The Role of Synthetic Data in Privacy-First Marketing
It was during a routine privacy compliance meeting that Pedro experienced his first "aha moment" about synthetic data. As Pedro's team struggled with balancing personalization demands against increasingly stringent privacy regulations, a colleague mentioned how a competitor had begun generating synthetic customer profiles that retained statistical accuracy without using any real personal information. The concept seemed almost paradoxical—creating data that wasn't real but could still drive accurate insights. This revelation sparked Pedro's journey into understanding how synthetic data could revolutionize marketing in an era where privacy has become both a regulatory requirement and a competitive advantage. What Pedro discovered was not just a tactical workaround but a fundamental paradigm shift in how marketers can ethically leverage data.
Introduction: The Privacy-Personalization Paradox
In today's digital landscape, marketers face an unprecedented challenge: delivering hyper-personalized experiences while respecting consumer privacy and complying with regulations like GDPR, CCPA, and Apple's App Tracking Transparency framework. The demise of third-party cookies, stricter consent requirements, and growing consumer privacy consciousness have created what Gartner analyst Andrew Frank calls "the privacy-personalization paradox."
Traditional approaches relied heavily on collecting and processing massive volumes of personal data, often with limited transparency. Enter synthetic data—artificially generated information that maintains the statistical properties and patterns of real data without containing any actual personal information. This emerging approach is becoming the bridge between personalization necessities and privacy imperatives.
1. Understanding Synthetic Data in Marketing
Synthetic data refers to artificially generated information that mirrors the statistical properties of real data without containing actual customer records. It comes in several forms:
Fully synthetic data
is created entirely through algorithmic means, capturing only the distribution patterns of original datasets.
Partially synthetic data
combines real data structures with artificially generated values for sensitive fields.
Hybrid approaches
use techniques like differential privacy to add controlled noise that preserves aggregate insights while protecting individual identities.
Dr. Cathy O'Neil, author of "Weapons of Math Destruction," notes that "synthetic data allows organizations to share the valuable patterns within their data while mathematically guaranteeing that no individual's information is exposed."
2. Key Applications in Marketing
Synthetic data is transforming marketing processes across multiple domains:
Testing and Innovation
Brands like Unilever have created synthetic customer cohorts to test campaign performance without risking real customer data. This approach reduced their innovation cycle time by 30% while eliminating privacy risks.
Cross-Organization Collaboration
In a landmark initiative, competing financial institutions created a synthetic fraud detection database, sharing patterns without exposing sensitive customer information, resulting in a 22% improvement in fraud detection capabilities.
Training AI Models
Netflix reportedly uses synthetic viewing data to develop and test recommendation algorithms before deploying them with real user data, enhancing both privacy and development efficiency.
Marketing Simulation
L'Oréal has pioneered synthetic market simulation environments where thousands of campaign variants can be tested against synthetic consumer populations, providing insights that would be impossible to gather ethically from real customers.
3. The Technology Foundation
The generation of high-quality synthetic data relies on sophisticated technologies:
Generative Adversarial Networks (GANs)
create synthetic data through a competition between two neural networks—one generating fake samples and another discriminating between real and synthetic data.
Variational Autoencoders (VAEs)
learn the statistical distribution of real data and generate new samples that follow the same distribution.
Agent-Based Modeling
simulates individual consumer behaviors to create synthetic population data that reflects real-world dynamics.
According to research from MIT's Media Lab, synthetic data generated using these techniques can now match the analytical utility of real data at 95-99% accuracy for many marketing applications.
4. Challenges and Limitations
Despite its promise, synthetic data faces significant hurdles:
Quality Assurance
Ensuring synthetic data accurately represents real-world complexity without introducing subtle biases remains challenging.
Utility-Privacy Tradeoff
As Professor Helen Nissenbaum of Cornell Tech notes, "There is an inherent tension between data utility and perfect privacy that no synthetic data approach can completely resolve."
Regulatory Uncertainty
While synthetic data isn't explicitly addressed in most privacy regulations, legal frameworks are still evolving regarding its status.
Implementation Complexity
Organizations like Procter & Gamble have reported significant resource investments when transitioning to synthetic data approaches, with ROI taking 12-18 months to materialize.
5. The Future of Synthetic Data in Marketing
The trajectory of synthetic data points toward several emerging developments:
Democratization
Tools like DataSynthesizer and Mostly.ai are making synthetic data generation accessible to marketing teams without deep data science expertise.
Synthetic Data as a Service
Specialized providers are emerging that offer pre-generated synthetic consumer datasets reflecting specific market segments.
