Segmentation via Data Science
The revelation came to Arun during a routine review of their customer database. For years, their audience had been segmented using basic demographic categories—age, location, industry—with only modest success. That afternoon, while exploring patterns in the purchase data, Arun noticed something striking: customers who appeared identical demographically displayed radically different buying behaviors and lifetime values. On impulse, he analyzed their purchase recency, frequency, and monetary value collectively, revealing distinct behavioral clusters hidden within what seemed to be homogeneous segments. Implementing campaigns targeted to these behavioral patterns rather than demographics yielded immediate results—a 47% increase in response rates and 31% higher average order values. What began as casual data exploration transformed into Arun's passionate pursuit of advanced segmentation methodologies. Traditional categories had masked crucial behavioral differences driving actual business value. This experience launched Arun's deep dive into data science approaches to customer segmentation, revealing how multidimensional analysis could transform marketing effectiveness beyond superficial demographic classifications.
Introduction: The Scientific Revolution in Customer Segmentation
Customer segmentation has evolved from broad demographic categorization to sophisticated behavioral clustering. This evolution progressed through distinct phases: from intuition-based grouping to demographic analysis, from static customer categories to dynamic behavioral segments, and now to the frontier of multidimensional segmentation frameworks powered by machine learning that continuously refine customer understanding.
The integration of data science into segmentation—methodologies that identify meaningful patterns across multiple dimensions of customer behavior—represents what the Marketing Science Institute has identified as "the fundamental prerequisite for personalization at scale." In marketing contexts, this approach transforms superficial customer groupings into meaningful behavioral insights, replacing assumptions with evidence.
Research from the Customer Data Platform Institute indicates that organizations with advanced segmentation capabilities achieve 42% higher campaign response rates and 37% improved customer lifetime value compared to those using traditional approaches. Meanwhile, analysis published in the Journal of Marketing Analytics found that companies with sophisticated behavioral segmentation demonstrate 3.2x higher marketing ROI.
1. RFM Modeling
The foundation of behavioral segmentation begins with recency, frequency, and monetary value analysis.
a) Multidimensional Customer Value Assessment
Modern RFM frameworks incorporate nuanced approaches:
- Purchase recency decay curve analysis
- Engagement frequency pattern identification
- Monetary value normalization methods
- Cross-channel behavior integration
Case Study: E-commerce retailer Wayfair implemented a comprehensive RFM segmentation framework that identified their highest-value customer segment represented just 13% of their customer base but generated 47% of revenue. Further analysis revealed these customers shared distinctive browsing patterns before purchase, enabling targeted acquisition of similar high-value prospects and increasing new customer value by 27%.
b) Predictive Churn Identification
Sophisticated RFM applications predict future behavior:
- Early warning indicator development
- Engagement trajectory analysis
- Purchase interval pattern recognition
- Reactivation potential scoring
Case Study: Subscription service HelloFresh developed a predictive RFM model that identified subtle pre-cancellation behavioral patterns six weeks before customers typically unsubscribed. This early identification enabled proactive retention campaigns that reduced churn by 23% among targeted segments, dramatically improving customer lifetime value.
c) Cross-Sell Opportunity Mapping
Advanced RFM models identify product affinity patterns:
- Category migration path analysis
- Product adoption sequence identification
- Price sensitivity variation mapping
- Brand loyalty progression tracking
Case Study: Beauty retailer Sephora applied RFM analysis across product categories, discovering that customers who purchased skincare products within specific price ranges were 64% more likely to adopt premium cosmetics within 60 days. This insight enabled targeted cross-category campaigns that increased average revenue per customer by 31%.
2. Value-Based Cohorting
Effective segmentation strategies group customers by potential lifetime value.
a) Future Value Prediction Models
Modern value-based segmentation incorporates predictive dimensions:
- Customer lifetime value projection methodologies
- Retention probability assessment
- Share-of-wallet estimation
- Referral value inclusion
Case Study: Financial services company American Express implemented a value-based segmentation approach that assigned customers to cohorts based on projected three-year value rather than current spending. This forward-looking approach identified emerging high-value segments that were previously overlooked, enabling targeted retention efforts that increased high-potential customer retention by 34%.
b) Investment Optimization Frameworks
Value-based segmentation enables strategic resource allocation:
- Segment-specific acquisition cost targets
- Retention investment optimization
- Service level differentiation models
- Marketing budget allocation frameworks
Case Study: Telecommunications provider T-Mobile developed a value-based investment optimization model that reallocated marketing and service resources based on customer lifetime value projections. This approach increased high-value segment retention by 29% while reducing overall acquisition costs by selectively decreasing investment in low-value segments with high churn propensity.
