How Brands Can Use Incrementality Testing for Privacy Safe Measurement
The boardroom fell silent as Ray's largest e-commerce client questioned their latest campaign report. "These attribution numbers look impressive," their CMO said, "but how do we know these customers wouldn't have converted anyway?" Ray had been asking himself the same question for months. Their multi-touch attribution model, built on third-party cookies, showed excellent ROAS—yet something felt off. When certain channels were paused due to budget cuts, overall performance barely changed despite the model predicting significant drops. That weekend, Ray dove into research on incrementality testing, a methodology he'd only vaguely understood before. What began as professional necessity quickly became intellectual fascination. Here was an approach that didn't just track customer journeys but scientifically measured true marketing impact. As privacy regulations tightened and cookies began disappearing, this methodology that had existed on marketing's periphery suddenly seemed like the future of measurement itself.
Introduction: Beyond Correlation, Toward Causation
As third-party cookies crumble and device identifiers diminish, marketing measurement faces what the World Federation of Advertisers calls "the greatest disruption since the advent of digital advertising." Traditional attribution models—dependent on persistent user tracking—increasingly deliver incomplete or misleading insights in this privacy-first landscape.
Incrementality testing emerges as not merely an alternative but a methodological upgrade. Rooted in scientific experimental design, it answers the fundamental question: what incremental value do marketing investments actually generate? Unlike attribution models that infer causality from observed correlations, incrementality testing directly measures causal impact through controlled experimentation.
According to Professor Garrett Johnson of Northwestern University's Kellogg School of Management, "Incrementality testing represents a shift from passive observation to active experimentation—a transition that simultaneously improves measurement accuracy and reduces privacy concerns." This approach aligns perfectly with emerging privacy regulations by focusing on aggregate results rather than individual tracking.
1. The Experimental Foundation: Causation Through Controlled Testing
At its core, incrementality testing applies scientific experimental principles to marketing measurement. The methodology divides audiences into test (exposed to marketing) and control (unexposed) groups, measuring the difference in behaviors between them.
The gold standard approach, as outlined by Facebook's measurement team, employs randomized controlled trials (RCTs) that eliminate selection bias by randomly assigning users to test and control groups. This methodology addresses what Professor David Reiley of UC Berkeley identifies as "the fundamental attribution problem: distinguishing marketing-driven behaviors from behaviors that would have occurred organically."
This experimental design evolved from traditional holdout testing but incorporates sophisticated techniques including:
- Ghost ads: Serving blank impressions to control groups
- Intent-based segmentation: Creating matched control groups based on behavioral signals
- Multi-cell designs: Testing multiple variations simultaneously
These approaches enable what Stanford's Digital Marketing Program calls "the isolation of true incremental impact from background noise."
2. Implementation Strategies: From Theory to Organizational Practice
Leading organizations have developed systematic approaches to incrementality testing that balance scientific rigor with practical implementation:
Airbnb's "Experiment Everywhere" framework, described by their former Director of Experimentation Jeff Feng, integrates incrementality testing into their core marketing workflow. Rather than treating experiments as occasional validation exercises, they establish always-on test and control groups across channels, creating what Feng calls "a continuous feedback loop of incrementality insights."
Adidas similarly transformed their measurement approach after privacy regulations limited their traditional attribution capabilities. Their "Test & Learn" framework maintains a 10% persistently unexposed control group across digital channels, enabling what their VP of Global Media James Yeates describes as "a privacy-compatible source of truth for marketing effectiveness."
These implementations demonstrate that incrementality testing isn't merely a measurement technique but a transformation of how organizations approach marketing decision-making.
3. Cross-Channel Applications: Beyond Digital Boundaries
While initially popular in digital channels, incrementality testing methodologies have expanded to create holistic, cross-channel measurement systems:
Target's measurement team developed what they call "Media Mix Experimentation"—systematically varying marketing investments across geographical regions to measure incrementality across both online and offline channels. This approach enabled what their Chief Marketing Officer Rick Gomez describes as "privacy-safe measurement that captures online-to-offline effects without requiring individual-level tracking."
Professor Catherine Tucker of MIT Sloan observes that this expansion beyond digital enables "a more comprehensive understanding of marketing effectiveness that wasn't possible even with perfect digital attribution," creating a strategic advantage from what initially appeared to be a privacy-driven constraint.
4. Machine Learning Enhancements: Improving Accuracy and Efficiency
Advanced analytics and machine learning techniques have dramatically enhanced incrementality testing capabilities:
Booking.com's experimentation platform integrates causal machine learning algorithms to identify the most responsive audience segments within test results. This approach, which their Chief Scientist Lukas Vermeer calls "automated incrementality analysis," identifies which customer cohorts deliver the highest incremental response, enabling more precise targeting without relying on individual tracking.
Similarly, Wayfair's measurement team employs Bayesian statistical methods to model incrementality across customer segments, allowing them to run shorter experiments with smaller test groups while maintaining statistical confidence. This approach enables what their VP of Marketing Science Jim Hazen describes as "continuous incrementality testing at scale."
5. Organizational Transformation: The Cultural Shift Toward Experimentation
Successfully implementing incrementality testing requires significant organizational and cultural changes:
Microsoft's marketing measurement transformation involved what their CMO Chris Capossela calls "rebuilding our measurement culture around experimentation." This included retraining analysts, establishing a centralized experimentation platform, and aligning incentives around incremental metrics rather than attributed conversions.
Professor Stefan Thomke of Harvard Business School notes that this cultural transformation often represents the greatest challenge: "Organizations must embrace experimental thinking, including the willingness to discover that some cherished marketing activities generate no incremental value."
This perspective shift represents both the greatest challenge and opportunity of incrementality testing—it forces organizations to confront marketing's true impact rather than celebrating correlation-based metrics.
Conclusion: The Inevitable Experimental Future
As privacy regulations strengthen and technical capabilities for user-level tracking diminish, incrementality testing represents not merely a tactical alternative but a superior approach to marketing measurement. By directly measuring causal impact through experimental design, organizations simultaneously improve accuracy and eliminate privacy concerns.