Regulatory Acceptance
European Data Protection Board has begun drafting guidelines on synthetic data, potentially creating a framework for its approved use in marketing.
Cross-Channel Applications
Pioneering brands are building unified synthetic consumer journeys that span multiple touchpoints, enabling privacy-safe omnichannel strategy development.
Conclusion: A New Foundation for Ethical Marketing
Synthetic data represents more than a tactical response to privacy regulations—it offers a fundamentally different approach to data-driven marketing. By decoupling insight generation from personal data usage, it enables marketers to continue delivering personalized experiences while respecting consumer privacy boundaries.
As Christian Selchau-Hansen, CEO of Formation.ai, observes: "Synthetic data isn't about finding loopholes in privacy regulations; it's about reconceptualizing how we generate marketing insights in a way that's inherently more respectful of consumer privacy."
Organizations that master synthetic data approaches today are positioning themselves not just for regulatory compliance but for leadership in the privacy-first future of marketing.
Call to Action
For marketing leaders looking to incorporate synthetic data into their strategies:
Begin with a synthetic data pilot in a controlled environment, ideally focusing on a specific use case like campaign testing or audience segmentation.
Invest in cross-functional expertise that bridges marketing analytics, data science, and privacy governance.
Engage with emerging synthetic data communities and standards bodies to stay ahead of best practices and regulatory developments.
The future of marketing belongs to those who can innovate within privacy constraints rather than despite them—and synthetic data may well be the key to unlocking that capability.
Featured Blogs
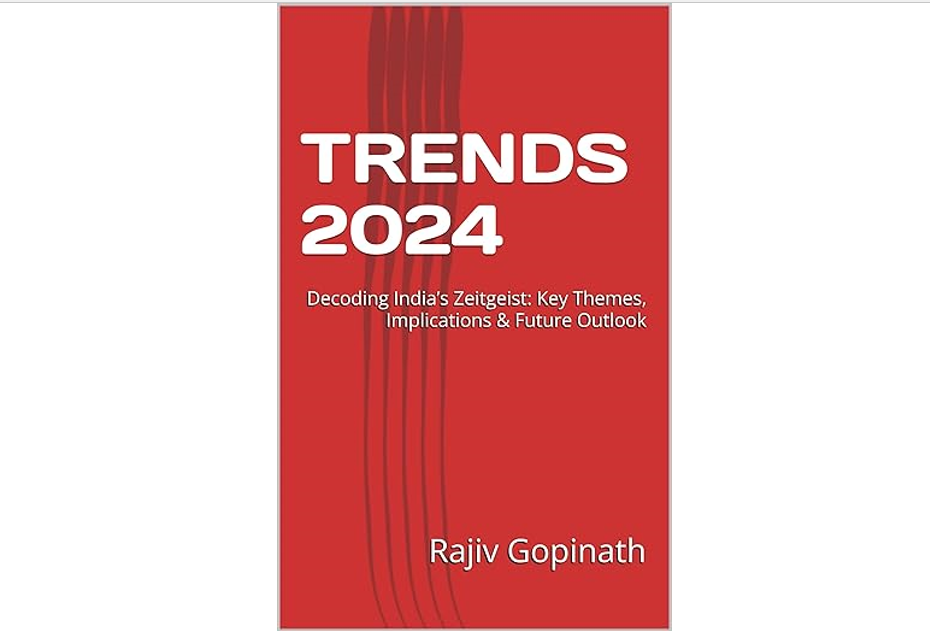
TRENDS 2024: Decoding India’s Zeitgeist: Key Themes, Implications & Future Outlook
