Predictive Analytics for Campaign Planning
The realization dawned on Arun during their quarterly campaign planning session. Despite having a sophisticated marketing automation platform and extensive customer data, they were still planning campaigns based primarily on historical performance and intuition. As the team debated which customer segments to target with their limited budget, Arun asked a simple question: "Instead of guessing which customers might convert, couldn't we build a model to predict it?" The room fell silent. That afternoon, Arun met with the data science team to explore what predictive capabilities might be possible with their existing data. Within weeks, they had developed basic predictive models that transformed their campaign planning process—shifting resources toward high-probability conversions and away from segments with a low likelihood of response. This experience launched Arun's exploration into predictive analytics for campaign planning, revealing how statistical forecasting can dramatically improve marketing resource allocation and campaign performance.
Introduction: The Predictive Revolution in Campaign Planning
Campaign planning has evolved from creative-driven processes to increasingly data-driven strategic exercises. This evolution has progressed through several distinct phases: from demographic targeting to behavioral segmentation, from backwards-looking analytics to predictive forecasting, and now to the frontier of AI-powered campaign optimization that continuously improves through machine learning.
The integration of predictive analytics into campaign planning represents what MIT Sloan Management Review has termed "the transformation from intuitive to algorithmic marketing." In increasingly complex multichannel environments, this approach transforms campaign development from an art based primarily on experience to a balanced discipline that combines creative excellence with computational precision.
Research from Forrester indicates that organizations employing sophisticated predictive approaches achieve 41% higher campaign ROI and 38% improved customer acquisition efficiency compared to those using traditional planning methods. Meanwhile, analysis from the Advertising Research Foundation shows that predictive campaign optimization delivers 3.2x better performance improvement than approaches based solely on historical results.
As marketing technology pioneer Mayur Gupta observes, "Predictive analytics doesn't replace marketing intuition—it augments it by revealing patterns and opportunities that would remain invisible through conventional analysis alone."
1. Lead Scoring
The most sophisticated applications of predictive analytics focus on identifying high-potential customers and prospects.
Multidimensional Lead Value Prediction
Advanced approaches to identifying high-value opportunities:
- Conversion propensity modeling techniques
- Revenue potential forecasting methodologies
- Cost-to-acquire prediction frameworks
- Lifetime value trajectory analysis
Example: Software company Salesforce developed a "Predictive Opportunity Scoring" system that analyzes thousands of historical lead interactions to predict conversion likelihood and potential deal size. Their model revealed unexpected indicators—discovering that companies rapidly adding users to free trials converted at 3.7x higher rates and purchased 2.4x larger contracts than those with steady trial usage, reshaping their campaign targeting strategy.
Engagement Sequence Prediction
Determining optimal paths to conversion:
- Next-best-action prediction frameworks
- Engagement pathway modeling
- Conversion milestone sequence analysis
- Interaction velocity impact measurement
Example: Marketing automation platform HubSpot implements "Engagement Sequencing Intelligence" that predicts the most effective series of touchpoints for different customer segments. Their predictive system identified that enterprise prospects who downloaded technical documentation before attending webinars converted at 47% higher rates than those following the reverse path—insights that transformed their campaign workflows.
2. Churn Likelihood
Predictive approaches have revolutionized retention marketing through advanced warning systems.
Early Churn Indicator Identification
Detecting subtle signals of potential defection:
- Pre-churn behavior pattern recognition
- Engagement decay trajectory analysis
- Competitive vulnerability scoring
- Satisfaction erosion prediction models
Example: Subscription service HelloFresh created a "Churn Prevention Intelligence" system that identifies subscribers likely to cancel up to 45 days before they actively consider cancellation. Their model discovered that subtle changes in meal selection behaviors—specifically, increasing selection of simpler recipes—preceded cancellation by an average of 37 days, creating a critical intervention window that improved retention by 28%.
Intervention Effectiveness Prediction
Forecasting which retention approaches will succeed:
- Rescue offer response probability modeling
- Retention message receptivity scoring
- Intervention timing optimization
- Retention effort ROI prediction
Example: Wireless carrier T-Mobile developed a "Retention Intervention Optimization" system that predicts not just which customers might leave but which specific retention offers would most effectively prevent individual customers from cancelling. This approach increased retention offer acceptance by 41% while reducing the average incentive cost by 23% by matching offers to specific churn risk factors.
3. Next-Best-Offer Systems
Predictive analytics has transformed cross-sell and upsell campaign planning.
