Personalization Engines for Loyalty
Last month, Ram received an email from a streaming service he subscribes to that stopped him in his tracks. "We noticed you haven't finished watching 'The Architecture of Decision,' but we thought you might enjoy these similar documentaries based on your viewing patterns." What struck him wasn't just the accuracy of the recommendation, but how it arrived precisely when his weekend schedule had opened up unexpectedly. As he browsed the suggested titles, each one resonated with his specific interests in behavioral economics and design thinking—topics he'd never explicitly told the platform he cared about. This moment crystallized for Ram how sophisticated personalization has become and how dramatically it's reshaping customer loyalty dynamics across industries.
Introduction: The Personalization Imperative
The evolution of customer loyalty has entered a new phase where mass personalization has given way to what industry analysts call "individualized engagement"—unique experiences tailored to specific customer contexts, behaviors, and preferences. Research from Epsilon indicates that 80% of consumers are more likely to purchase from brands offering personalized experiences, while Accenture reports that 91% of consumers are more likely to shop with brands providing relevant recommendations and offers.
This shift represents more than incremental improvement—it's a fundamental reimagining of how loyalty is earned and maintained. According to McKinsey research, companies that excel at personalization generate 40% more revenue from those activities than average performers. This article examines how leading organizations build comprehensive personalization capabilities that drive sustained loyalty and competitive advantage.
Building Personalization Roadmaps
Data Foundation Assessment
Effective personalization begins with systematic data infrastructure evaluation. Netflix's personalization journey started not with algorithms but with a comprehensive data architecture overhaul, consolidating customer data from 13 disparate systems to create unified customer profiles. Organizations must conduct similar data foundation assessments, identifying gaps in both collection and integration capabilities.
Journey Mapping for Personalization Opportunities
Strategic personalization requires identifying high-impact customization points across the customer journey. Sephora's personalization roadmap prioritizes intervention points through a "moment mapping" methodology, systematically rating interaction points on both emotional significance and personalization feasibility to identify optimal starting points.
Capability Maturity Models
Leading organizations develop staged implementation plans based on capability maturity. Spotify employs a five-stage personalization maturity model from basic segmentation through predictive and eventually generative personalization, creating clear capability milestones rather than attempting everything simultaneously.
Cross-Functional Governance
Successful personalization requires new operational models. Starbucks established a dedicated "personalization council" with rotating membership from marketing, IT, operations, and privacy/legal teams, creating shared ownership and systematic stakeholder alignment for personalization initiatives.
Ethical Frameworks
Forward-thinking organizations establish formal ethical boundaries for personalization efforts. Microsoft's personalization guidelines include specific thresholds for "helpful" versus "creepy" personalization, with formal review processes for initiatives that might cross perceived privacy boundaries.
AI and Machine Learning
Predictive Modeling Evolution
The sophistication of personalization algorithms has advanced dramatically. Amazon's recommendation engines have evolved from simple collaborative filtering to complex deep learning systems that improve outcomes by 35% through multi-dimensional behavior modeling rather than one-dimensional purchase history.
Real-Time Decision Engines
Advanced personalization systems now operate in true real-time. Bank of America's personalization platform evaluates over 500 variables within 300 milliseconds to deliver customized mobile app experiences, adjusting interface elements, content, and offers based on immediate context.
Natural Language Processing for Preference Discovery
Leading systems now incorporate unstructured data analysis. Airbnb's preference engine analyzes review text, search queries, and customer service interactions using natural language processing to identify unarticulated preferences that explicit surveys miss, increasing recommendation relevance by 24%.
Computer Vision Applications
Visual analysis creates new personalization vectors. Wayfair's "visual search" capability allows customers to upload room photos, with AI analyzing existing décor styles to make contextually relevant recommendations, increasing conversion rates by 37% for users engaging with the feature.
Reinforcement Learning Systems
The most advanced personalization engines employ reinforcement learning approaches. YouTube's recommendation algorithm uses multi-armed bandit testing to continuously optimize for engagement while systematically exploring recommendation diversity, balancing exploitation of known preferences with exploration of potential new interests.
