How Data Anonymization is Enabling Smarter Marketing Decisions
During a marketing strategy session last year, Pedro and his team faced a pivotal moment that changed his perspective on data forever. They had meticulously planned a campaign targeting high-value prospects based on detailed behavioral profiles. Just days before launch, their legal team flagged significant privacy compliance issues. The granular tracking they relied on violated emerging regulations, and they faced a painful choice—delay the campaign or launch with dramatically reduced targeting precision. The resulting compromise produced mediocre results, and Pedro found himself wondering: is this the inevitable future of marketing—a constant tension between effectiveness and privacy? This question launched his exploration into data anonymization techniques, where he discovered not a compromise but a revolution—a suite of sophisticated approaches that protect individual privacy while enabling even more robust marketing intelligence. What began as a crisis evolved into a profound insight: anonymization isn't marketing's constraint but its future.
Introduction: Beyond the Privacy-Utility Tradeoff
Marketing has traditionally operated on a fundamental assumption: greater personalization requires more personal data. This created what Stanford privacy researcher Arvind Narayanan calls "the privacy-utility tradeoff"—the perceived inverse relationship between data privacy and marketing effectiveness. However, this paradigm is being challenged by sophisticated anonymization techniques that transform how organizations measure marketing performance without compromising individual privacy.
Gartner predicts that by 2025, 75% of the world's population will have its personal data protected by privacy regulations. Simultaneously, the deprecation of third-party cookies and device identifiers is dismantling traditional attribution models. In response, forward-thinking organizations are deploying anonymization approaches that enable what marketing attribution expert Avinash Kaushik calls "intelligence without invasion"—deriving actionable insights while respecting consumer privacy preferences and regulatory requirements.
1. Differential Privacy: The Mathematical Foundation
Originally developed by Microsoft Research, differential privacy has evolved from theoretical concept to practical marketing application. This mathematical framework adds carefully calibrated "noise" to datasets, making individual identification impossible while preserving aggregate insights.
Google's implementation of differential privacy in their Attribution 360 platform exemplifies this evolution. Their system enables conversion measurement across channels without exposing individual user journeys. According to research published in the Harvard Business Review, this approach maintains 92% of measurement accuracy while eliminating individual exposure risk.
As privacy economist Alessandro Acquisti observes, differential privacy represents "not just a technical solution but a conceptual breakthrough—the mathematical proof that privacy and utility aren't inherently opposed." The approach is particularly valuable in sensitive industries like healthcare and finance, where companies like Anthem have used differentially private analysis to identify high-value marketing channels without exposing protected health information.
2. Federated Analytics: Distributed Intelligence
Federated analytics has evolved from Google's work on keyboard prediction to a powerful marketing measurement approach. The technique processes data locally on user devices rather than centralizing it, sharing only aggregated insights with central systems.
Pinterest's implementation of federated analytics demonstrates its marketing power. Their "on-device attribution" measures ad effectiveness without tracking users across websites. According to their Chief Marketing Officer Andréa Mallard, this approach "maintained measurement accuracy while completely eliminating cross-site tracking concerns," resulting in a 23% increase in opt-in rates.
This evolution addresses what privacy scholar Helen Nissenbaum calls "contextual integrity"—respecting the context in which data was originally shared. As marketing professor Clayton Christensen noted shortly before his passing, "Federated analytics represents a disruptive innovation in marketing measurement—maintaining performance while fundamentally changing the data architecture."
3. Homomorphic Encryption: Computing on Encrypted Data
Once considered theoretically interesting but practically impossible, homomorphic encryption has evolved into viable marketing technology. This approach enables computation on encrypted data without decryption, allowing analytics while maintaining complete data privacy.
LiveRamp's Data Clean Room technology demonstrates this evolution. Their platform enables brands like Unilever to match audience segments with publisher data without either party exposing raw information. According to research from Winterberry Group, this approach has recovered approximately 85% of the targeting capability traditionally provided by third-party cookies.