c) Experience Personalization Matrices
Sophisticated value segmentation drives experience differentiation:
- Channel preference optimization
- Communication frequency calibration
- Offer sensitivity modeling
- Loyalty program tiering strategies
Case Study: Hotel chain Marriott implemented value-based experience personalization that adjusted everything from check-in procedures to communication frequency based on lifetime value projections. This approach increased high-value guest satisfaction scores by 26% while simultaneously reducing operational costs by aligning service investments with customer potential.
3. Lookalike Targeting
Advanced analytics enable precision prospecting through similarity modeling.
a) Multi-Signal Profile Development
Modern lookalike approaches incorporate diverse data inputs:
- Behavioral attribute weighting methodologies
- Contextual signal integration
- Intent indicator identification
- Engagement pattern recognition
Case Study: Fitness brand Peloton developed a sophisticated lookalike modeling approach that incorporated over 50 distinct signals from their existing high-value customer base. This multidimensional profile enabled them to identify acquisition prospects with 47% higher conversion rates and 36% higher first-year value compared to their previous demographic targeting approach.
b) Cross-Platform Audience Extension
Sophisticated lookalike systems function across digital ecosystems:
- Seed audience optimization strategies
- Platform-specific signal adaptation
- Expansion rate calibration methods
- Performance feedback loop implementation
Case Study: Clothing retailer Everlane implemented cross-platform lookalike targeting that extended their customer profile across multiple advertising platforms. By optimizing their seed audience composition and expansion parameters, they achieved 39% lower customer acquisition costs while maintaining consistent post-purchase value metrics.
c) Offline-to-Online Extension Models
Advanced lookalike approaches bridge physical and digital contexts:
- Geographic behavior pattern mapping
- Retail transaction similarity modeling
- Online-offline behavior correlation analysis
- Channel migration prediction
Case Study: Retailer Target developed a sophisticated offline-to-online lookalike system that identified digital acquisition prospects with similar characteristics to their high-value in-store customers. This approach created 28% higher omnichannel purchase rates among new customers compared to their previous digital acquisition strategies.
Conclusion: The Strategic Impact of Advanced Segmentation
As data science capabilities continue advancing, customer segmentation is evolving beyond basic categorization toward multidimensional understanding that drives personalized experiences at scale. The organizations achieving breakthrough performance are those moving beyond demographic proxies toward behavioral intelligence that reveals why customers act as they do.
The integration of machine learning into segmentation enables previously impossible pattern recognition, identifying subtle behavioral clusters that predict future value while enabling truly personalized marketing approaches. These systems reduce the gap between customer needs and organizational offerings while simultaneously increasing marketing efficiency through precision targeting.
As these methodologies mature, segmentation is increasingly recognized as a strategic capability rather than a marketing tactic, creating unprecedented opportunities for differentiation through deep customer understanding.
Call to Action
For marketing leaders seeking to establish segmentation excellence:
- Implement data infrastructures that capture behavioral signals beyond transactions
- Develop multidimensional segmentation frameworks incorporating recency, frequency, and value
- Establish future value prediction capabilities that guide investment decisions
- Create dynamic segmentation approaches that evolve with customer behavior
- Integrate segmentation insights into product development and experience design processes
The future of marketing effectiveness belongs not to those with the largest databases or the most detailed demographic profiles, but to organizations that systematically translate behavioral patterns into actionable customer intelligence through disciplined, data-driven segmentation strategies.
