Creating an Experimentation Backlog
During a quarterly strategy meeting, the realization struck Arun. The marketing team was conducting tests, but without structure or clear purpose. Ideas were being randomly implemented based on who spoke loudest in meetings or what competitors were doing. After one particularly heated debate about which landing page variation to try next, Arun sketched a simple matrix on the whiteboard—effort on one axis and potential impact on the other. He asked everyone to place their test ideas on this grid. The visual clarity was immediate and transformative. High-impact, low-effort ideas that had been overlooked suddenly became obvious priorities. The team began documenting each test thoroughly, including hypotheses and outcomes. Within six months, their conversion rates improved by 43%, but more importantly, they developed a testing culture that valued evidence over opinion. This experience launched Arun's exploration into systematic experimentation frameworks, revealing how organized testing backlogs could transform marketing effectiveness beyond random tactical changes.
Introduction: The Strategic Evolution of Marketing Experimentation
Marketing experimentation has evolved from occasional tactical tests to systematic programs driving organizational learning. This evolution progressed through several distinct phases: from sporadic isolated tests to regular testing programs, from uncoordinated experiments to strategic experimentation roadmaps, and now to the frontier of comprehensive experimentation operating systems that drive continuous improvement across all customer touchpoints.
The development of structured experimentation backlogs—prioritized inventories of test hypotheses organized by strategic value—represents what McKinsey research has identified as "the distinguishing characteristic of digitally mature organizations." In marketing contexts, this approach transforms random testing into systematic hypothesis validation, replacing guesswork with evidence-based decision making.
Research from the Digital Optimization Forum indicates that organizations with formalized experimentation backlogs achieve 36% higher conversion rates and implement 54% more tests annually compared to those with ad-hoc approaches. Meanwhile, analysis published in the International Journal of Digital Marketing found that companies with mature experimentation programs demonstrate 3.1x higher digital revenue growth.
1. Prioritizing by Effort and Impact
The foundation of effective experimentation backlogs begins with systematic prioritization frameworks.
a) Strategic Impact Assessment
Modern impact evaluation frameworks incorporate multiple dimensions:
- Revenue potential quantification
- Strategic initiative alignment scoring
- Customer experience enhancement measurement
- Competitive differentiation potential
Case Study: Travel booking platform Booking.com implemented a comprehensive impact scoring system for their experimentation backlog, requiring each test proposal to receive numerical ratings across five strategic dimensions. This approach revealed that checkout flow optimizations, previously considered tactical improvements, actually represented their highest-impact testing opportunity, leading to a focused experimentation program that increased conversion rates by 22%.
b) Implementation Effort Estimation
Sophisticated effort frameworks quantify multiple resource requirements:
- Development hours estimation
- Cross-functional dependency mapping
- Technical complexity assessment
- Maintenance burden projection
Case Study: E-commerce platform Shopify developed a standardized effort scoring matrix for their experimentation backlog that incorporated both implementation and analysis complexity. This system revealed that several seemingly simple tests actually required significant analytical resources to properly interpret, helping them redirect efforts toward truly efficient opportunities that delivered 31% higher ROI on experimentation investments.
c) Portfolio Optimization Models
Advanced experimentation programs balance test diversity:
- Risk distribution across test types
- Learning value assessment beyond immediate gains
- Capability development considerations
- Testing velocity impact analysis
Case Study: Financial services company Capital One implemented a portfolio approach to experimentation that deliberately balanced quick-win tests with strategic learning opportunities. This balanced backlog approach not only delivered immediate conversion improvements of 17% but also generated insights that influenced their product roadmap, creating sustained competitive advantage through systematic learning.