Behavioral Targeting
Micro-Moment Identification
Sophisticated personalization systems identify specific behavioral triggers. Uber Eats analyzes over 30 contextual variables including time, weather, and prior order patterns to identify precise moments when food delivery recommendations will be most relevant, increasing conversion by 21% through behavioral timing alone.
Emotional State Detection
Advanced systems now incorporate sentiment analysis. Spotify's "mood detection" algorithms analyze not just what users listen to but playback patterns like skips and repeats to infer emotional states, adjusting recommendations to align with detected moods rather than just genre preferences.
Implicit Preference Modeling
Beyond explicit preferences, leading systems infer unspoken needs. LinkedIn's personalization engine analyzes subtle signals like dwell time on profiles and partial search queries to build "shadow profiles" of career interests members haven't explicitly articulated, increasing job recommendation relevance by 42%.
Habit Formation Design
Strategic personalization aims to develop habitual engagement. Pinterest's personalization strategy specifically targets the "21-day habit formation window" for new users, with progressively personalized content designed to establish usage patterns that convert to long-term engagement, resulting in 29% higher retention rates.
Cross-Device Behavioral Integration
Sophisticated systems connect behaviors across touchpoints. Disney's personalization platform integrates mobile app usage, park movement patterns, and entertainment choices to create unified guest profiles, delivering recommendations that acknowledge complete customer contexts rather than channel-specific behaviors.
Actionable Takeaways
Conduct a Personalization Capability Assessment
Evaluate your organization against a formal personalization maturity model. Assess data infrastructure, algorithm sophistication, cross-channel integration capabilities, and organizational readiness to identify specific capability gaps requiring investment.
Develop an MVT (Minimum Viable Test) Approach
Create rapid testing protocols for personalization initiatives. Marriott's personalization team employs two-week testing cycles with clear success metrics, allowing rapid iteration rather than prolonged deployment cycles.
Implement Value-Based Personalization
Move beyond generic personalization to value-aligned customization. REI's personalization engine adjusts communications and offers based not just on purchase history but detected value alignment with sustainability, adventure, or community priorities, increasing response rates by 31%.
Create Personalization Measurement Standards
Develop comprehensive metrics beyond conversion. The North Face established a balanced scorecard approach measuring immediate conversion lift, secondary engagement behaviors, and long-term loyalty indicators to assess personalization effectiveness holistically.
Establish Algorithmic Transparency Principles
Develop clear guidelines for algorithm explainability. Mastercard's personalization platform includes "logic explanation modules" that allow both business users and customers to understand recommendation rationales, building trust through transparency.
Call to Action
The personalization capabilities gap between leaders and laggards continues to widen, creating significant competitive vulnerability for organizations that delay comprehensive implementation. Begin by conducting a personalization readiness assessment examining your organization's data infrastructure, analytical capabilities, and organizational alignment.
Develop a staged personalization roadmap with clear capability milestones, starting with high-impact, lower-complexity opportunities. Establish a formal personalization governance structure with representatives from technology, marketing, operations, and legal/privacy teams to ensure coordinated implementation.
Implement a balanced measurement framework that evaluates personalization impact on both immediate conversion metrics and longer-term loyalty indicators. Regularly review personalization performance against industry benchmarks to identify emerging capability gaps.
Most importantly, recognize that effective personalization requires ongoing evolution rather than one-time implementation. The organizations achieving sustainable loyalty advantages are those building systematic personalization capabilities that continuously adapt to changing customer expectations and technological possibilities.
