How Privacy First Marketing is Changing the Rules of Attribution
During a quarterly marketing review meeting last year, Ray and his team were puzzled by contradictory data. Their attribution model showed declining performance across digital channels, yet overall sales were up significantly. Upon deeper investigation, they discovered that over 40% of customer journeys had become "dark" due to privacy changes—invisible to tracking systems yet clearly contributing to business outcomes. This disconnect between measured performance and actual results sparked Ray’s fascination with how privacy regulations were fundamentally reshaping marketing measurement. He began investigating how forward-thinking brands were adapting their attribution approaches for this new reality, and what he discovered was nothing short of a measurement revolution.
Introduction: The Attribution Crisis
For over a decade, digital marketers enjoyed unprecedented visibility into customer journeys. Multi-touch attribution models promised to reveal exactly which touchpoints influenced purchases, allowing for precise optimization of marketing spend. This system rested on a technical foundation of cross-site tracking, third-party cookies, and device fingerprinting—technologies now being systematically dismantled by privacy regulations, browser restrictions, and changing consumer expectations.
The impact has been profound. According to research from the Interactive Advertising Bureau, over 60% of marketers report significant disruption to their measurement capabilities due to privacy changes. A Boston Consulting Group study found that traditional attribution models now miss between 30-40% of conversion paths, creating a growing "blind spot" in marketing analytics.
This attribution crisis represents both a challenge and an opportunity for marketers to rethink measurement fundamentals. As Avinash Kaushik, Google's Digital Marketing Evangelist, observes: "The privacy revolution isn't destroying measurement—it's forcing us to build more sustainable, consumer-respectful approaches to understanding marketing effectiveness."
1. The Renaissance of Marketing Mix Modeling
As granular attribution becomes less viable, marketers are rediscovering and reinventing marketing mix modeling (MMM)—a statistical approach that correlates marketing investments with business outcomes without requiring individual-level tracking.
Traditional MMM, developed decades ago for television advertising, used basic regression analysis to estimate channel impact. Today's advanced MMMs leverage machine learning, bayesian statistics, and automated time-series analysis to provide more nuanced insights. According to Forrester Research, investment in advanced MMM solutions has increased by 67% since 2020 as companies seek privacy-compliant measurement alternatives.
Nike provides an instructive case study. Following Apple's iOS 14.5 privacy changes, the company experienced a 30% reduction in attributable conversions from digital advertising. Rather than increasing tracking efforts, Nike shifted to an advanced MMM approach that incorporated first-party signals without compromising consumer privacy. This model revealed that the company's brand campaigns delivered 2.8x more value than previously recognized, while some performance channels were significantly overvalued by last-click attribution.
As Jim Lecinski, former VP at Google and marketing professor at Northwestern University, notes: "MMM isn't a step backward—it's an evolution toward measurement that respects consumer privacy while providing actionable business insights."
2. The Incrementality Imperative
Beyond modeling, leading organizations are embracing experimental approaches to measurement focused on incrementality—the true causal impact of marketing activities.
Facebook's Conversion Lift and Google's Brand Lift studies have long offered controlled experiments to measure incremental impact, but these tools typically operated within their respective platforms. The new challenge is conducting cross-channel incrementality measurement in a privacy-compliant way.
Telecommunications giant Vodafone pioneered a solution through what they call "geo-testing"—running different marketing strategies in matched geographical areas and measuring the differential business impact. This approach allowed them to identify that certain digital channels previously credited with driving conversions were actually capturing demand generated elsewhere. By reallocating budget based on incrementality rather than attribution, Vodafone increased marketing ROI by 20%.
Measurement expert Nancy Smith, CEO of Analytic Partners, describes this shift: "Attribution tells you who got credit. Incrementality tells you what actually worked. As privacy restrictions limit attribution, incrementality becomes the north star of effective measurement."
3. The First-Party Data Advantage
Organizations with robust first-party data ecosystems enjoy significant advantages in privacy-era measurement. By creating unified customer views across owned touchpoints, they can develop attribution insights without relying on third-party tracking.
Retail giant Target developed what they call "Guest ID" attribution—a measurement system linking online and offline behaviors through their loyalty program. This approach allowed them to maintain 85% visibility into customer journeys despite cookie restrictions. The key insight was focusing measurement on known customers who had explicitly provided consent for data usage.
This shift toward first-party measurement requires significant technical and organizational changes. According to research from Winterberry Group, companies that successfully implement first-party measurement strategies invest an average of 1.2% of marketing budgets in data infrastructure—more than double the industry average.
As Tina Moffett, Principal Analyst at Forrester, observes: "First-party data isn't just a privacy compliance tool—it's the foundation of sustainable measurement in a world where third-party signals are increasingly restricted."
