Data-Led GTM Launch Debriefs
The pattern finally emerged for Nitish after their third consecutive product launch followed an eerily similar trajectory—strong initial adoption, followed by a sudden engagement cliff at week four. Gathering cross-functional leaders into their war room, Nitish projected six months of user data across three launches. The silence was deafening as everyone processed the identical drop-off patterns. The VP of Product finally broke the silence: "We've been celebrating early wins without seeing the complete story." That afternoon transformed their entire go-to-market approach as they restructured their launch evaluation framework to capture leading indicators that predicted these engagement cliffs weeks before they materialized. What began as a troubleshooting session evolved into a systematic rethinking of how they measure, interpret, and learn from launch data across organizational boundaries. This experience sparked Nitish's fascination with data-led GTM launch debriefs, revealing how structured post-launch analysis could transform not just future launches but fundamentally improve product development and organizational learning.
Introduction: The Evolution of Launch Analysis
Product and service launches have evolved from intuition-driven events measured by sales figures to data-informed campaigns evaluated through multidimensional success metrics. This evolution represents a fundamental shift in how organizations approach market entry—moving from siloed departmental responsibility to integrated cross-functional accountability.
Research from McKinsey Digital indicates that companies implementing structured data-led debriefs experience 38% higher success rates on subsequent launches. Meanwhile, analysis from the Product Development and Management Association shows that organizations with formal launch learning systems achieve market traction 2.7x faster than those without such frameworks.
1. Cross-functional Metrics
Modern launch evaluation requires integrated measurement systems that connect departmental objectives while revealing interdependencies.
Integrated Success Definitions
Contemporary launch frameworks unify departmental goals:
- Unified OKR alignment across departments
- Leading indicator interdependency mapping
- Shared accountability metrics
- Success threshold consistency
Example: Adobe's Creative Cloud shifted to what they call a "Launch Lattice" framework, where Marketing, Product, Sales, and Customer Success teams establish interlocking metrics with explicit dependencies. Their system identifies how marketing acquisition quality impacts product activation rates, which influence sales expansion opportunities and ultimately drive customer lifetime value. This integrated approach increased cross-sell conversion by 23% while reducing customer acquisition costs by 17%.
Attribution Model Alignment
Advanced attribution connects user journeys across touchpoints:
- Multi-touch attribution across department boundaries
- Incrementality testing frameworks
- Channel interaction effect measurement
- Sequential influence modeling
Example: Slack implemented "GTM Attribution Mesh" to track how pre-launch activities influenced post-launch behaviors. Their system revealed that developer documentation quality was a stronger predictor of enterprise deployment success than initial sales engagement, leading to a fundamental redesign of their enterprise GTM sequence that accelerated deployment timelines by 41%.
2. Lag vs. Lead Indicators
Identifying predictive signals that forecast outcomes before they materialize represents a critical evolution in launch intelligence.
Predictive Signal Identification
Modern launch analysis seeks early predictive patterns:
- Early behavior pattern analysis
- Engagement sequence modeling
- Drop-off precursor identification
- Activation quality assessment
Example: Shopify developed a "Signal Cascade Model" that identified specific merchant onboarding behaviors occurring within the first 72 hours that predicted 90-day success with 83% accuracy. This model allowed intervention teams to identify at-risk merchants based on early signals rather than waiting for churn to materialize, increasing merchant retention by 28%.
Intervention Trigger Systems
Advanced approaches establish automated response mechanisms:
- Real-time alert thresholds
- Graduated intervention protocols
- Cross-functional response coordination
- Dynamic resource allocation
Example: HubSpot created an "Early Warning Network" that monitors 17 leading indicators during new feature launches, automatically triggering specific team responses when metrics deviate from expected ranges. When their CRM redesign showed engagement pattern concerns, this system activated targeted training campaigns three weeks before traditional metrics would have revealed issues, preserving 34% of potentially churned users.
3. Learning Repository
Systematically capturing, organizing, and operationalizing launch insights transforms individual experiences into organizational knowledge.
Knowledge Capture Systems
Structured approaches convert observations into actionable intelligence:
- Standardized debrief protocols
- Pattern recognition frameworks
- Assumption validation documentation
- Counter-narrative evaluation
Example: Atlassian established a "Launch Learning Library" with standardized templates capturing not just what happened during launches but why outcomes differed from expectations. Their system explicitly documents base assumptions, market responses, and attribution analysis. This repository has been credited with helping them reduce "repeat mistakes" by 72% across product lines while accelerating successful pattern replication.