Featured Blogs
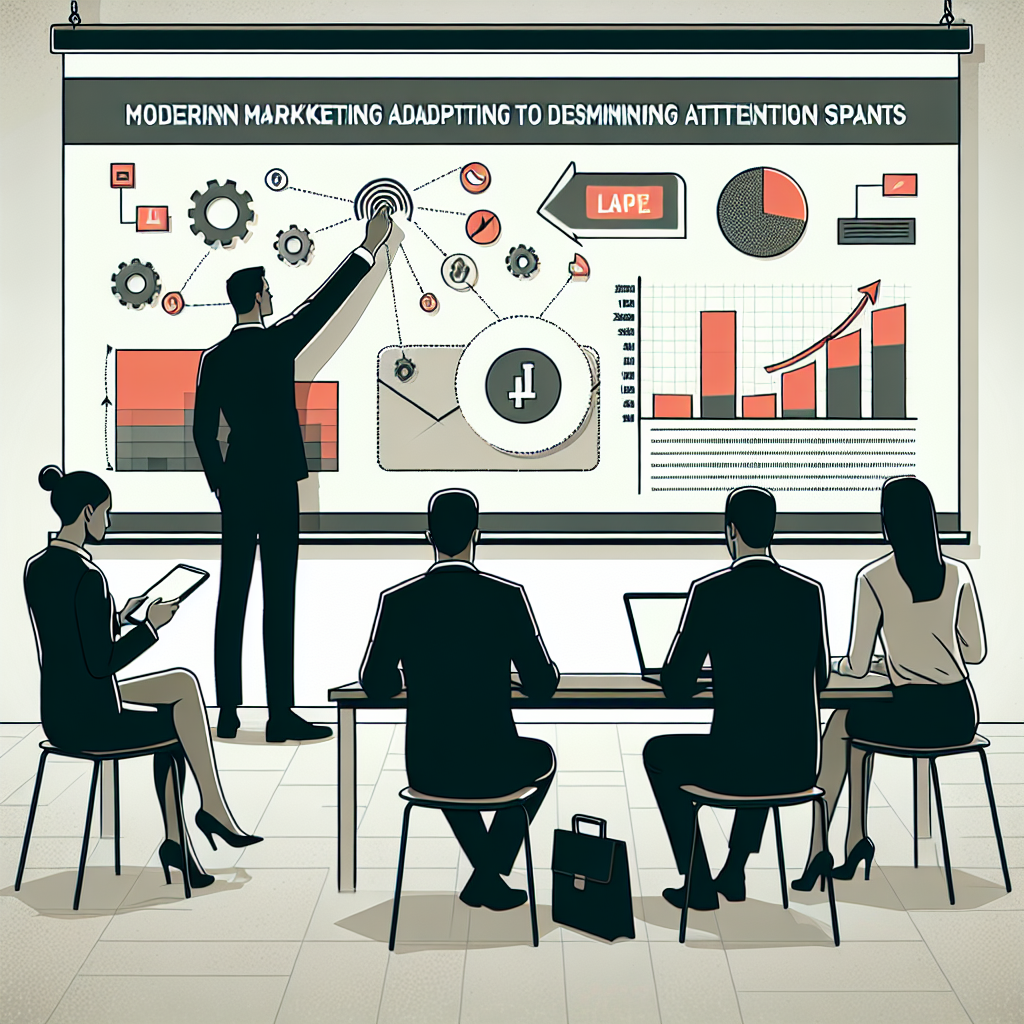
How the Attention Recession Is Changing Marketing