2. Documenting Outcomes
Capturing and processing test results creates compounding organizational intelligence.
a) Structured Knowledge Management
Leading organizations implement comprehensive documentation frameworks:
- Standardized hypothesis documentation templates
- Results analysis methodologies
- Statistical confidence assessment guides
- Institutional learning categorization systems
Case Study: HubSpot implemented a "Test Wiki" knowledge management system that documented every experiment, including failed tests. This approach revealed patterns in user behavior across multiple experiments that individual test analyses had missed. Their systematic documentation identified that simplification consistently outperformed feature addition across their platform, leading to a product strategy shift that increased activation rates by 34%.
b) Cross-Organizational Learning Distribution
Sophisticated experimentation programs distribute insights effectively:
- Role-based insight distribution mechanisms
- Learning synthesis frameworks
- Implementation guidance development
- Cross-functional insight application workshops
Case Study: Adobe implemented a quarterly "Experimentation Summit" where insights from their extensive testing program were synthesized and distributed across product, marketing, and customer success teams. This systematic knowledge sharing accelerated the application of insights across their organization, resulting in 28% faster implementation of successful test outcomes across their product portfolio.
3. Iteration Plans
Translating test results into continuous improvement requires systematic approaches.
a) Test Sequencing Frameworks
Advanced organizations map logical test progressions:
- Build-measure-learn cycle implementation
- Progressive hypothesis refinement methodologies
- Insight dependency mapping
- Validation threshold advancement systems
Case Study: Streaming service Netflix developed a "Family Tree" approach to experimentation where each test spawns multiple follow-up hypotheses, creating branching paths of inquiry. This structured iteration planning increased their testing velocity by 47% while improving the strategic coherence of their experimentation program, driving sustained improvements in viewer engagement metrics.
b) Resource Allocation Optimization
Effective iteration planning optimizes experimentation resources:
- Test velocity balancing with statistical rigor
- Technical debt consideration in implementation
- Analysis capacity planning
- Cross-functional coordination schedules
Case Study: Marketing automation platform Mailchimp implemented quarterly experimentation planning cycles that balanced immediate optimization opportunities with strategic learning initiatives. This approach not only improved their resource utilization by 29% but also increased the implementation rate of successful tests from 62% to 87%, creating accelerated performance improvement across their platform.
Conclusion: The Organizational Impact of Structured Experimentation
As digital marketing continues evolving, experimentation backlogs are transforming from tactical optimization tools to strategic assets driving organizational learning and adaptation. The companies achieving breakthrough performance are those moving beyond random testing toward experimentation operating systems that continuously validate assumptions and generate insights.
The integration of AI into experimentation management is enabling previously impossible test ideation, analysis, and application—identifying patterns across hundreds of experiments to reveal fundamental customer behavior principles rather than isolated tactical insights.
As these methodologies mature, experimentation is increasingly becoming a core organizational capability rather than a marketing function, creating unprecedented opportunities for companies to adapt to changing market conditions with evidence-based agility.
Call to Action
For marketing leaders seeking to establish experimentation excellence:
- Implement experimentation management platforms that support structured hypothesis development
- Develop clear prioritization frameworks balancing immediate gains with strategic learning
- Establish knowledge management systems that capture insights beyond test results
- Create cross-functional experimentation communities spanning marketing, product, and analytics
- Integrate experimentation outcomes into strategic planning and investment decisions
The future of marketing effectiveness belongs not to those with the biggest budgets or the most creative teams, but to organizations that systematically discover what works for their specific business context through disciplined, strategic experimentation programs that build compounding intelligence over time.