The transition requires significant investment in methodological expertise, technical infrastructure, and organizational change. However, as Professor Scott Galloway of NYU Stern observes, "The privacy-driven shift toward experimental measurement may ultimately deliver what marketers have always sought but rarely achieved: genuine understanding of marketing's causal impact on business outcomes."
Call to Action
For marketing leaders navigating measurement transformation, three priorities emerge:
- Begin with pilot incrementality tests in high-investment channels to develop methodological expertise and demonstrate the approach's value
- Invest in experimental infrastructure and expertise, focusing on statistical rigor and test design capabilities
- Initiate the cultural transformation by reframing success metrics around incremental impact rather than attributed conversions
The organizations that execute on these priorities will not merely survive the measurement disruption—they will develop a sustainable competitive advantage through superior understanding of marketing's true impact in the privacy-first era.
Featured Blogs
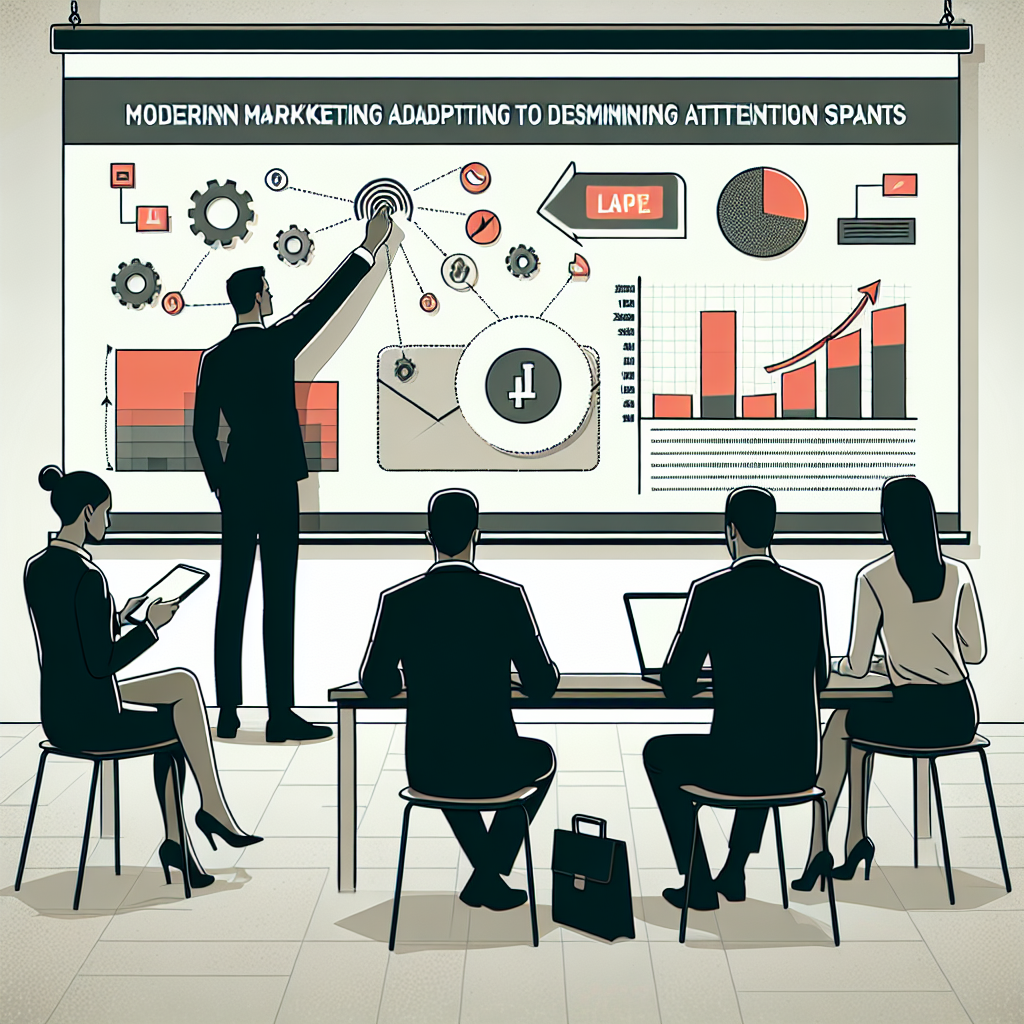
How the Attention Recession Is Changing Marketing
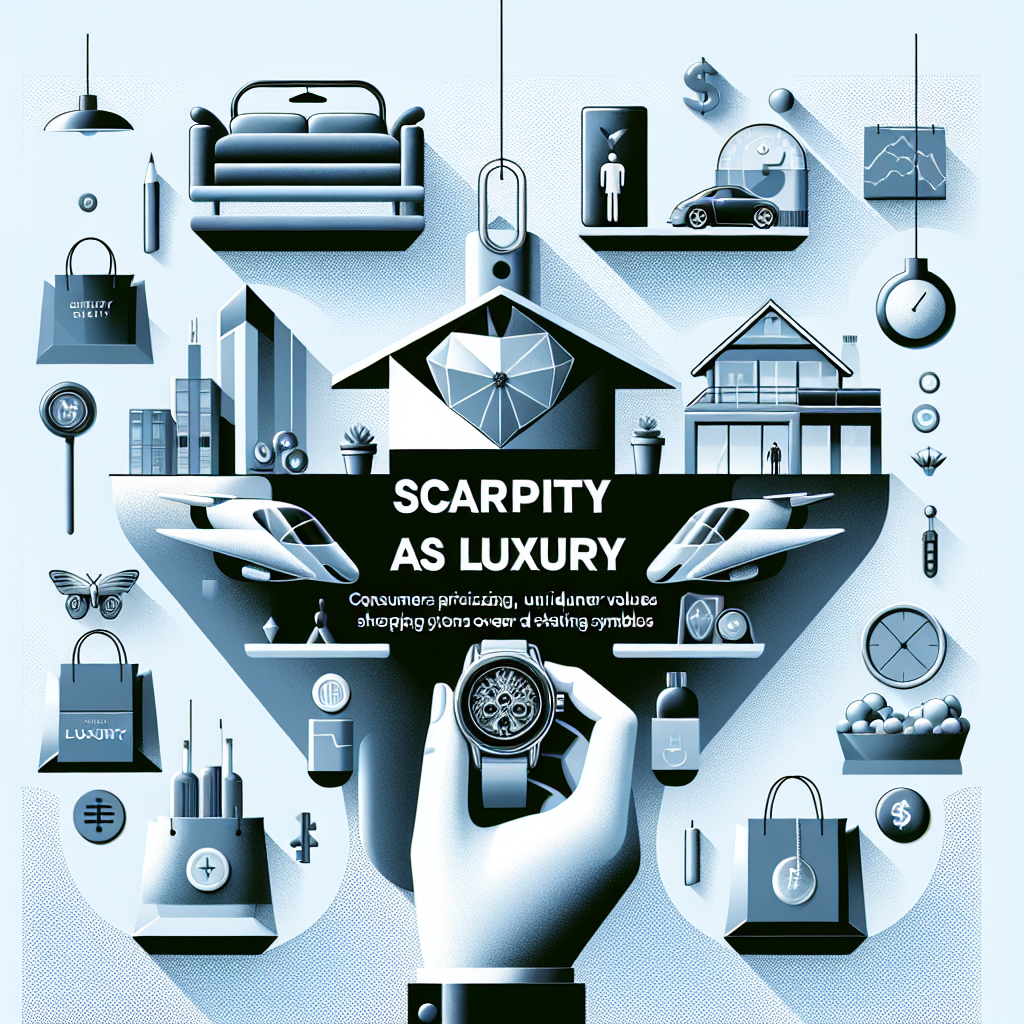