This represents what cryptography expert Matthew Green calls "the holy grail of privacy-preserving computation." The approach is particularly valuable for collaborations between brands and retailers. When Procter & Gamble implemented homomorphic encryption for analyzing Walmart purchase data, they identified product affinity patterns that increased campaign ROI by 18% while maintaining strict data boundaries between organizations.
4. Machine Learning on Synthetic Data
The application of synthetic data—artificially generated information statistically identical to real data but containing no actual individuals—represents one of the most promising anonymization developments. This approach creates what MIT Technology Review calls "privacy by simulation" rather than redaction.
Mastercard's implementation of synthetic data for marketing analytics showcases this evolution. Their Data for Good program generates synthetic transaction datasets that preserve spending patterns while eliminating re-identification risk. According to their Chief Data Officer JoAnn Stonier, this enabled marketing partners to develop "twice as many viable consumer insights with zero privacy risk."
This approach addresses what Carnegie Mellon researcher Latanya Sweeney demonstrated decades ago—that seemingly anonymized data could often be re-identified through correlation with other datasets. As marketing science researcher Elea Feit observes, "Synthetic data doesn't just mask individuals—it eliminates them entirely while preserving the patterns that drive marketing decisions."
5. Multiparty Computation: Collaborative Intelligence
Secure multiparty computation (MPC) has evolved from theoretical cryptography to practical marketing application. This approach enables multiple organizations to analyze combined data without any party seeing the others' information.
Facebook's Conversions API with MPC exemplifies this evolution. Their system allows advertisers to verify campaign performance by matching conversions with ad exposures without either Facebook or the advertiser accessing the other's raw data. Research by the World Federation of Advertisers shows this methodology maintains 94% of attribution accuracy while eliminating cross-site tracking.
This represents what privacy economist Garrett Johnson calls "the collaborative future of marketing measurement"—where insights emerge from secure computation across organizational boundaries rather than centralized data collection. When L'Oréal implemented MPC-based measurement with major retailers, they uncovered attribution insights that improved ROAS by 23% while maintaining strict data separation between partners.
Conclusion: The Convergence of Privacy and Intelligence
The evolution of these anonymization approaches reveals a profound shift in marketing measurement—from sacrificing either privacy or utility to enhancing both simultaneously. The most sophisticated marketing organizations now recognize that anonymization isn't merely a compliance requirement but a strategic advantage that builds consumer trust while enabling deeper marketing intelligence.
This represents what marketing philosopher Seth Godin describes as the shift from "data-hungry to data-smart" practices—where the quality of analysis rather than the quantity of collection drives marketing performance. The organizations succeeding in this new landscape understand that privacy-first isn't just about what data you can't collect—it's about extracting more value from the data you can ethically access.
Call to Action
For marketing leaders navigating this privacy-first measurement landscape:
- Conduct an attribution audit to identify where personal data is truly necessary versus where anonymized approaches could provide similar insights with reduced privacy risk.
- Invest in building first-party data excellence while simultaneously developing anonymization capabilities that extend its utility beyond organizational boundaries.
- Collaborate with privacy engineering teams early in measurement design rather than retrofitting privacy after systems are built.
The future belongs to organizations that recognize anonymization not as a limitation but as the foundation for more sustainable, trusted, and ultimately more effective marketing intelligence.
