Budgeting for AI Tools in Marketing
Paul recently found himself engaged in a revealing conversation with the CMO of a Fortune 500 retail company during an industry conference. While standing next to a lavish AI vendor booth that was showcasing the latest marketing solutions, she leaned in to confess, "We've spent over two million on AI tools in the past year, but I can't confidently tell my CEO what return we've generated." Her team had eagerly adopted a variety of AI solutions, including generative content platforms, predictive analytics engines, and customer journey optimization tools, but they did so without a cohesive strategy or measurement framework. "We're drowning in capabilities but struggling to translate them into business outcomes," she admitted. This interaction perfectly encapsulated the challenge many marketing leaders face: effectively budgeting for AI when the technology is crucial for competitiveness yet difficult to evaluate using traditional ROI frameworks.
Introduction: The AI Revolution in Marketing Resource Allocation
Artificial intelligence has fundamentally transformed marketing budget allocation models across industries. Research from Gartner indicates that AI-related investments now comprise 17-23% of total marketing technology budgets, with this percentage projected to reach 30% by 2026. This rapid growth reflects the transition of AI from experimental innovation to core operational capability.
The financial dynamics of AI investment differ significantly from traditional marketing technology. While conventional marketing platforms typically deliver predictable value based on established use cases, AI technologies often follow exponential value curves—starting with modest returns during learning phases before delivering substantial impact as systems mature and data accumulates. This pattern necessitates new approaches to budget justification and performance measurement.
1. Generative Content Costs
Content production represents one of the most visible applications of AI in marketing, with generative tools now capable of creating everything from ad copy to images to video content. The economics of these tools create new budgeting challenges as organizations balance cost reduction opportunities against quality and brand consistency requirements.
Organizations have responded by developing tiered content frameworks that distinguish between fully AI-generated content (typically for high-volume, operational communications), AI-assisted content (where human creators leverage AI tools), and premium human-created content (for flagship brand moments). Budget allocations across these tiers vary significantly by industry, with B2B organizations typically allocating 40-50% to AI-assisted content while consumer brands maintain higher investments in human-led creative.
The most sophisticated organizations have moved beyond viewing AI content tools as simple cost reduction mechanisms and instead developed value models that incorporate both efficiency gains and effectiveness improvements. These models account for not just the direct cost savings from automated content production but also the value of increased personalization, testing capacity, and speed-to-market that AI enables.
2. Predictive Analytics Subscriptions
Predictive capabilities represent the most mature application of AI in marketing, with tools now capable of forecasting everything from campaign performance to customer lifetime value to emerging market trends. The subscription-based business models of these platforms create distinct budgeting considerations compared to traditional marketing technologies.
Organizations have evolved from departmental subscriptions to enterprise-wide approaches that integrate predictive capabilities across marketing functions. This consolidation typically reduces total expenditure by 15-20% while increasing utility through unified data models and consistent analytical approaches. Budget allocations for these platforms have shifted from marketing technology to data infrastructure categories, reflecting their foundational importance.
The value case for predictive analytics has matured from efficiency-focused arguments to performance-based justifications. Research indicates that organizations with mature predictive capabilities demonstrate 23% higher marketing ROI and 31% lower customer acquisition costs compared to industry peers. These performance differentials provide clear financial justification for sustained investment even in challenging budget environments.
3. Efficiency vs. Creative Value
The tension between efficiency gains and creative effectiveness represents perhaps the most nuanced aspect of AI budgeting. Organizations must balance the quantifiable cost reductions offered by automation against the less tangible but potentially more valuable benefits of human creativity and strategic thinking.
Marketing leaders have responded by developing new budget allocation frameworks that distinguish between operational and strategic applications of AI. Operational applications focus on automating routine tasks and typically deliver clearly defined efficiency improvements. Strategic applications augment human capabilities and generate value through enhanced decision quality and creative innovation rather than simple cost reduction.
The most sophisticated organizations have implemented value-based budgeting approaches that incorporate both efficiency metrics and effectiveness indicators. These frameworks recognize that while AI can reduce the cost of creating and deploying marketing assets by 30-60%, the true value often stems from the ability to increase personalization, experiment more rapidly, and reallocate human resources to higher-value strategic activities.
Call to Action
Marketing leaders must transform how they approach AI budgeting to realize the full potential of these technologies while avoiding wasteful investments. Begin by conducting a comprehensive AI capability audit that distinguishes between tools that primarily deliver efficiency gains and those that enhance strategic capabilities. This distinction is critical for developing appropriate value expectations and measurement frameworks.