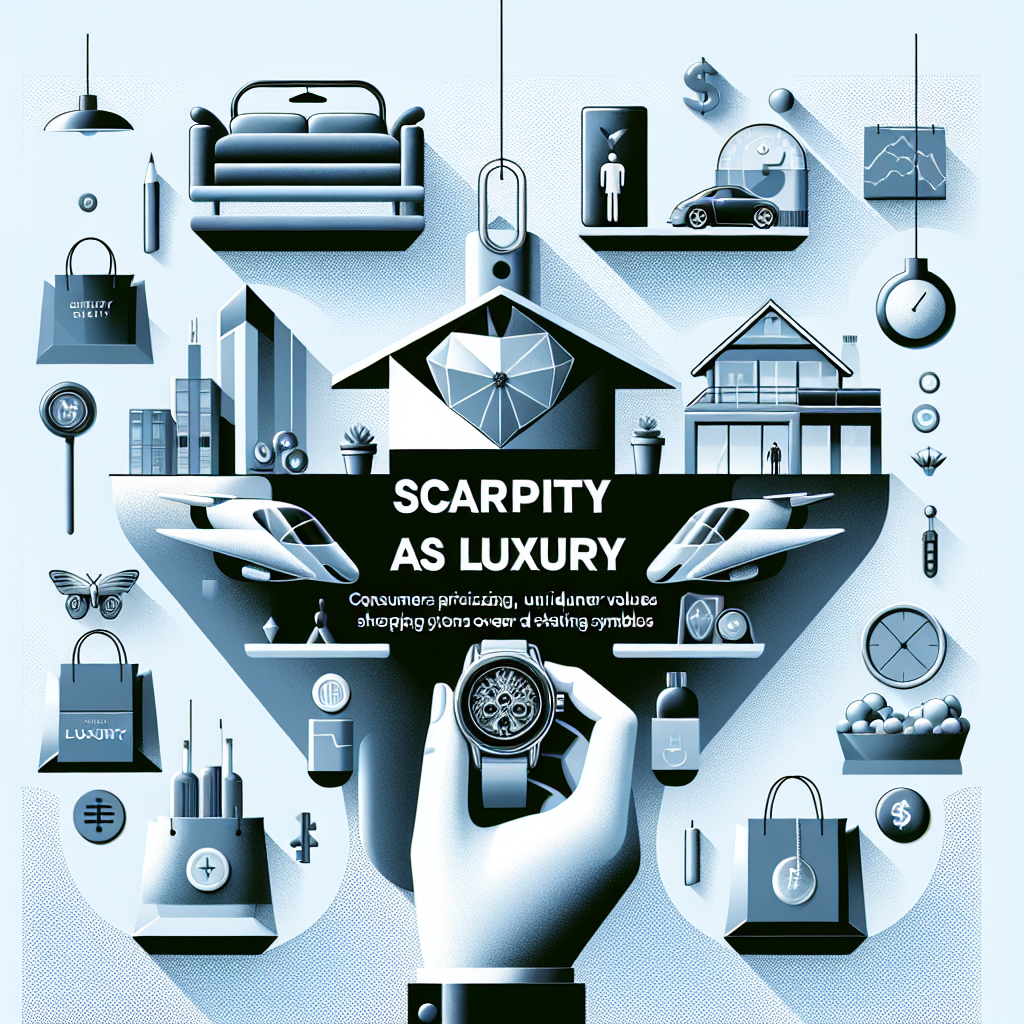
The New Luxury Why Consumers Now Value Scarcity Over Status