Featured Blogs
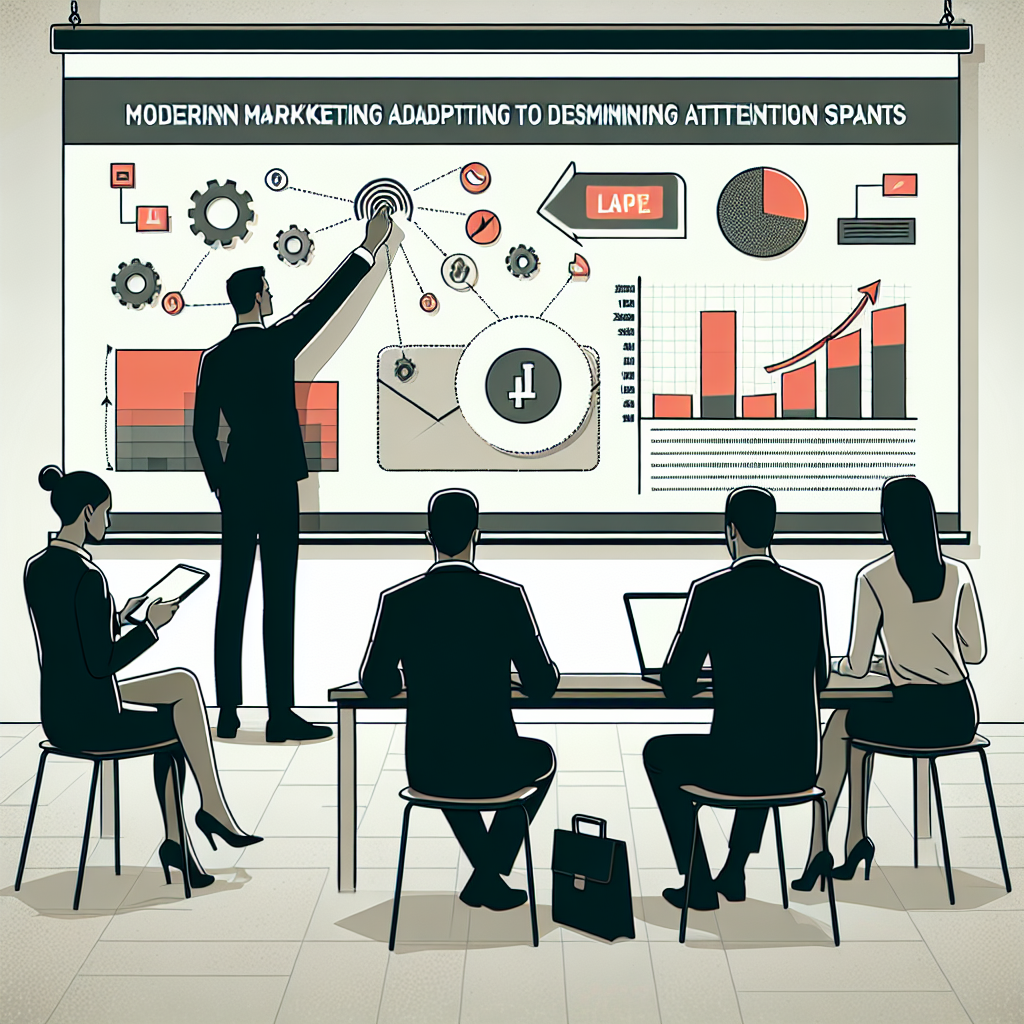
How the Attention Recession Is Changing Marketing