Product Affinity Prediction
Forecasting product interest beyond obvious connections:
- Non-obvious correlation discovery
- Sequential purchase pattern analysis
- Product compatibility scoring
- Need state prediction frameworks
Example: E-commerce retailer Wayfair implements "Discovery Prediction" technology that identifies unexpected product affinities based on subtle behavioral patterns rather than just category relationships. Their system revealed that customers purchasing certain lighting fixtures were 5.2x more likely to purchase specific area rugs within 30 days—an insight with no obvious causal connection but strong predictive value that transformed their cross-selling campaigns.
Optimal Offer Timing Prediction
Determining when customers are most receptive:
- Purchase cycle prediction models
- Life event anticipation frameworks
- Receptivity window identification
- Timing optimization algorithms
Example: Financial services company Capital One developed a "Next-Best-Moment" system that predicts not just which offers might interest customers but precisely when those offers should be presented. Their analysis revealed that credit limit increase acceptance was 37% higher when offered 7-10 days after a customer's primary direct deposit rather than at month-end—timing precision that significantly improved campaign performance.
Conclusion: The Predictive Future of Campaign Planning
As noted by marketing scientist Kevin Hillstrom, "Modern marketing is increasingly about predicting what will happen rather than simply analyzing what did happen." For marketing leaders, this insight suggests that predictive