CRM as the Marketing Brain
The revelation occurred for Arun during a crucial campaign postmortem. Despite significant investment in creative assets and media spend, their latest product launch had yielded disappointing results. As they analyzed the data, a troubling pattern emerged—they had targeted thousands of customers with messaging about features they already owned, while neglecting others who perfectly matched their ideal customer profile. The disconnect traced back to a fundamental problem: their customer data was scattered across twelve different systems, creating a fragmented understanding that undermined every marketing decision. That evening, Arun drafted a proposal to reimagine their approach, positioning their CRM not as a mere sales tool but as the cognitive center of their entire marketing operation. Within six months of implementing this centralized intelligence model, their campaign performance metrics increased by 47%, while marketing costs decreased by 23%. This transformation fundamentally changed Arun's understanding of CRM—not as a contact database but as the organizational memory and intelligence engine driving every customer interaction. This realization launched his exploration into CRM-centered marketing, revealing how sophisticated customer data architecture has become the cornerstone of marketing effectiveness.
Introduction: The Cognitive Revolution in Customer Data Management
Customer Relationship Management has evolved from transactional record-keeping to sophisticated intelligence systems that serve as the cognitive foundation of modern marketing organizations. This evolution has progressed through several distinct phases: from contact databases to interaction repositories, from siloed sales tools to integrated customer platforms, and now to the frontier of intelligence systems that generate insights, predictions, and recommendations that drive marketing strategy and execution.
The development of CRM as a marketing intelligence center represents what McKinsey has identified as "a critical strategic asset" for companies seeking competitive advantage. In high-performing organizations, CRM now serves not just as a system of record but as the central nervous system connecting all customer-facing functions.
Research from Forrester indicates that organizations with marketing-integrated CRM systems achieve 71% higher customer retention rates and 59% greater marketing ROI compared to those using CRM primarily for sales. Meanwhile, a study published in the Journal of Database Marketing found that companies with unified customer data architectures demonstrate 3.6x faster time-to-insight and 2.7x greater ability to identify emerging customer trends.
1. Unified Customer Profiles: Creating a Single Source of Truth
Modern CRM systems create comprehensive customer understanding:
a) Identity Resolution Frameworks
Sophisticated systems unify fragmented identities:
- Cross-device identity matching
- Anonymous-to-known user reconciliation
- Household and organizational hierarchy mapping
- Persistent identification methodologies
Example: Financial services leader American Express implemented an identity resolution framework within their CRM that connects an average of 7.2 different customer identifiers per cardholder. This system creates what they call "persistent identity graphs" that maintain continuity despite changing contact information, devices, or relationship status. This capability increased their cross-channel matching accuracy by 63% and marketing personalization effectiveness by 41%.
b) Behavioral History Integration
Comprehensive profiles include full interaction timelines:
- Cross-channel engagement unification
- Product usage behavior integration
- Service interaction consolidation
- Latent interest signal collection
Example: Software company Adobe incorporated product usage behavior directly into their CRM profiles, creating what they call "experience timelines" that show not just marketing interactions but product feature adoption patterns. This integration revealed that customers following specific feature adoption sequences had 3.1x higher lifetime value, allowing for targeted intervention with users showing suboptimal usage patterns.
c) Relationship Context Management
Strategic CRM models capture relationship dimensions:
- Multi-product relationship mapping
- Service history integration
- Sentiment trend analysis
- Influence network visualization
Example: Telecommunications provider Verizon implemented relationship strength indicators within their CRM that combine tenure, service history, and recent interaction sentiment. These indicators drive different communication approaches, resulting in a 37% increase in retention for previously identified at-risk customers and a 29% higher cross-sell acceptance for promoters.
2. Data Enrichment: Expanding Customer Understanding
Enhanced profiles create deeper customer insight:
a) First-Party Data Activation
Organizations maximize owned data assets:
- Cross-departmental data integration
- Historical interaction analysis
- Propensity model development
- Behavioral pattern recognition
Example: Retail giant Walmart unified point-of-sale, e-commerce, pharmacy, and financial services data into their customer profiles, creating what their CMO calls "commercial relationship context." This enrichment revealed previously hidden pattern correlations—such as seasonal health purchases predicting specific grocery needs—enabling anticipatory marketing that increased basket size by 23% for targeted customers.
b) Third-Party Intelligence Integration
External data amplifies internal understanding:
- Demographic data incorporation
- Firmographic enhancement
- Intent signal integration
- Life event identification
Example: Real estate platform Zillow integrated third-party life event data into their CRM, allowing them to identify customers experiencing marriage, relocation, or family expansion before explicit home search behavior begins. This predictive enrichment allows earlier engagement, resulting in 41% higher conversion rates compared to waiting for direct property search signals.
c) AI-Powered Insight Generation
Advanced analytics transform data into intelligence:
- Predictive behavior modeling
- Next-best-action recommendations
- Lifetime value forecasting
- Churn risk identification
Example: Streaming service Spotify implemented an AI system that analyzes listening patterns to identify "content fatigue" indicators three weeks before users typically reduce engagement. These early warning signals trigger personalized discovery campaigns that have reduced churn by 32% among detected at-risk subscribers.
