Segmenting Beyond Demographics
The aha moment occurred for Arun during a marketing strategy session he was leading for a subscription-based media company struggling with plateauing growth. As they reviewed their traditional demographic segments—age ranges, income brackets, education levels—something felt fundamentally disconnected from the actual customer behaviors they were observing. On a whim, Arun suggested they reorganize their audience data based on content consumption patterns rather than demographic attributes. The resulting visualization revealed something remarkable: clusters of highly similar behavior that cut across wildly different demographic groups. For instance, a 23-year-old urban designer and a 67-year-old retired teacher were consuming almost identical content, while two 35-year-old suburban professionals with matching demographic profiles showed completely divergent preferences. "We've been speaking different languages to people who want the same thing, and the same language to people who want different things," the CMO realized. That moment transformed Arun's understanding of audience segmentation, illustrating that identity-based attributes may be less predictive of needs and behaviors than what people actually do, value, and need. This experience launched Arun's exploration into multidimensional segmentation approaches, revealing how organizations that move beyond demographic shortcuts can discover dramatically more effective ways to understand and serve their audiences.
Introduction: The Multidimensional Evolution of Market Segmentation
The practice of market segmentation has progressed through several evolutionary stages as organizations have developed increasingly sophisticated understanding of what truly drives customer behavior. This evolution has moved from demographic profiling to psychographic analysis, from firmographic categorization to behavioral observation, and now to the frontier of multidimensional segmentation that integrates behavioral patterns, value indicators, and need states into unified audience understanding.
The shift beyond demographic-centric segmentation represents what the Journal of Consumer Research has identified as "the third wave of segmentation science"—where organizations recognize that who customers are may be less important than what they do, what they value, and what they need in specific contexts.
Research from McKinsey indicates that organizations implementing multidimensional segmentation approaches demonstrate 26% higher marketing ROI and 22% stronger customer retention compared to those relying primarily on demographic models. Meanwhile, analysis from the Marketing Science Institute suggests that behaviorally-driven segmentation produces 3.2x more predictive power for purchase intent than demographic variables alone.
As segmentation expert Kristin Luck observes in her research on next-generation audience modeling, "Demographics may tell you who customers are, but behavior reveals what they actually care about—and in most cases, behavior is the superior predictor of future actions."
1. Integrate Behavior, Value, and Needs Analysis
The most sophisticated segmentation approaches combine multiple dimensions for comprehensive understanding.
a) Behavioral Pattern Identification
Forward-thinking organizations now prioritize behavioral signals in segmentation:
- Transaction sequence analysis frameworks
- Content consumption pattern mapping
- Feature and functionality usage clustering
- Engagement rhythm and frequency profiling
Example: Streaming service Spotify developed "Taste Profiles" that segment users primarily by listening patterns rather than age or location. This approach revealed behavior-based segments like "Discovery Seekers" and "Nostalgia Listeners" that cut across demographics, allowing for recommendations that achieved 37% higher engagement than demographic-based approaches.
b) Value-Based Customer Tiering
Segmenting based on economic relationship characteristics:
- Customer lifetime value prediction models
- Future potential value assessment frameworks
- Investment efficiency scoring methodologies
- Resource allocation optimization approaches
Example: Telecommunications provider T-Mobile implemented "Value Horizon Segmentation" that looks beyond current revenue to assess future potential value. This approach identified previously overlooked high-potential segments among younger customers with limited current spending but strong growth trajectories, resulting in 31% better resource allocation efficiency.
c) Needs-Based Contextual Analysis
Identifying underlying needs that drive purchase decisions:
- Job-to-be-done identification frameworks
- Problem resolution pattern analysis
- Goal achievement approach clustering
- Outcome prioritization assessment
Example: Healthcare provider Kaiser Permanente developed "Health Journey Segments" that group patients by health management needs rather than conditions or demographics. This approach revealed that a 35-year-old with diabetes and a 70-year-old with heart disease might share the same fundamental need for simplified medication management, enabling solution development that achieved 42% higher adoption rates.