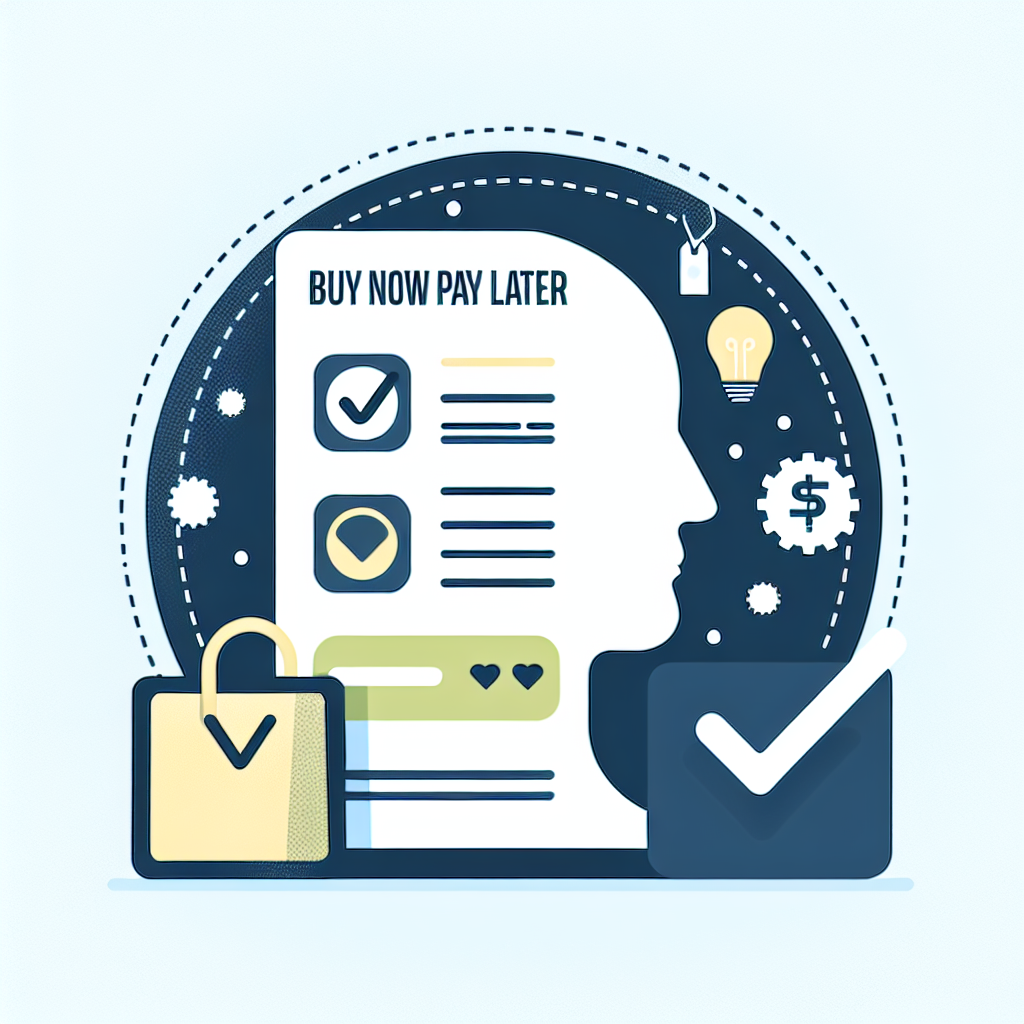
The Psychology Behind Buy Now Pay later
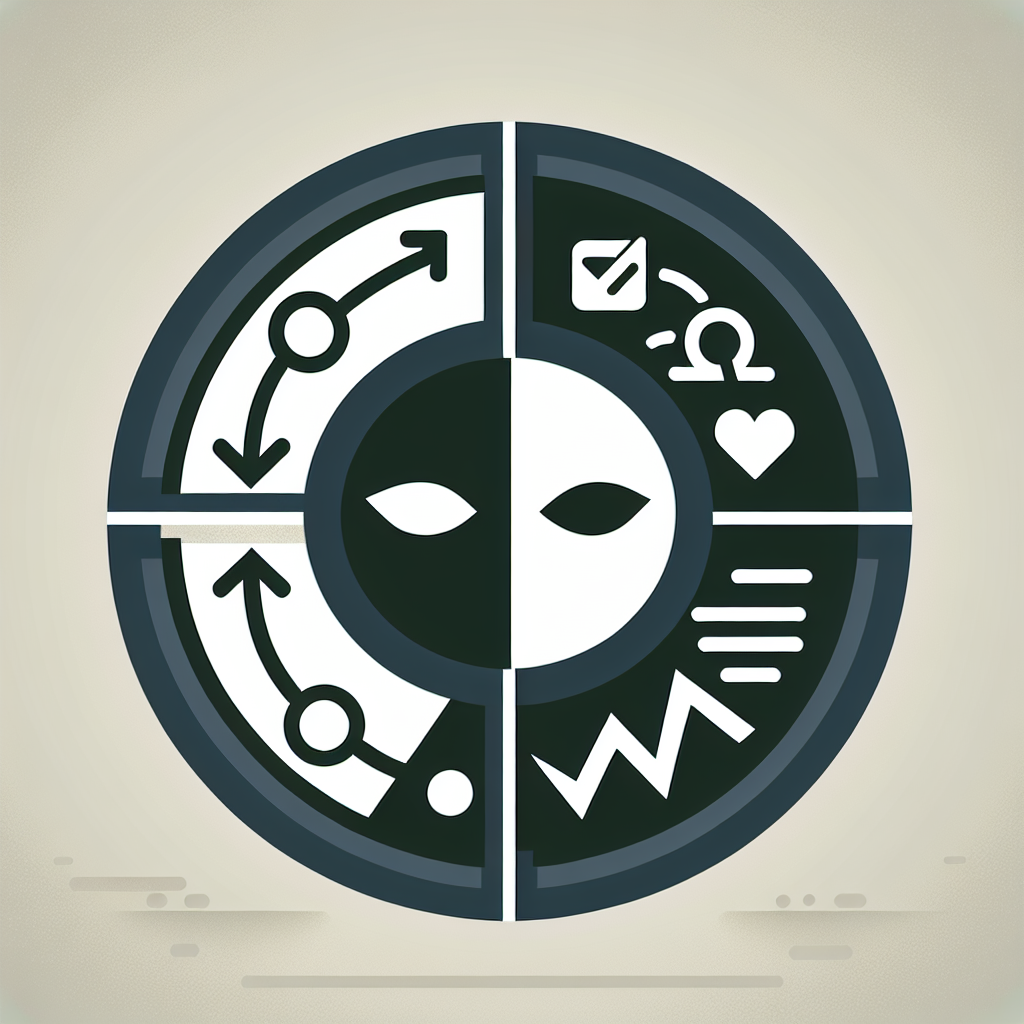