3. Campaign Segmentation: Translating Insight into Action
Strategic segmentation creates actionable targeting:
a) Multi-dimensional Segmentation Frameworks
Sophisticated approaches combine multiple factors:
- Behavioral recency-frequency-monetary value models
- Lifecycle stage classification systems
- Need-based segmentation frameworks
- Engagement intensity scoring
Example: Hotel chain Marriott implemented a multi-dimensional segmentation model combining travel frequency, booking patterns, and loyalty status with property preference indicators. This framework identified a previously unrecognized segment of "location-loyal occasional travelers" who visit the same destination infrequently but consistently, enabling targeted marketing that increased repeat bookings by 34% for this segment.
b) Dynamic Audience Management
Modern systems create fluid, real-time audiences:
- Behavior-triggered segment transitions
- Progressive qualification frameworks
- Engagement-based audience movement
- Interest intensity escalation models
Example: Investment firm Fidelity created dynamic audience frameworks that automatically move customers between segments based on detected financial life stages and engagement patterns. This approach replaced static demographic segments with behavior-based groupings, increasing campaign relevance scores by 47% and conversion rates by 38%.
c) Propensity-Based Prioritization
Advanced targeting incorporates likelihood models:
- Conversion probability scoring
- Response propensity modeling
- Channel receptivity prediction
- Offer sensitivity calculation
Example: Insurance provider Progressive implemented a response propensity model that predicts not just which customers are likely to need particular coverage, but also their probability of responding to specific message types and channels. This capability allowed them to prioritize outreach to high-response-probability segments first, increasing overall campaign efficiency by 43% while maintaining total conversion volume.
Conclusion: The Intelligence Imperative
As noted by customer data expert and former Gartner analyst Martin Kihn, "The most valuable marketing asset isn't creative or media or even brand—it’s the depth and accuracy of customer understanding." For marketing leaders, this insight suggests that CRM strategy may now be more fundamental to marketing success than traditional creative or channel capabilities.
The transformation of CRM into a marketing intelligence center represents more than technical evolution—it requires a fundamental shift in how organizations conceive of customer data. Those who master this discipline create significant competitive advantage through deeper customer understanding, more relevant engagement, and more efficient resource allocation.
As these technologies continue to evolve, successful organizations will be those that maintain a balance between algorithmic intelligence and human insight, creating systems that augment rather than replace the marketer's judgment.
Call to Action
For marketing leaders seeking to develop CRM as their marketing brain:
- Conduct an audit of customer data fragmentation across the organization
- Develop a unified customer data architecture that prioritizes marketing use cases
- Create governance frameworks that maintain data quality and privacy compliance
- Build cross-functional teams that bridge marketing, IT, and data science
- Implement hypothesis testing approaches that continuously refine customer understanding
The future of marketing effectiveness belongs not to those with the largest budgets or the most creative campaigns, but to those who build the most comprehensive, accurate understanding of their customers and embed that intelligence into every marketing decision and customer interaction.