Featured Blogs
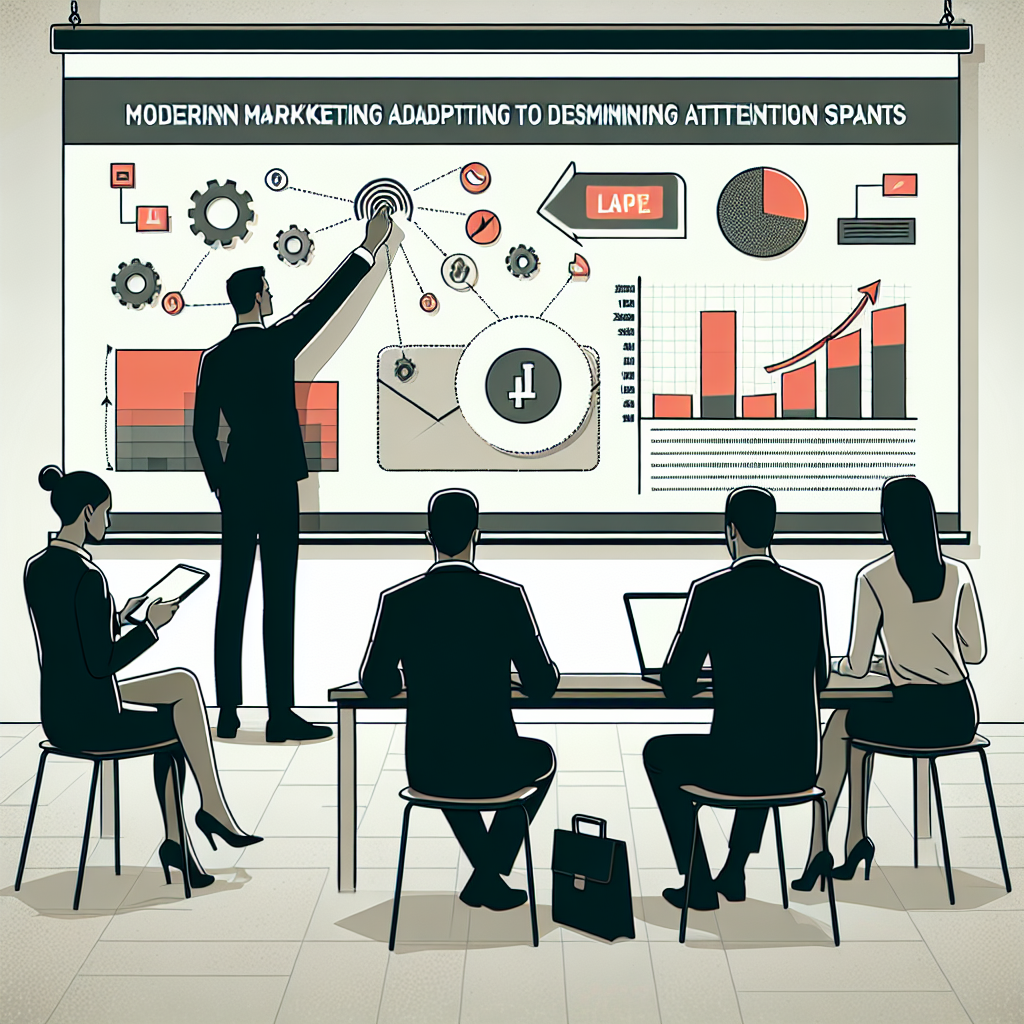
How the Attention Recession Is Changing Marketing
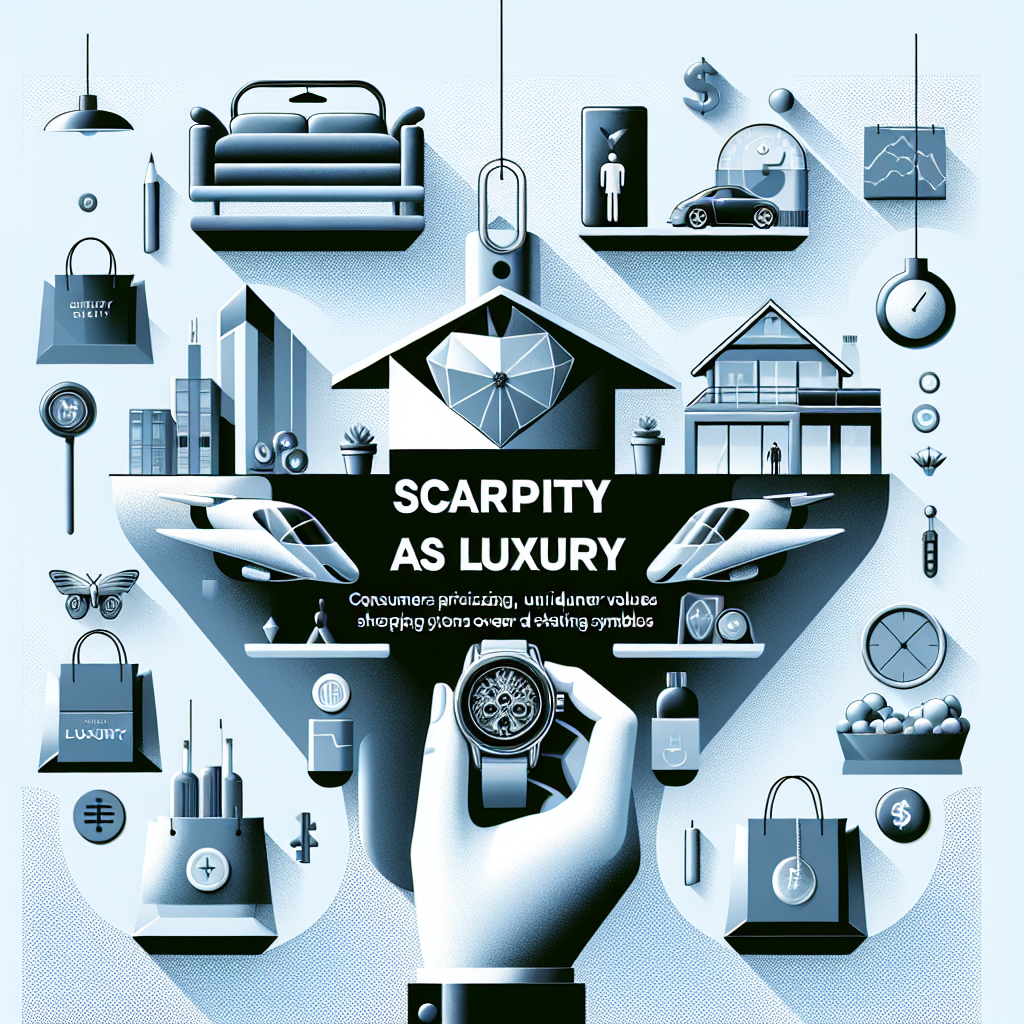
