A/B Testing Done Right
The notification appeared on my screen during a weekly marketing review: "Congratulations! Your homepage test reached statistical significance." Excited, I immediately prepared to implement the winning variant—after all, the data showed a 12% increase in click-through rates. But something didn't feel right. Digging deeper, I discovered our test had run for only three days during a holiday weekend with barely 500 visitors per variant. Despite the tool's confidence declaration, we were about to make a permanent change based on inadequate data. This near-miss transformed my approach to A/B testing, leading me to realize that significance notifications and flashy dashboards often mask fundamental testing flaws. My journey to understand proper testing methodology began that day, showing me that A/B testing done right is both art and science—requiring rigorous hypothesis formation, appropriate statistical power, and thoughtful interpretation.
Introduction: Beyond Random Testing
A/B testing has evolved from a novel marketing tactic to an essential practice for data-driven organizations. Yet despite widespread adoption, research from ConversionXL Institute reveals that over 65% of A/B tests are conducted without proper hypothesis documentation, while Optimizely's industry analysis found that 57% of tests are stopped prematurely based on misleading early results.
True A/B testing excellence goes beyond simply dividing traffic and measuring differences—it requires methodical hypothesis design, proper statistical power through adequate sample sizes, and interpreting results in ways that drive meaningful action. Without these foundational elements, testing programs often generate misleading insights that waste resources and potentially harm business outcomes.
As testing pioneer Ron Kohavi noted in his landmark Microsoft research paper, "Controlled experiments provide the gold standard for establishing causality and driving product decisions." However, this gold standard is only achieved when testing is approached with proper scientific rigor and business context.
1. Hypothesis Design
The foundation of effective A/B testing lies in developing clear, testable hypotheses rooted in customer understanding and business objectives.
a) Problem-Based Hypothesis Formation
Successful testing organizations structure hypotheses to address specific observed problems:
- Starting with quantitative data identifying conversion barriers
- Incorporating qualitative insights from customer research
- Targeting specific user behaviors rather than general outcomes
- Creating explicit connections between proposed changes and expected impacts
Example: E-commerce retailer Wayfair transformed their testing program by implementing a "Problem-Solution-Result" hypothesis framework. Each test document begins with observable customer friction points identified through analytics and user testing, followed by specific UI/UX modifications addressing those friction points, and explicit predictions about behavioral changes. This approach increased their test success rate from 14% to 31%.
b) Variable Isolation
Professional testing programs maintain stringent control over test variables:
- Testing single changes or cohesive change groups with common theoretical basis
- Documenting all elements that differ between control and variants
- Maintaining consistent external factors during test periods
- Creating logical variant progressions to isolate effects
Example: Travel platform Airbnb's marketing team uses a "Minimum Viable Test" approach where each experiment isolates the smallest possible change needed to validate a specific hypothesis. This approach enabled them to determine that changing a single word in their call-to-action from "Book" to "Reserve" increased conversion by 6.3%, allowing precise understanding of which element drove the improvement.
c) Expected Outcome Specification
Mature testing programs define success criteria before launching tests:
- Establishing primary and secondary success metrics prior to test launch
- Documenting minimum detectable effect sizes worth implementing
- Specifying test durations and sample requirements in advance
- Creating pre-analysis plans to prevent data fishing expeditions
Example: Investment platform Betterment requires all test proposals to include "Decision Criteria" documentation specifying the exact metrics that will determine success and the threshold of improvement required to implement changes. This practice eliminated post-hoc rationalization of results and increased their rate of actionable test outcomes by 28%.