capabilities may be the key to achieving sustainable competitive advantage in increasingly crowded marketplaces.
The integration of predictive analytics into campaign planning represents more than just technical innovation—it fundamentally transforms how organizations conceive, develop, and optimize their marketing investments.
Featured Blogs
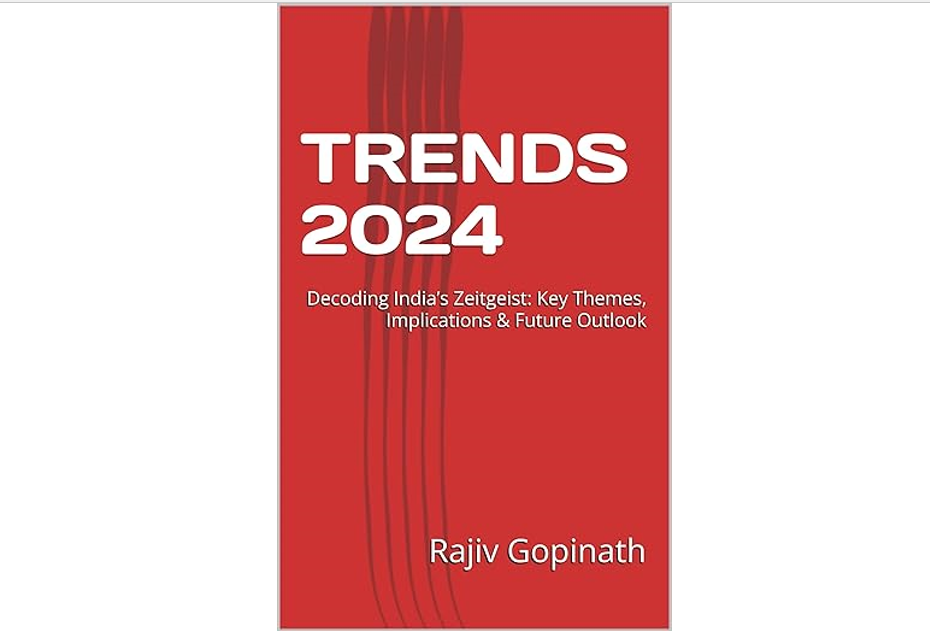