The New Luxury Why Consumers Now Value Scarcity Over Status
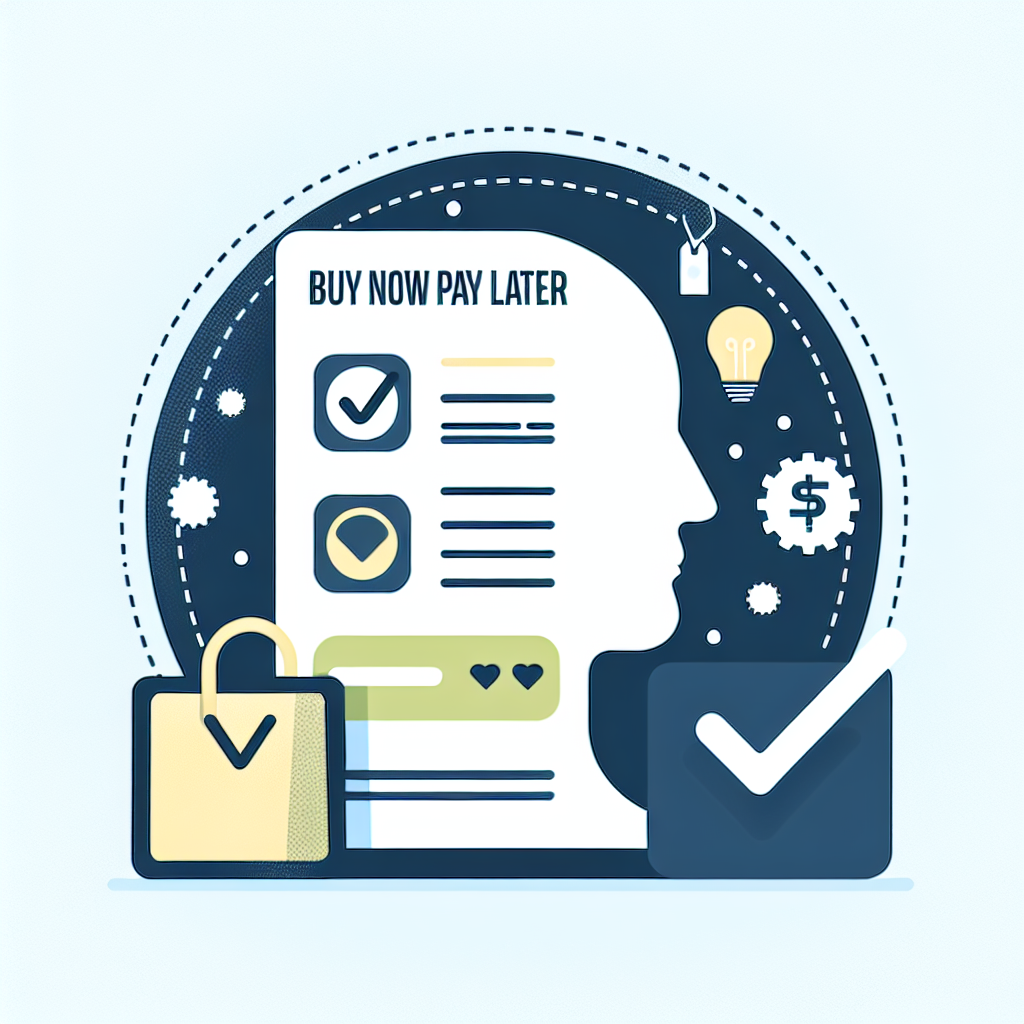