Implement portfolio management approaches to AI investments rather than evaluating tools in isolation. Group related capabilities into strategic initiatives with clear business objectives and established success metrics. This approach prevents the fragmentation of AI investments into disconnected tools without cohesive value narratives.
Finally, develop new collaboration models between marketing, IT, and finance teams to govern AI investments effectively. Establish cross-functional committees that review AI expenditures quarterly against both technical and business metrics. These governance structures should focus not just on cost management but on value realization, ensuring that efficiency gains are translated into either bottom-line improvements or reinvestment in growth initiatives.
The organizations that thrive in the AI-enabled marketing landscape will be those that move beyond viewing artificial intelligence as either a cost-cutting mechanism or an innovation experiment. By developing sophisticated budgeting approaches that balance efficiency with effectiveness and short-term returns with long-term capability building, marketing leaders can transform AI from a financial burden into a sustainable competitive advantage.
Featured Blogs
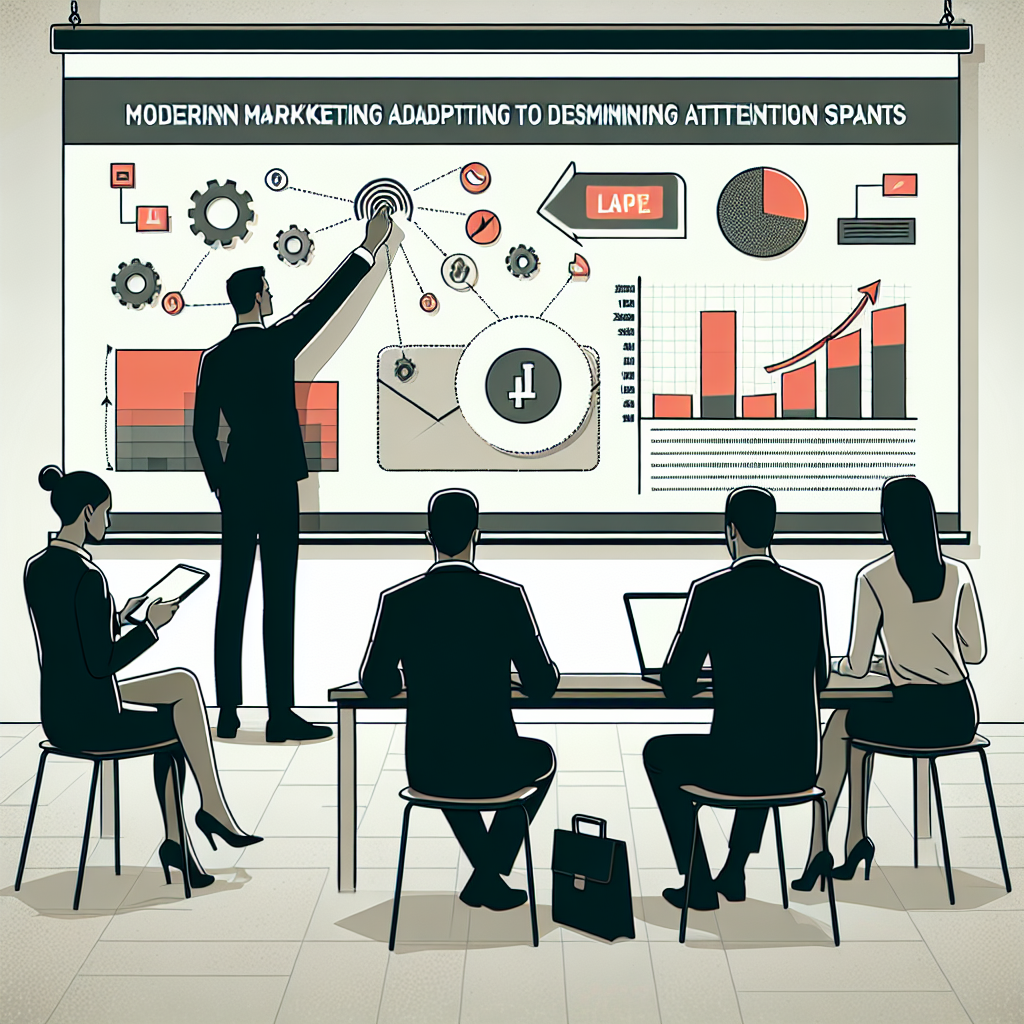
How the Attention Recession Is Changing Marketing
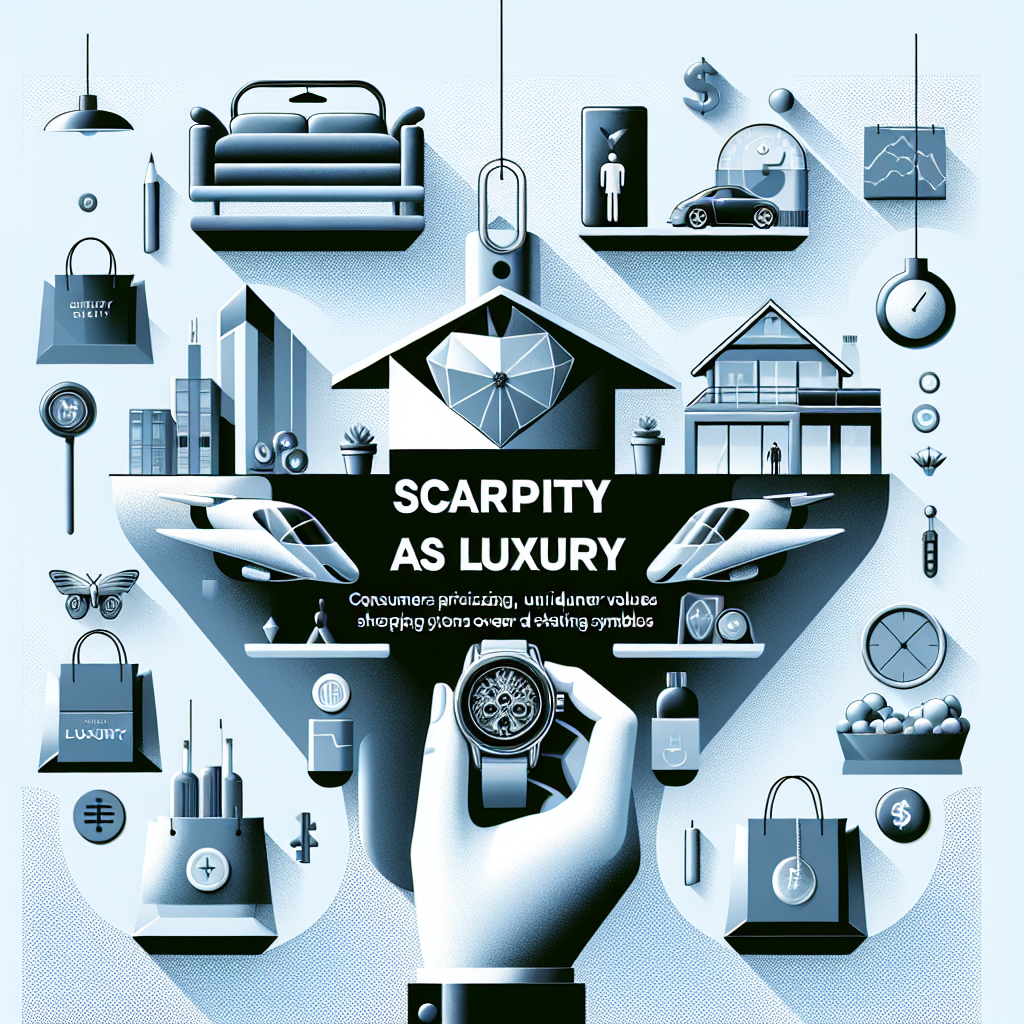
The New Luxury Why Consumers Now Value Scarcity Over Status