Featured Blogs
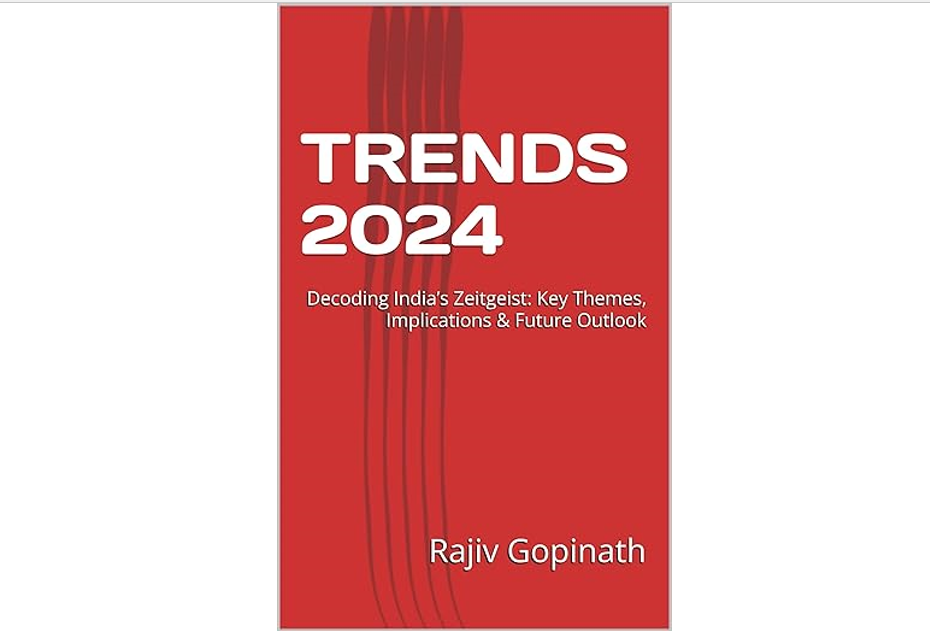
TRENDS 2024: Decoding India’s Zeitgeist: Key Themes, Implications & Future Outlook