4. The Rise of Data Clean Rooms
To bridge the gap between privacy and measurement, a new category of technology has emerged: data clean rooms. These secure environments allow multiple parties to analyze overlapping data sets without exposing underlying customer information.
Google's Ads Data Hub, Amazon Marketing Cloud, and independent solutions like InfoSum enable companies to match their first-party data with platform data in privacy-compliant ways. Research from the IAB found that adoption of clean room technologies increased by 173% between 2021 and 2023, reflecting their growing importance in privacy-era measurement.
Unilever utilized clean room technology to analyze campaign effectiveness across multiple walled gardens without compromising consumer privacy. This approach revealed that their cross-platform campaigns were 37% more effective than platform-specific initiatives—an insight impossible to gain through traditional attribution.
"Clean rooms represent the future of cross-platform measurement," explains media measurement expert Jane Clarke. "They balance the legitimate business need for campaign insights with the ethical and regulatory requirements for privacy protection."
5. Probabilistic Attribution & Machine Learning
As deterministic tracking declines, probabilistic approaches based on statistical modeling and machine learning are gaining prominence. These systems don't track individuals but instead predict likely conversion paths based on aggregated behavioral patterns.
Cosmetics retailer Sephora developed a machine learning attribution system that identifies patterns in anonymized, aggregated data to predict conversion likelihood across touchpoints. This approach maintained attribution accuracy within 5% of previous models while eliminating reliance on third-party cookies.
AI measurement specialist Rex Briggs explains: "The future of attribution isn't perfect tracking—it's intelligent inference. By applying machine learning to consented, aggregated data, we can derive meaningful insights without comprehensive tracking."