The New Luxury Why Consumers Now Value Scarcity Over Status
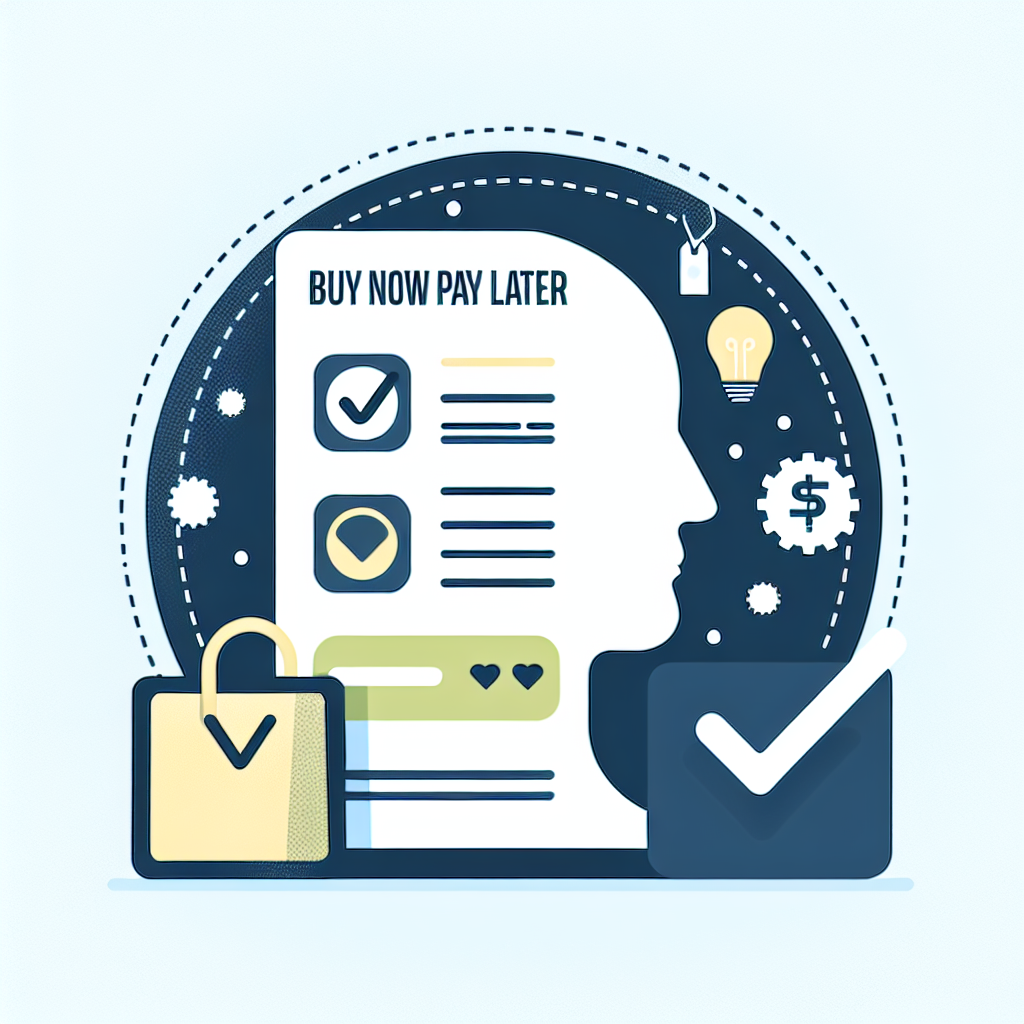
The Psychology Behind Buy Now Pay later
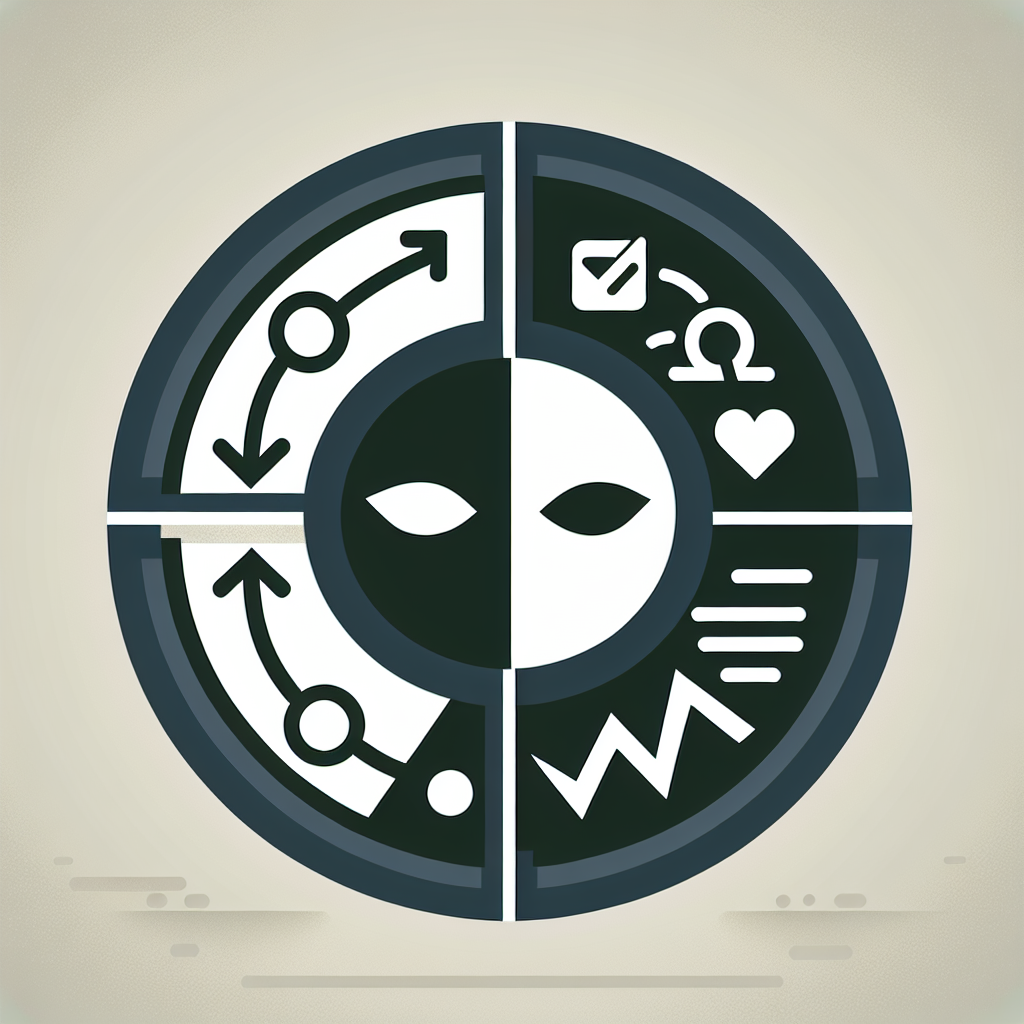
The Role of Dark Patterns in Digital Marketing and Ethical Concerns
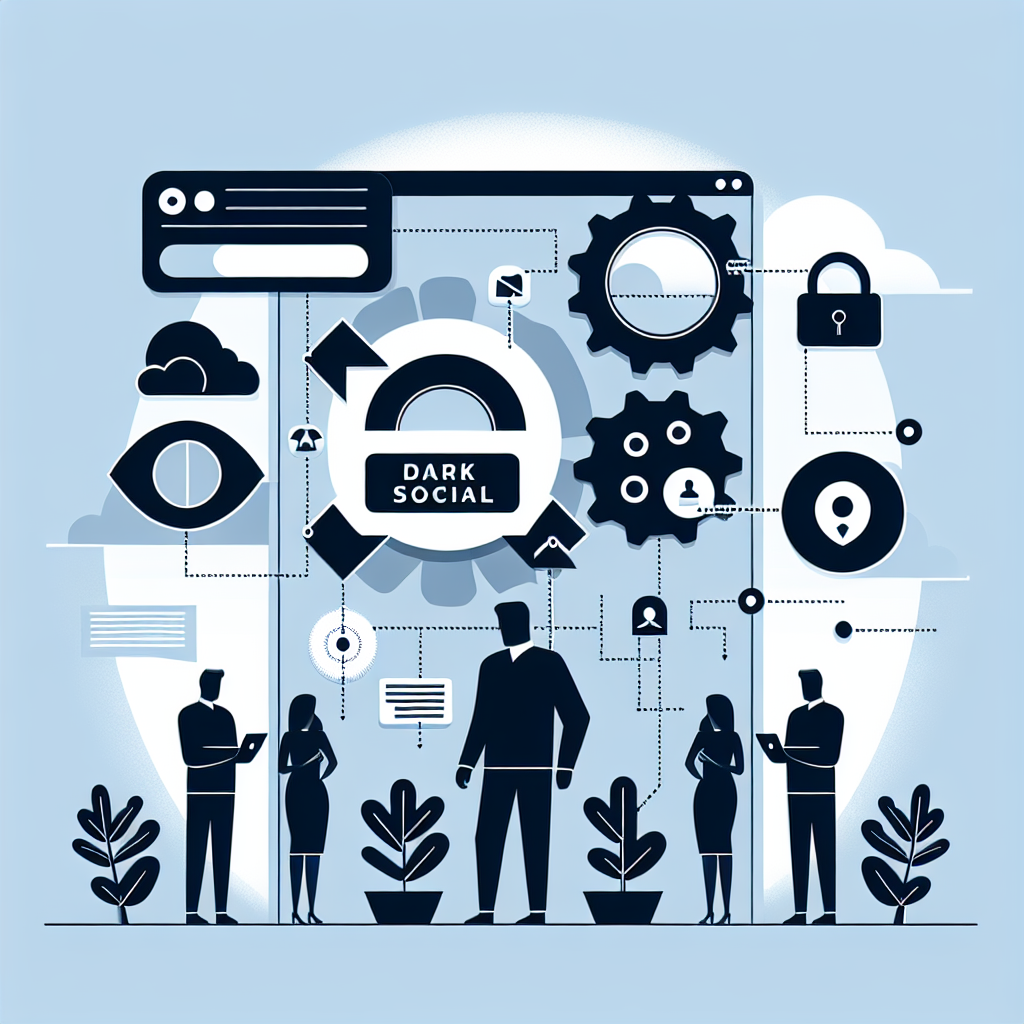
The Rise of Dark Social and Its Impact on Marketing Measurement