Featured Blogs
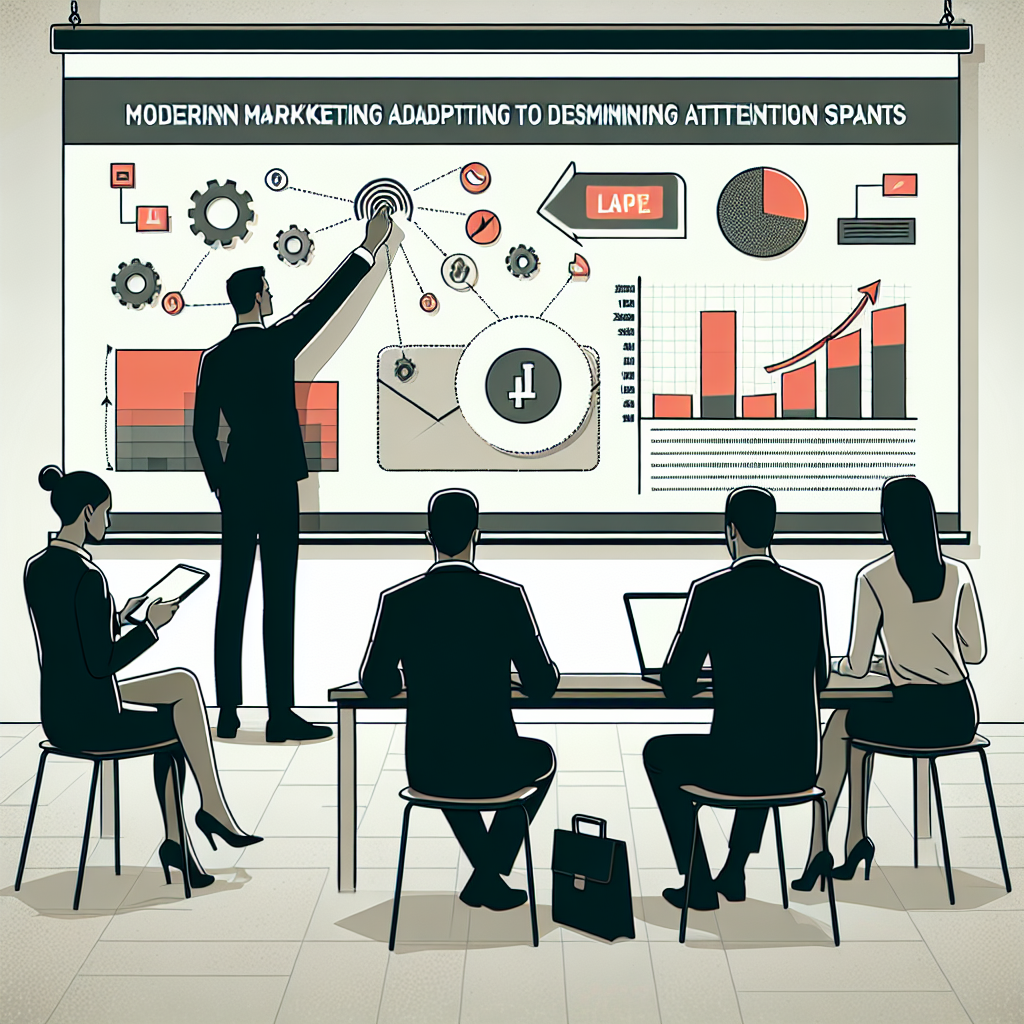
How the Attention Recession Is Changing Marketing