2. Leverage Customer Data Platforms Effectively
Beyond conceptual frameworks, organizations need technical infrastructure for multidimensional segmentation.
a) Unified Data Architecture Development
Building technology infrastructure that enables integrated understanding:
- Cross-channel data integration frameworks
- Identity resolution system implementation
- Attribute unification methodologies
- Progressive profile development approaches
Example: Retail conglomerate Target implemented a comprehensive Customer Data Platform that unifies transaction data, digital behavior, loyalty program activity, and customer service interactions. This unified view enabled the identification of previously invisible segments like "Seasonal Gift Givers" who make high-value purchases infrequently but predictably, resulting in 28% higher marketing efficiency for these segments.
b) Advanced Analytics Implementation
Applying sophisticated analysis to reveal meaningful patterns:
- Machine learning pattern detection approaches
- Unsupervised clustering methodology application
- Predictive modeling for segment identification
- Natural language processing for unstructured data insights
Example: Insurance company Progressive developed "Behavioral Risk Segments" using machine learning algorithms that analyze driving patterns from telematics devices. This approach identified risk segments based on actual driving behavior rather than demographic proxies, resulting in 33% more accurate pricing and 26% improved customer retention.
c) Dynamic Segmentation Systems
Creating frameworks that adapt to changing behaviors and needs:
- Real-time segment assignment methodologies
- Behavioral shift detection systems
- Life stage transition identification
- Context-aware segment application
Example: Financial services company Capital One implemented "Financial Journey Segmentation" that dynamically reassigns customers based on changing financial behaviors. This approach automatically adjusted messaging and offers when customers showed signs of life changes like home buying or retirement planning, resulting in 39% higher response rates to relevant offers.
3. Create Unified Audience Views Across the Organization
For maximum impact, multidimensional segments must be operationalized enterprise-wide.
a) Cross-Functional Segment Activation
Enabling segment utilization across organizational functions:
- Marketing campaign segment application frameworks
- Product development segment utilization approaches
- Customer service segment-based experience design
- Sales interaction segment guidance systems
Example: Software company Adobe created a "Segment Center of Excellence" that ensures consistent application of multidimensional segments across marketing, product, sales, and support functions. This unified approach has driven 29% improvement in cross-sell success rates and significantly higher feature adoption by ensuring all customer touchpoints align with segment-specific needs.
b) Segment Storytelling and Socialization
Building organizational understanding and adoption:
- Segment persona narrative development
- Cross-departmental segment education programs
- Segment-specific insight distribution systems
- Use case and application scenario creation
Example: Hospitality company Marriott developed an immersive "Guest Segment Immersion Program" that brings their multidimensional traveler segments to life through storytelling, data visualization, and experiential learning for employees. This program increased segment-based decision making by 41% across the organization.
c) Segment-Based Decision Frameworks
Creating systematic approaches to segment-informed choices:
- Strategic planning segment application methodologies
- Resource allocation segment-based prioritization
- Innovation roadmap segment alignment
- Market expansion segment opportunity assessment
Example: Consumer packaged goods company Unilever implemented "Need-State Decision Frameworks" that require all new product development initiatives to explicitly target specific need-based segments rather than demographic groups. This approach has been credited with 36% higher new product success rates and significantly improved portfolio efficiency.
Conclusion: The Multidimensional Future of Customer Understanding
As noted by segmentation researcher Byron Sharp in his work on how brands grow, "The most effective marketing isn't based on who customers are—it's based on understanding the patterns in what they actually do." For marketing leaders, this insight suggests that moving beyond demographic shortcuts to multidimensional understanding may be the key to truly effective customer engagement.
The implementation of behavior, value, and needs-based segmentation represents more than just analytical improvement—it fundamentally transforms how organizations understand their audiences, enabling experiences that resonate at a deeper level because they address actual behaviors and needs rather than assumed characteristics.
As these approaches mature, organizations will increasingly focus not just on demographic identifiers but on the complex interplay of behaviors, values, and needs that truly drive customer decisions, creating unprecedented opportunities for relevance, resonance, and relationships built on genuine understanding.
Call to Action
For marketing leaders looking to implement multidimensional segmentation strategies:
- Develop research methodologies that prioritize behavior observation over identity attributes
- Invest in unified data infrastructure that enables cross-channel behavioral understanding
- Create cross-functional teams to ensure segment application across the organization
- Build measurement systems that evaluate segment predictive power and business impact
- Experiment with machine learning approaches to identify non-intuitive segment patterns
The future of marketing belongs not to those who categorize customers by who they are, but to those who understand them through the lens of what they do, what they value, and what they need—creating connections based on genuine understanding rather than demographic assumptions.