2. Sample Size and Confidence
Proper statistical power ensures test results reliably reflect true user preferences rather than random variation.
a) Power Analysis
Leading testing organizations calculate required sample sizes based on rigorous statistical principles:
- Determining baseline conversion rates for key metrics
- Establishing minimum detectable effect sizes based on business impact
- Setting appropriate statistical power (typically 80%)
- Calculating required sample sizes before test launch
Example: Media company Condé Nast developed a centralized "Test Calculator" tool that requires marketers to input baseline metrics, minimum valuable improvement thresholds, and test duration parameters. The system then calculates required traffic and prevents tests from launching until sample size requirements can be met, reducing inconclusive tests by 41%.
b) Test Duration Management
Effective testing programs establish appropriate timeframes for measurement:
- Running tests through complete business cycles (typically full weeks)
- Accounting for time-based variables (day of week, time of month)
- Implementing fixed sample stopping rules
- Avoiding peeking and early stopping based on preliminary results
Example: Software company HubSpot implements "Test Duration Locks" that prevent analyzing results until pre-determined sample sizes are reached, regardless of apparent early trends. This discipline eliminated the 32% of tests previously stopped prematurely due to misleading early data, significantly improving decision quality.
c) Segmentation Planning
Sophisticated testing accounts for audience composition impact on results:
- Identifying key segments before test launch
- Ensuring adequate sample sizes for critical segments
- Creating stratified sampling approaches when necessary
- Balancing segment insights with overall population validity
Example: Financial services company Capital One uses "Segment-First Testing" where experiment designs ensure sufficient statistical power not just for overall populations but for key customer segments. This approach revealed that website navigation changes showing positive overall impact were actually harming their highest-value customer segment—an insight that would have been missed with traditional testing approaches.
3. Actionable Interpretation
Translating test results into valuable business actions requires going beyond surface-level statistical significance.
a) Business Impact Calculation
Leading organizations translate statistical results into concrete business implications:
- Converting percentage lifts into revenue and profit projections
- Calculating return on implementation investment
- Estimating long-term customer lifetime value impacts
- Comparing results against opportunity costs
Example: E-commerce platform Shopify developed an "Impact Translator" dashboard that automatically converts test lift metrics into projected annual revenue impact based on traffic forecasts and customer lifetime value models. This system helps prioritize implementation resources based on business value rather than just statistical significance.
b) Causality Analysis
Effective testing programs investigate why results occurred, not just what happened:
- Analyzing user behavior paths throughout test experiences
- Segmenting results to identify differential impacts
- Conducting follow-up qualitative research with test participants
- Testing multiple variants to isolate effective elements
Example: Transportation company Uber uses "Causal Chain Analysis" for all significant test results, examining the sequence of user behaviors that changed between control and test groups. This approach helped them understand that a successful pricing display test worked not because of the new design itself but because it increased the percentage of users who viewed additional service tiers—leading to more valuable implementation decisions.
c) Learning Integration
Mature testing organizations systematically incorporate test insights into broader knowledge:
- Maintaining cross-functional test review sessions
- Creating knowledge repositories of test results and insights
- Identifying patterns across multiple related tests
- Updating best practice documentation based on test outcomes
Example: Streaming service Netflix implemented "Test Pattern Recognition" reviews where quarterly analysis identifies themes across dozens of individual experiments. This practice revealed that tests reducing choice complexity consistently outperformed those adding options across multiple interface areas—creating a valuable design principle that has guided numerous subsequent changes.
Call to Action
For marketing leaders seeking to elevate their A/B testing practices:
- Implement standardized hypothesis templates requiring problem statements, proposed solutions, and predicted outcomes
- Develop power calculators that prevent underpowered tests from launching
- Create pre-registration systems where test parameters and analysis plans are documented before launch
- Build business impact models translating test results into revenue and profit projections
- Establish cross-functional test review sessions focused on understanding causal mechanisms
- Develop knowledge management systems capturing insights across multiple tests
The organizations gaining the greatest competitive advantage through testing are not simply those running the most experiments, but those conducting rigorous tests with well-formed hypotheses, proper statistical power, and thoughtful interpretation of results—transforming testing from a tactical activity into a strategic capability that drives continuous improvement and market leadership.