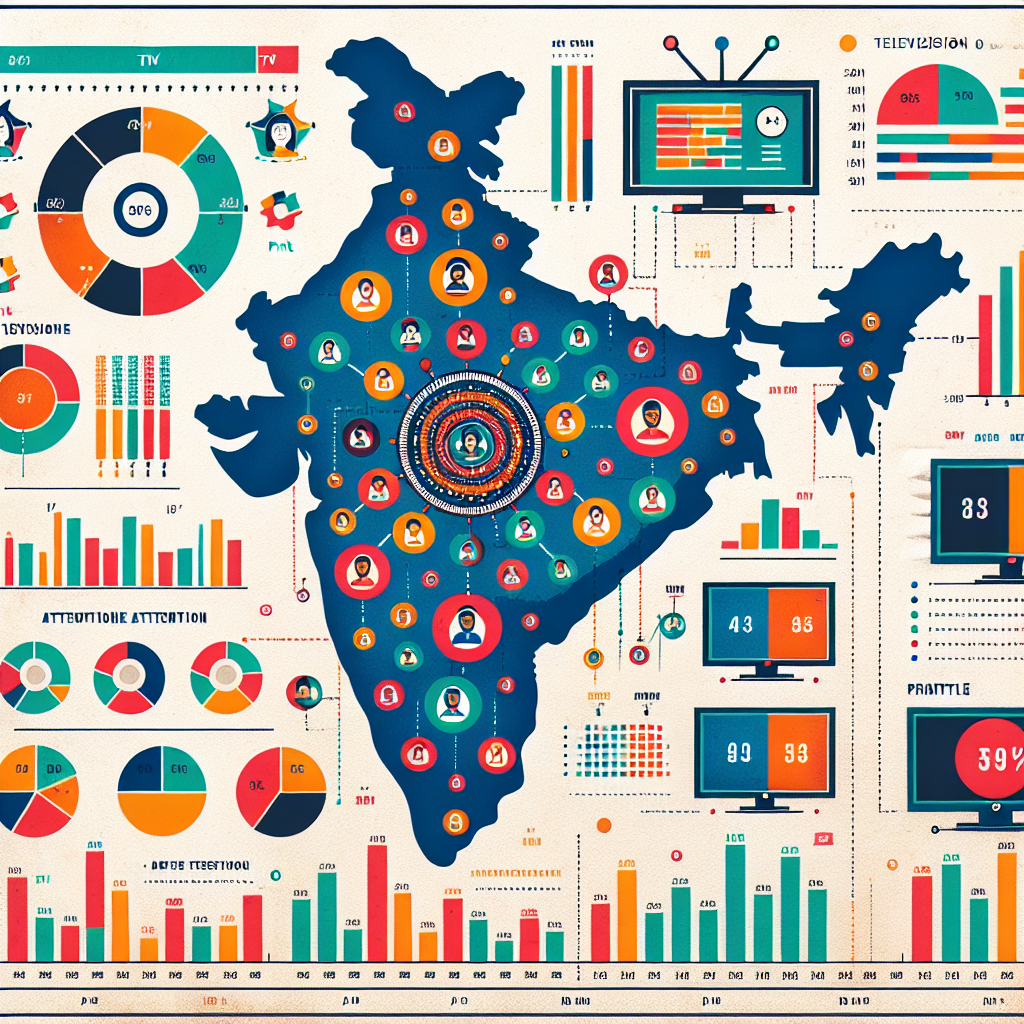
How to better quantify attention in TV and Print in India
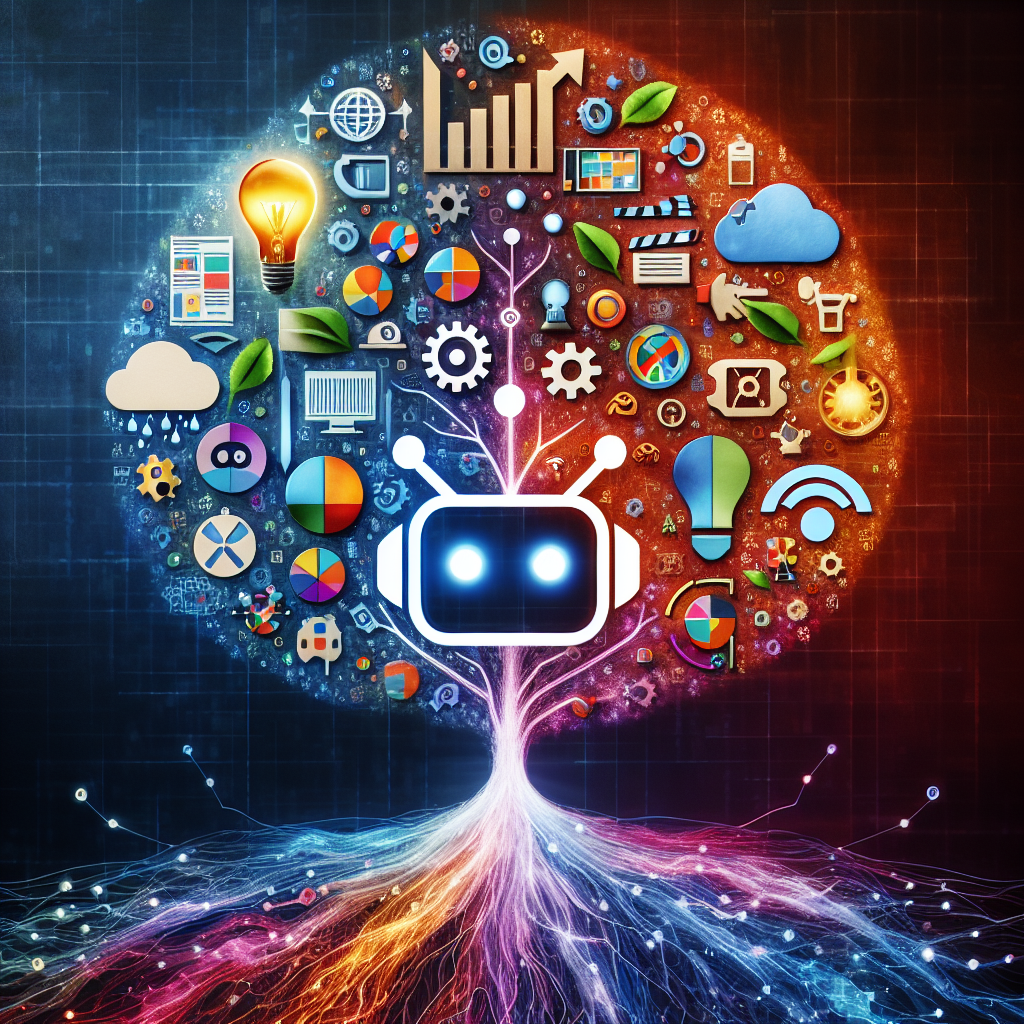