The Role of Dark Patterns in Digital Marketing and Ethical Concerns
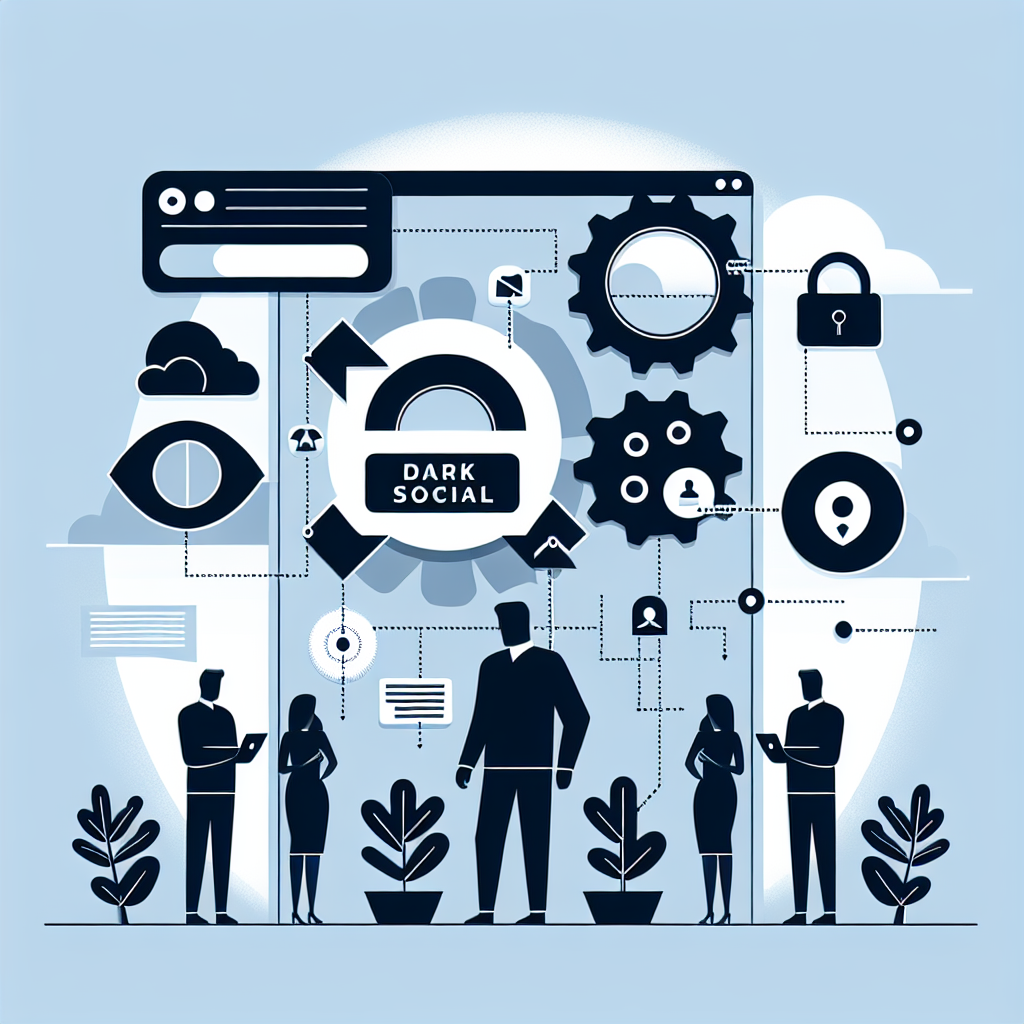