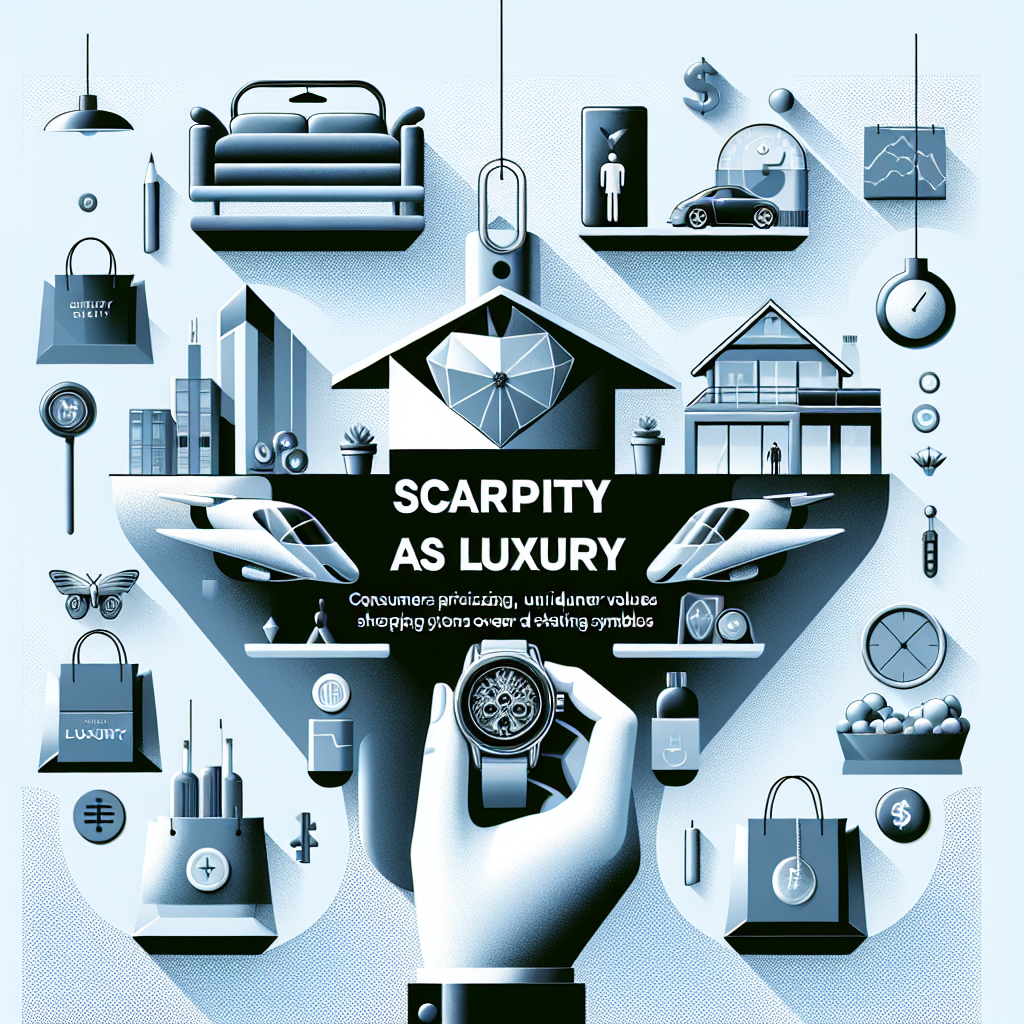
The New Luxury Why Consumers Now Value Scarcity Over Status