Cross-Launch Pattern Recognition
Advanced systems identify success patterns across diverse initiatives:
- Meta-analysis of launch performance
- Success factor identification
- Failure pattern recognition
- Contextual variable isolation
Example: Salesforce implemented "Launch Intelligence" methodology that analyzes patterns across product launches to isolate universal success factors from context-specific variables. This approach revealed that enterprise adoption speed correlated more strongly with integration ecosystem readiness than with feature completeness, redirecting pre-launch investment priorities and improving time-to-value by 37% for new offerings.
Conclusion: The Future of Launch Intelligence
The future of effective go-to-market strategies lies not in departmental expertise but in organizational learning systems that turn launch experiences into institutional knowledge. As market conditions continue evolving with increasing speed, companies that establish robust debrief protocols, identify meaningful leading indicators, and maintain cross-functional alignment will build sustainable competitive advantage through superior market execution.
The most successful organizations will move beyond seeing launches as isolated events and recognize them as learning opportunities that shape organizational capability over time. By treating each launch as both a market entry and a knowledge creation opportunity, businesses can create enduring value while continuously refining their go-to-market approach.
Call to Action
For executives and teams seeking to transform their launch performance:
- Establish cross-functional debrief protocols that integrate departmental perspectives
- Develop leading indicator dashboards that provide early warning capabilities
- Create searchable learning repositories with standardized documentation
- Implement pattern recognition systems across multiple launches
- Foster a culture that values learning from both successes and failures.
Featured Blogs
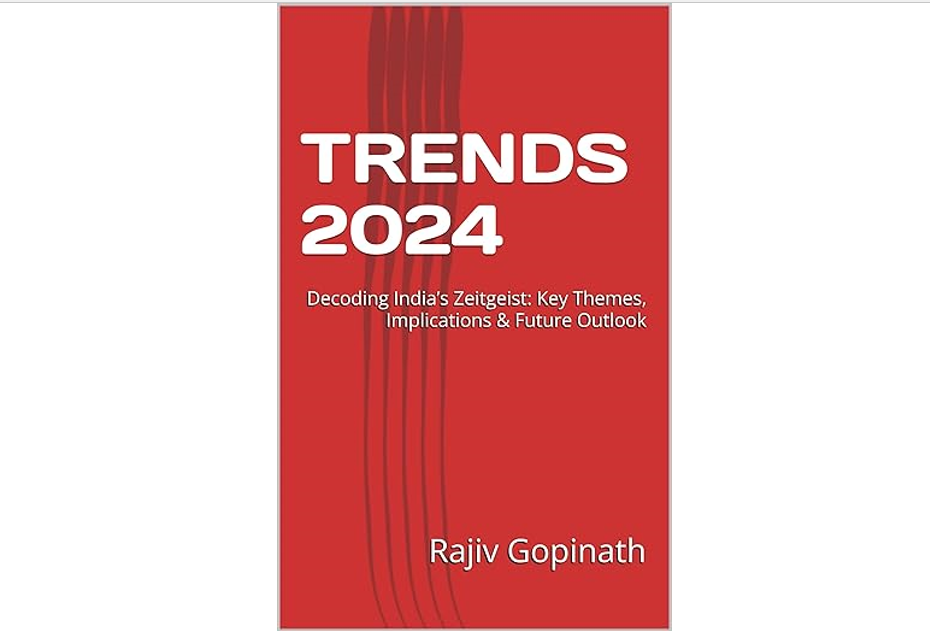