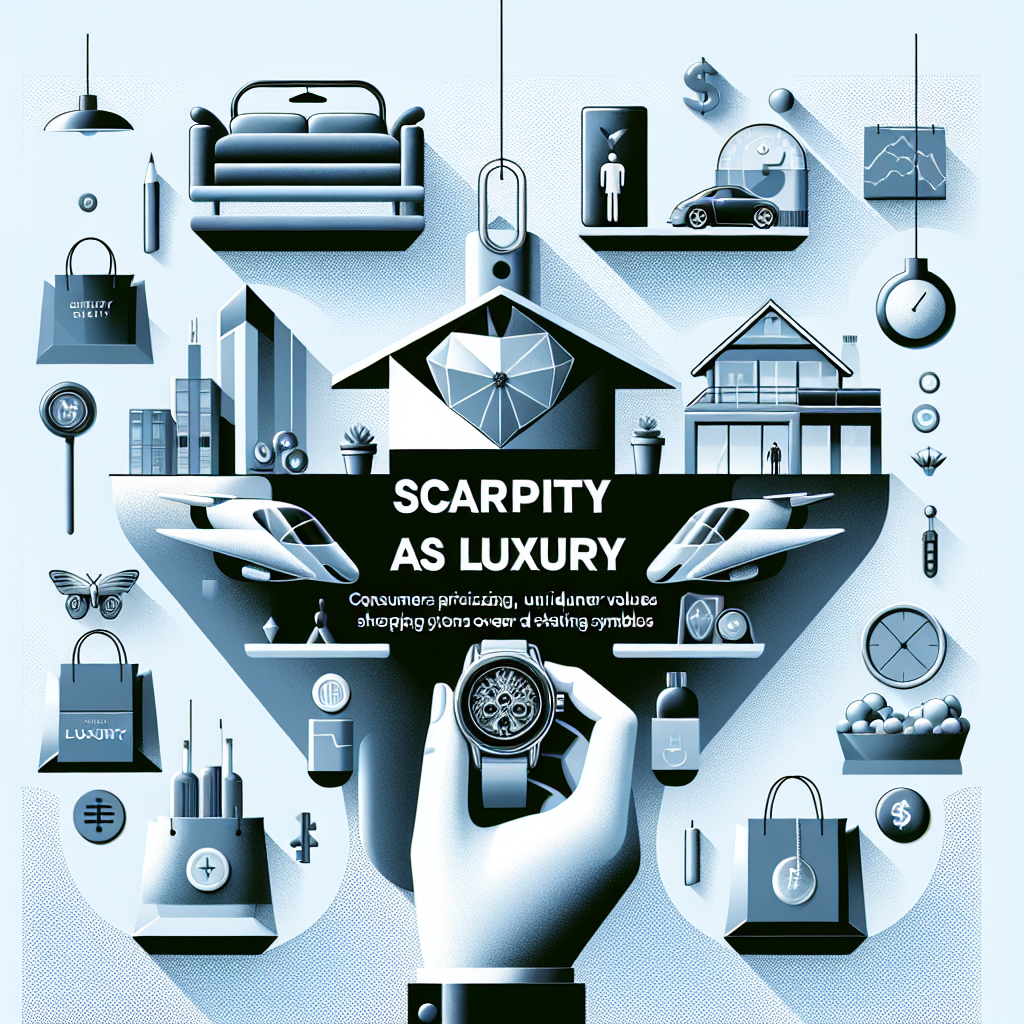
The New Luxury Why Consumers Now Value Scarcity Over Status