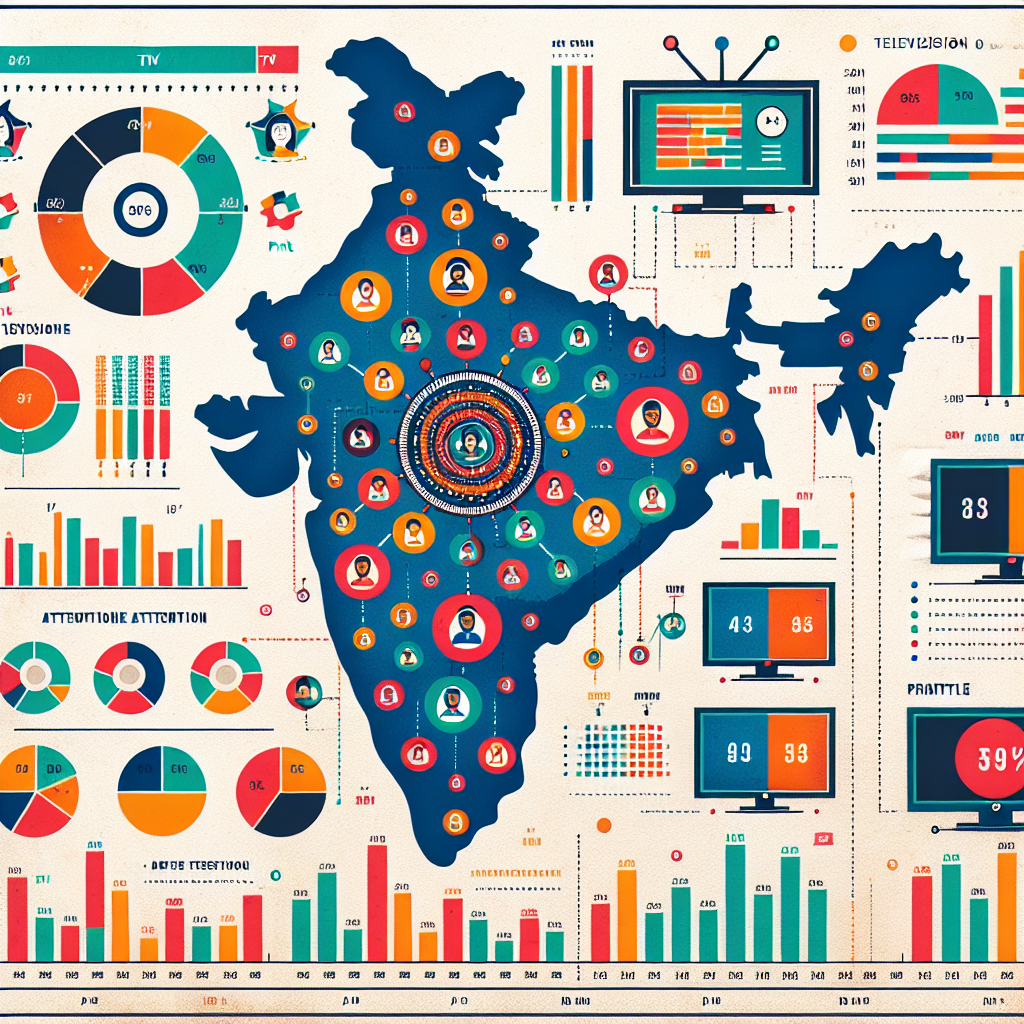
How to better quantify attention in TV and Print in India
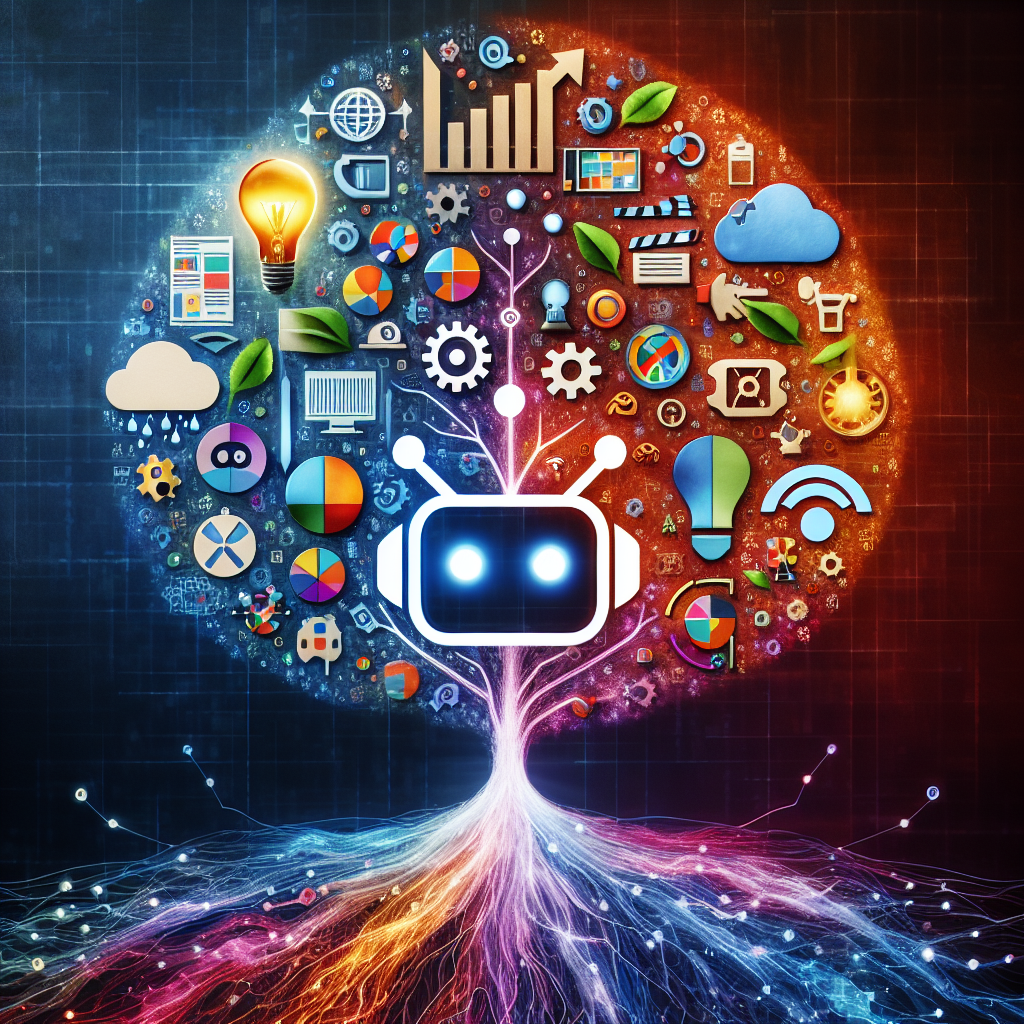
AI in media agencies: Transforming data into actionable insights for strategic growth
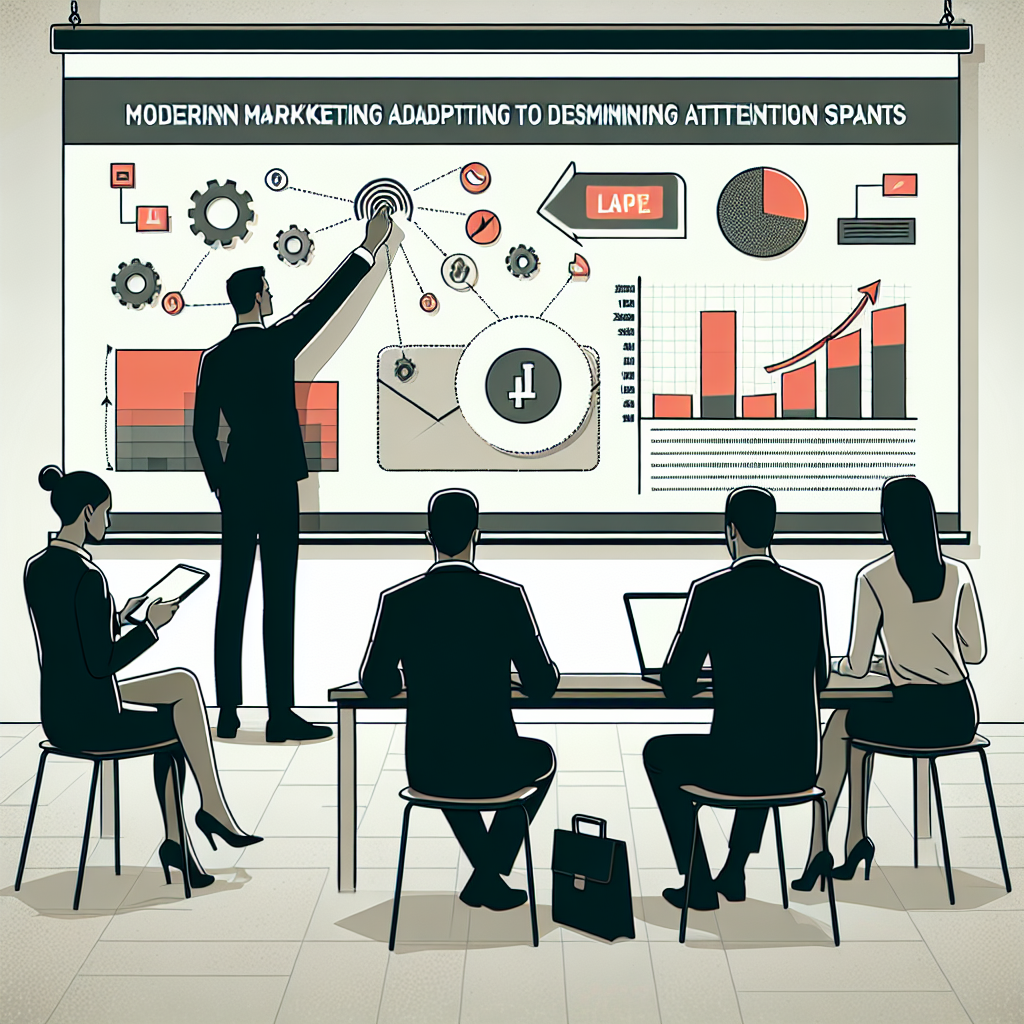
How the Attention Recession Is Changing Marketing
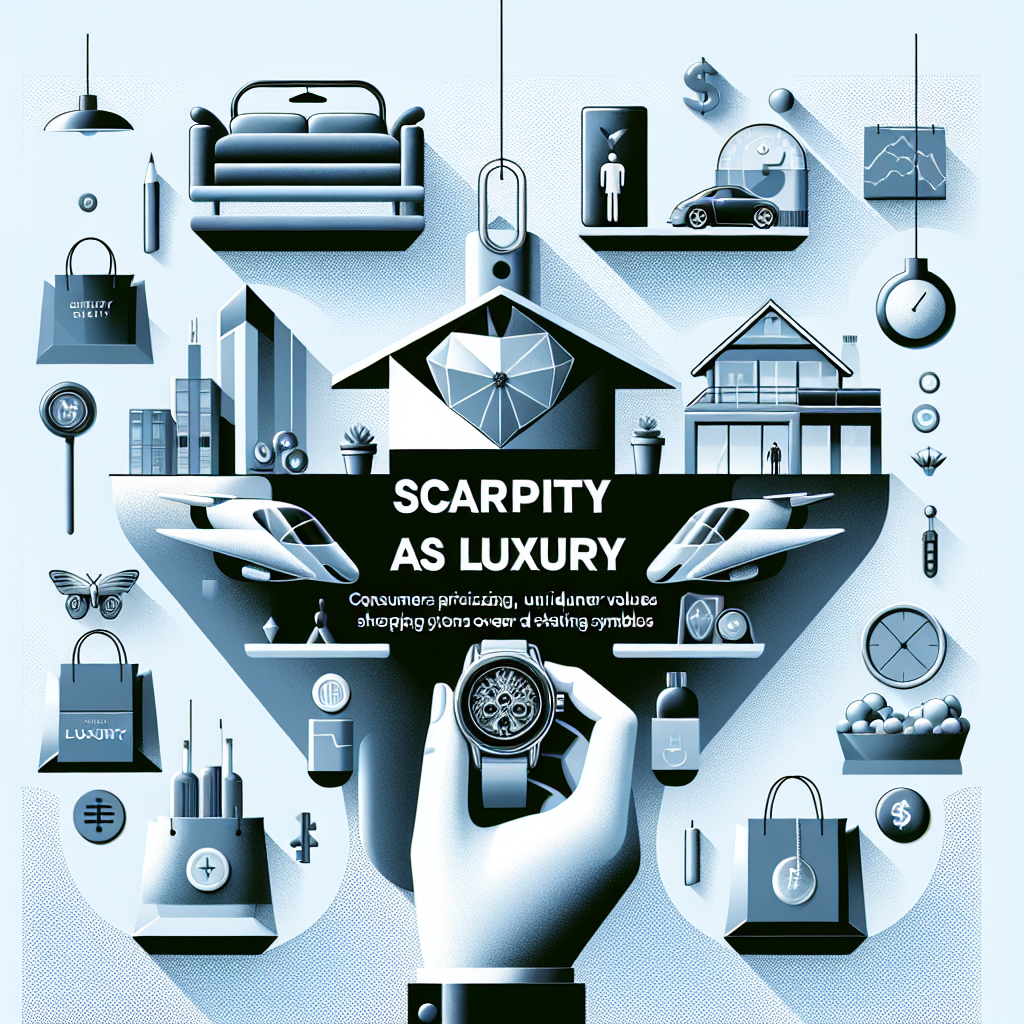