TRENDS 2024: Decoding India’s Zeitgeist: Key Themes, Implications & Future Outlook
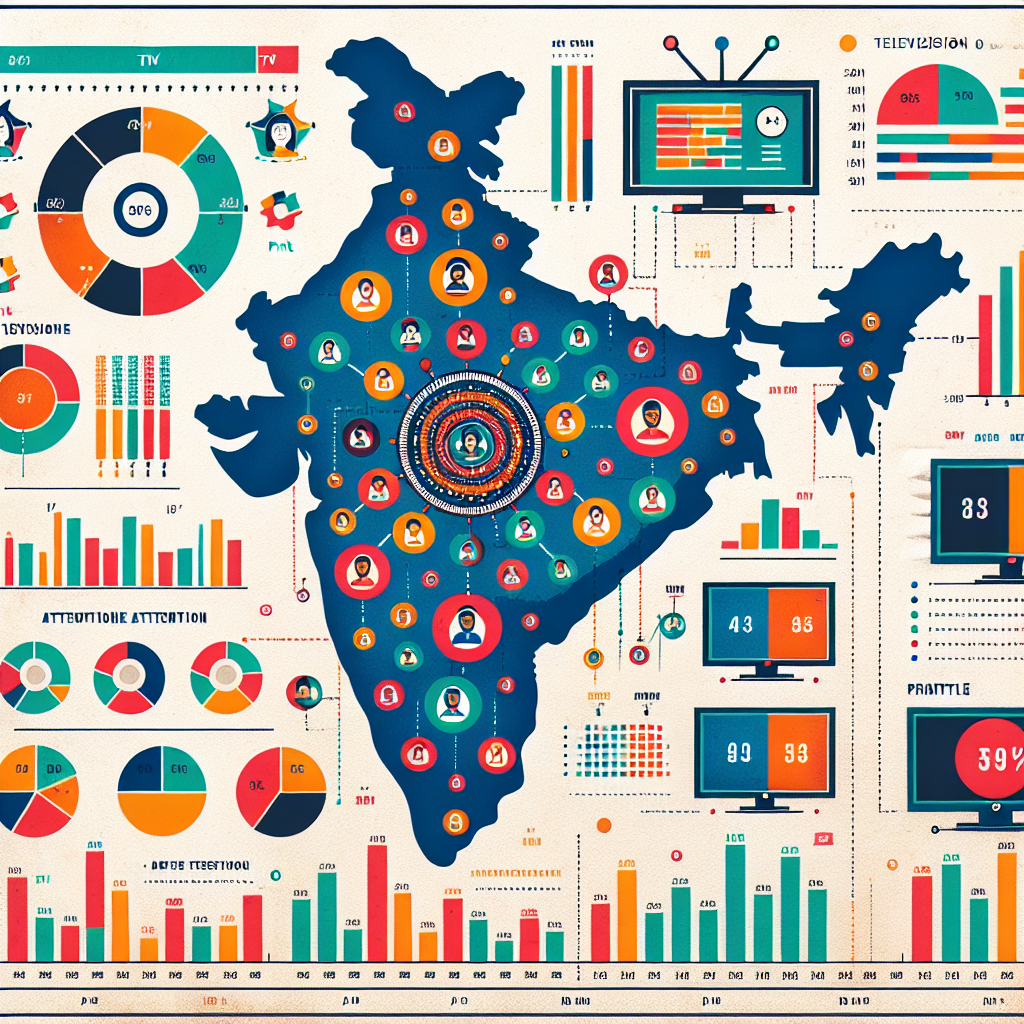
How to better quantify attention in TV and Print in India
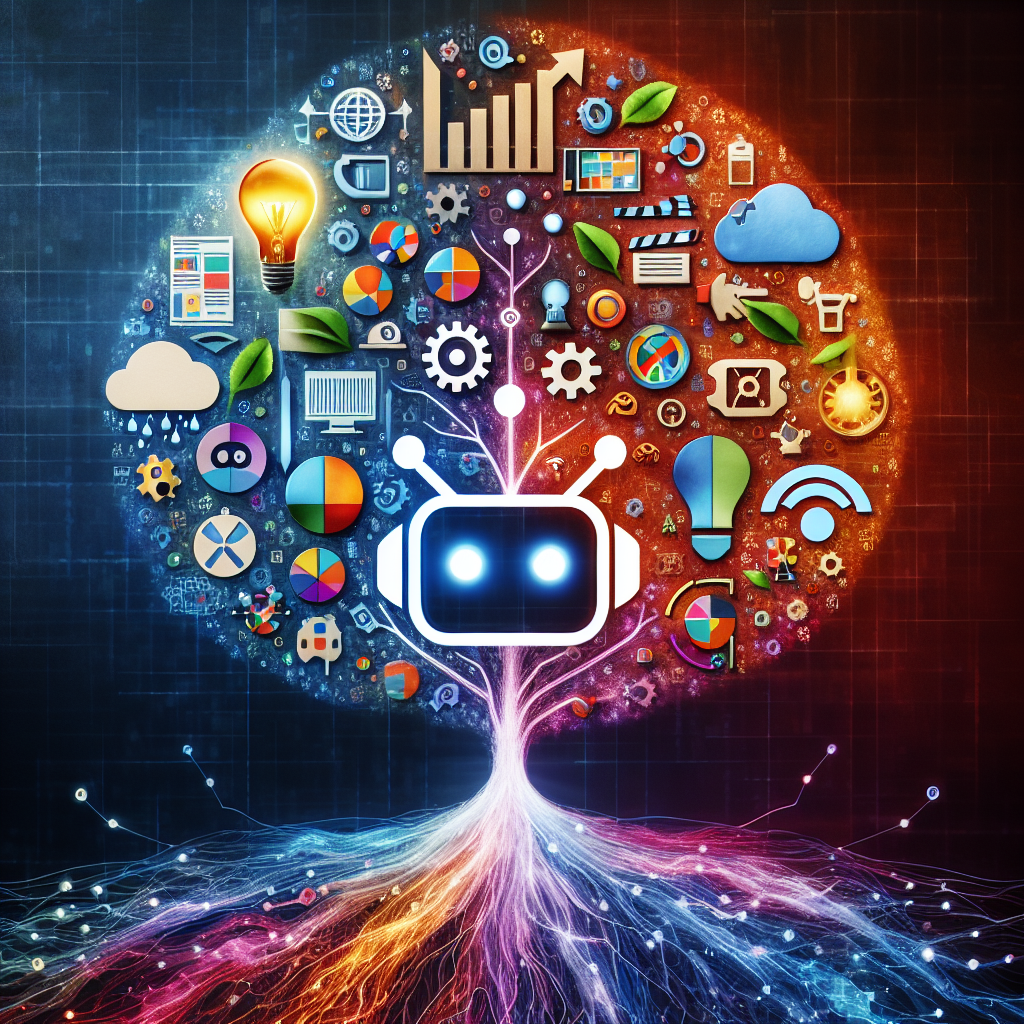
AI in media agencies: Transforming data into actionable insights for strategic growth
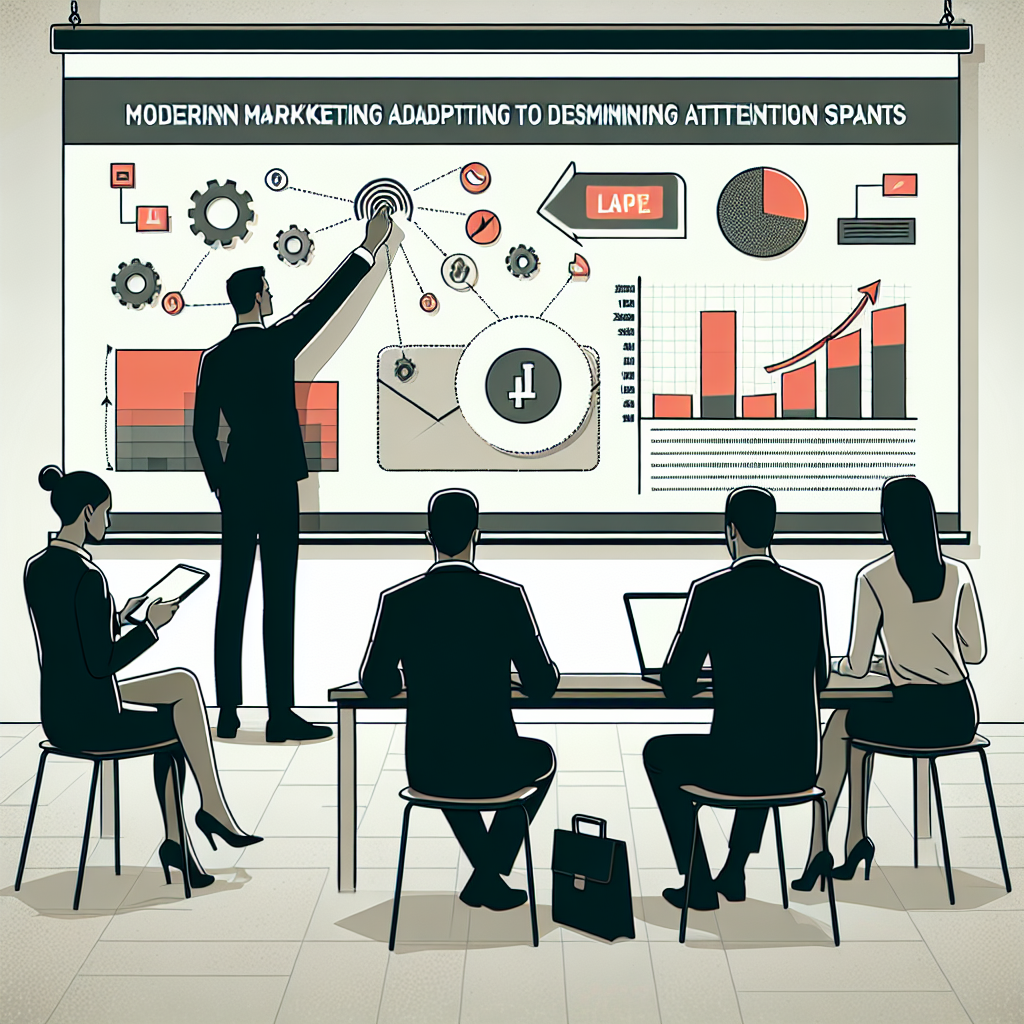
How the Attention Recession Is Changing Marketing
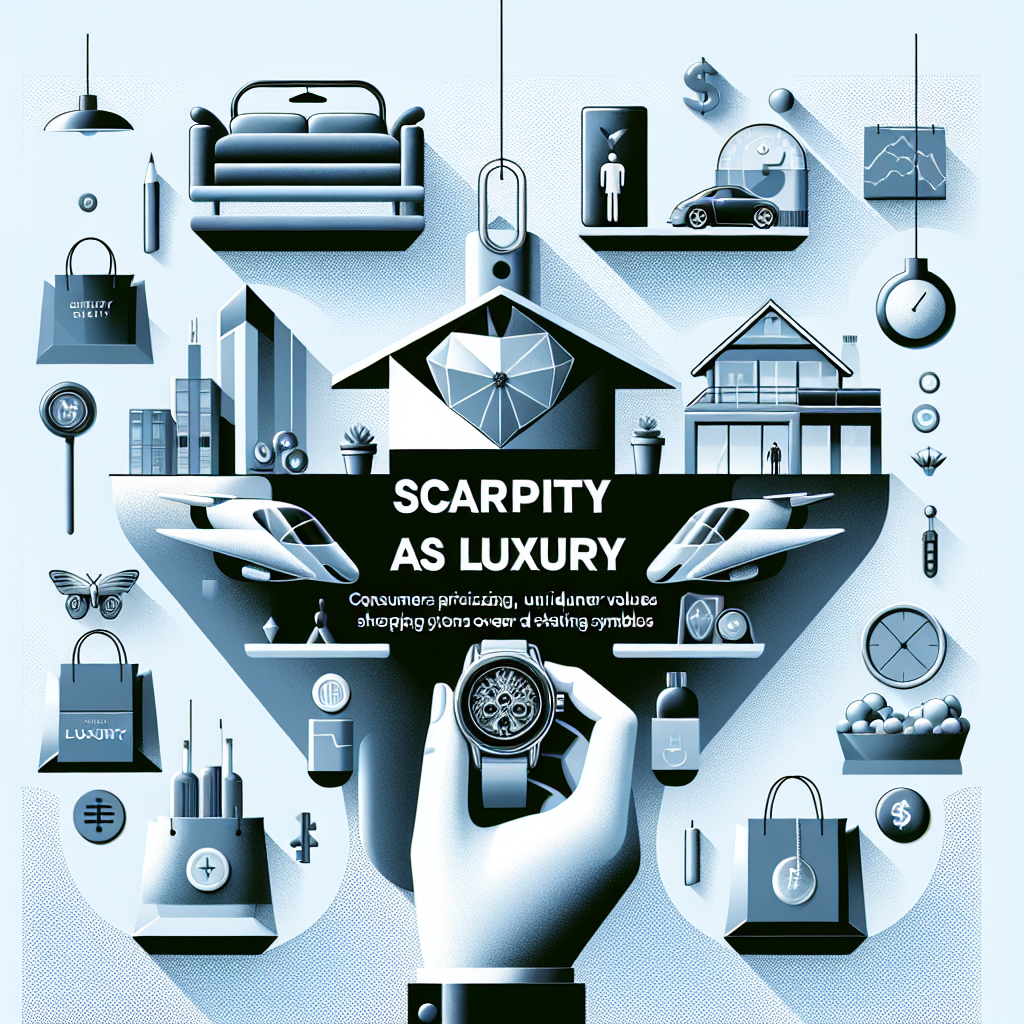