Conclusion: From Precision to Intelligence
The privacy revolution in digital marketing doesn't signal the end of attribution but rather its evolution. As granular tracking becomes less viable, measurement is shifting from an engineering challenge ("how do we track everything?") to an intelligence challenge ("how do we derive meaningful insights from incomplete data?").
The most successful organizations in this new era will be those that balance multiple measurement approaches—experimental designs, advanced modeling, first-party analytics, and machine learning—to create holistic views of marketing effectiveness without compromising consumer privacy.
As marketing measurement pioneer Byron Sharp observes, "Perfect attribution was always an illusion. The privacy revolution isn't limiting our understanding—it's forcing us to acknowledge the complexity of marketing influence and develop more sophisticated approaches to measurement."
Call to Action
For marketing leaders navigating this measurement transformation, several imperatives emerge:
- Audit your measurement stack to identify privacy vulnerabilities and dependencies on deprecated tracking technologies
- Invest in complementary measurement approaches that balance attribution with incrementality testing and marketing mix modeling
- Develop first-party data infrastructure that enables owned attribution capabilities
- Explore clean room technologies that facilitate privacy-compliant data collaboration
- Train marketing teams on statistical thinking and experimental design to reduce dependency on automated attribution
By embracing these priorities, organizations can transform the privacy challenge from a measurement crisis into an opportunity to develop more sophisticated, sustainable approaches to understanding marketing effectiveness.
Featured Blogs
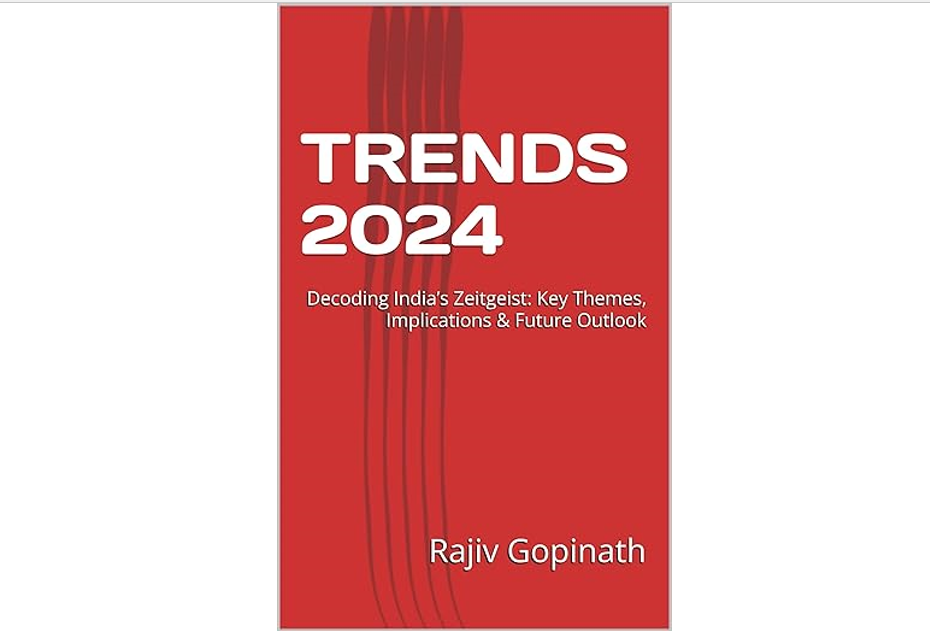
TRENDS 2024: Decoding India’s Zeitgeist: Key Themes, Implications & Future Outlook
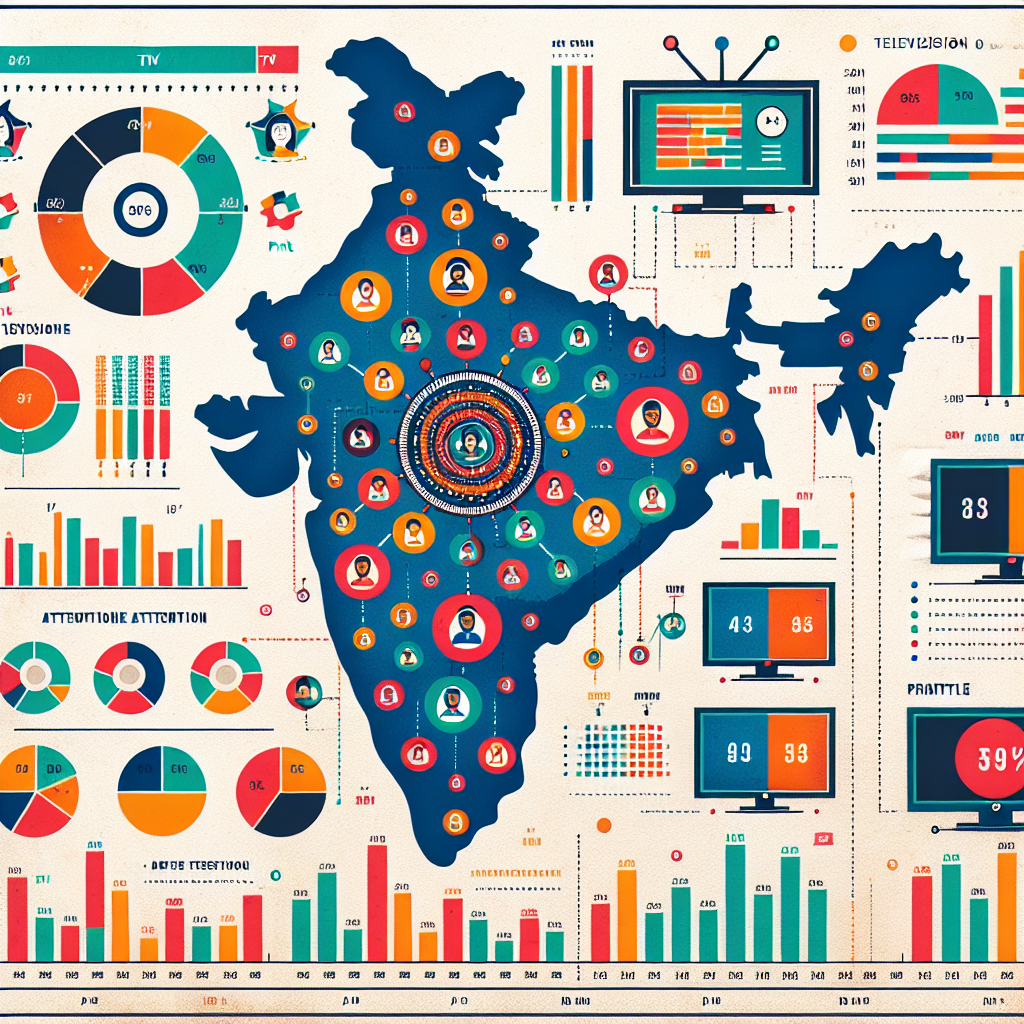
How to better quantify attention in TV and Print in India