Featured Blogs
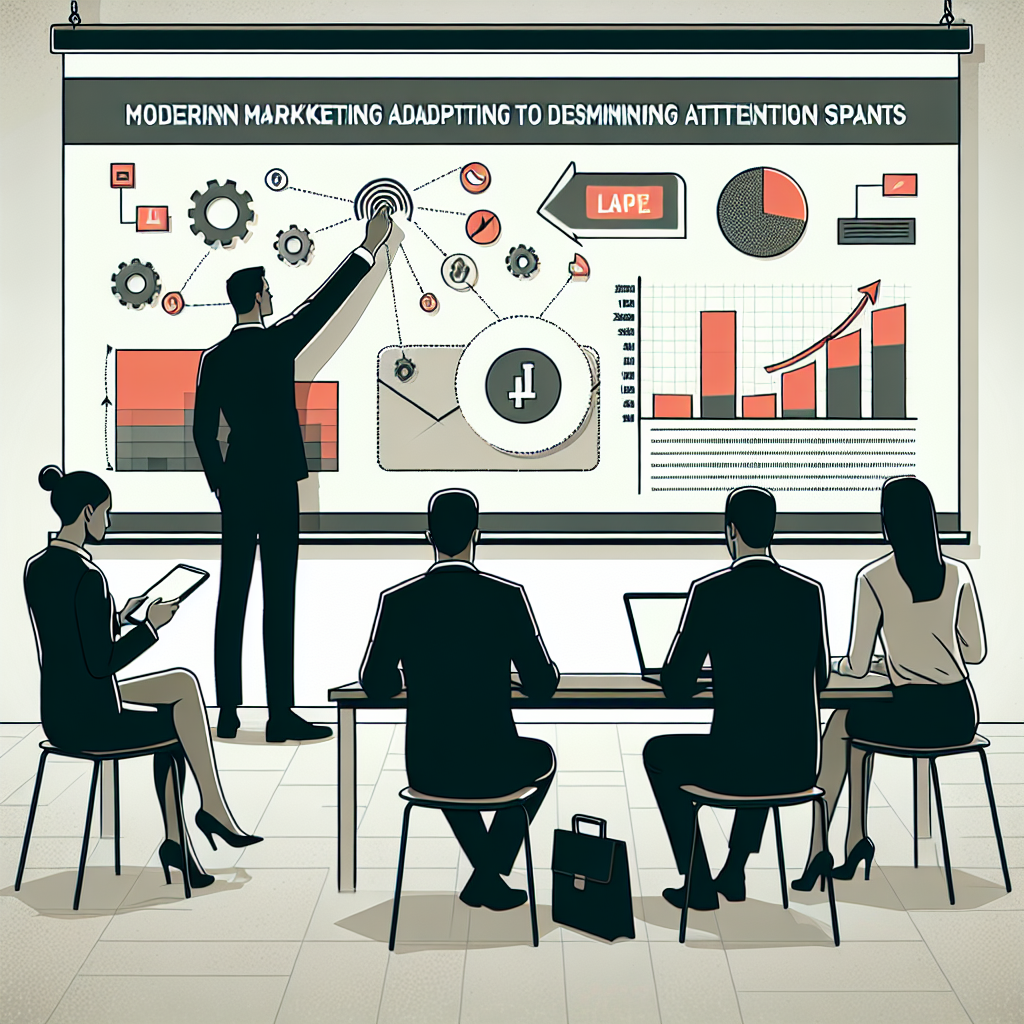
How the Attention Recession Is Changing Marketing