The New Luxury Why Consumers Now Value Scarcity Over Status
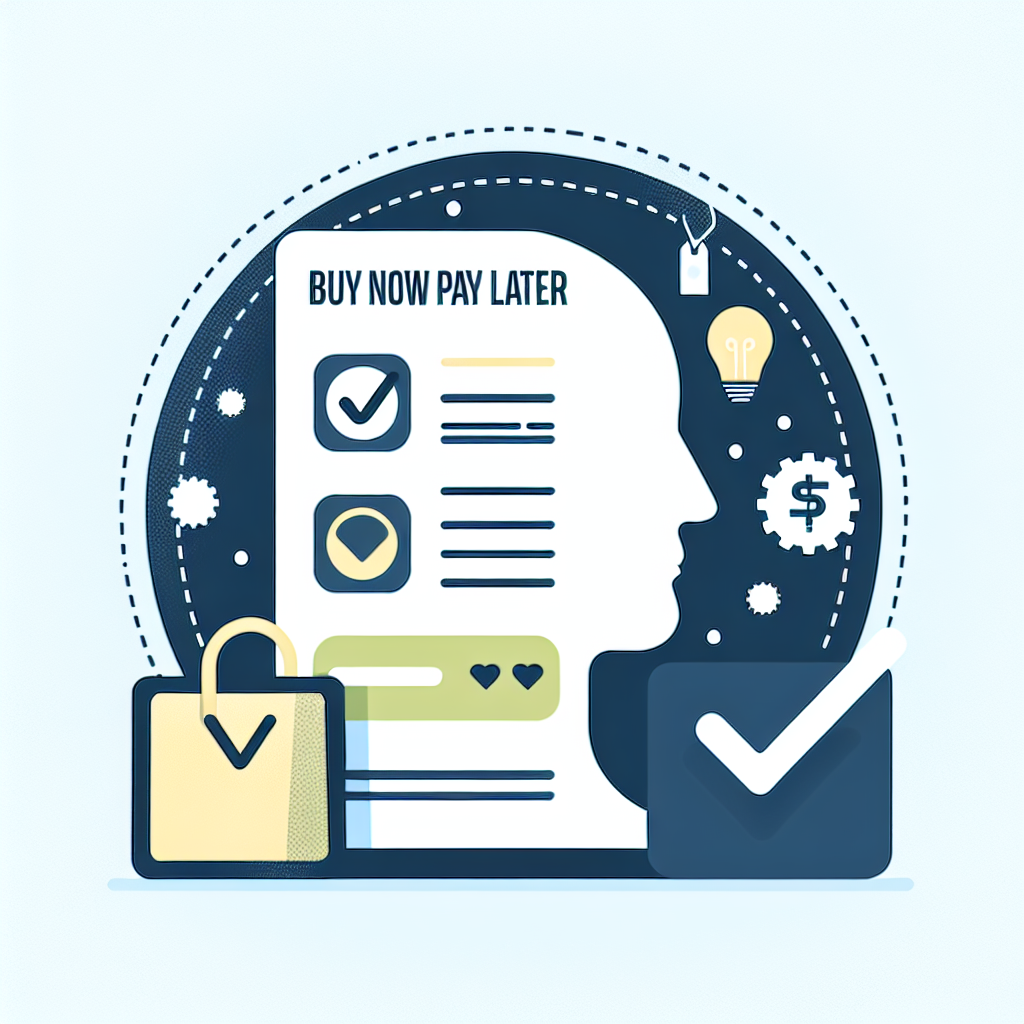
The Psychology Behind Buy Now Pay later
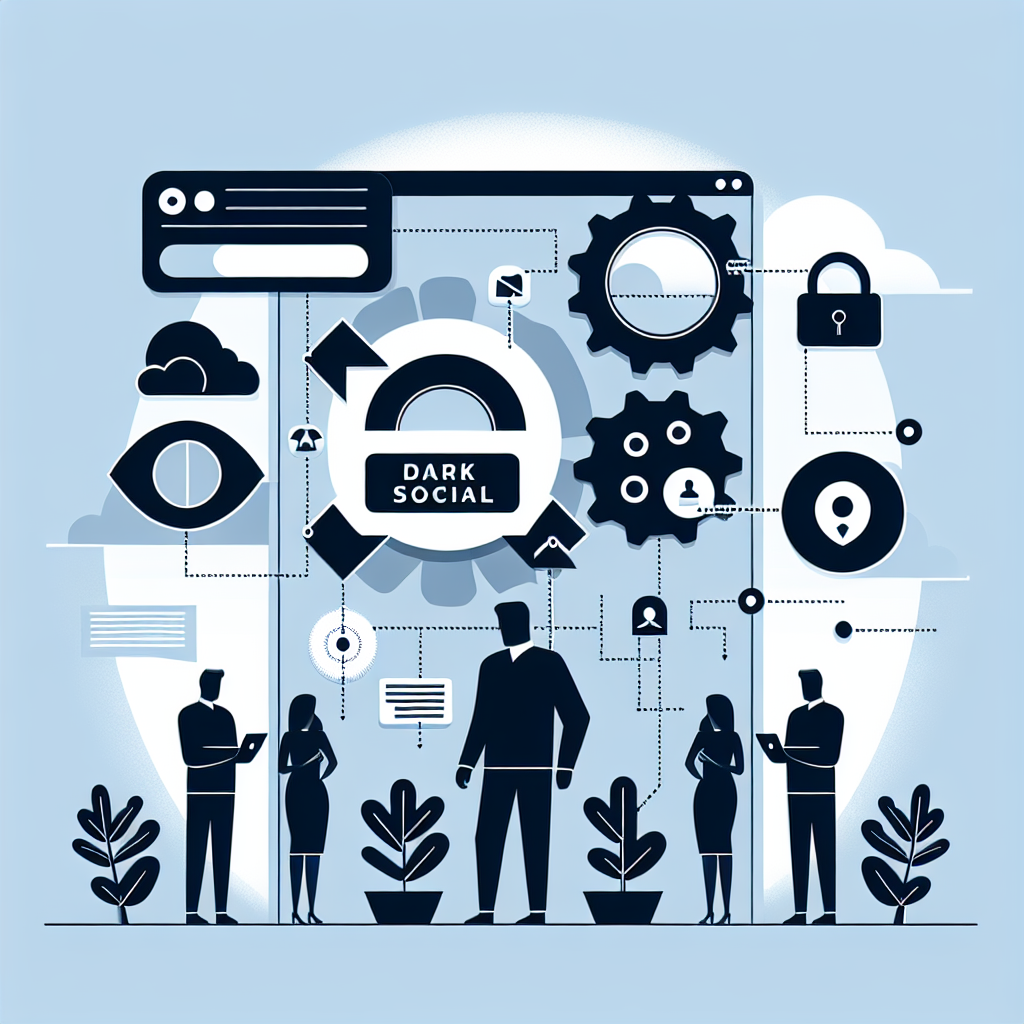
The Rise of Dark Social and Its Impact on Marketing Measurement
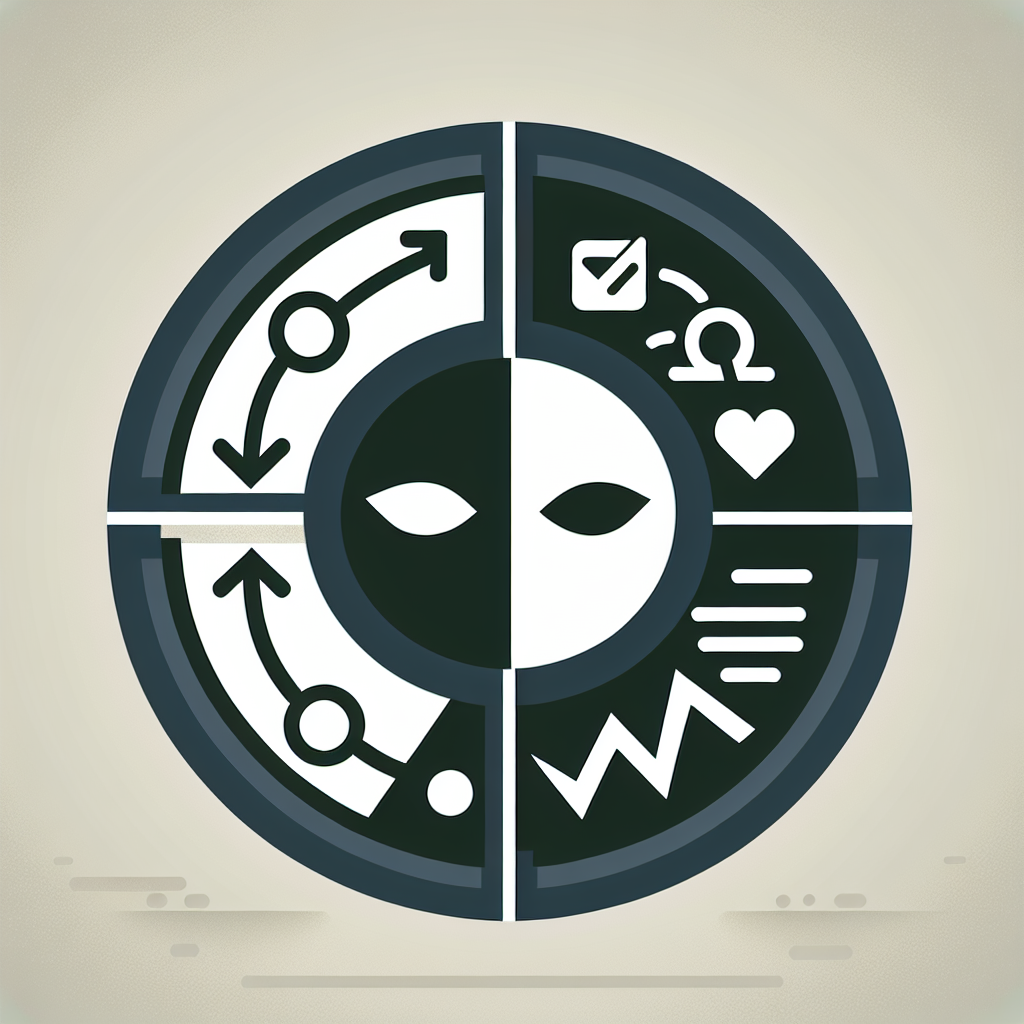
The Role of Dark Patterns in Digital Marketing and Ethical Concerns
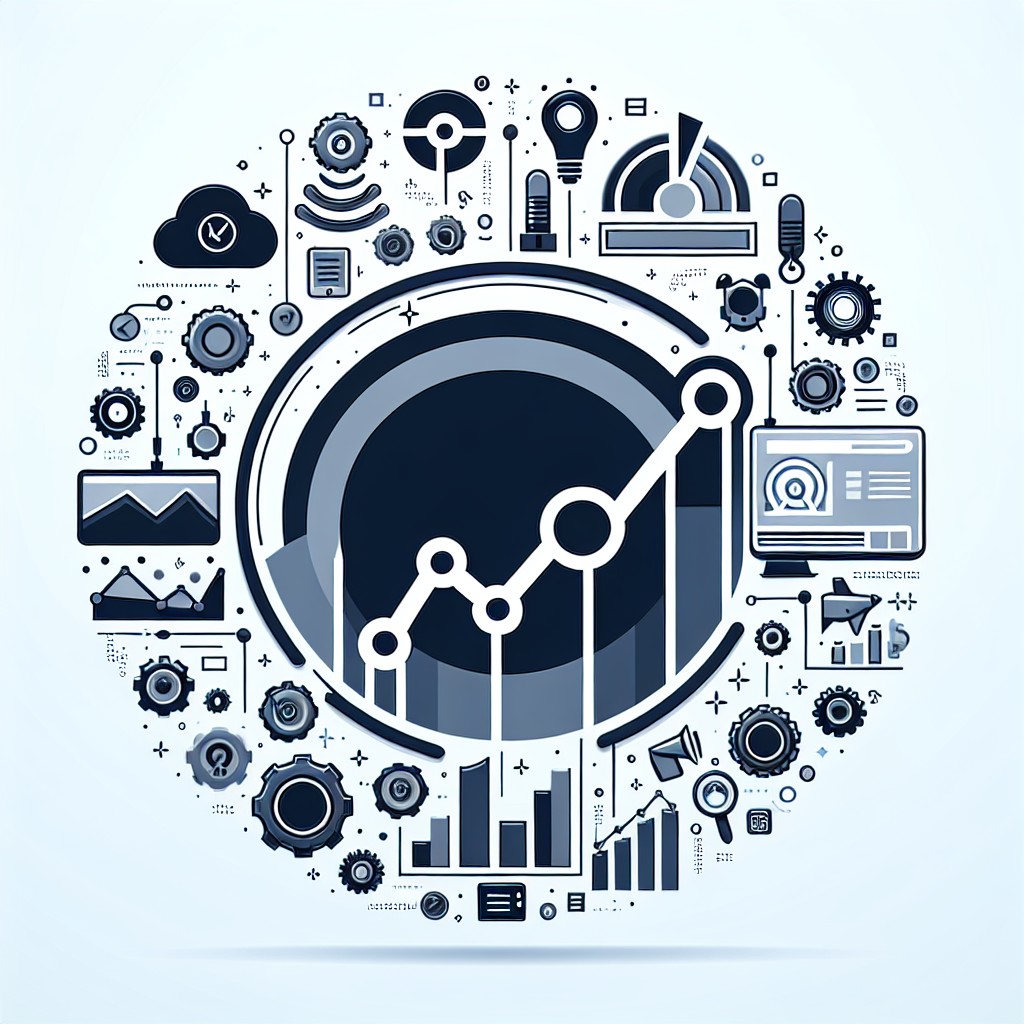