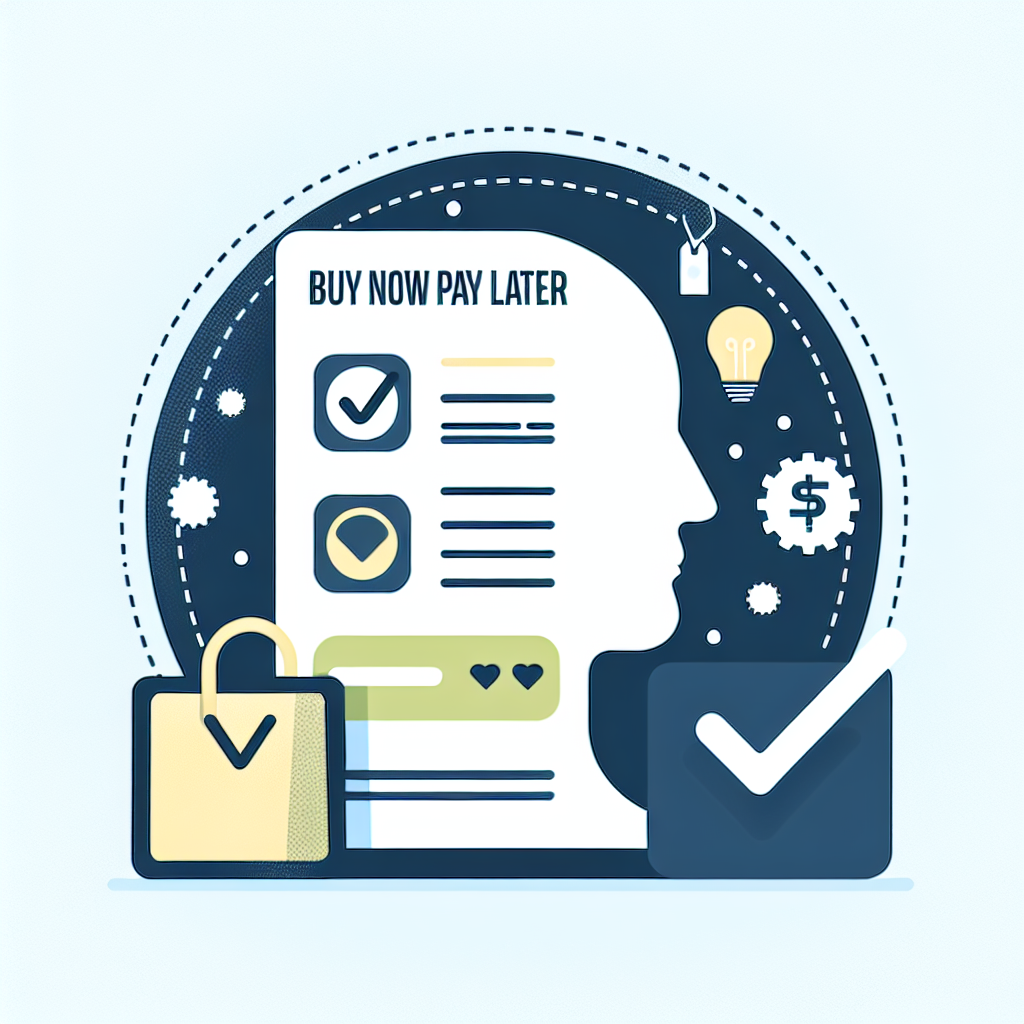
The Psychology Behind Buy Now Pay later
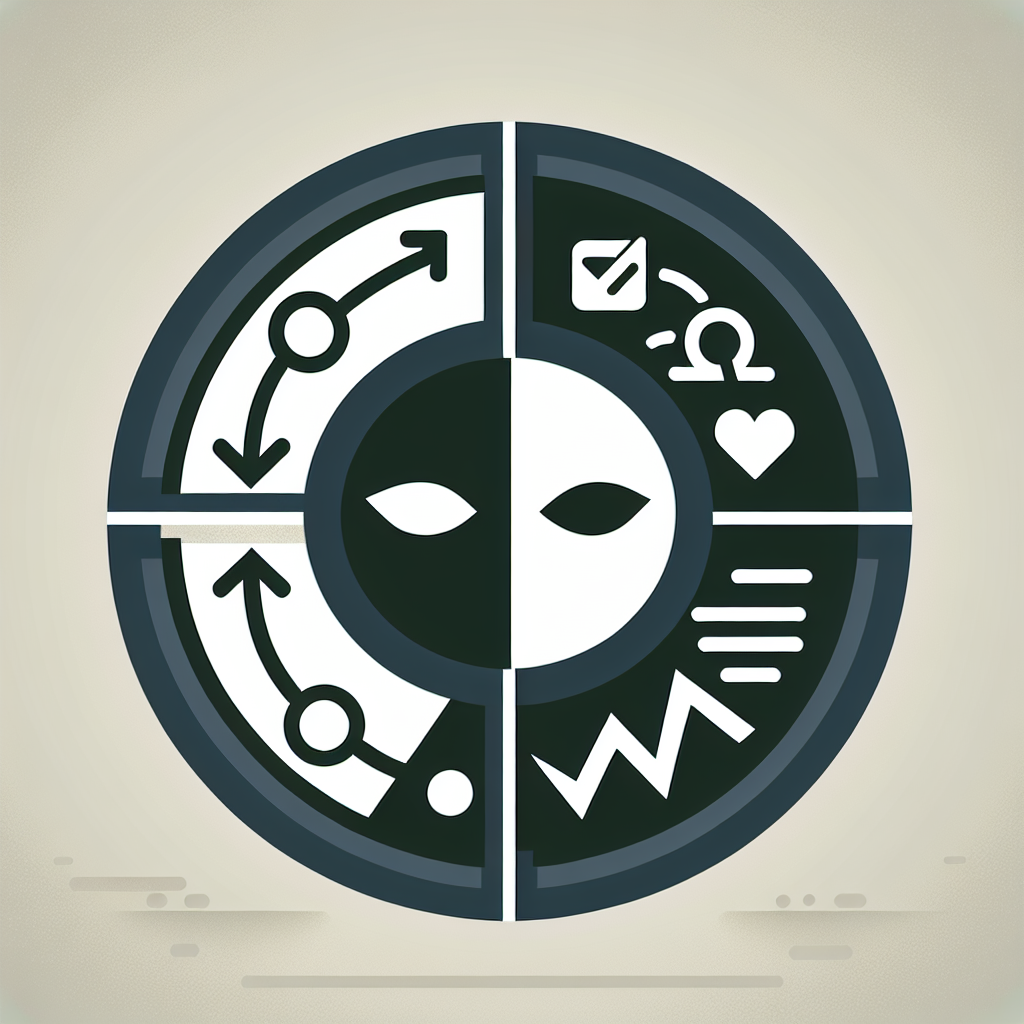