The New Luxury Why Consumers Now Value Scarcity Over Status
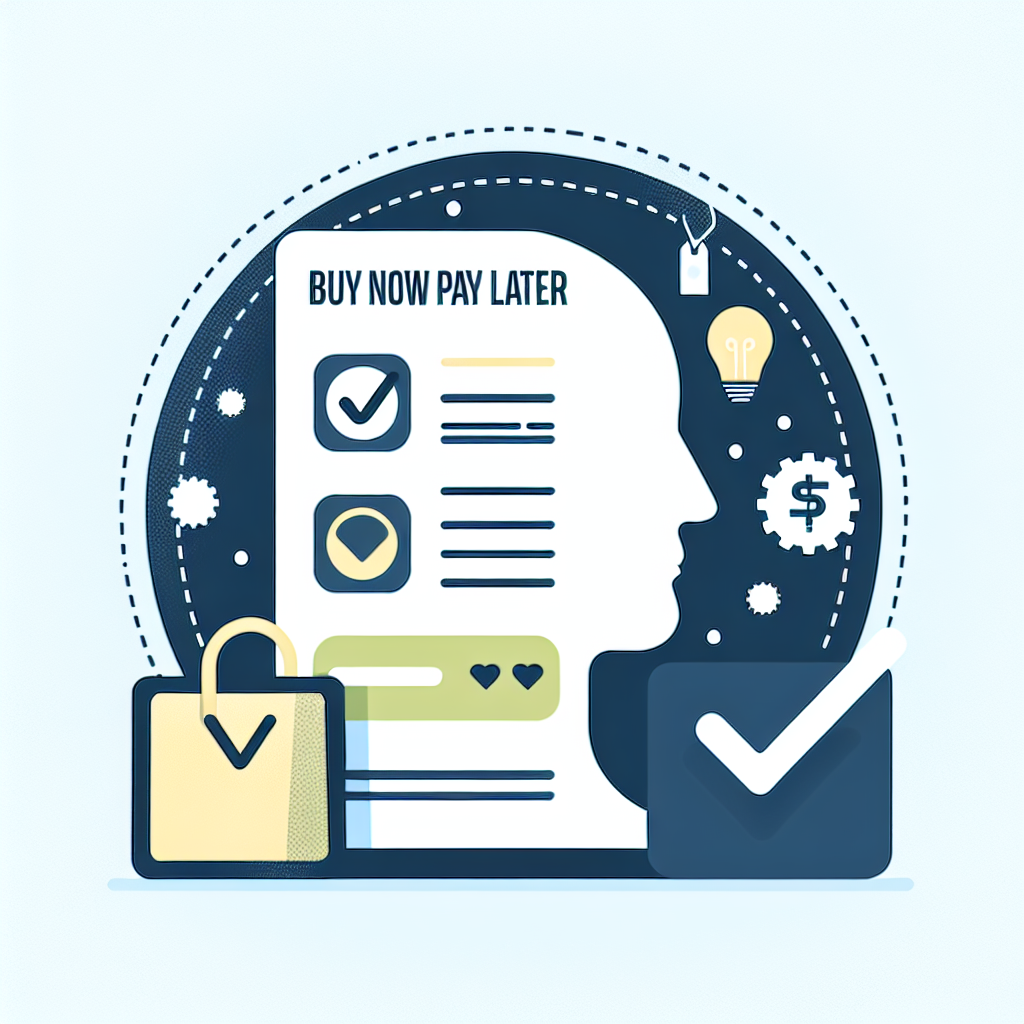
The Psychology Behind Buy Now Pay later
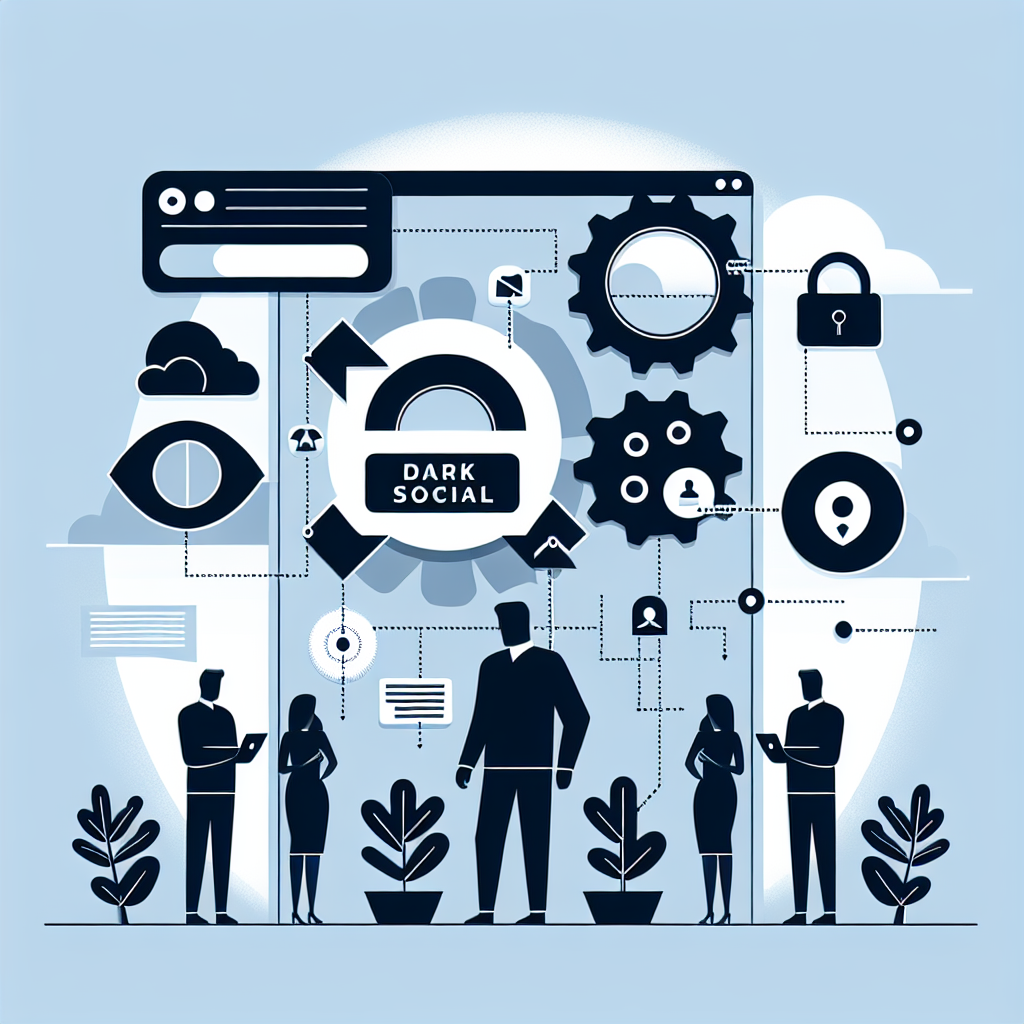
The Rise of Dark Social and Its Impact on Marketing Measurement
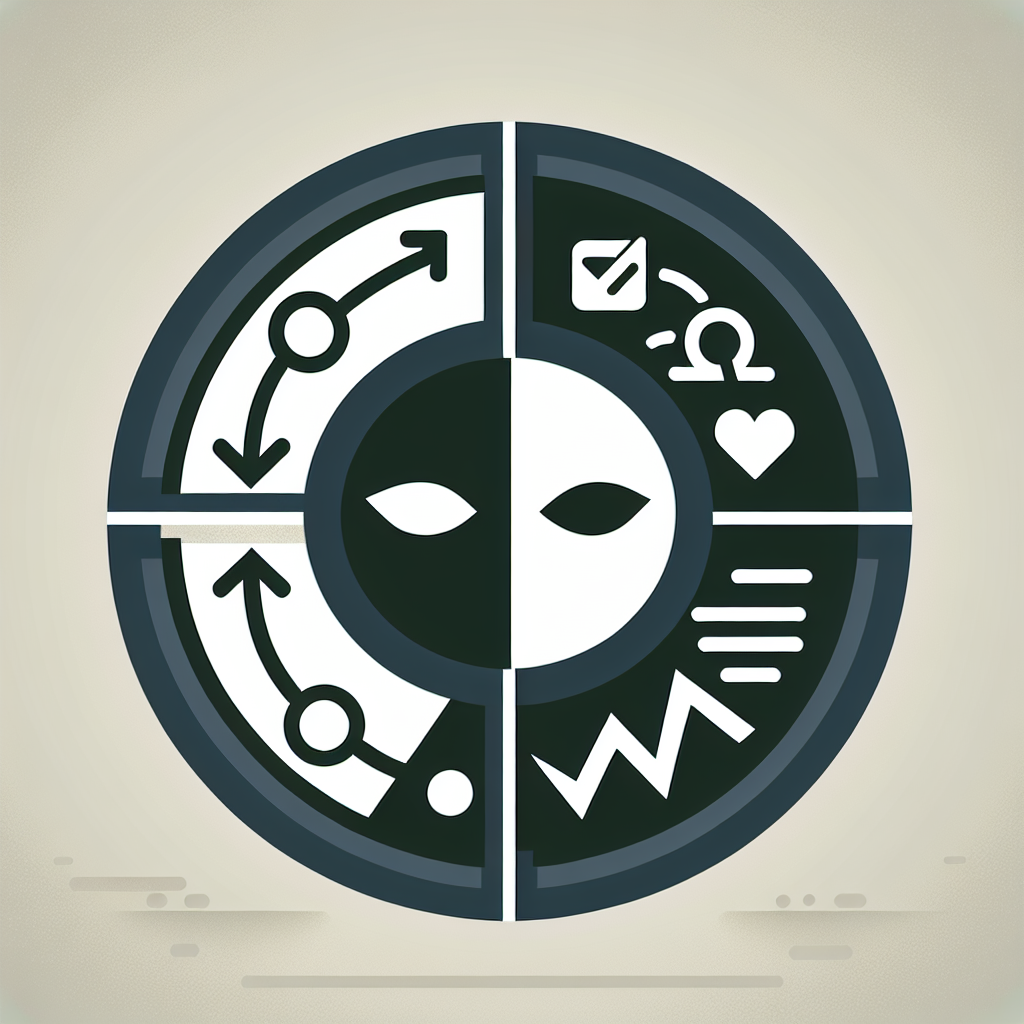
The Role of Dark Patterns in Digital Marketing and Ethical Concerns
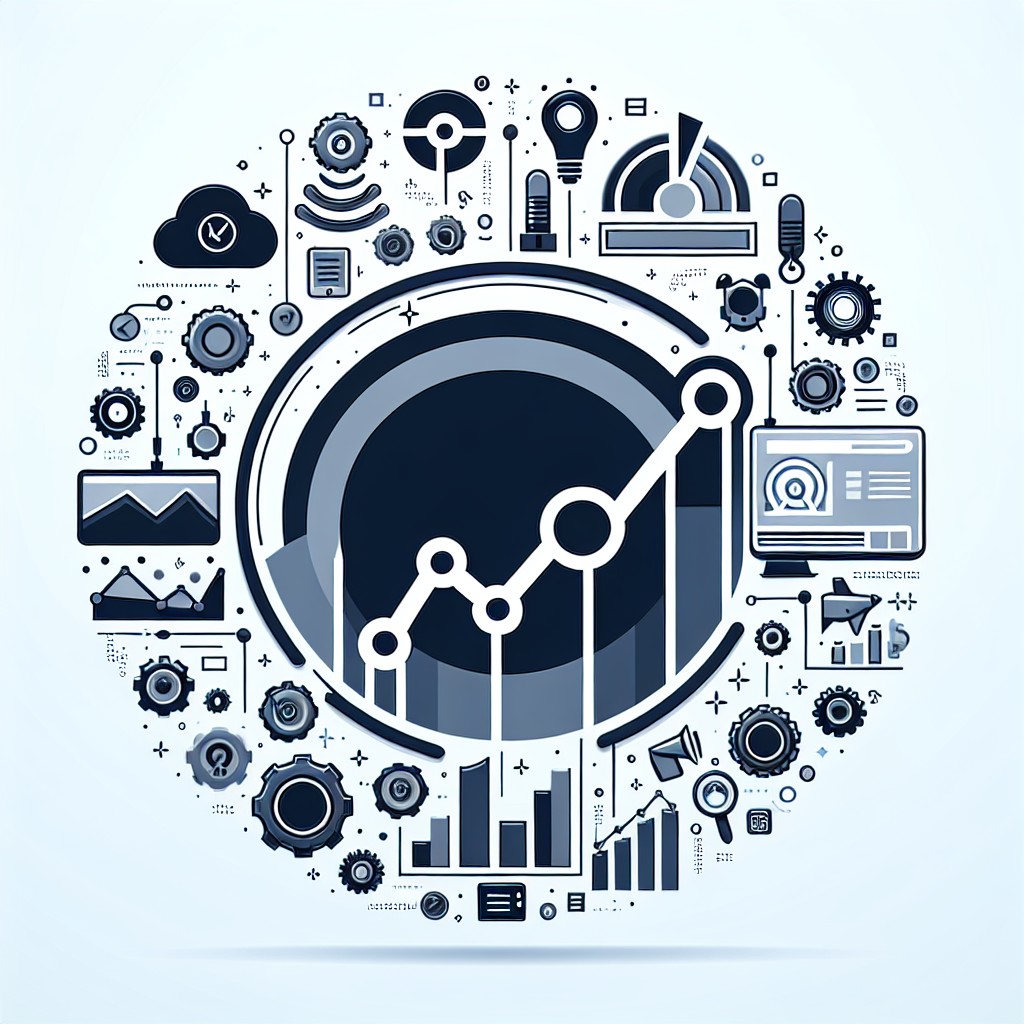