Featured Blogs
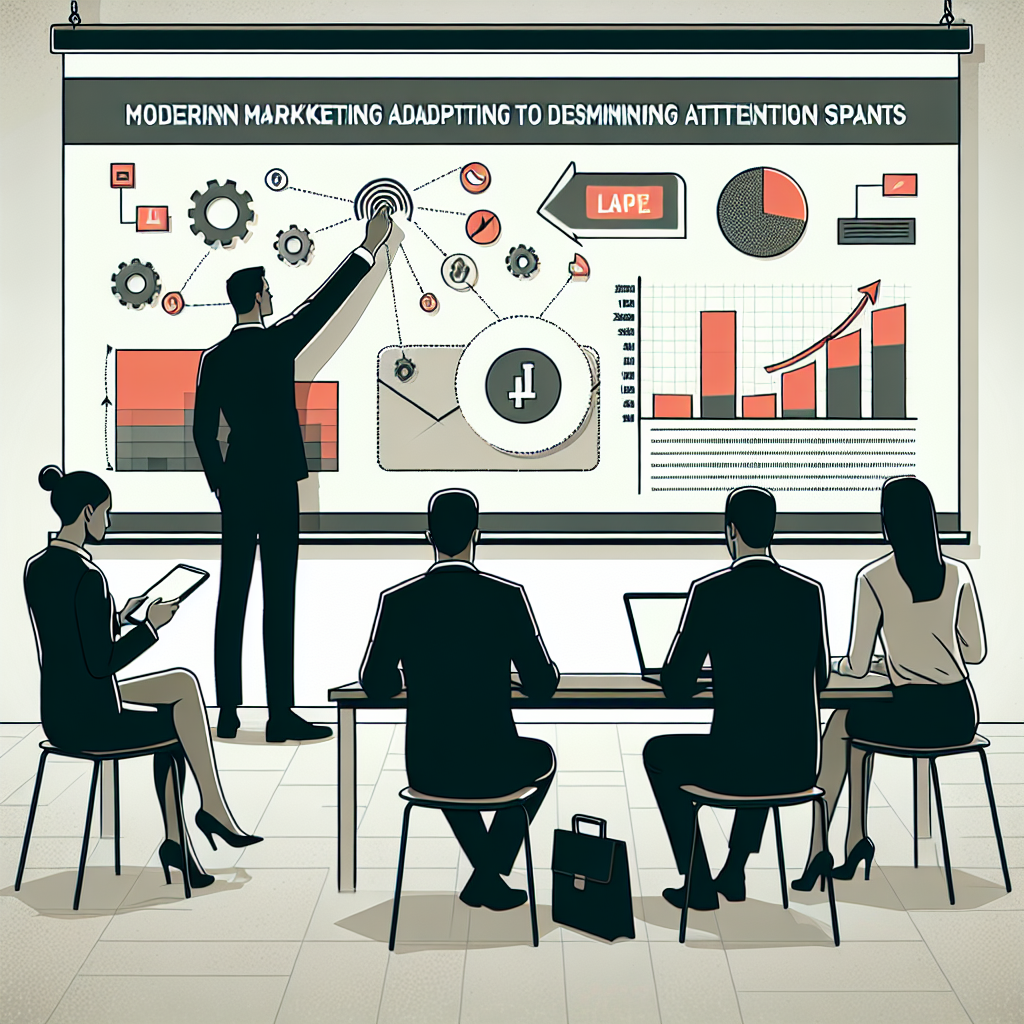
How the Attention Recession Is Changing Marketing