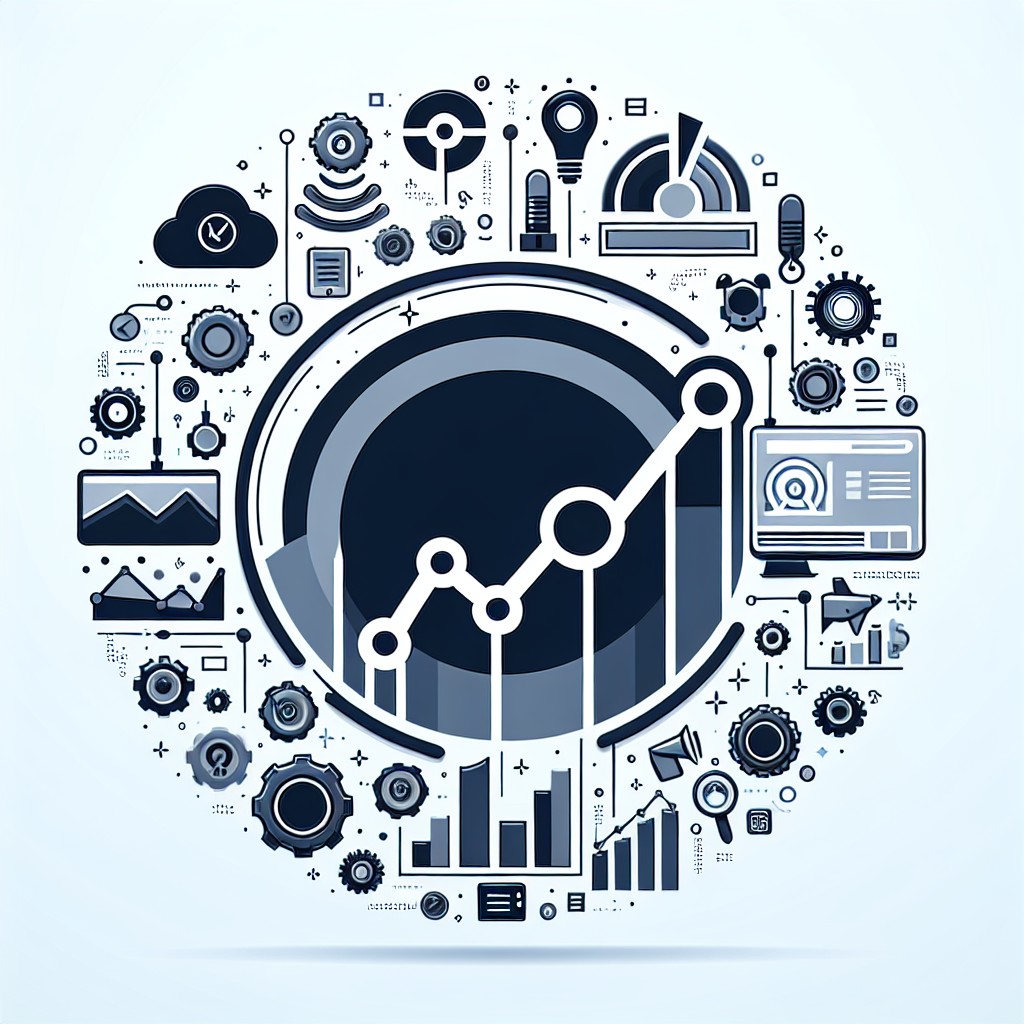
The Future of Retail Media Networks and What Marketers Should Know
Recent Blogs
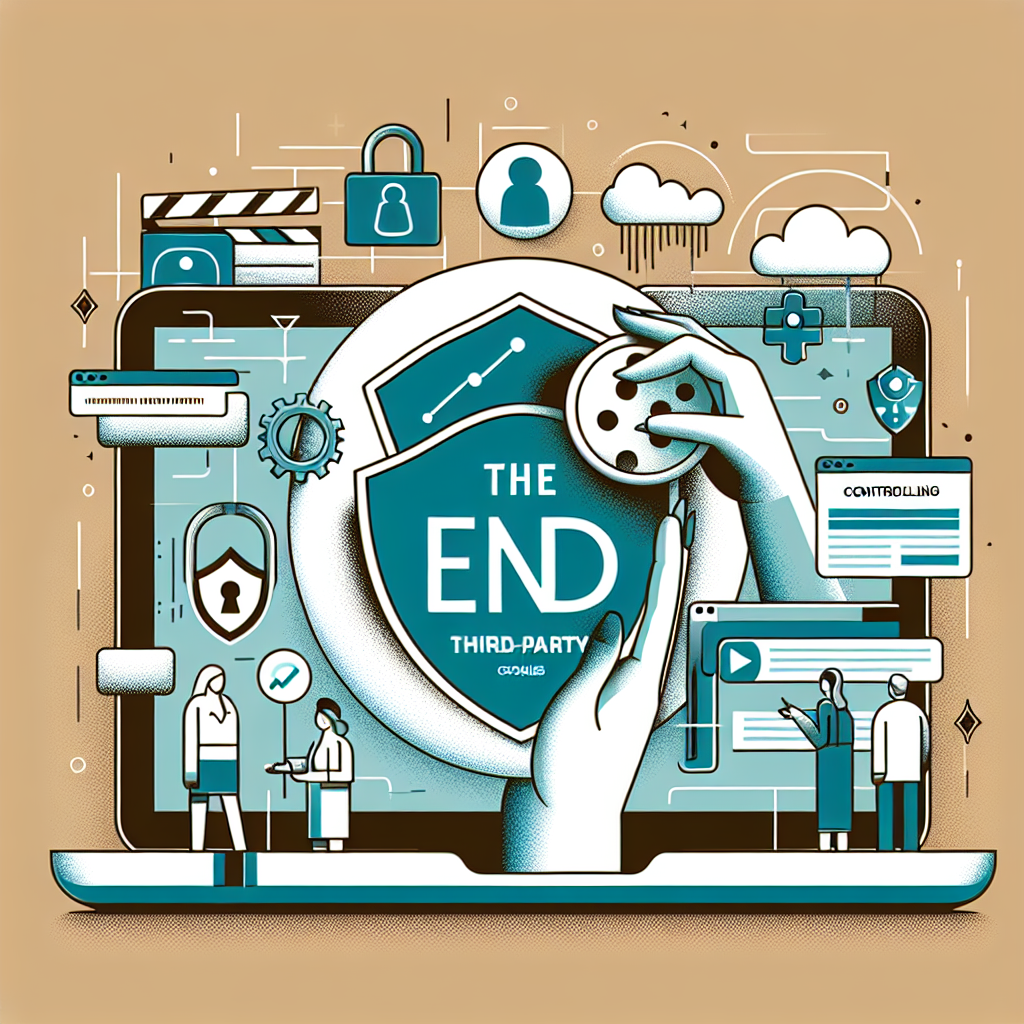
Why the Death of Third-Party Cookies is a Win for Consumer Privacy
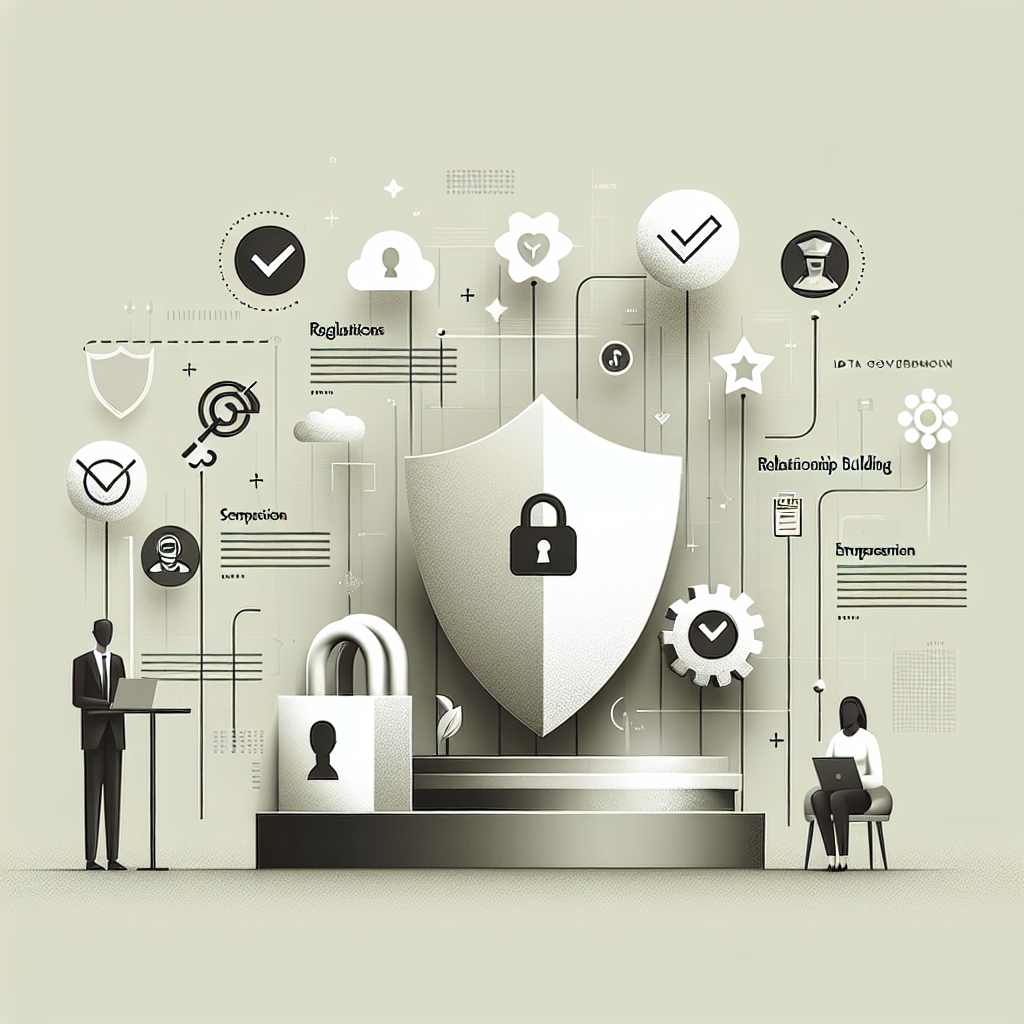
Why Brands Need to Rethink Data Governance in a Privacy-First Era