TRENDS 2024: Decoding India’s Zeitgeist: Key Themes, Implications & Future Outlook
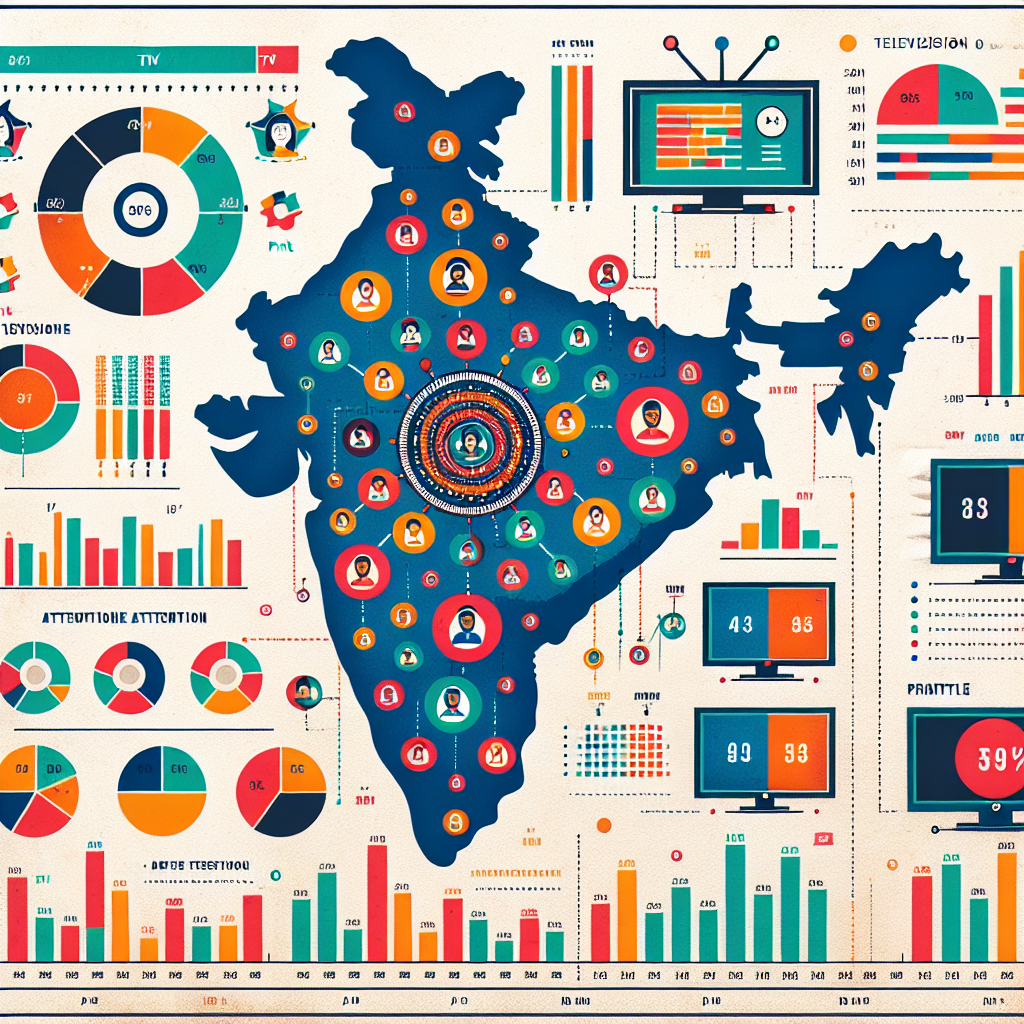
How to better quantify attention in TV and Print in India
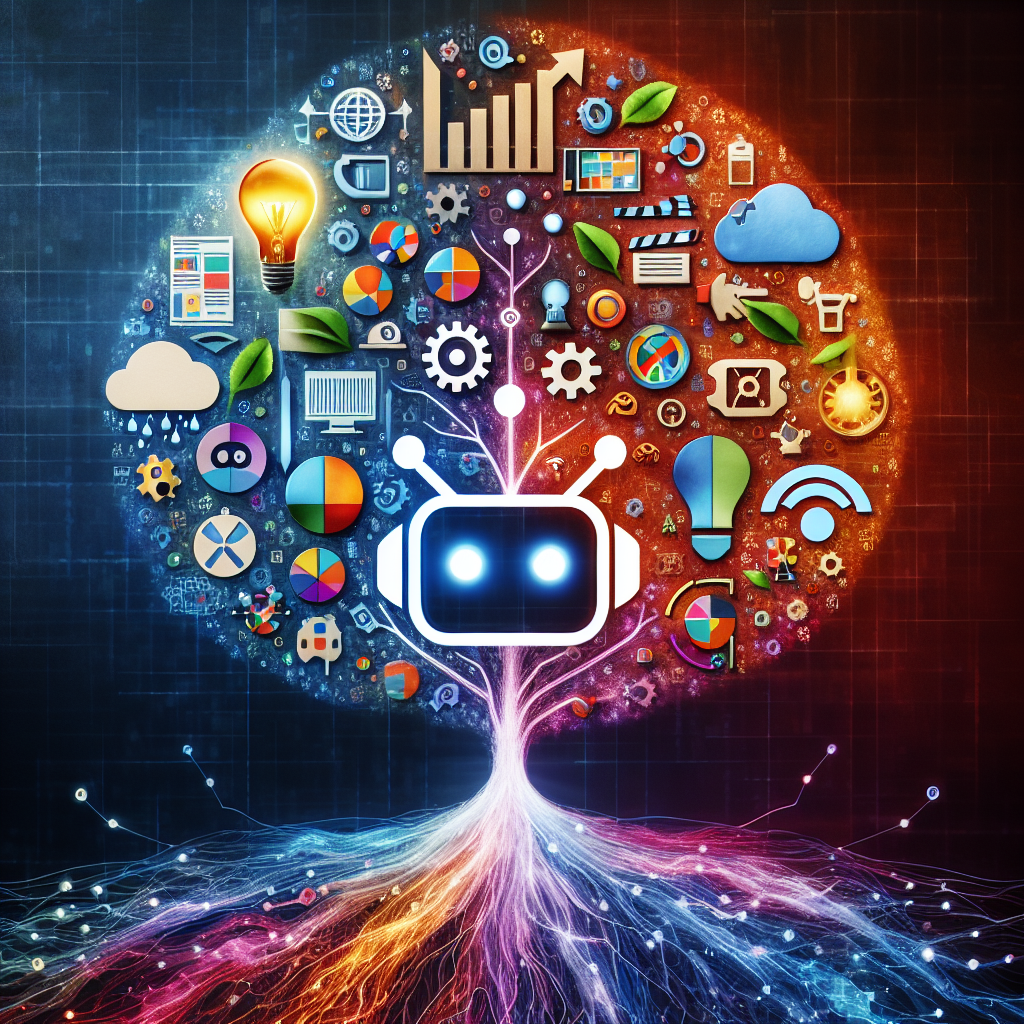
AI in media agencies: Transforming data into actionable insights for strategic growth
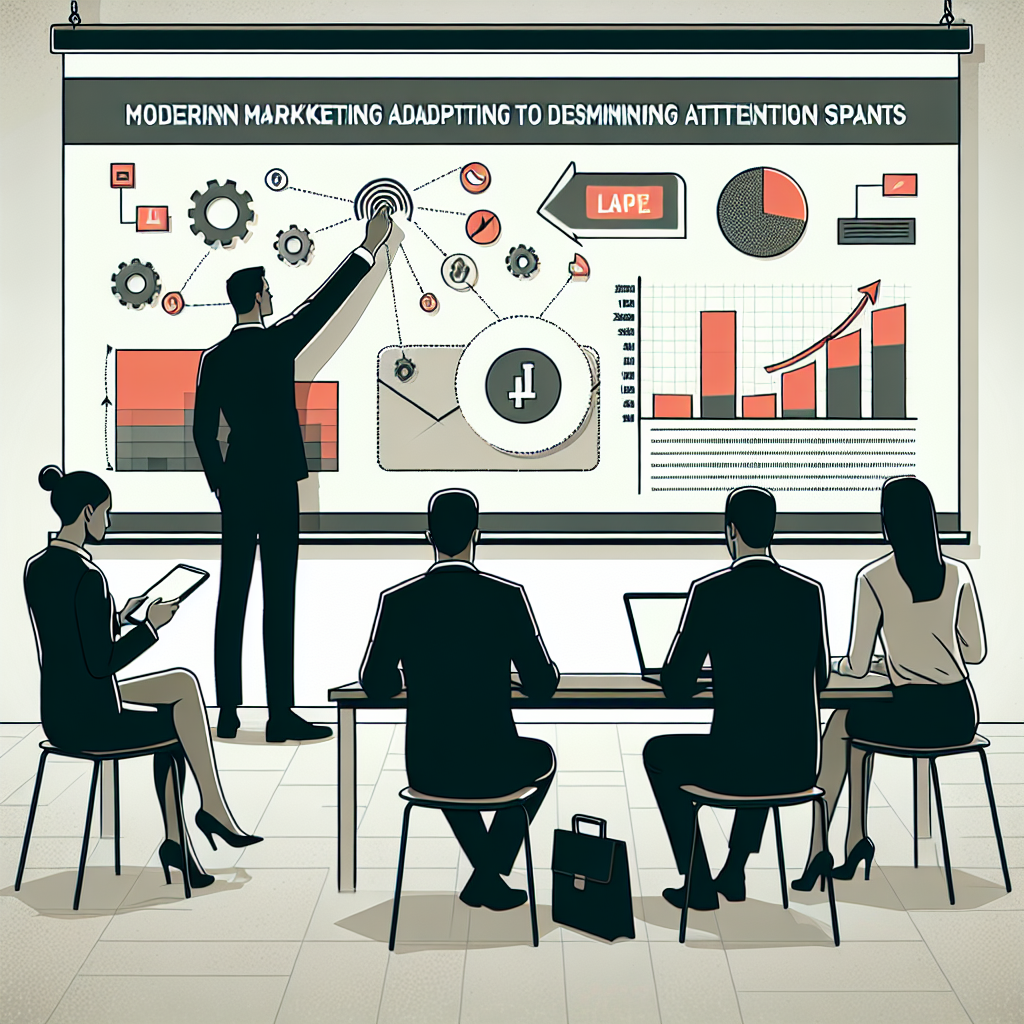
How the Attention Recession Is Changing Marketing
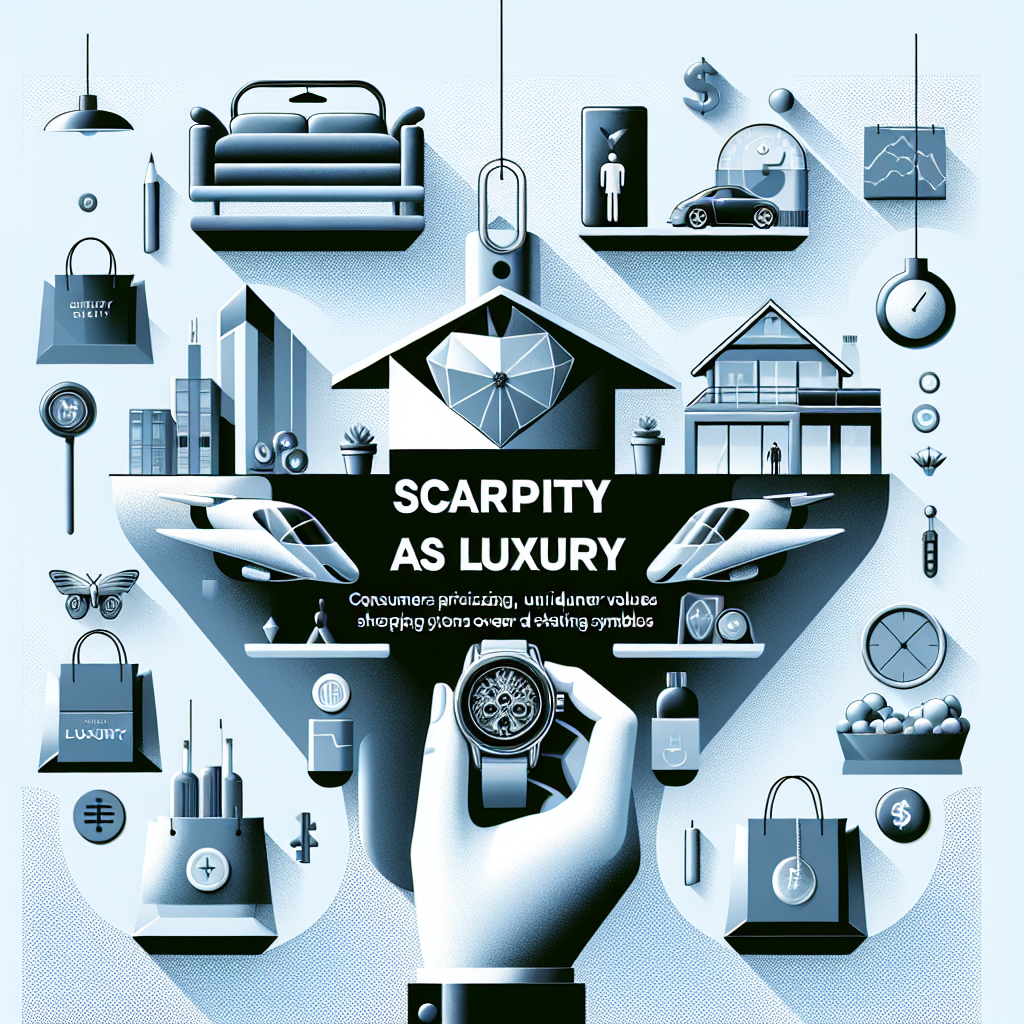