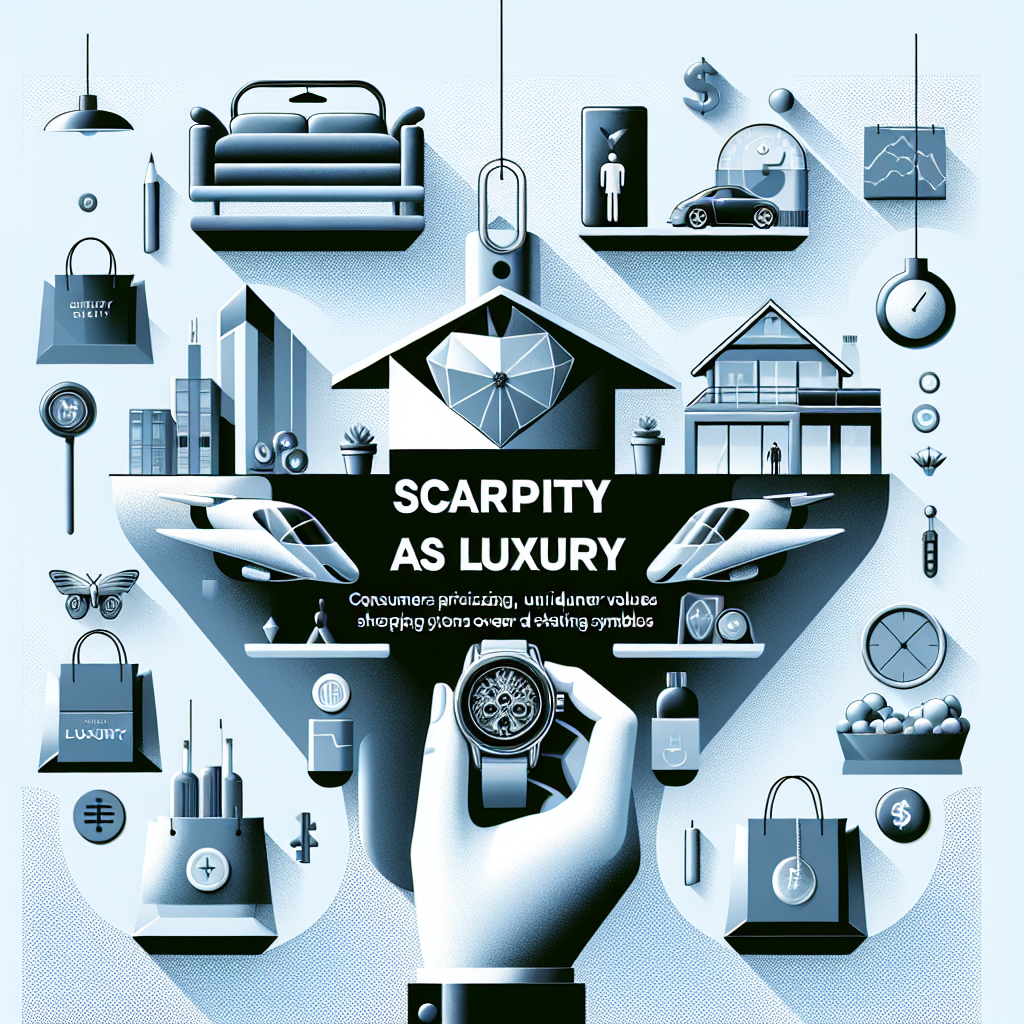
The New Luxury Why Consumers Now Value Scarcity Over Status
