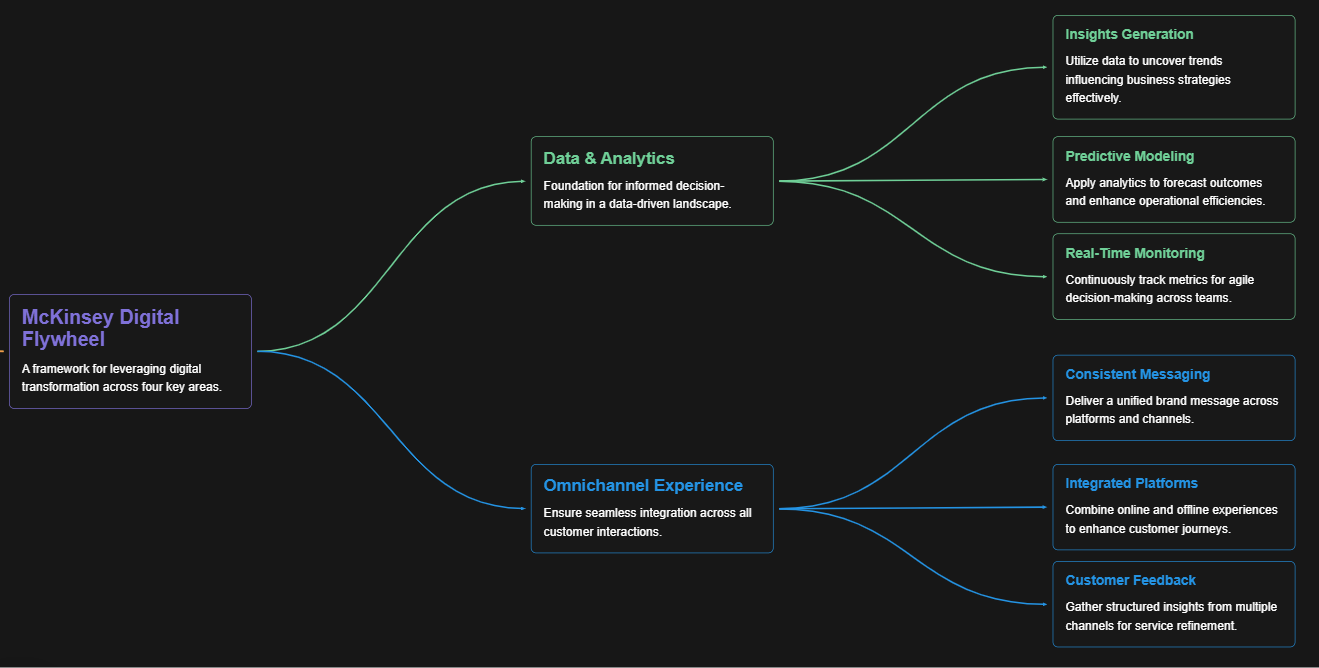
McKinsey Digital Flywheel
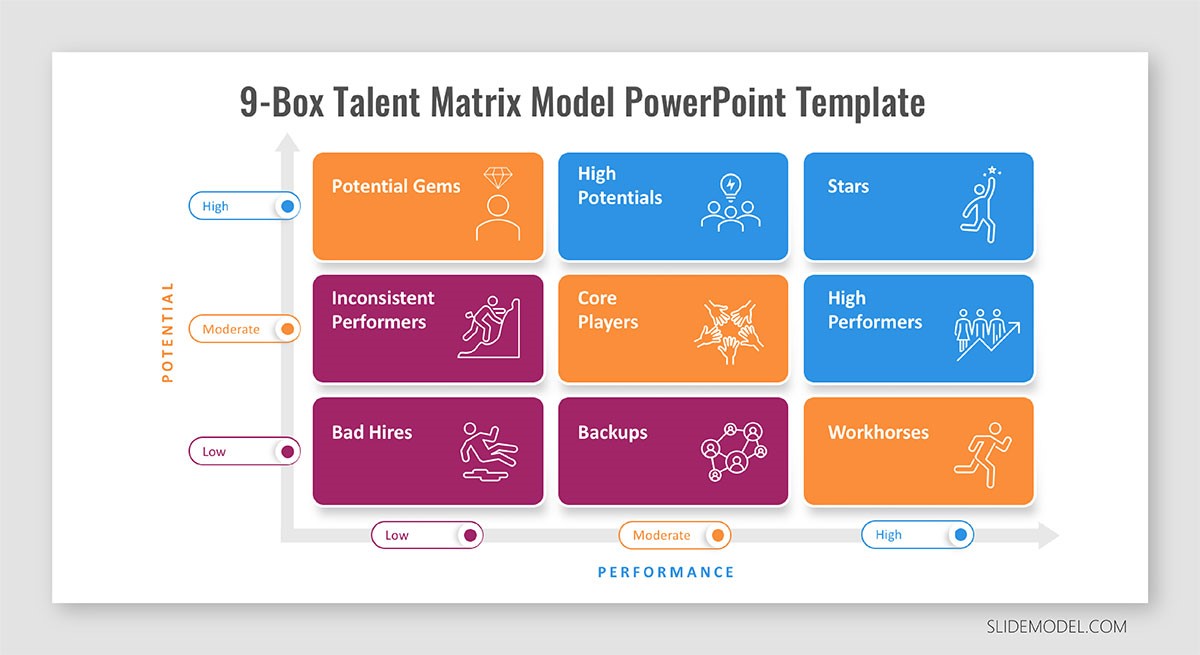