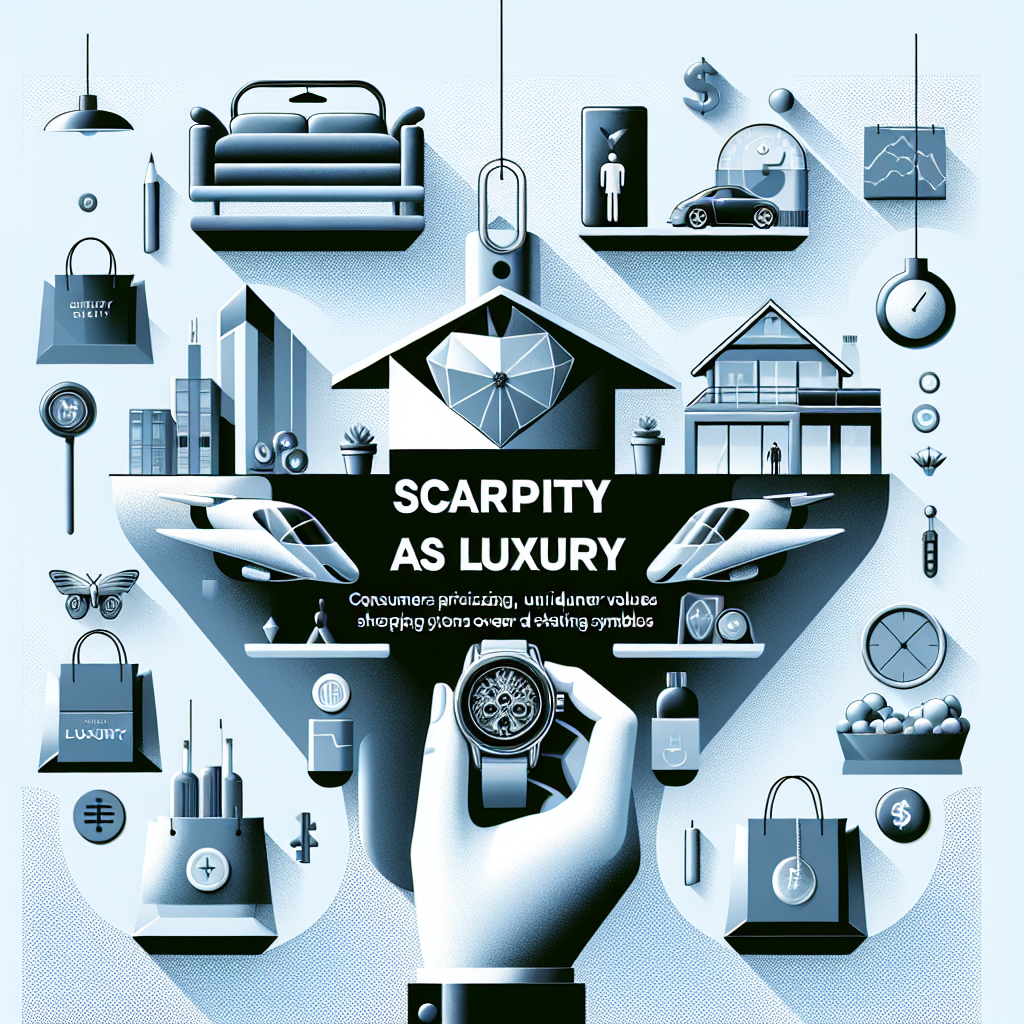
The New Luxury Why Consumers Now Value Scarcity Over Status