The Role of Dark Patterns in Digital Marketing and Ethical Concerns
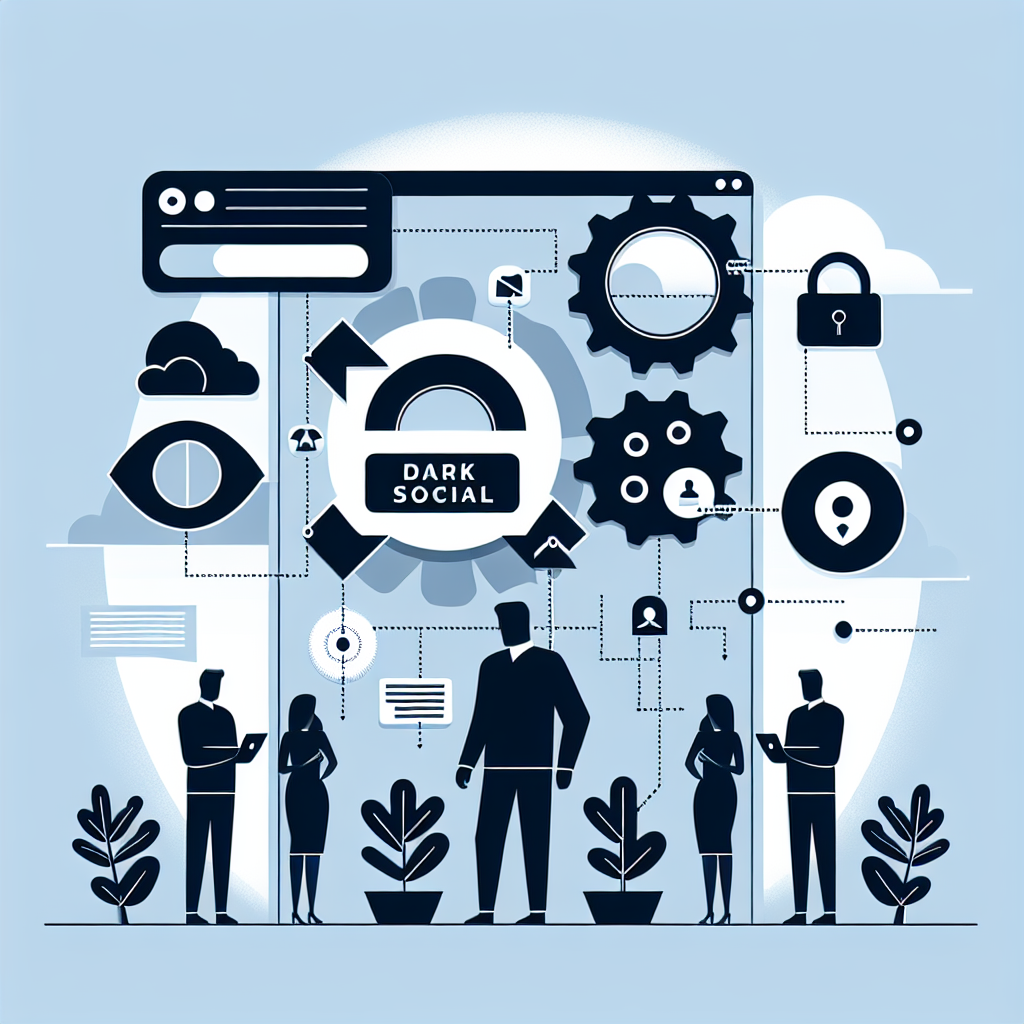