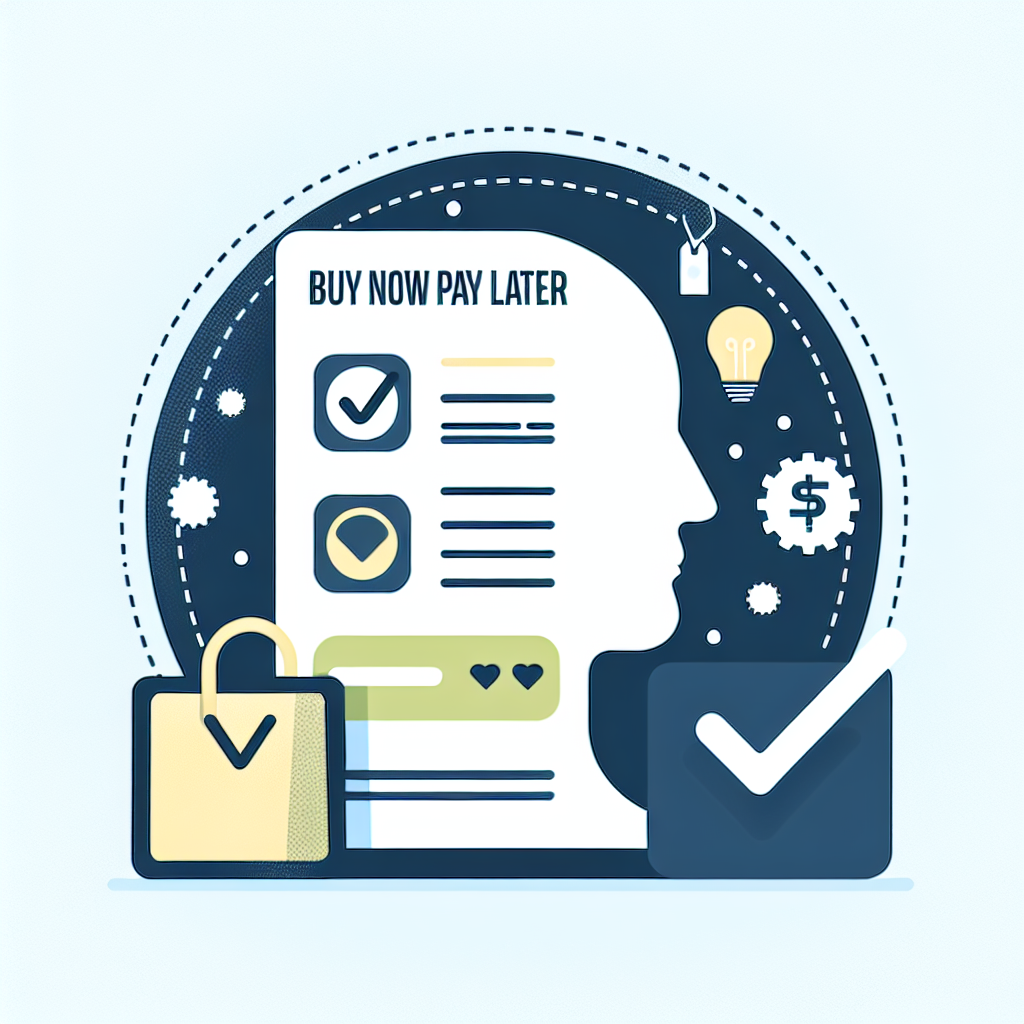
The Psychology Behind Buy Now Pay later
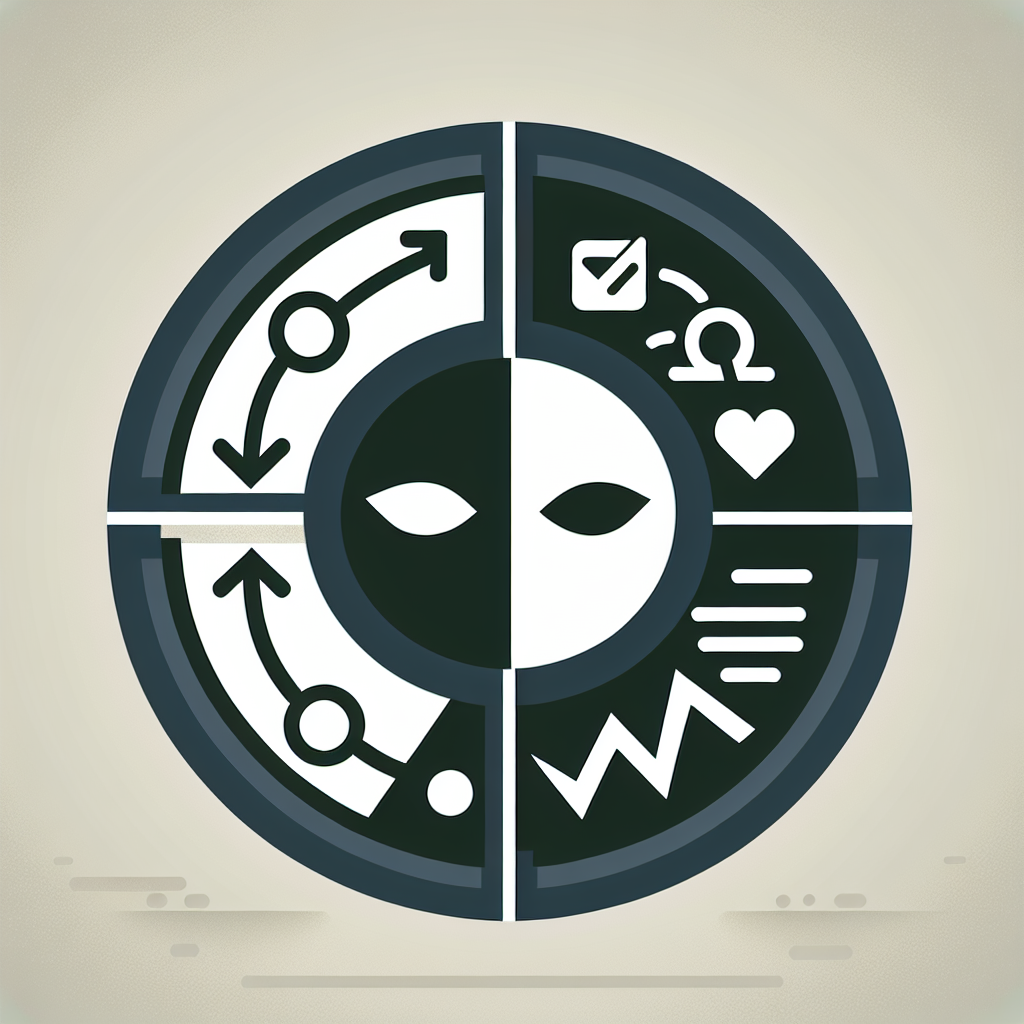