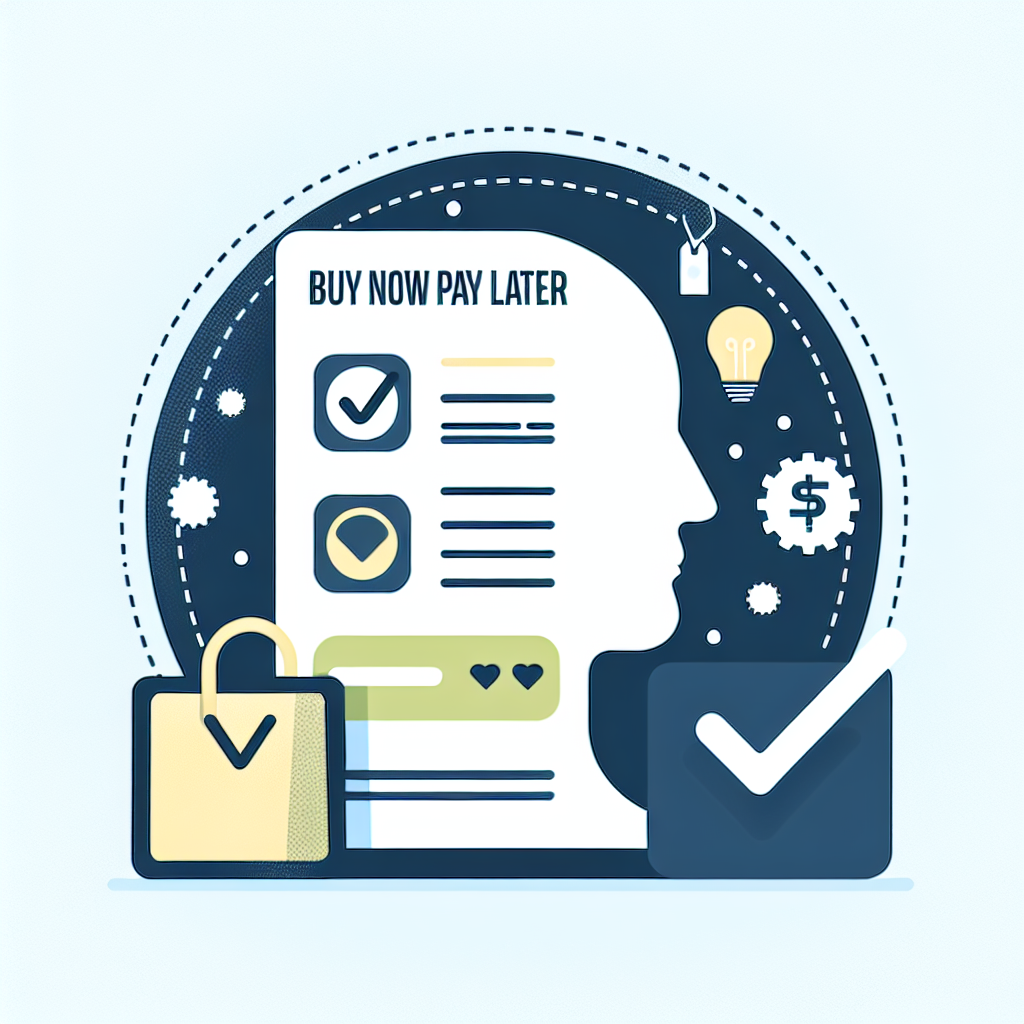
The Psychology Behind Buy Now Pay later
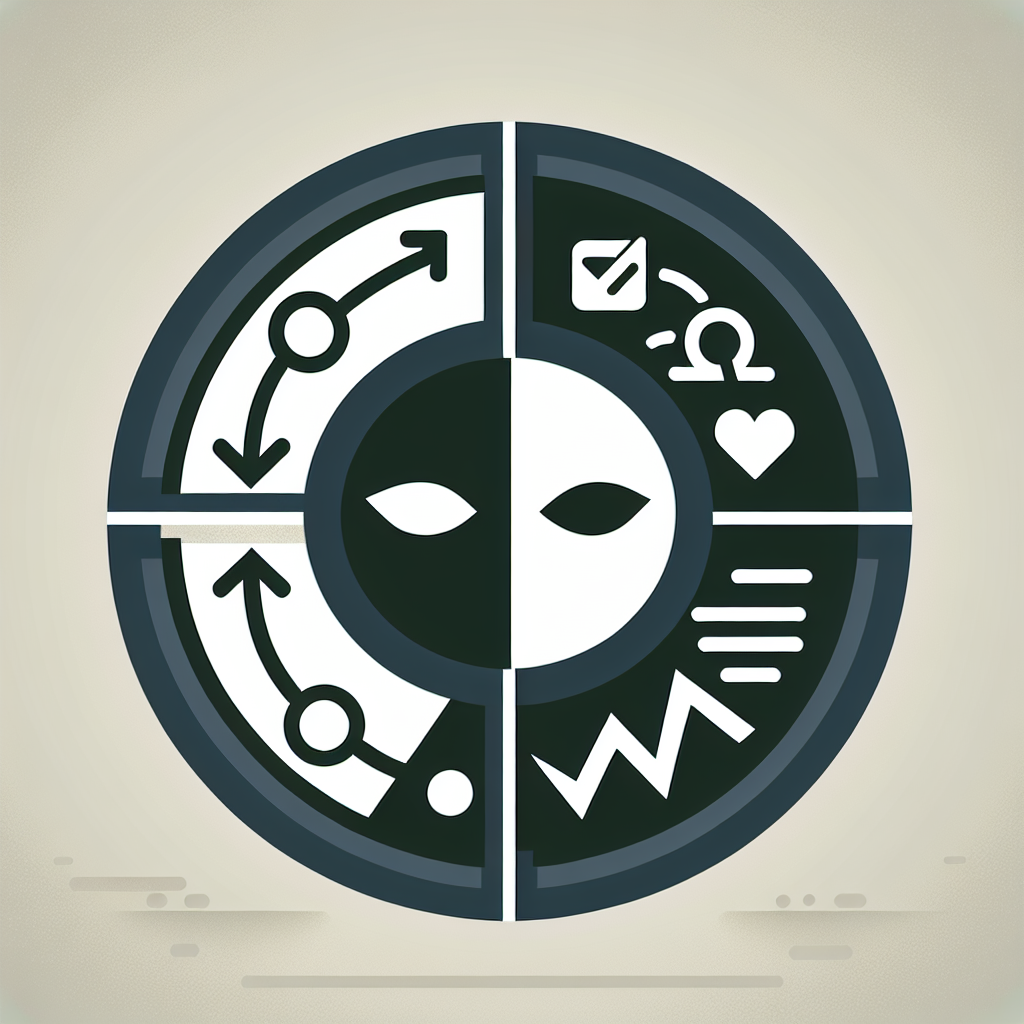