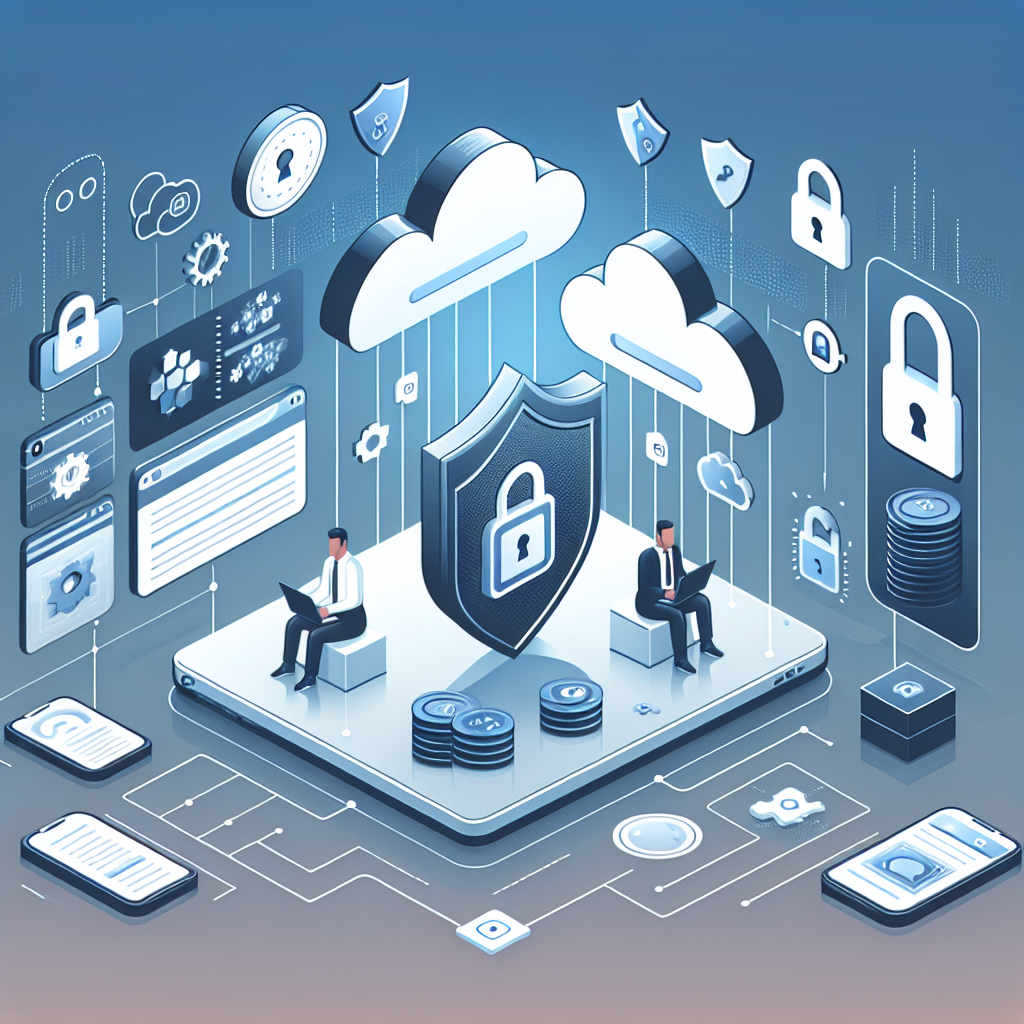
The Shift to a Privacy-First Digital Landscape
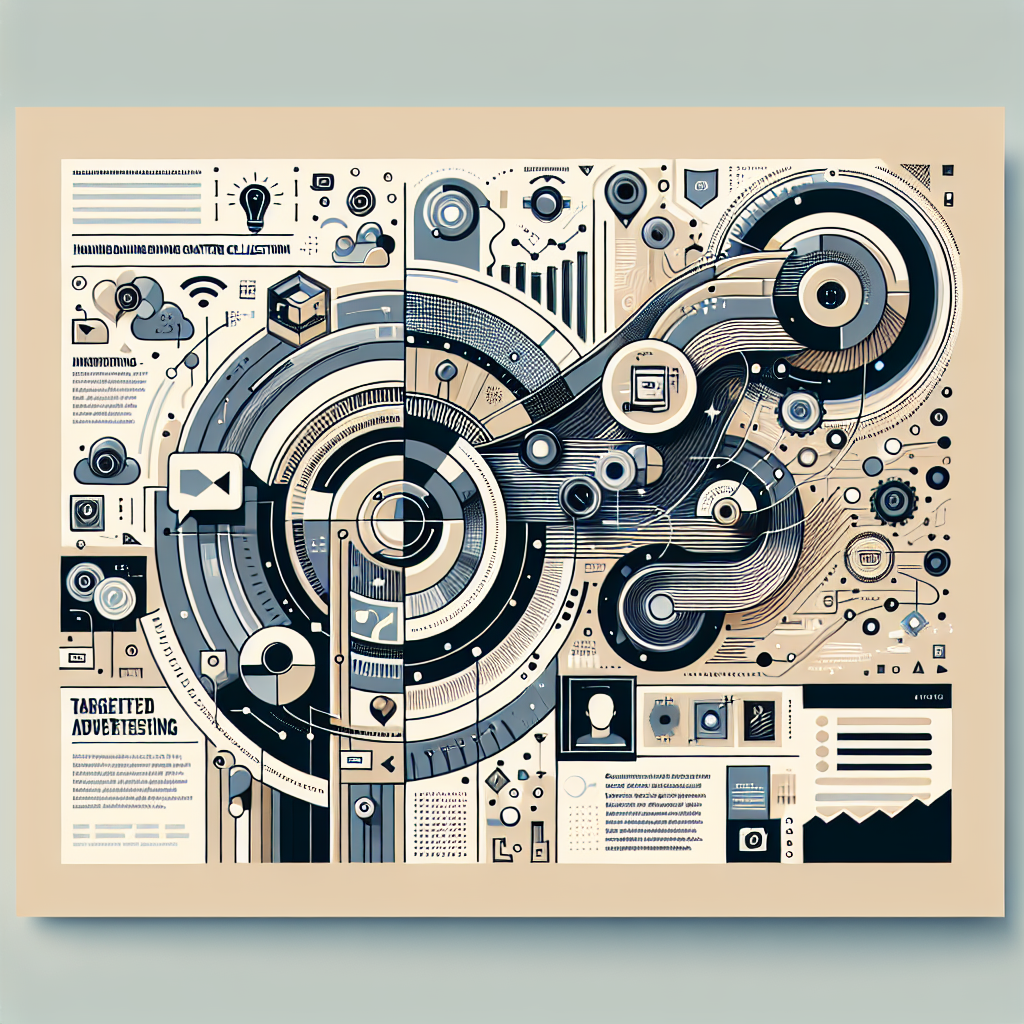
What Google's Privacy Sandbox Means for Marketers
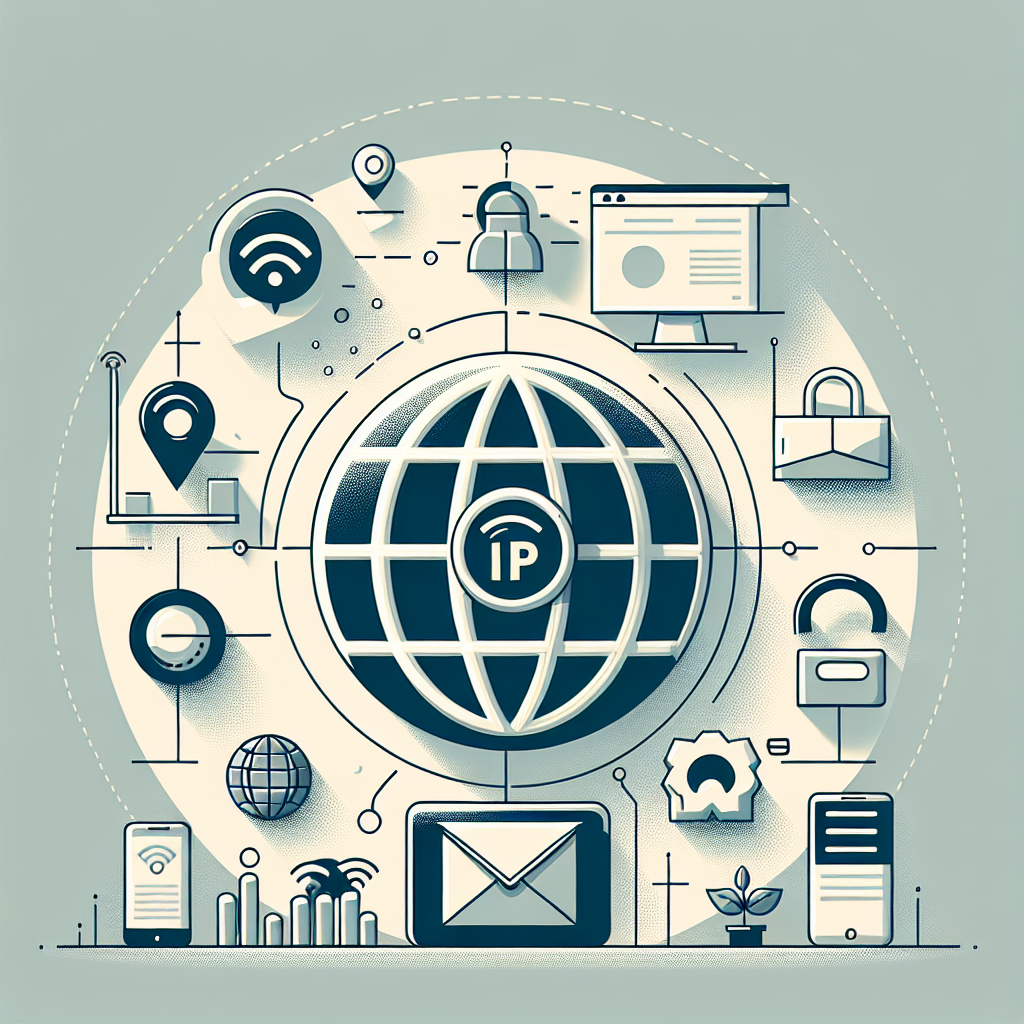
The Role of IP-Based Targeting in a Post-Cookie World
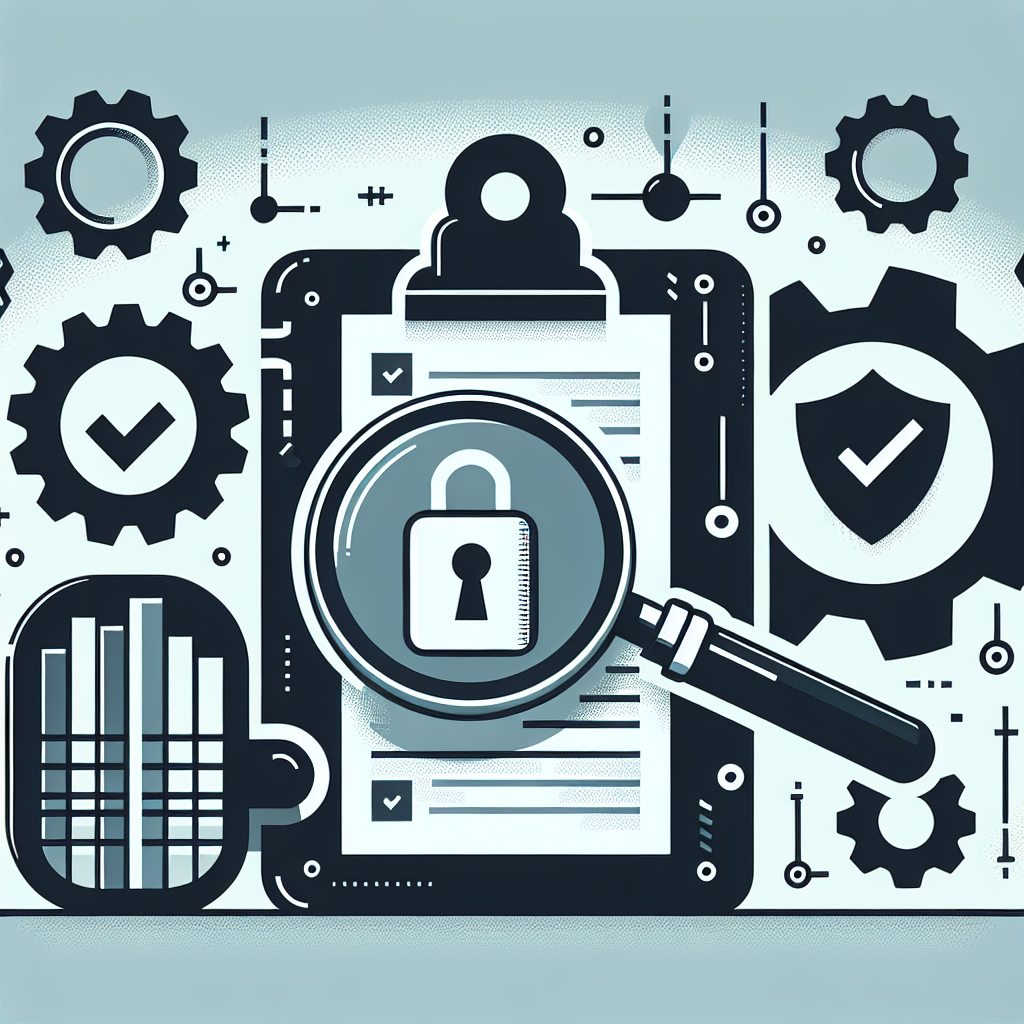