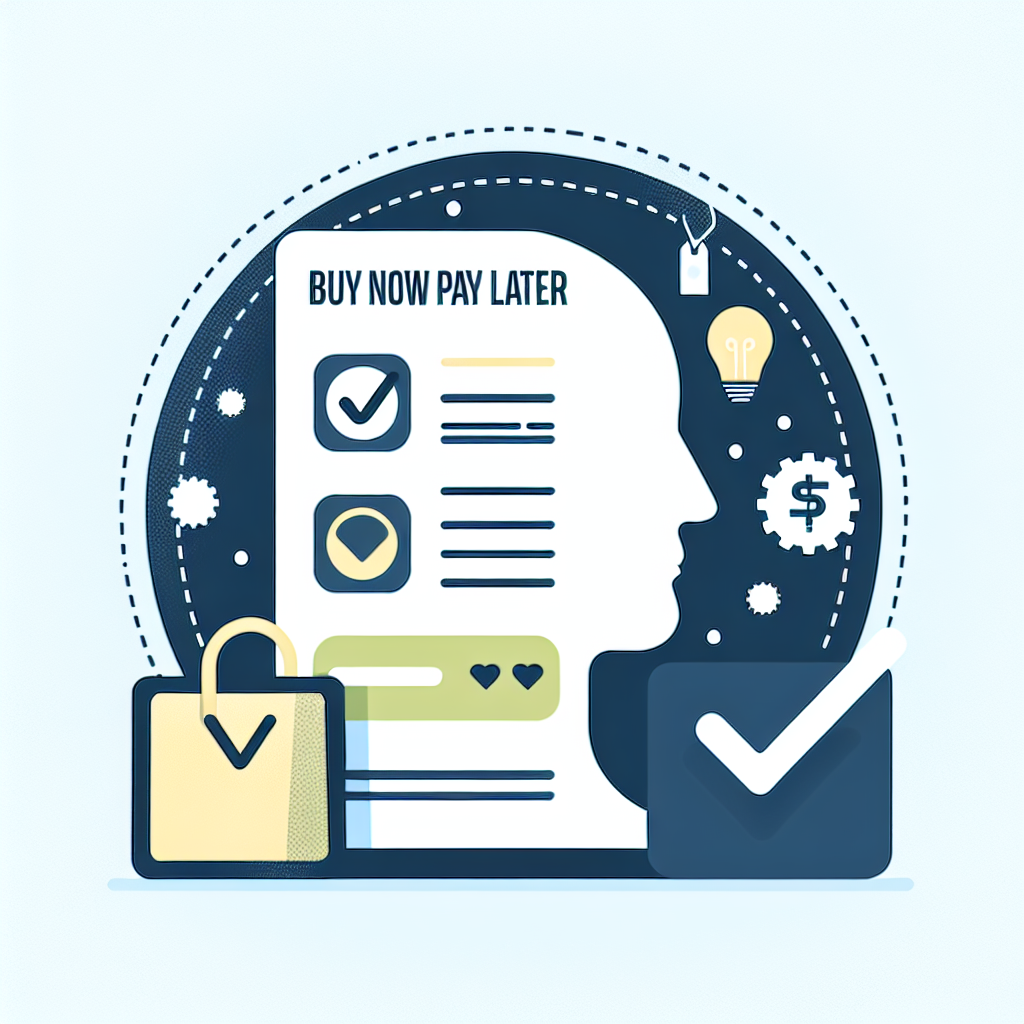
The Psychology Behind Buy Now Pay later
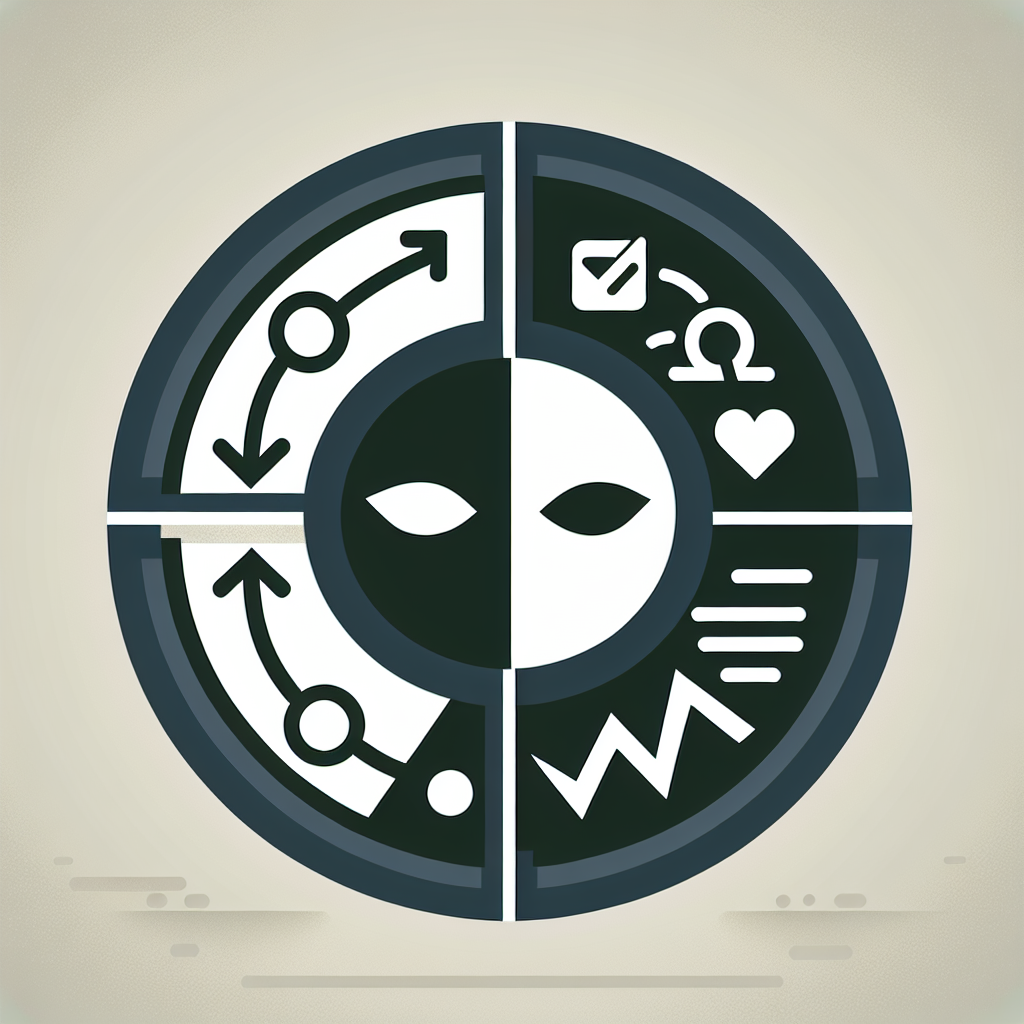
The Role of Dark Patterns in Digital Marketing and Ethical Concerns
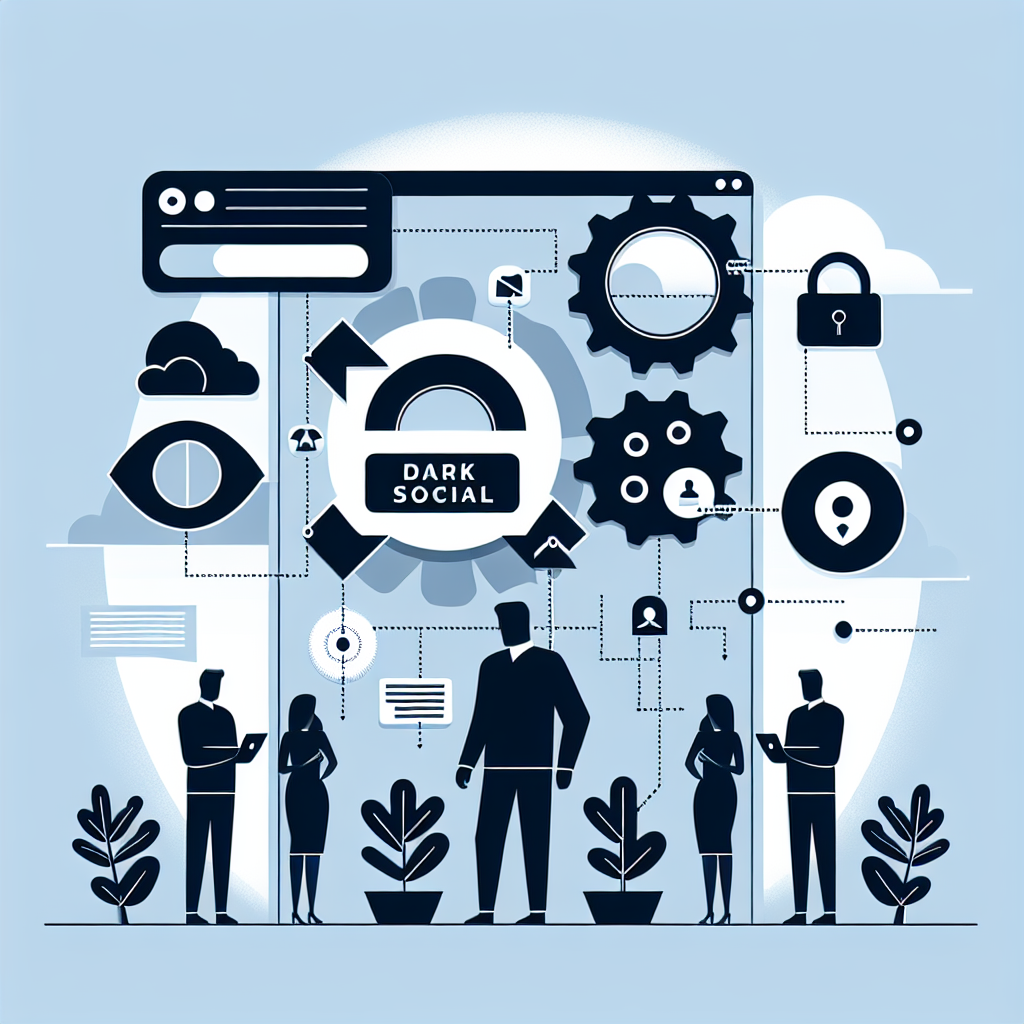
The Rise of Dark Social and Its Impact on Marketing Measurement
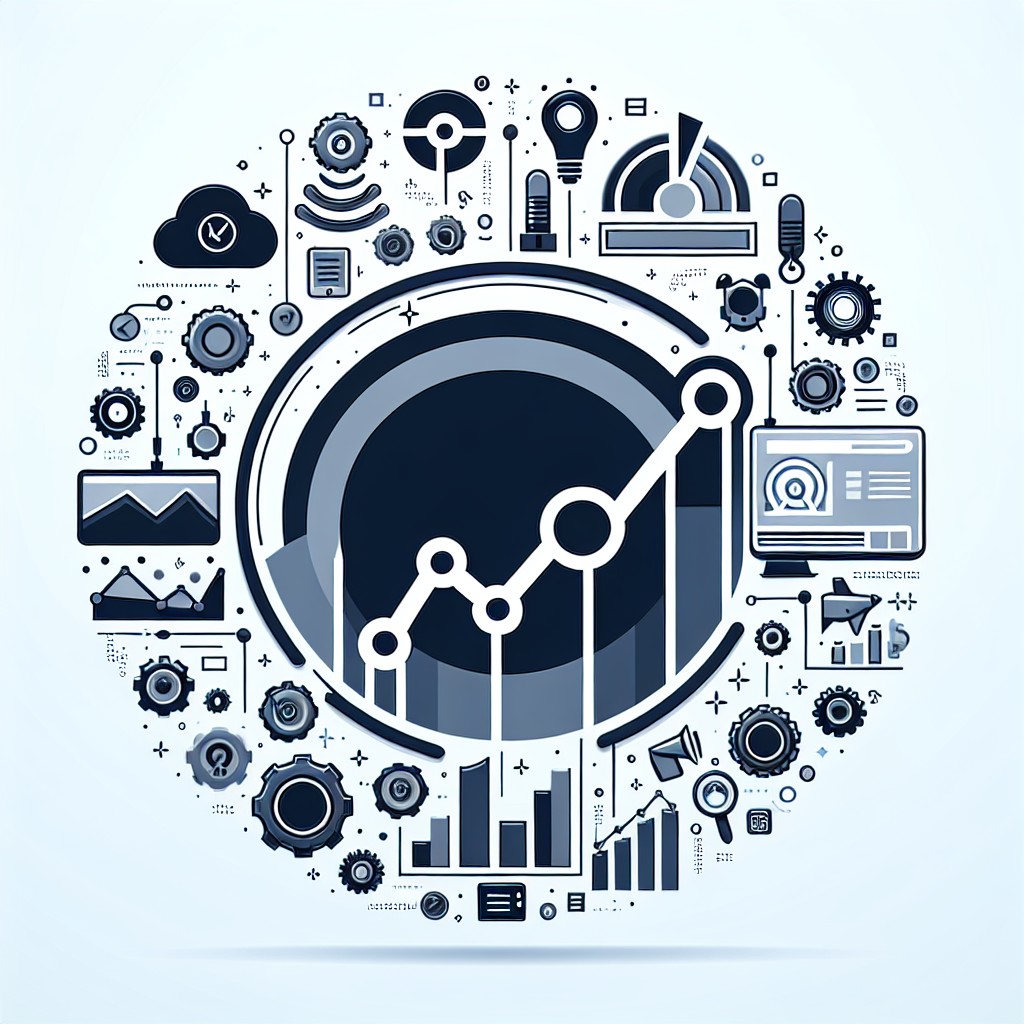