The Role of Dark Patterns in Digital Marketing and Ethical Concerns
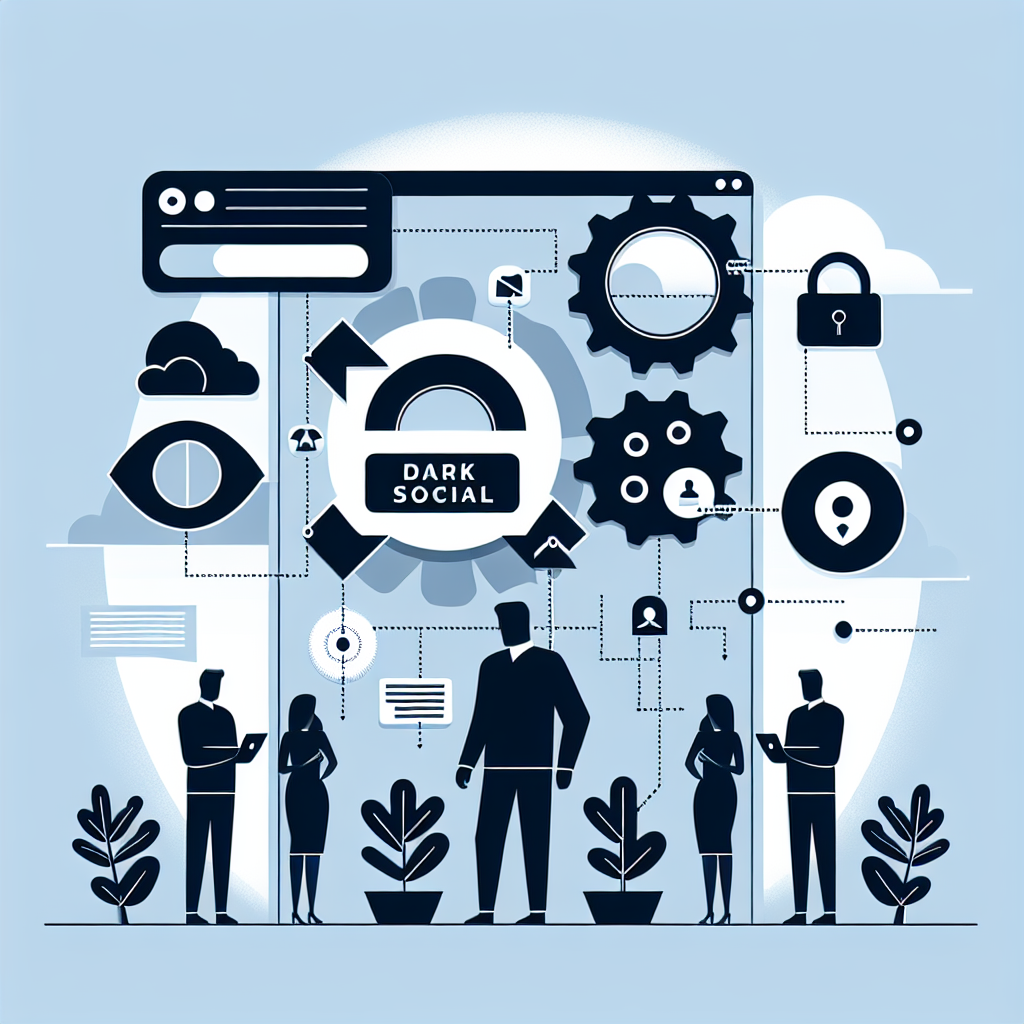