The Rise of Dark Social and Its Impact on Marketing Measurement
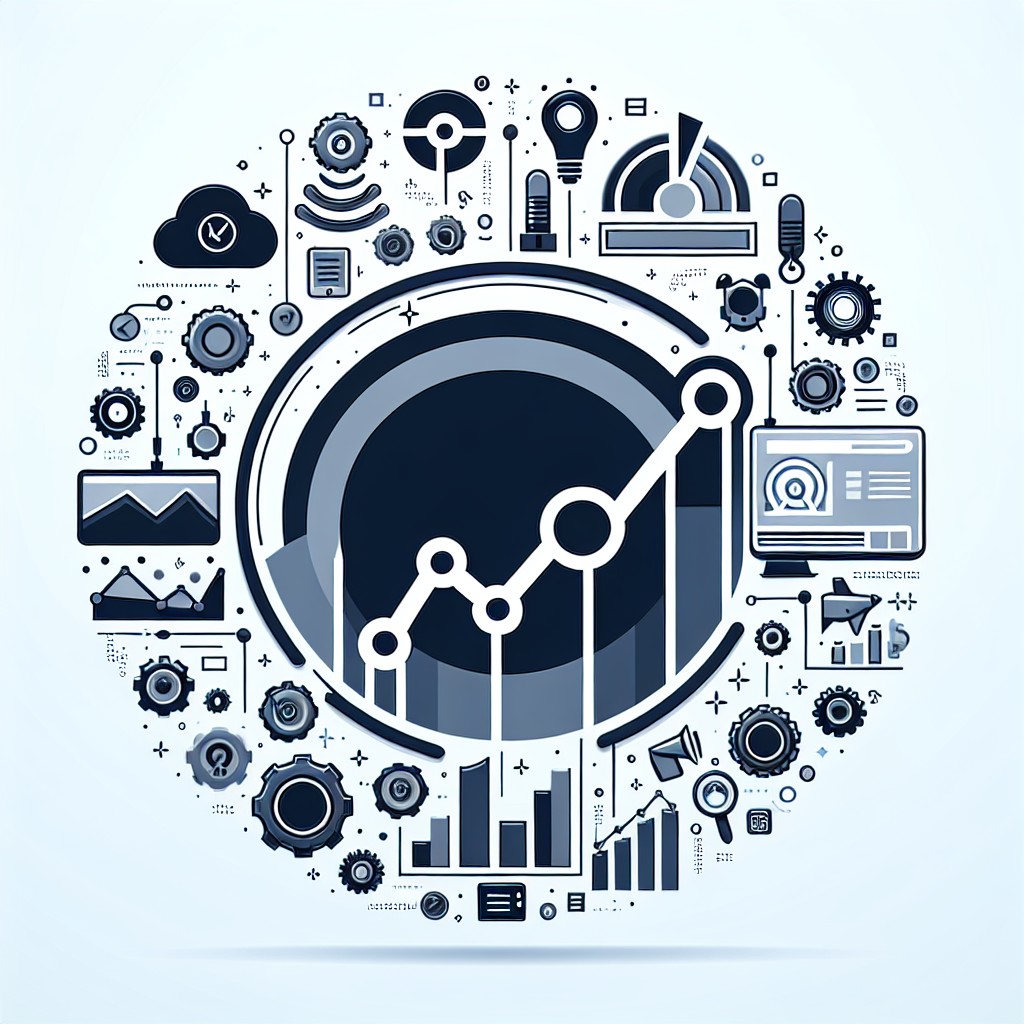