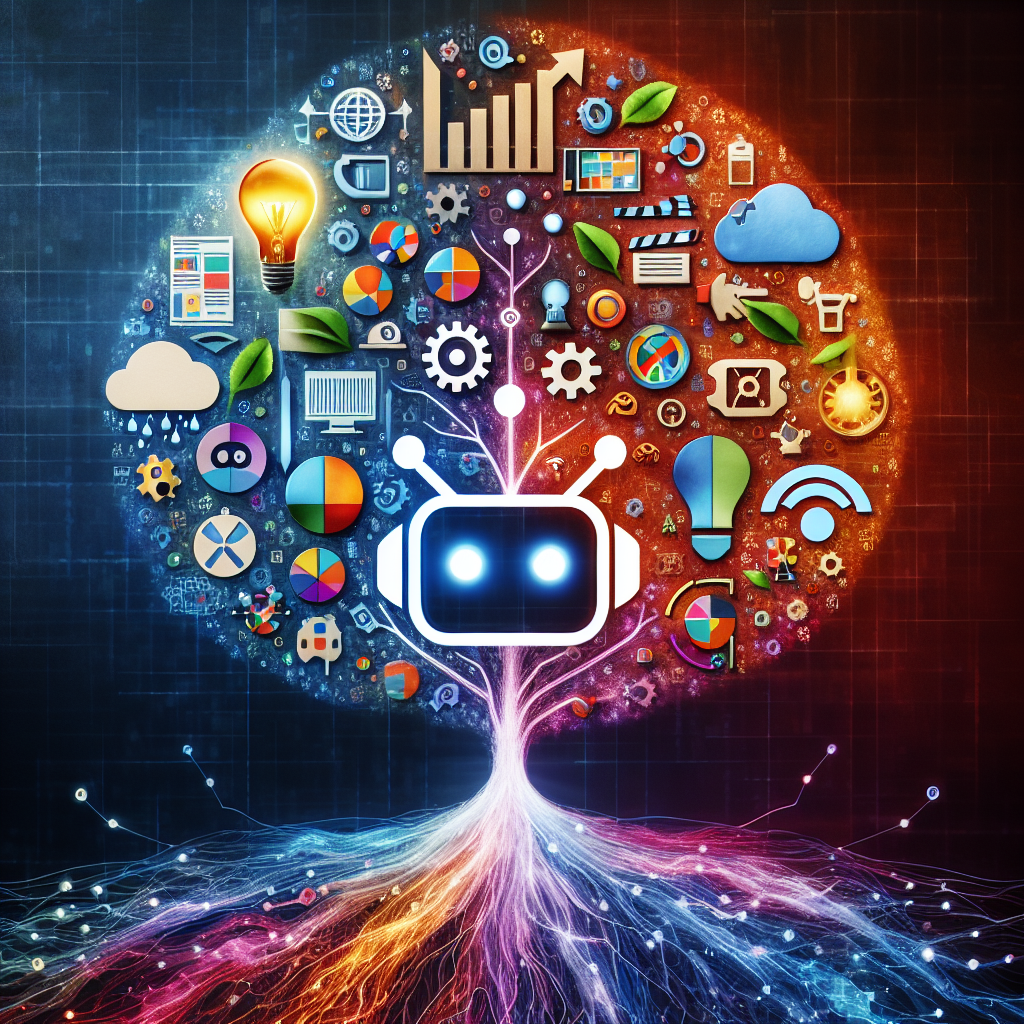
AI in media agencies: Transforming data into actionable insights for strategic growth
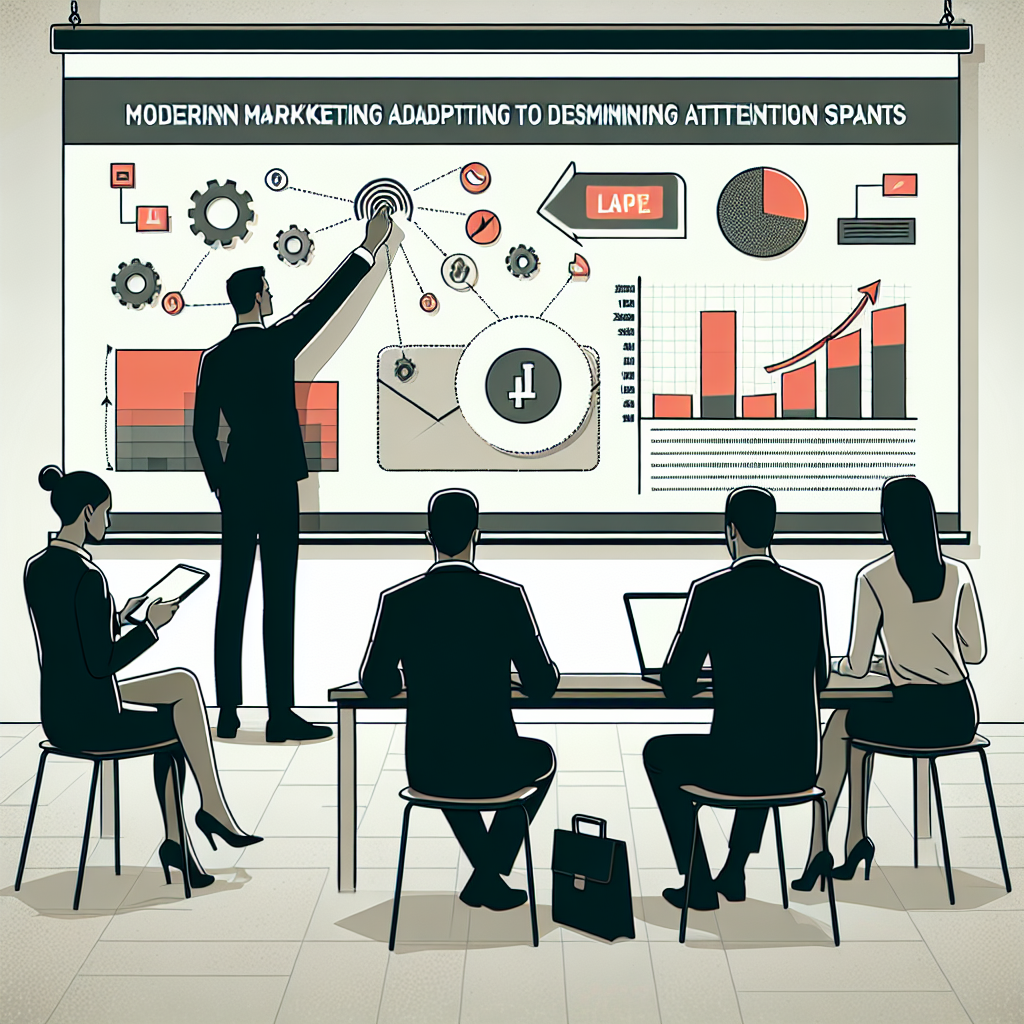
How the Attention Recession Is Changing Marketing
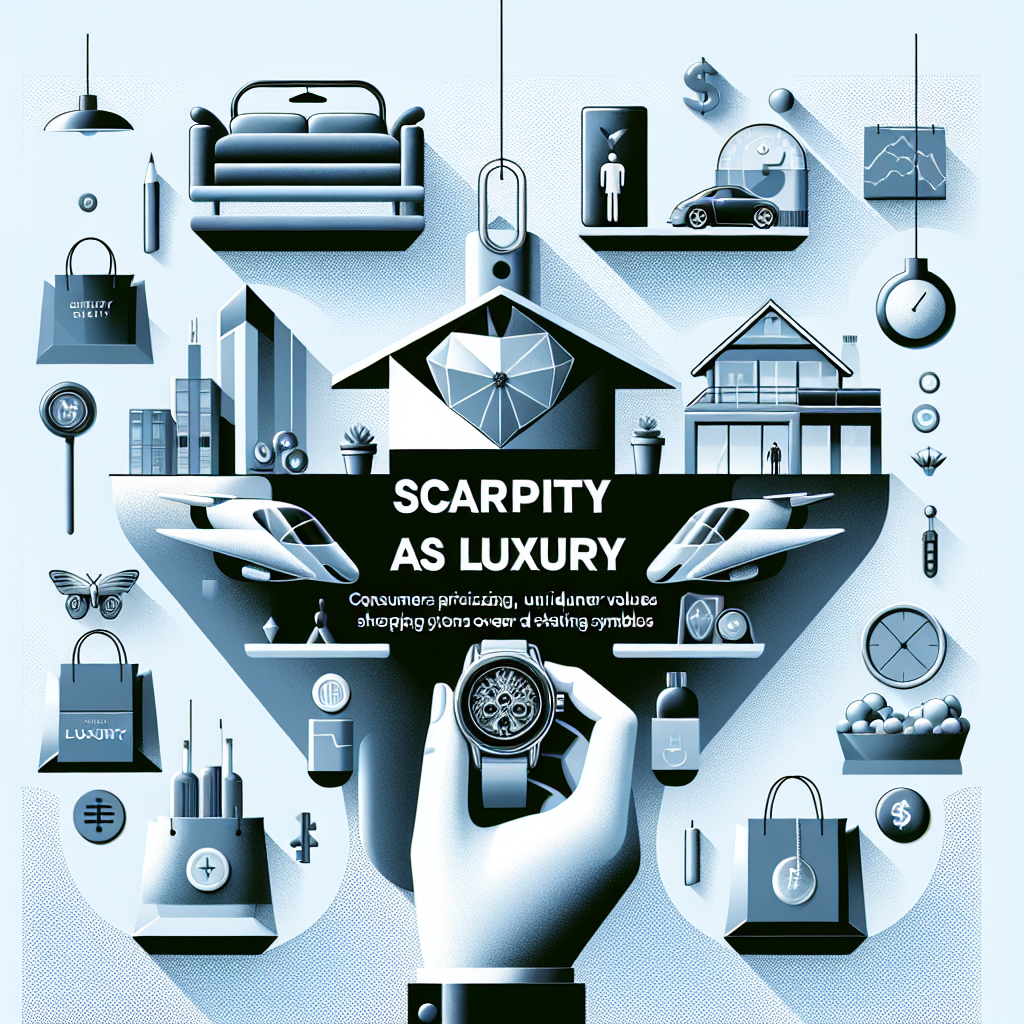
The New Luxury Why Consumers Now Value Scarcity Over Status
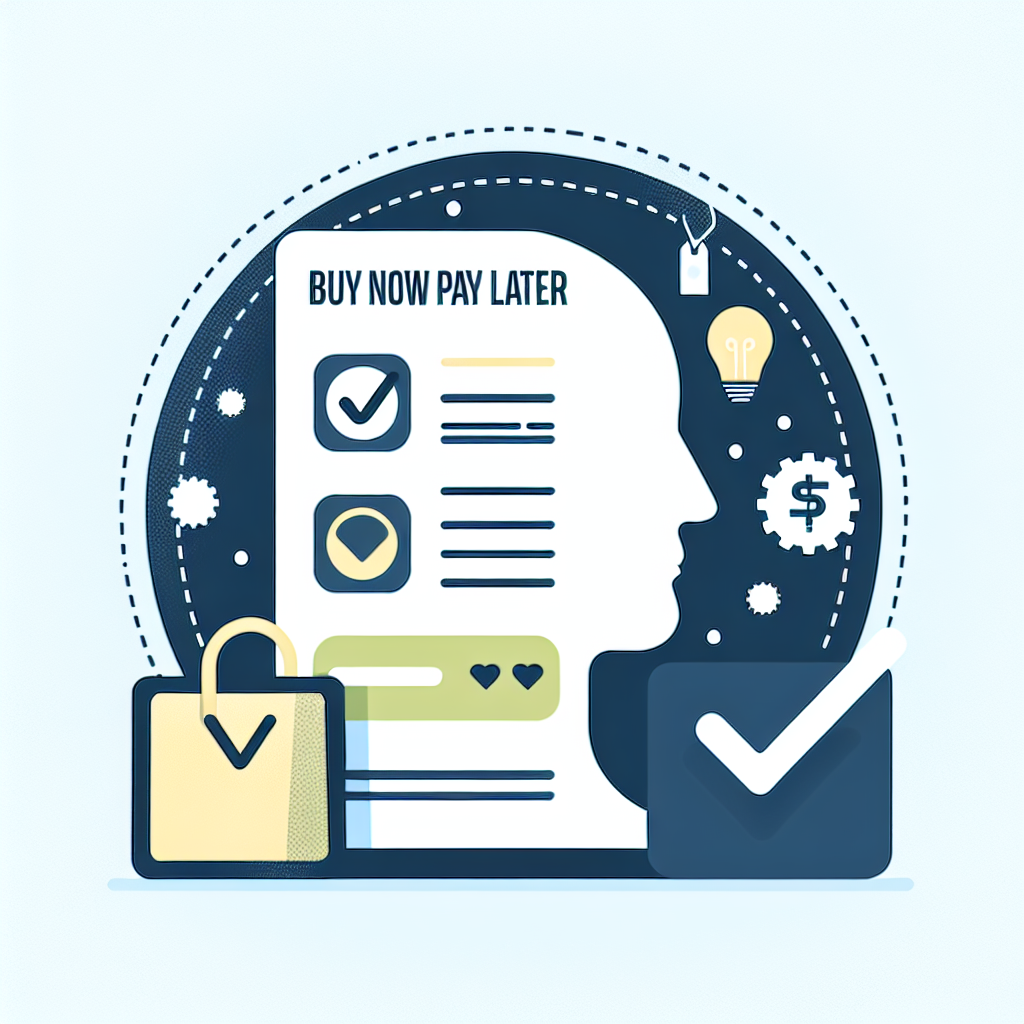
The Psychology Behind Buy Now Pay later
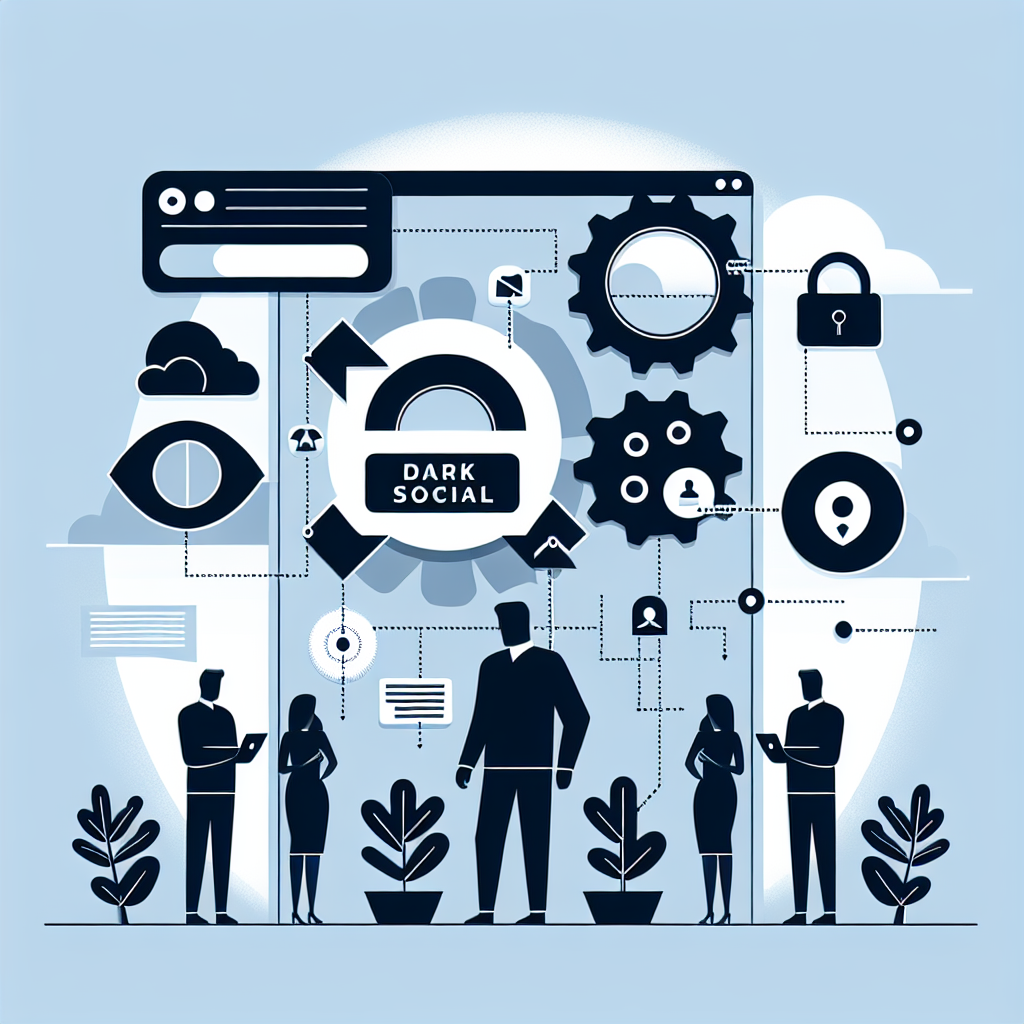
The Rise of Dark Social and Its Impact on Marketing Measurement
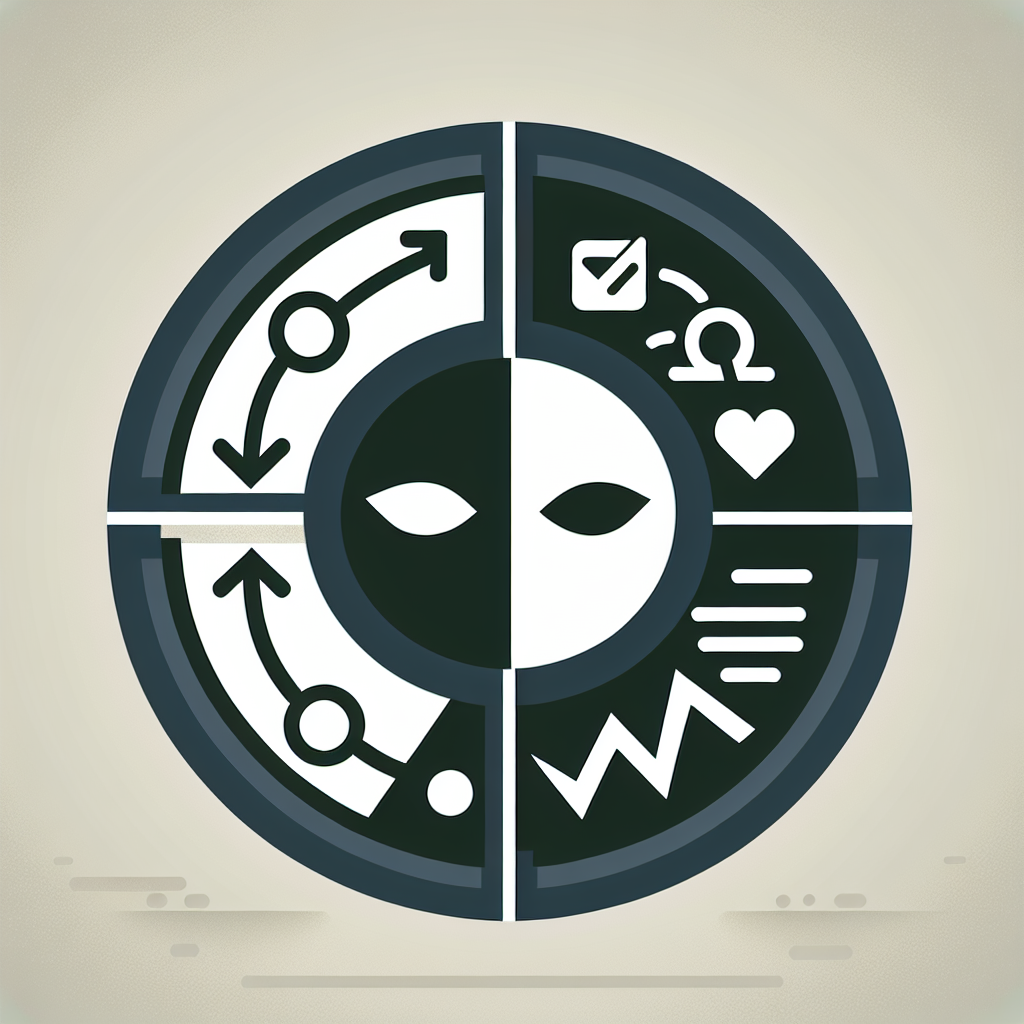
The Role of Dark Patterns in Digital Marketing and Ethical Concerns
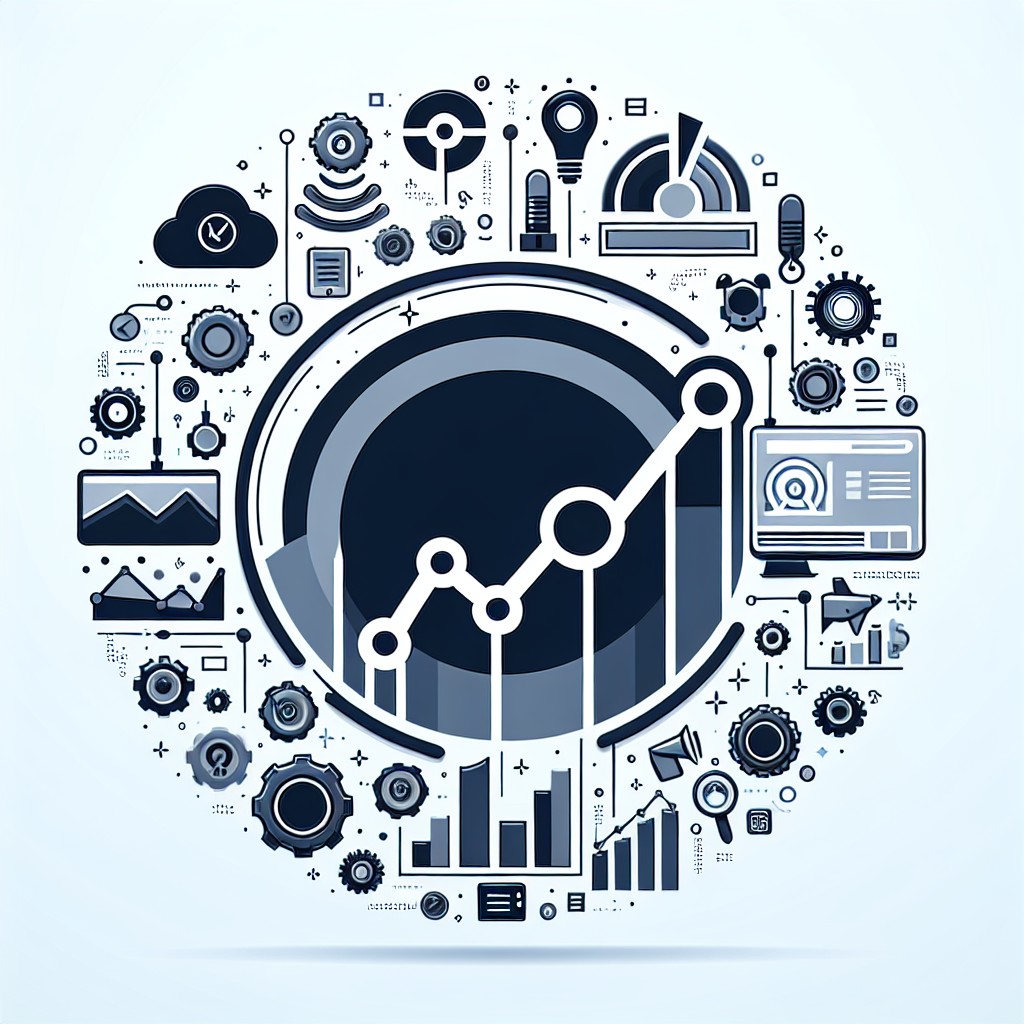