AI in media agencies: Transforming data into actionable insights for strategic growth
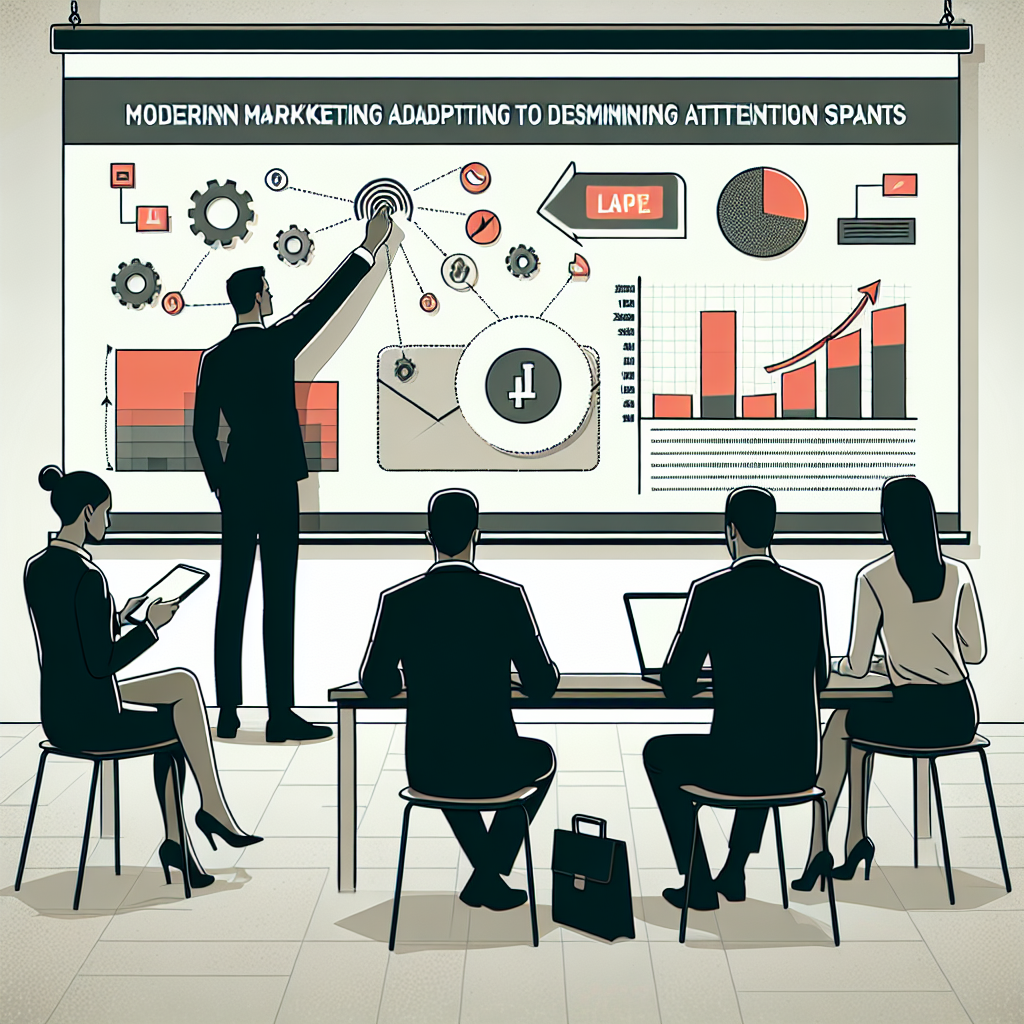
How the Attention Recession Is Changing Marketing