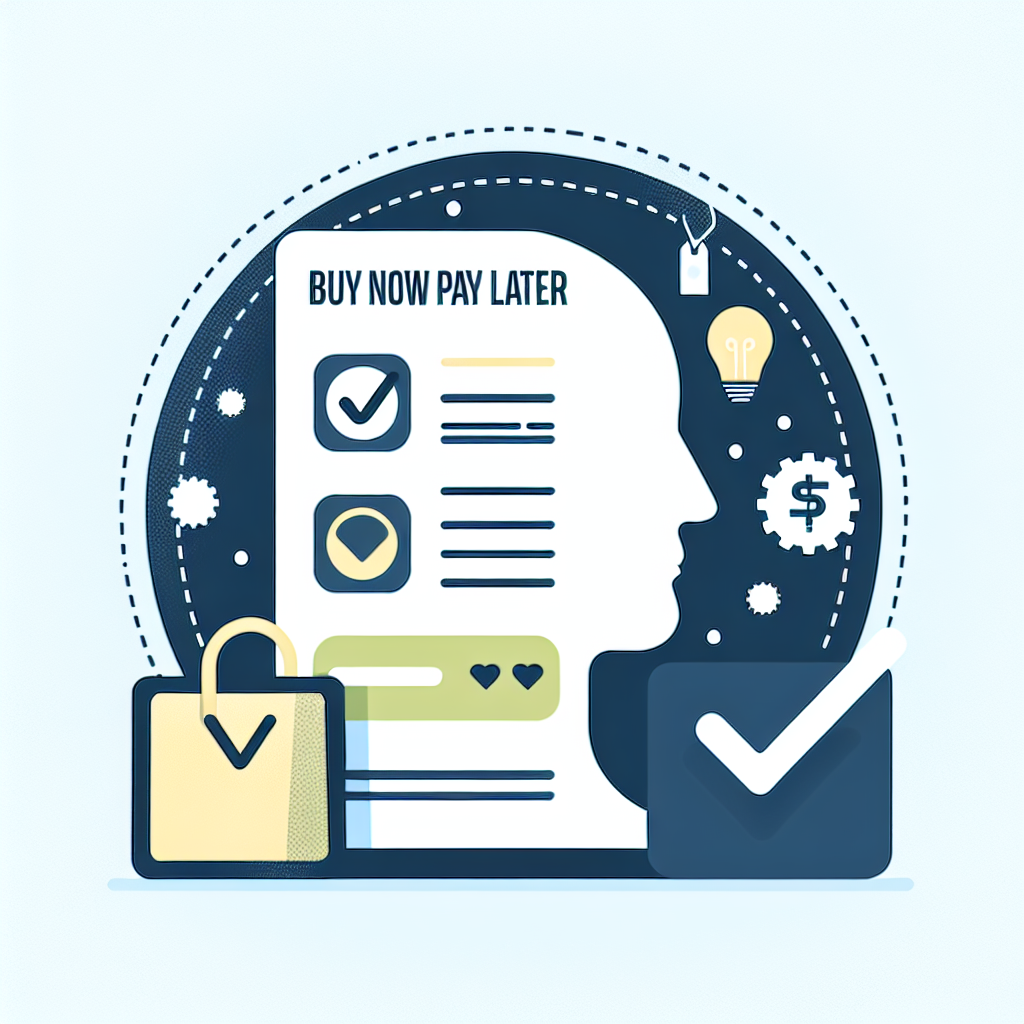
The Psychology Behind Buy Now Pay later
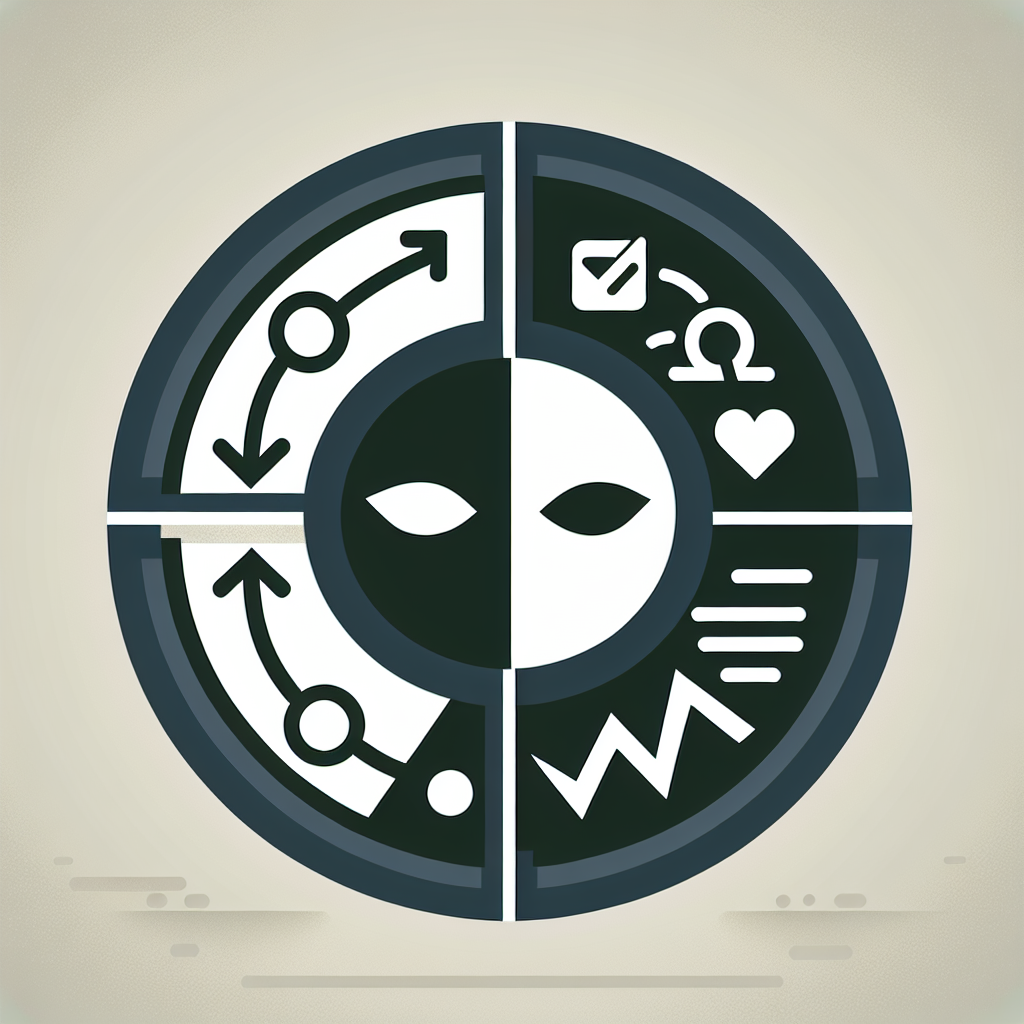