The Rise of Dark Social and Its Impact on Marketing Measurement
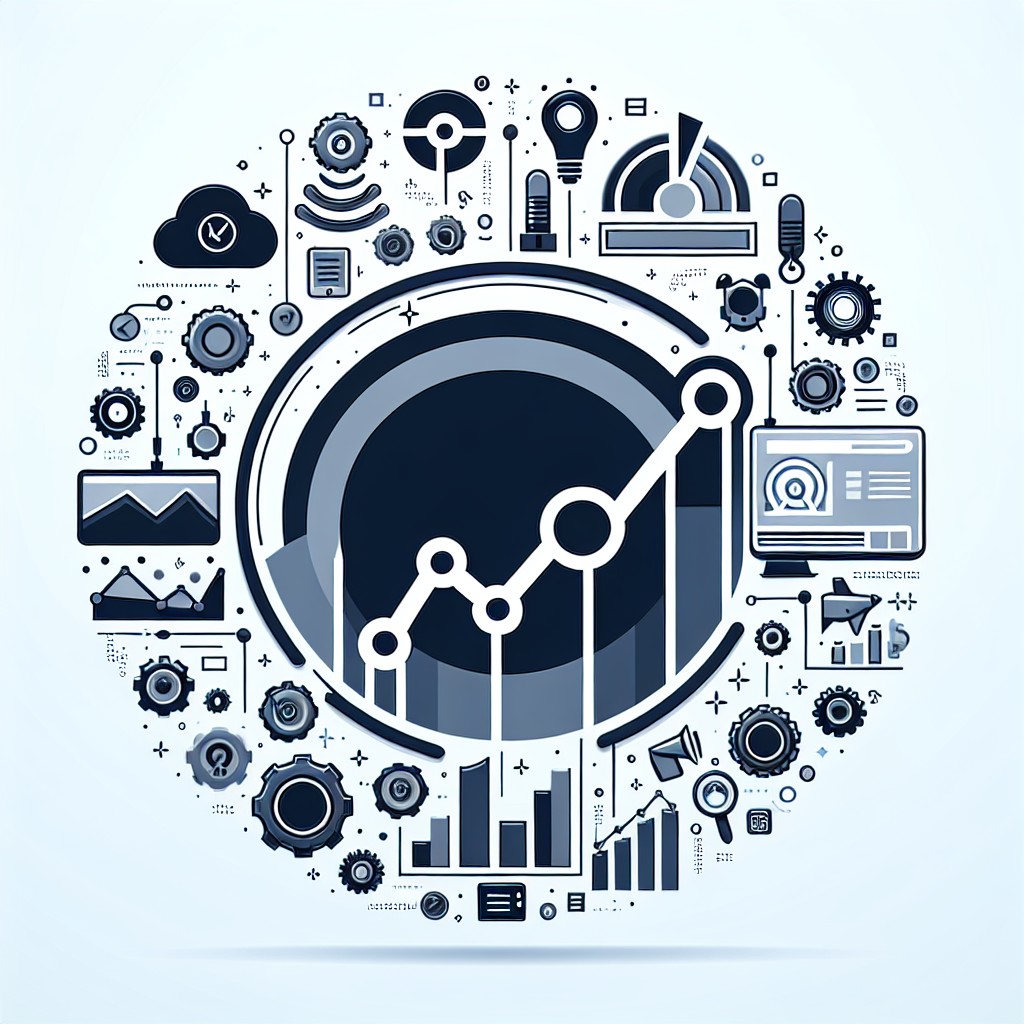