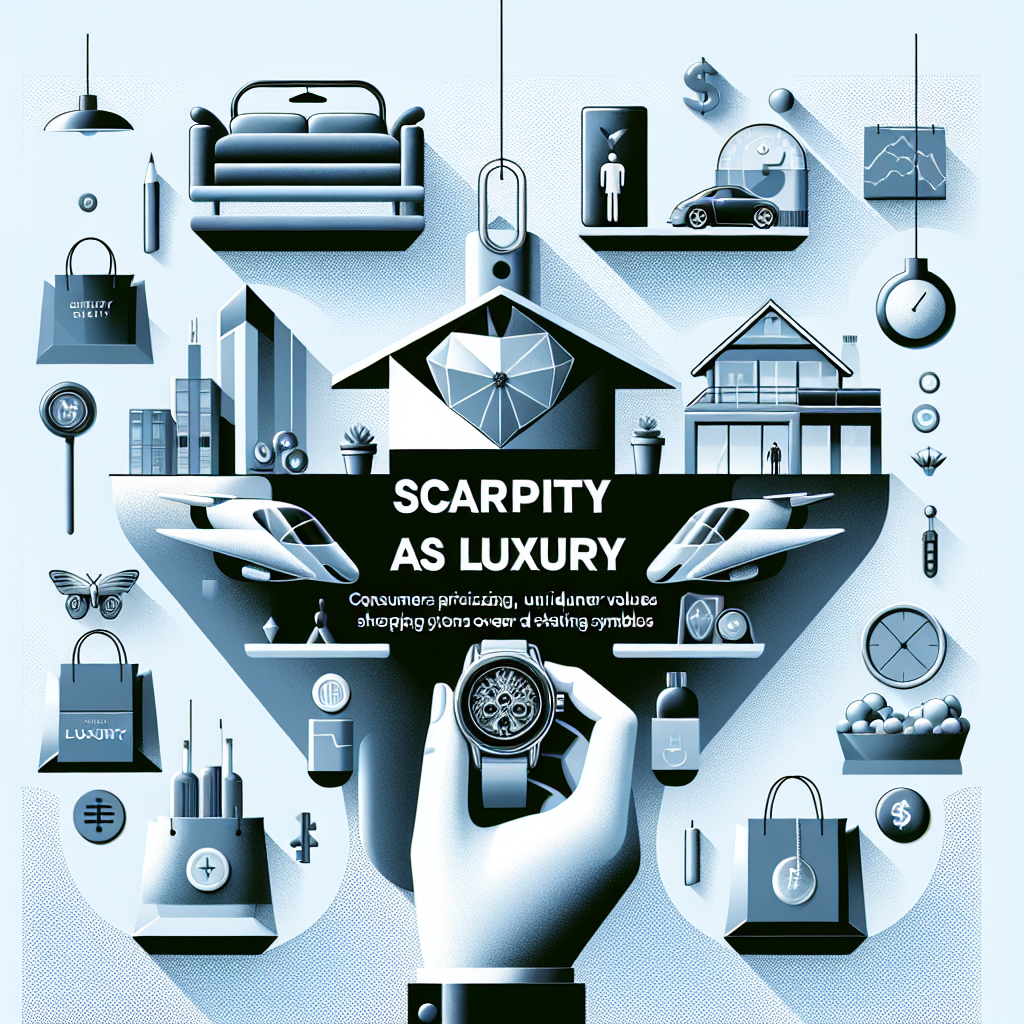
The New Luxury Why Consumers Now Value Scarcity Over Status
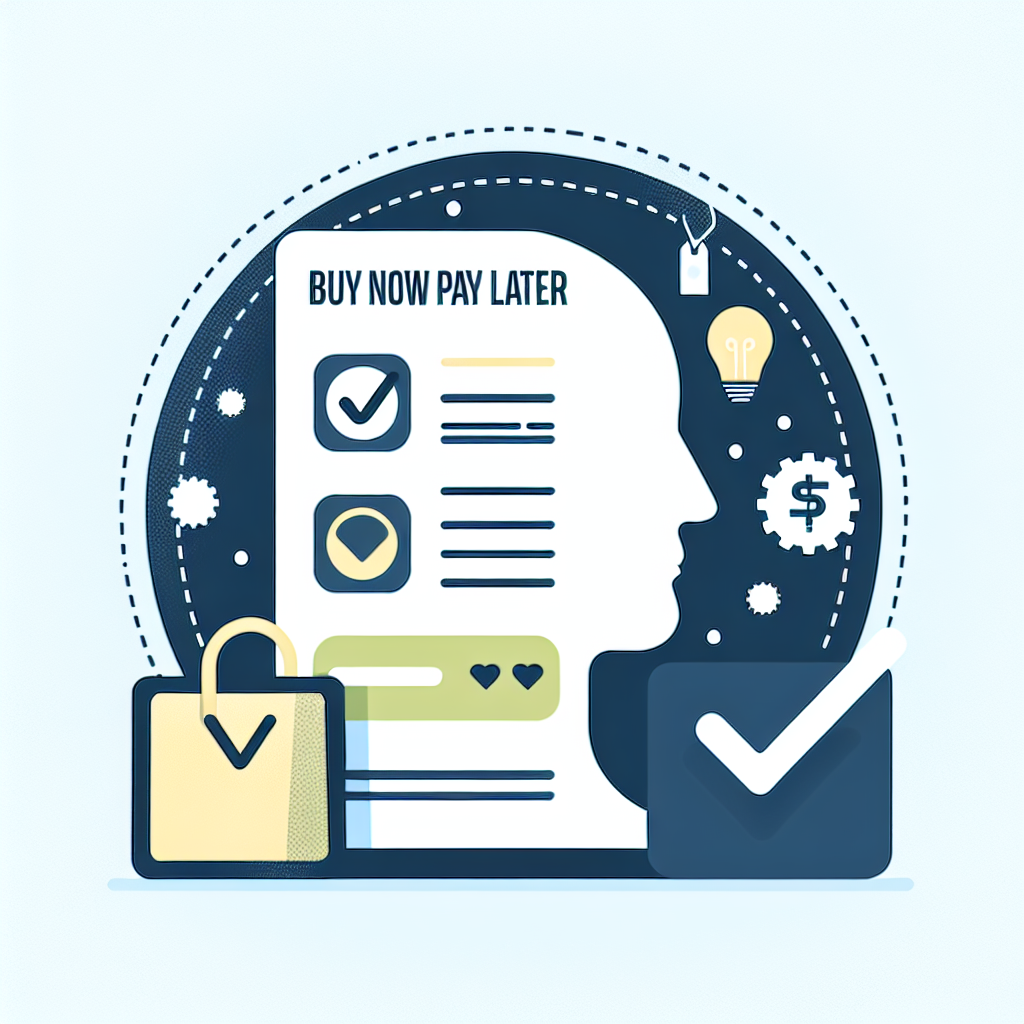