The Role of Dark Patterns in Digital Marketing and Ethical Concerns
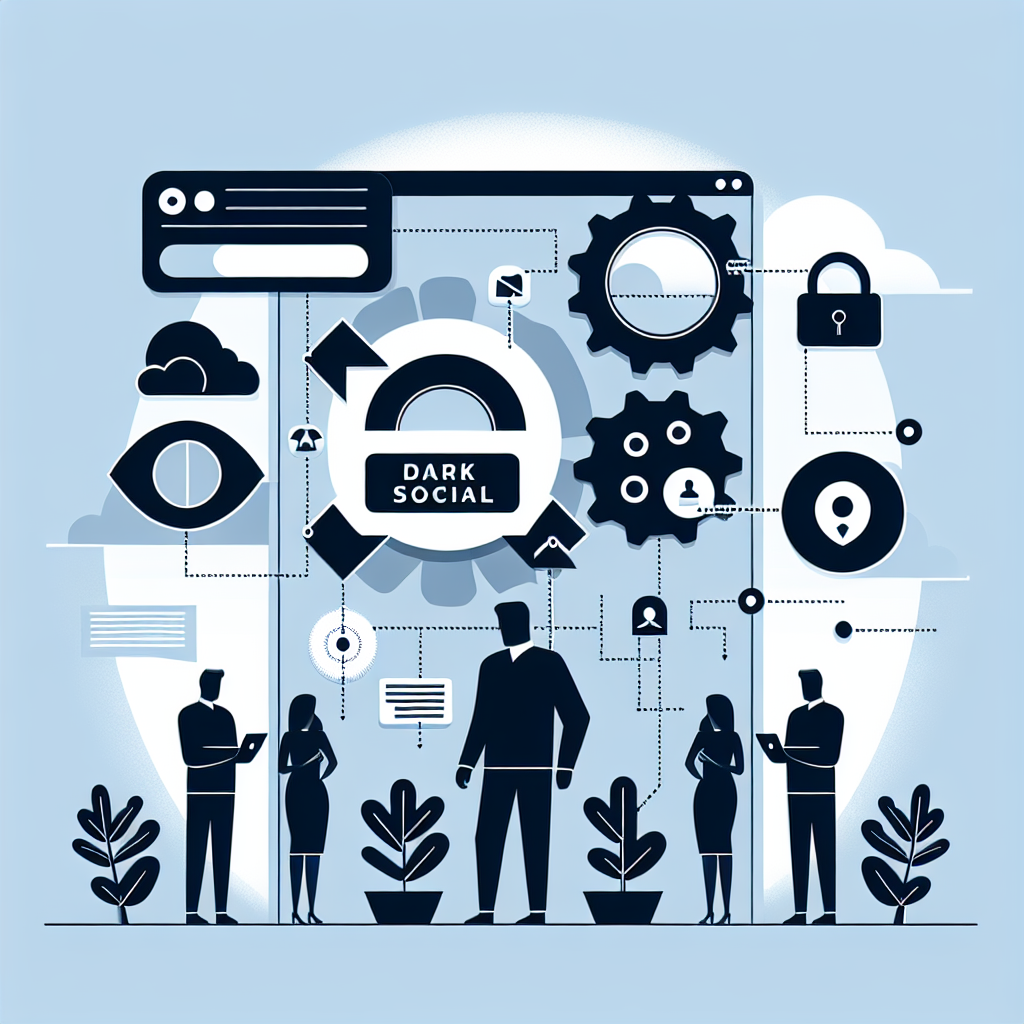