McKinsey 9-Box Talent Matrix
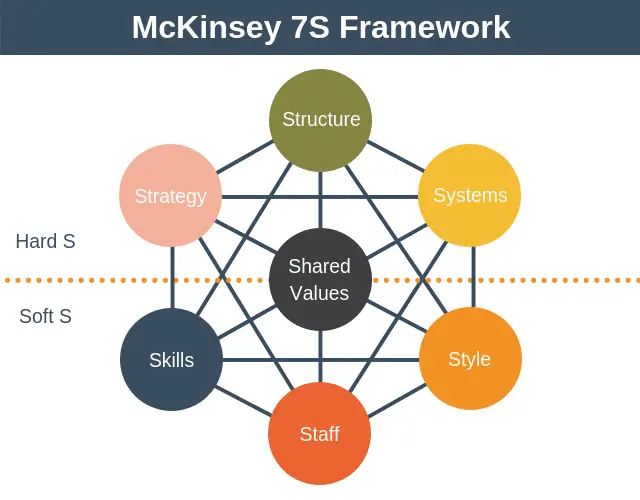
McKinsey 7S Framework
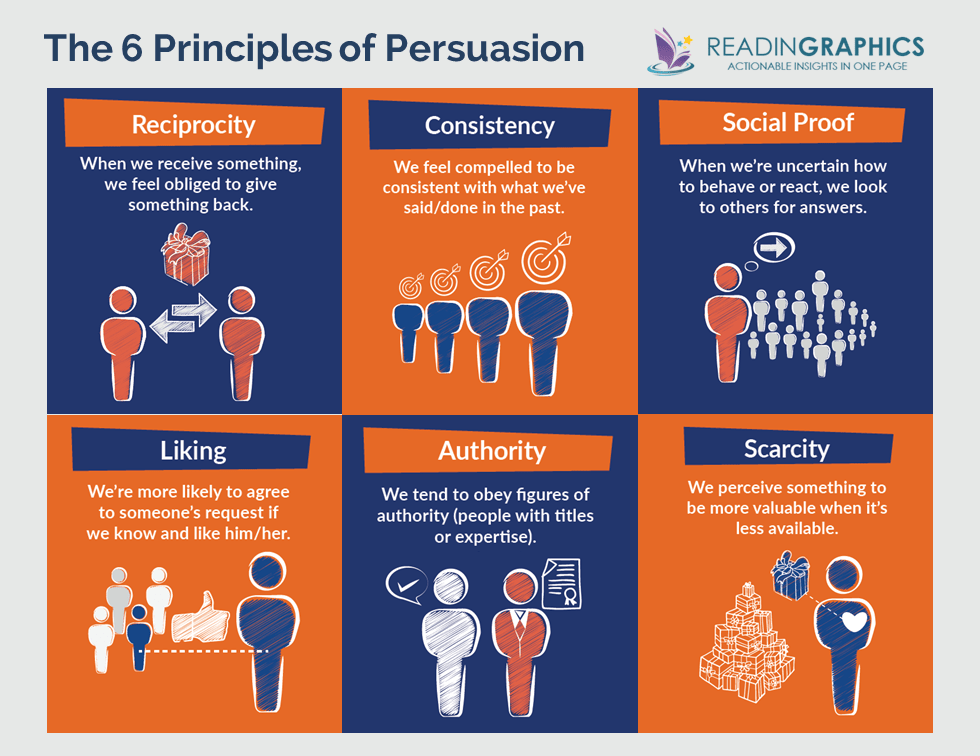
The Psychology of Persuasion in Marketing
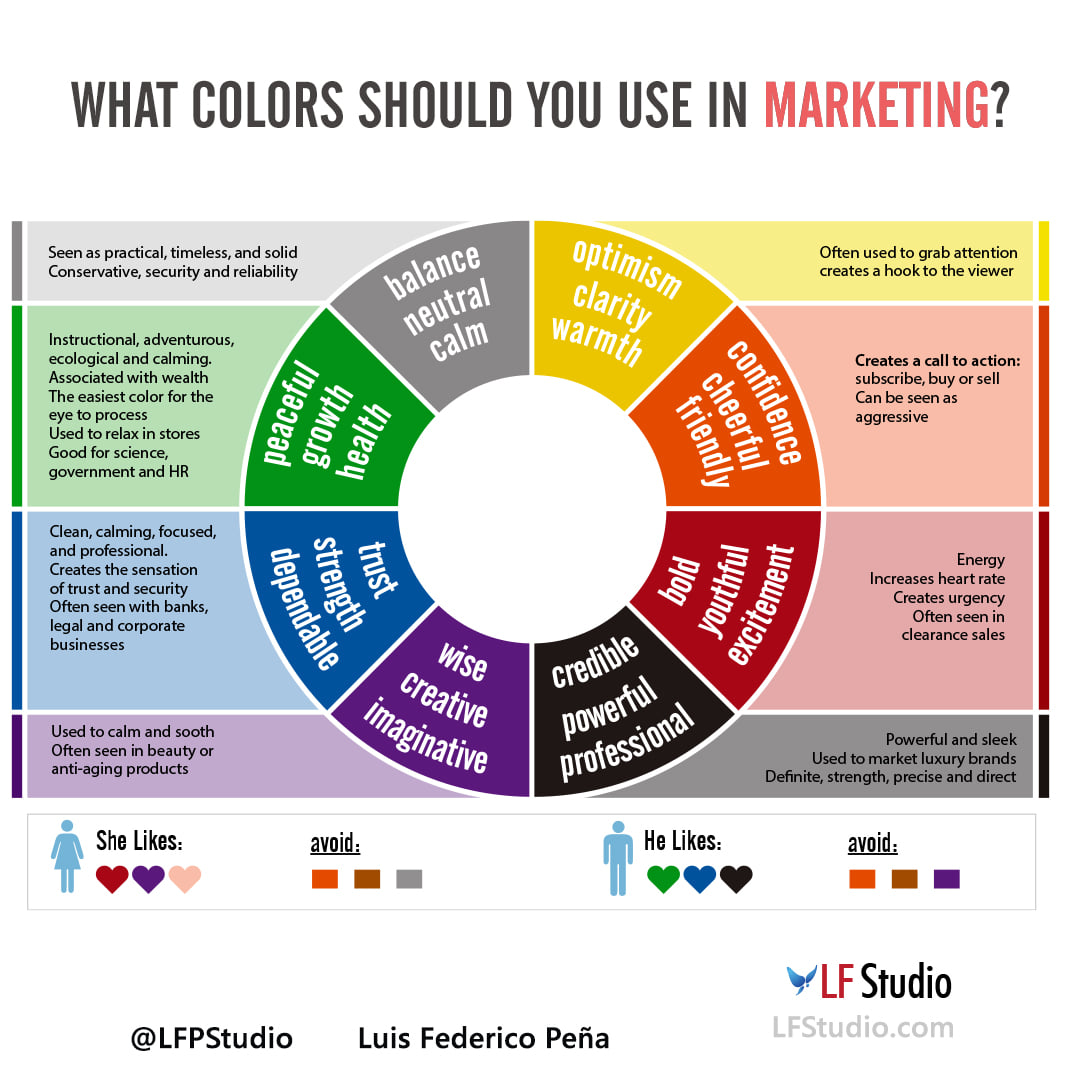