The Role of Dark Patterns in Digital Marketing and Ethical Concerns
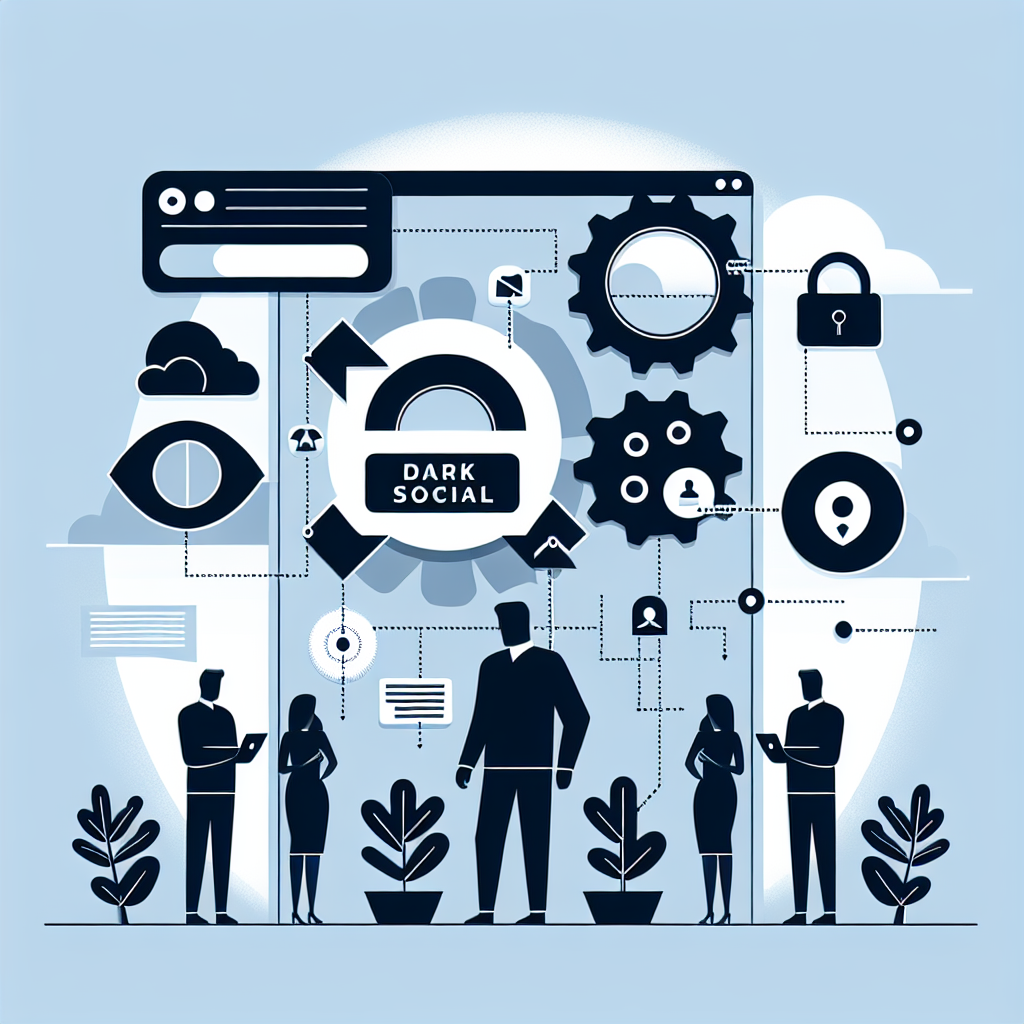