The Psychology Behind Buy Now Pay later
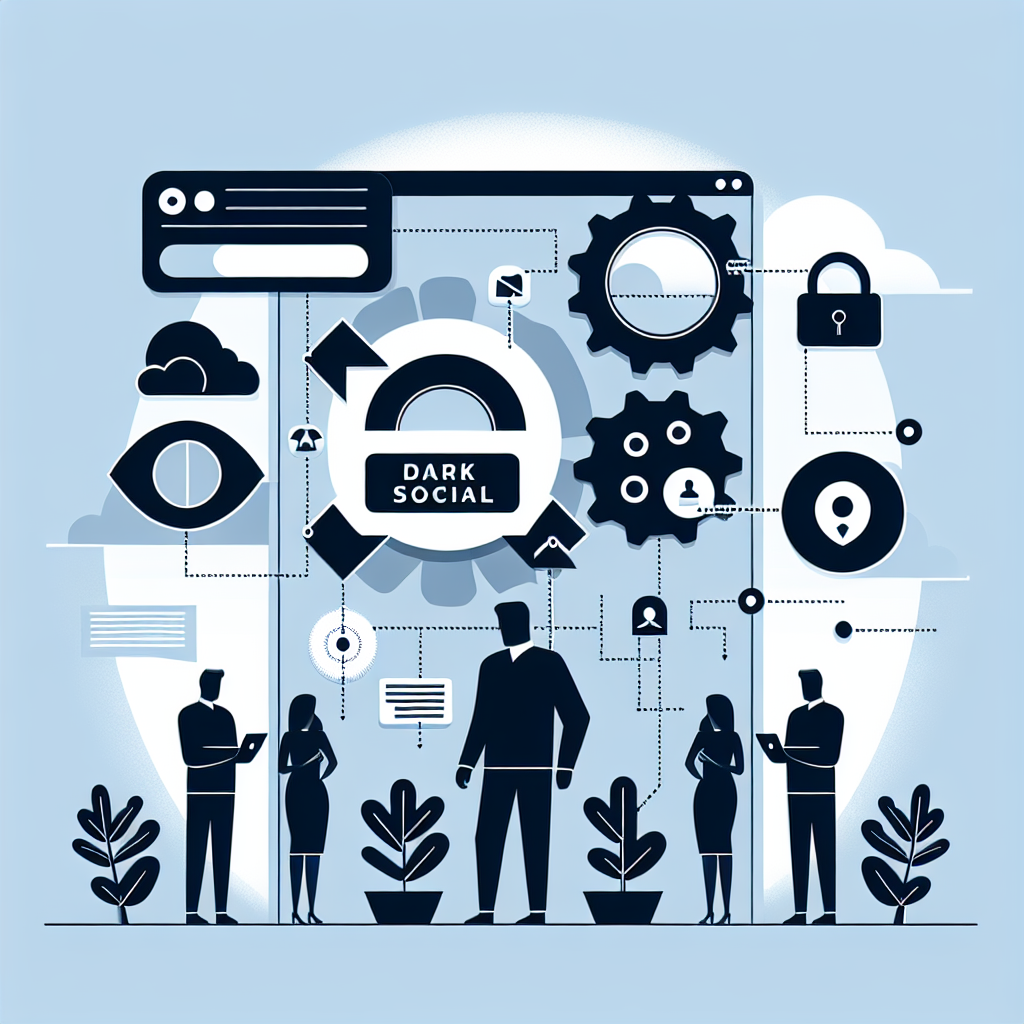
The Rise of Dark Social and Its Impact on Marketing Measurement