The Influence of Colors on Branding and Marketing Psychology
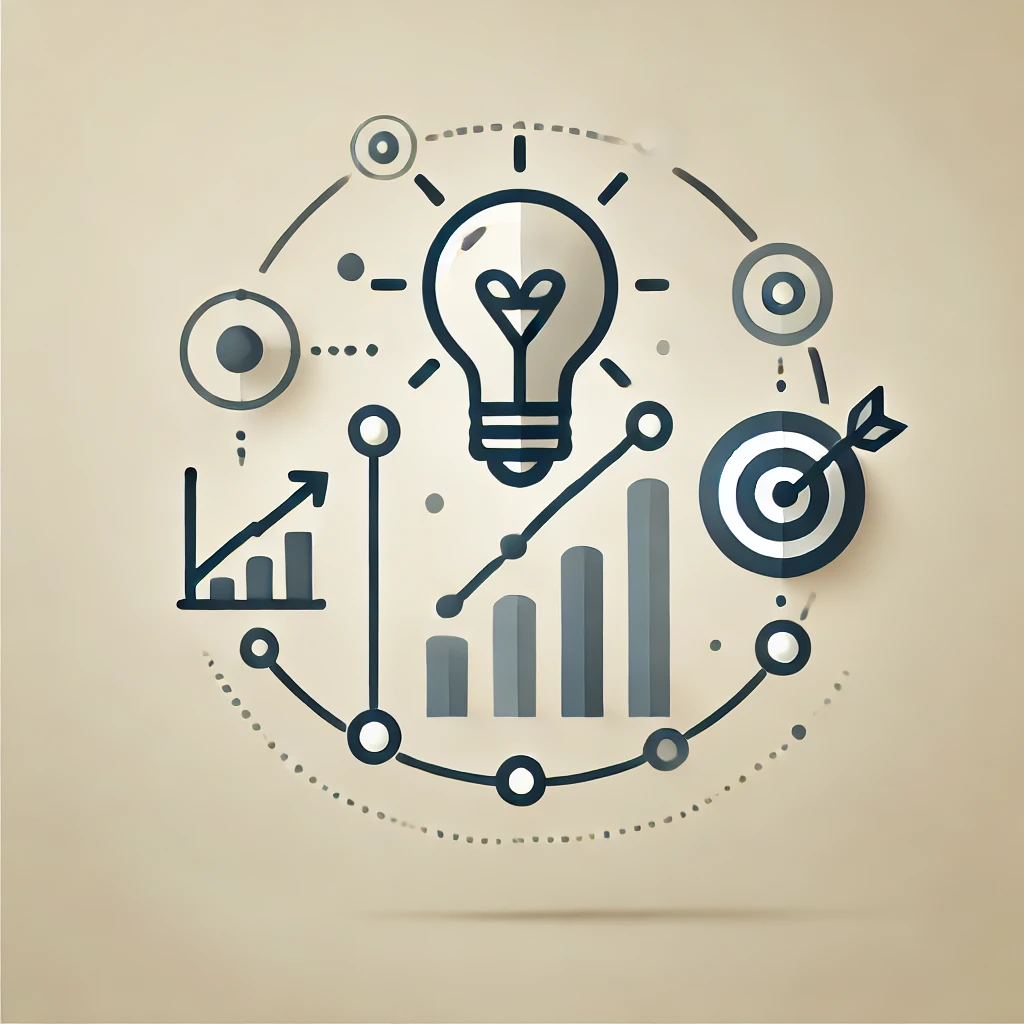