The Rise of Dark Social and Its Impact on Marketing Measurement
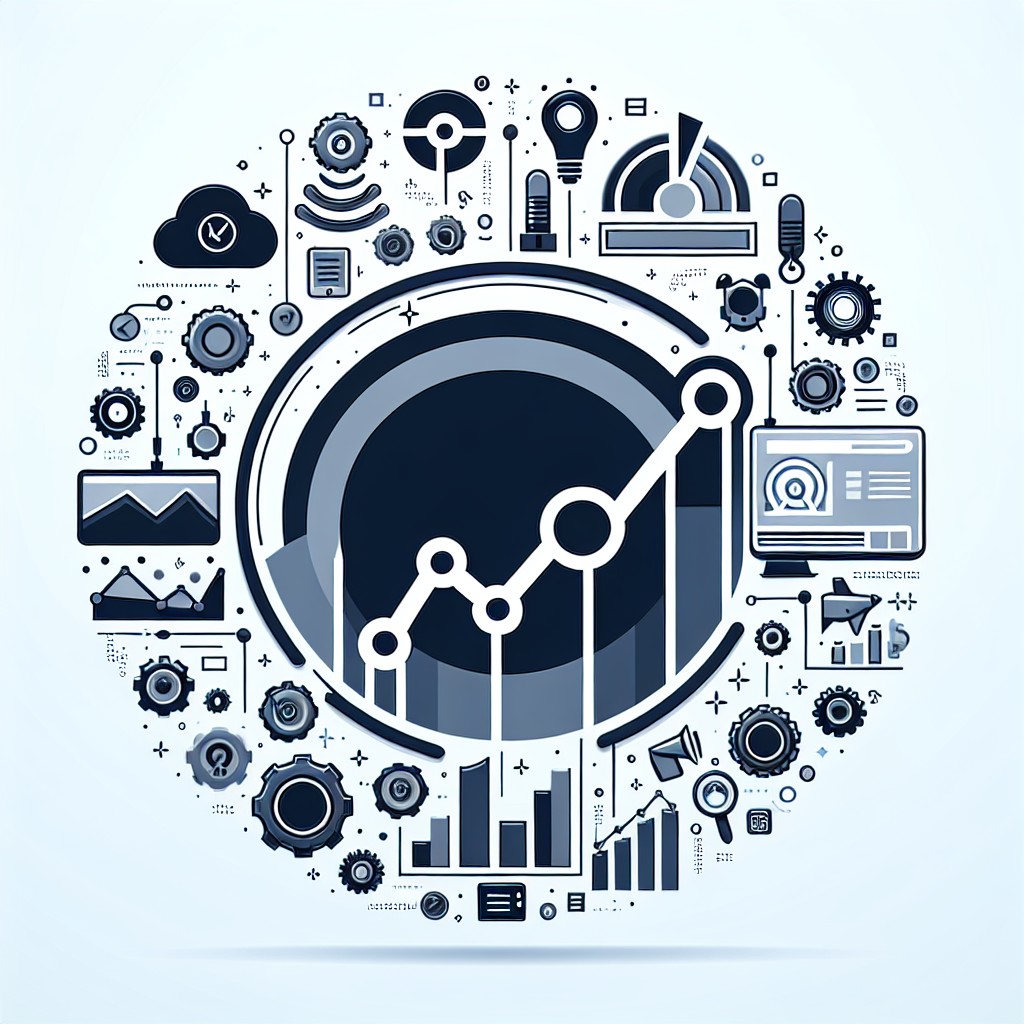