The Rise of Dark Social and Its Impact on Marketing Measurement
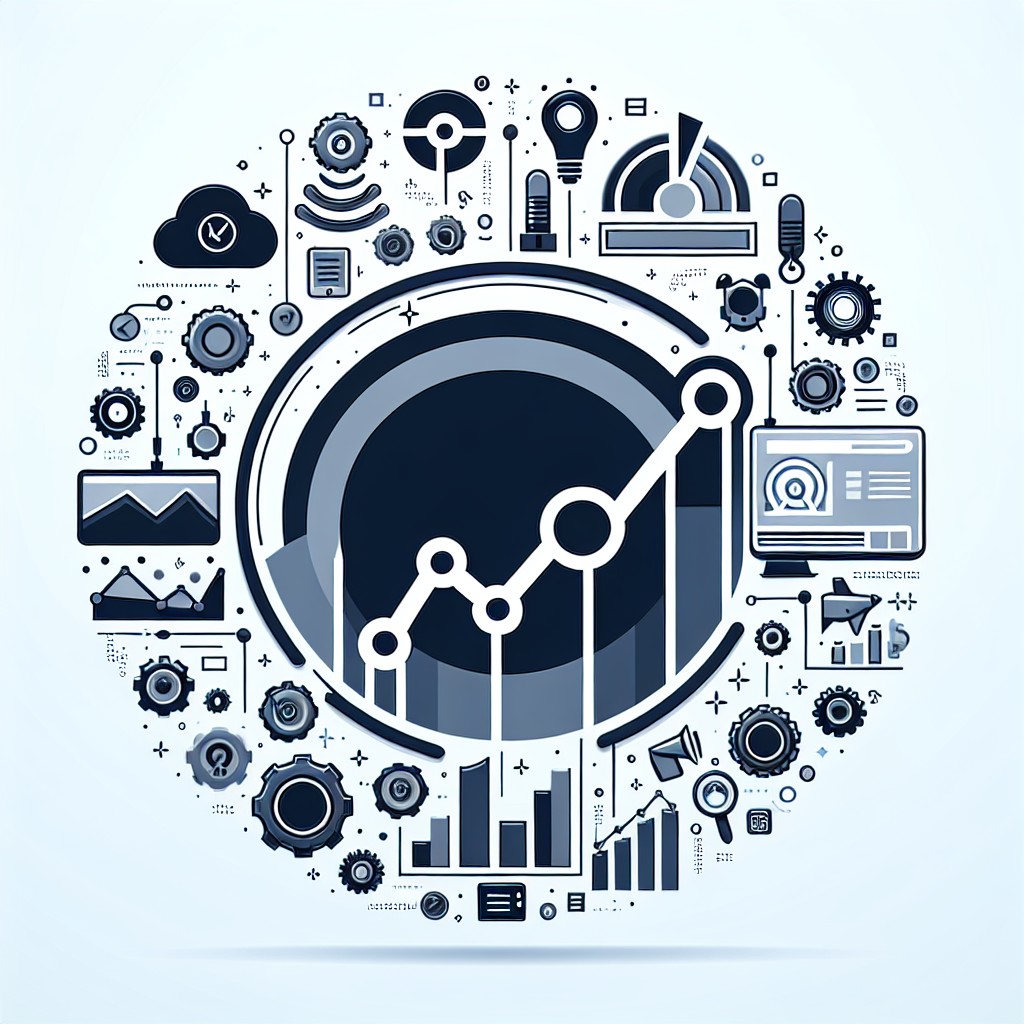