The Rise of Dark Social and Its Impact on Marketing Measurement
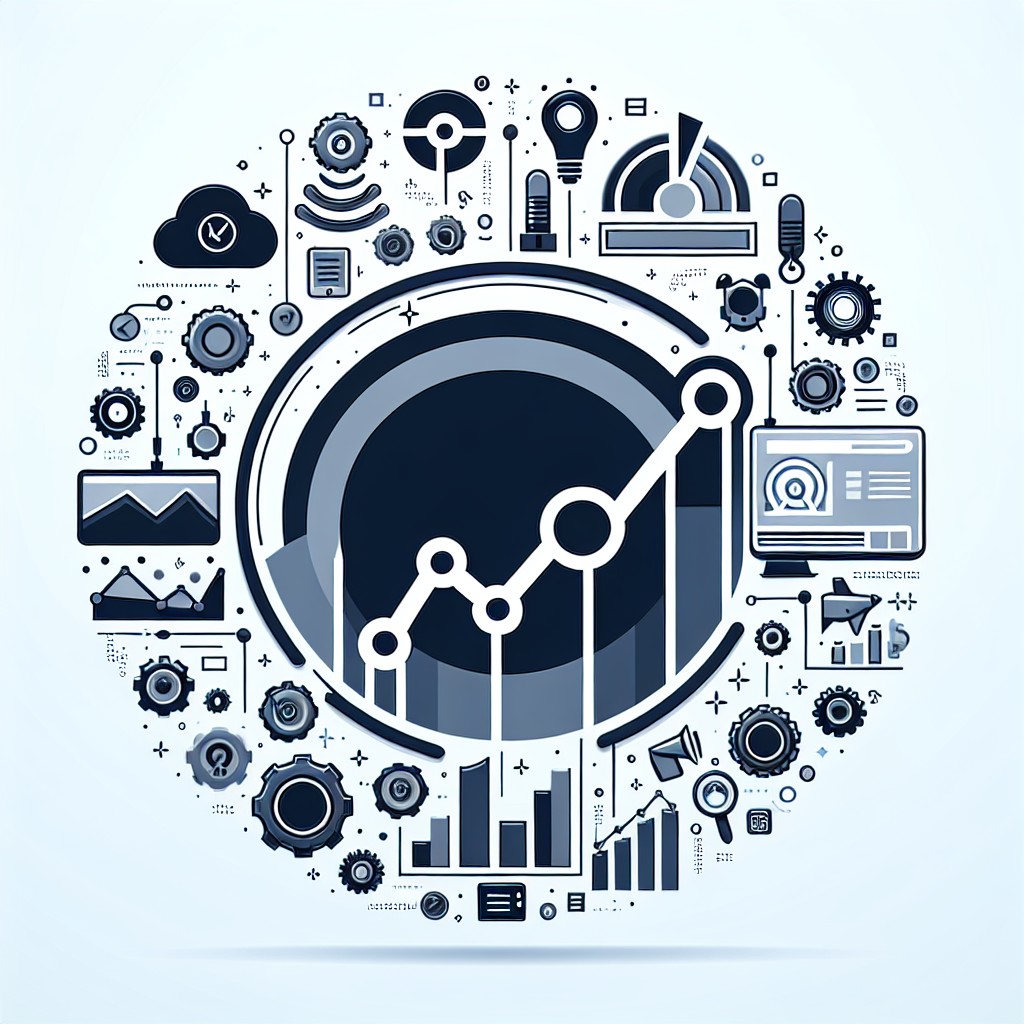