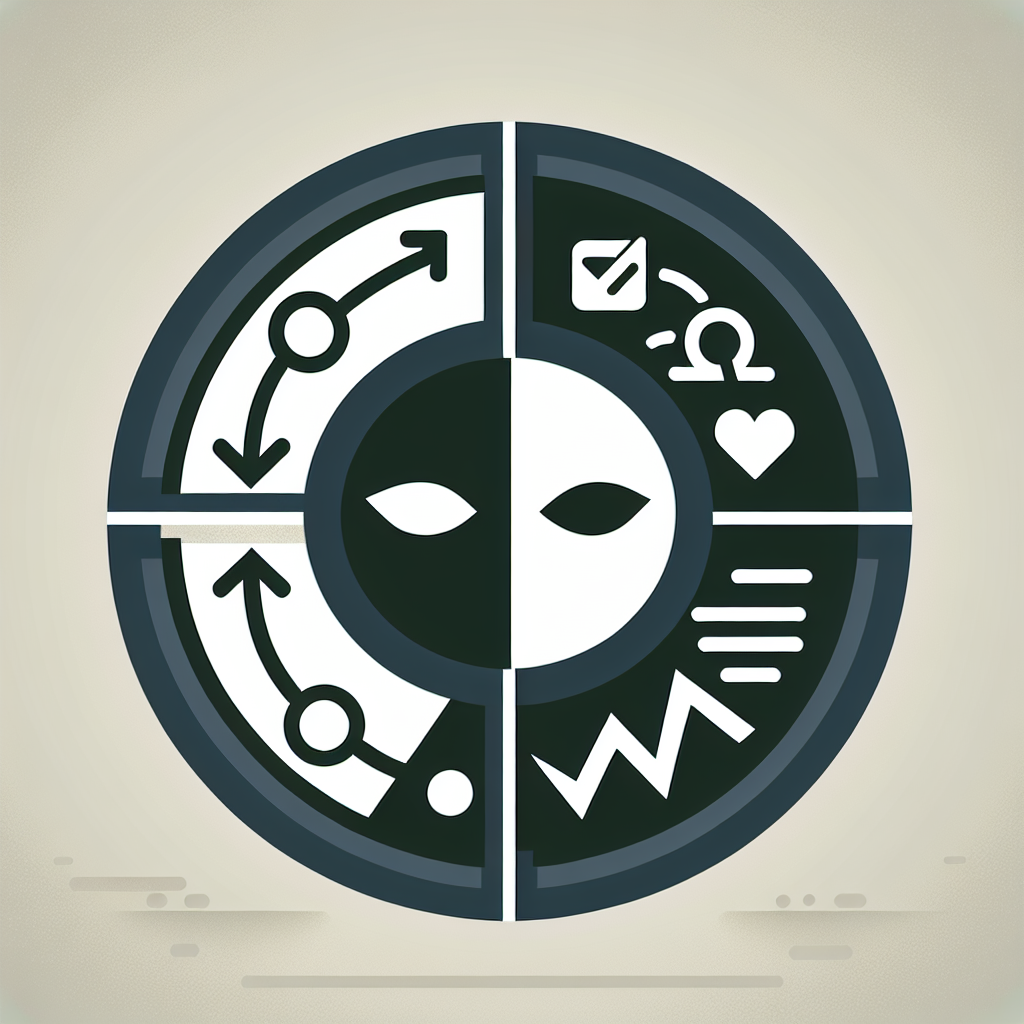
The Role of Dark Patterns in Digital Marketing and Ethical Concerns
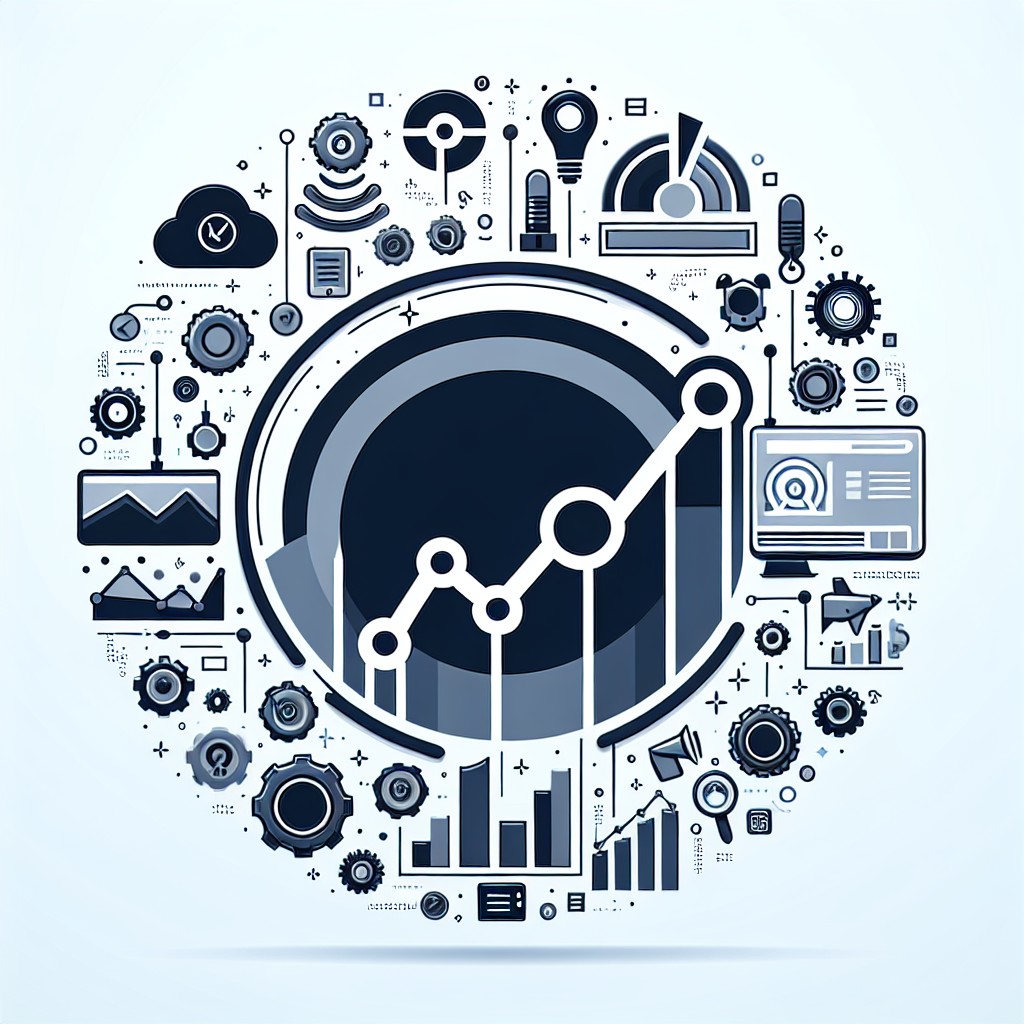