The New Luxury Why Consumers Now Value Scarcity Over Status
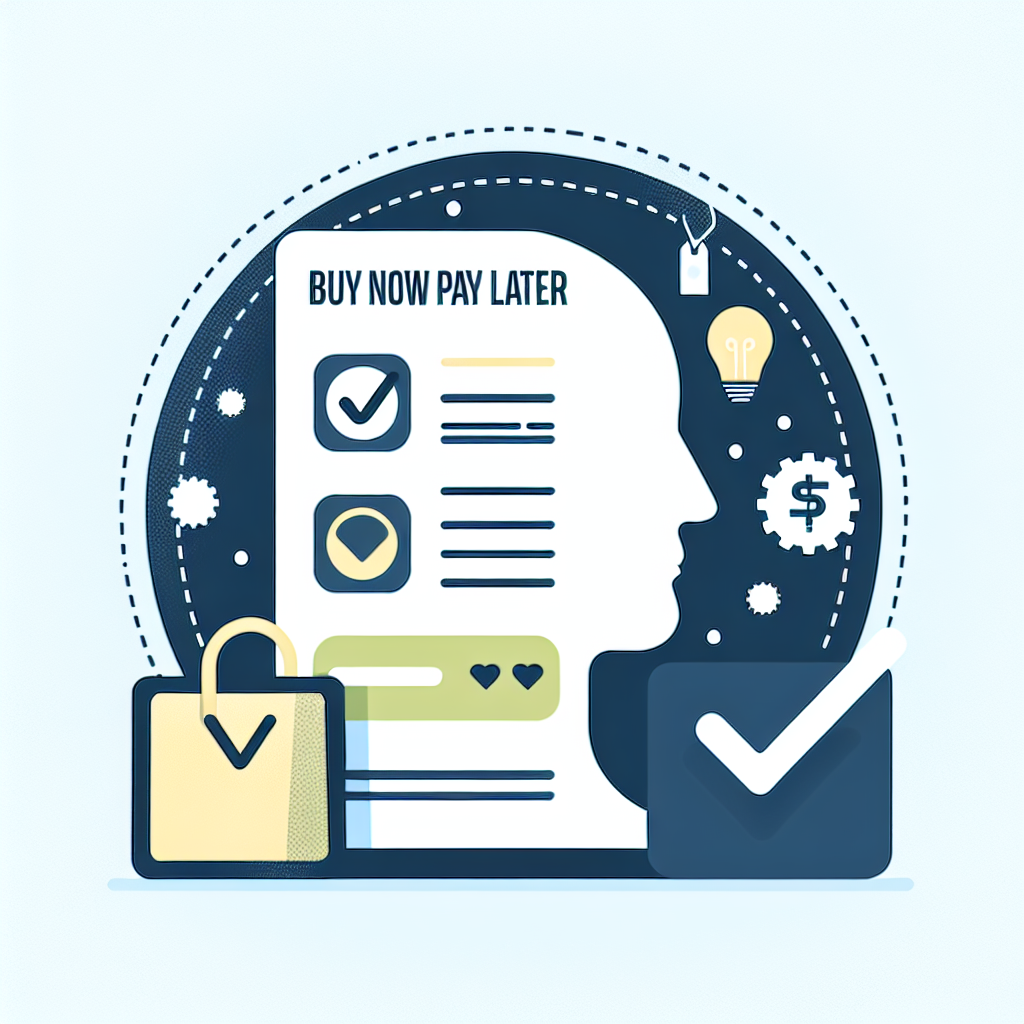
The Psychology Behind Buy Now Pay later
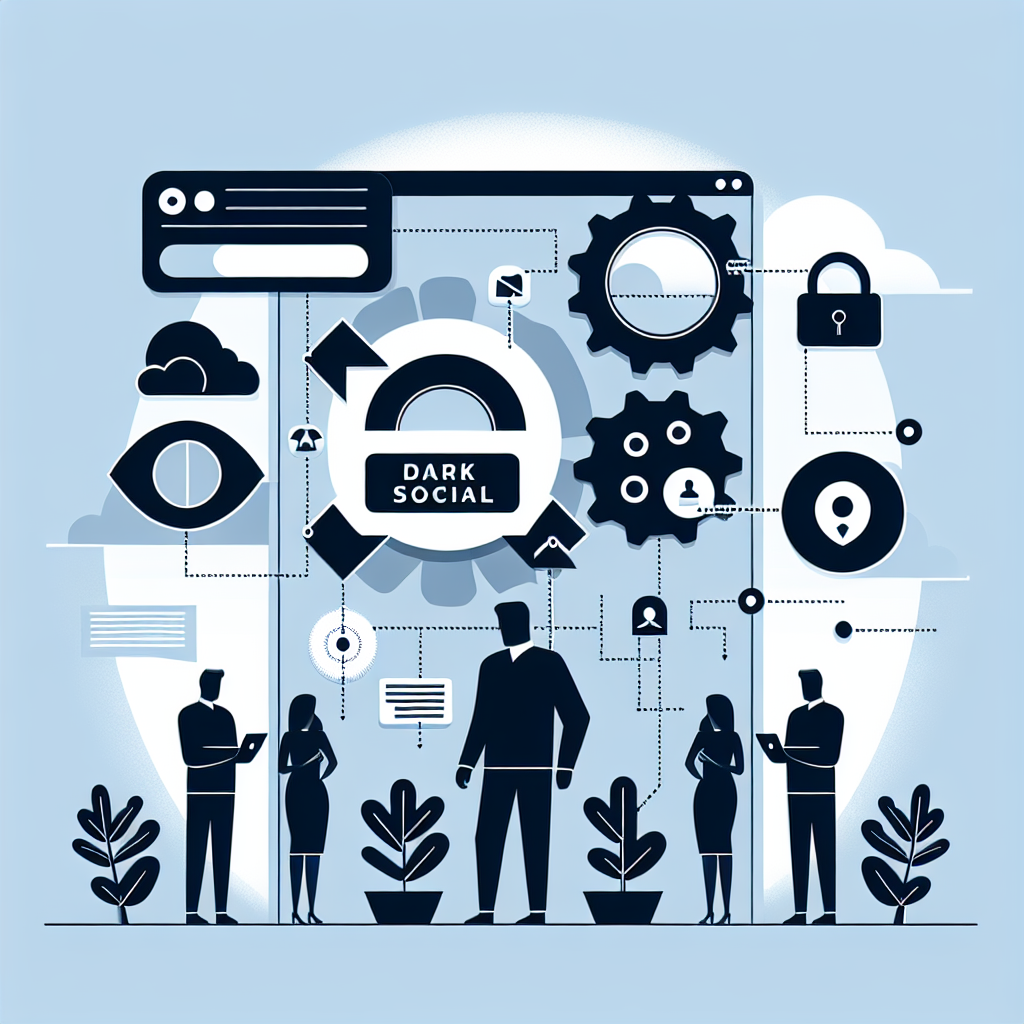
The Rise of Dark Social and Its Impact on Marketing Measurement
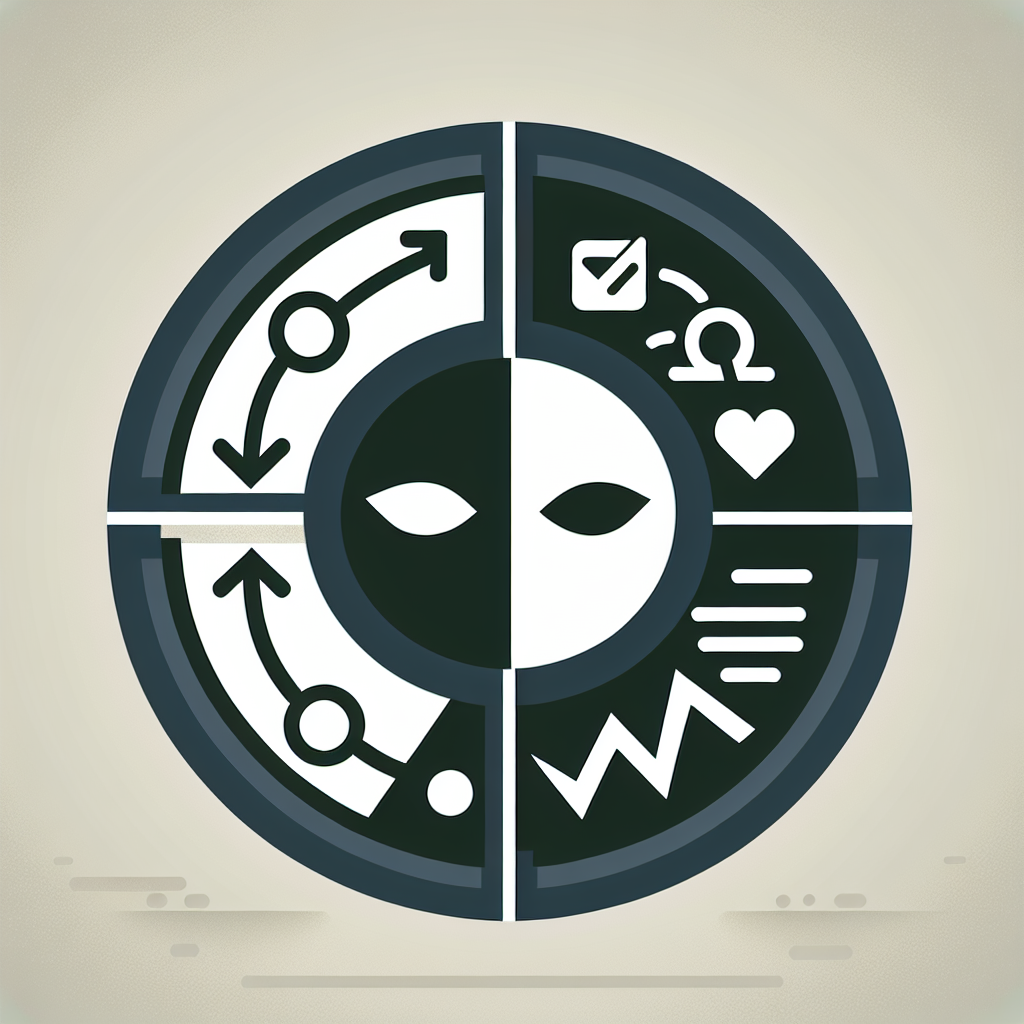
The Role of Dark Patterns in Digital Marketing and Ethical Concerns
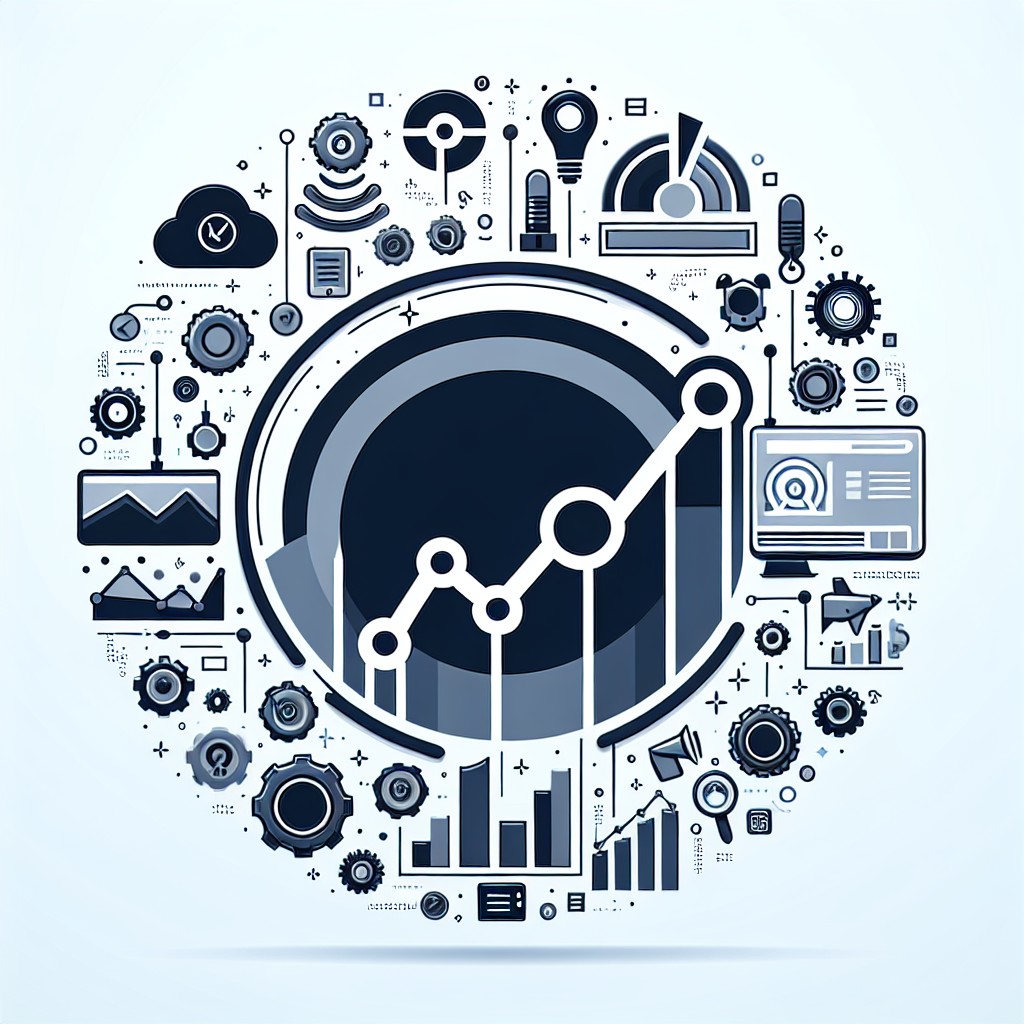