The Psychology Behind Buy Now Pay later
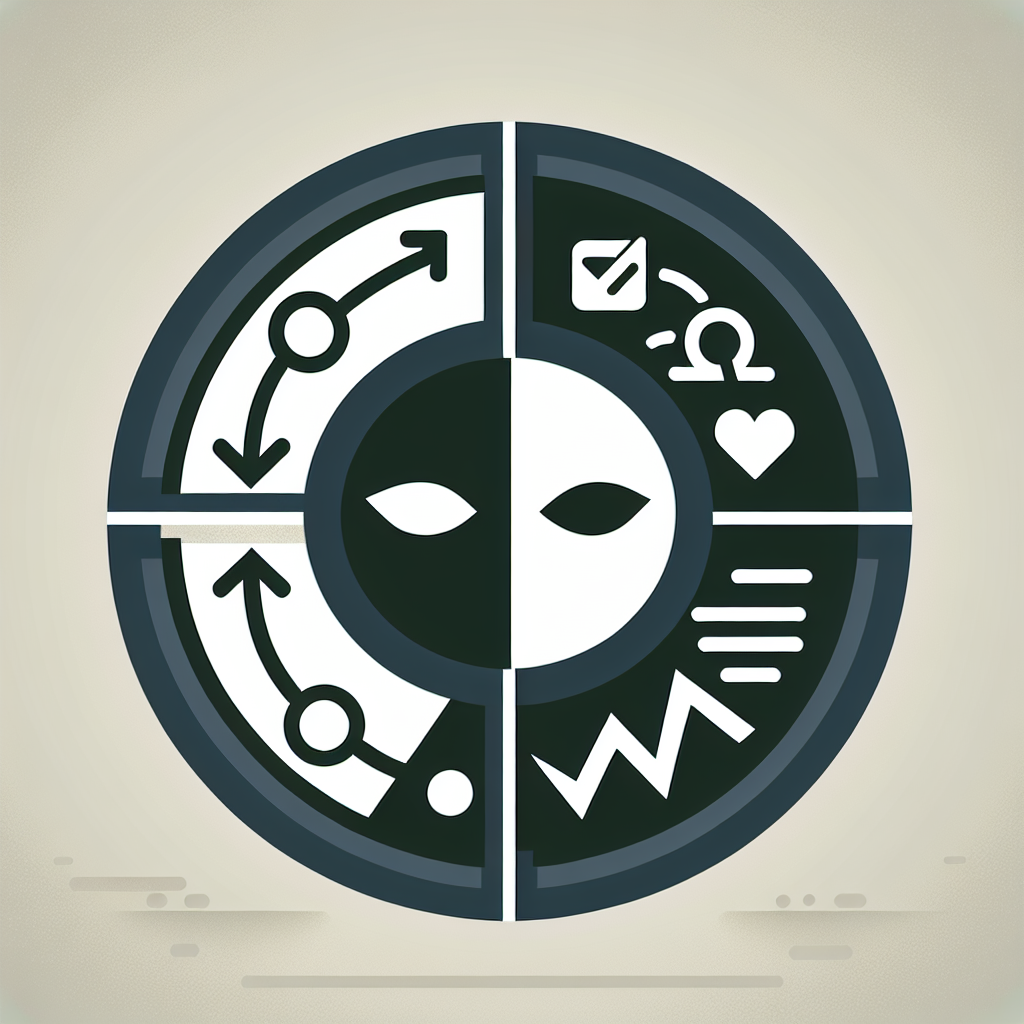
The Role of Dark Patterns in Digital Marketing and Ethical Concerns
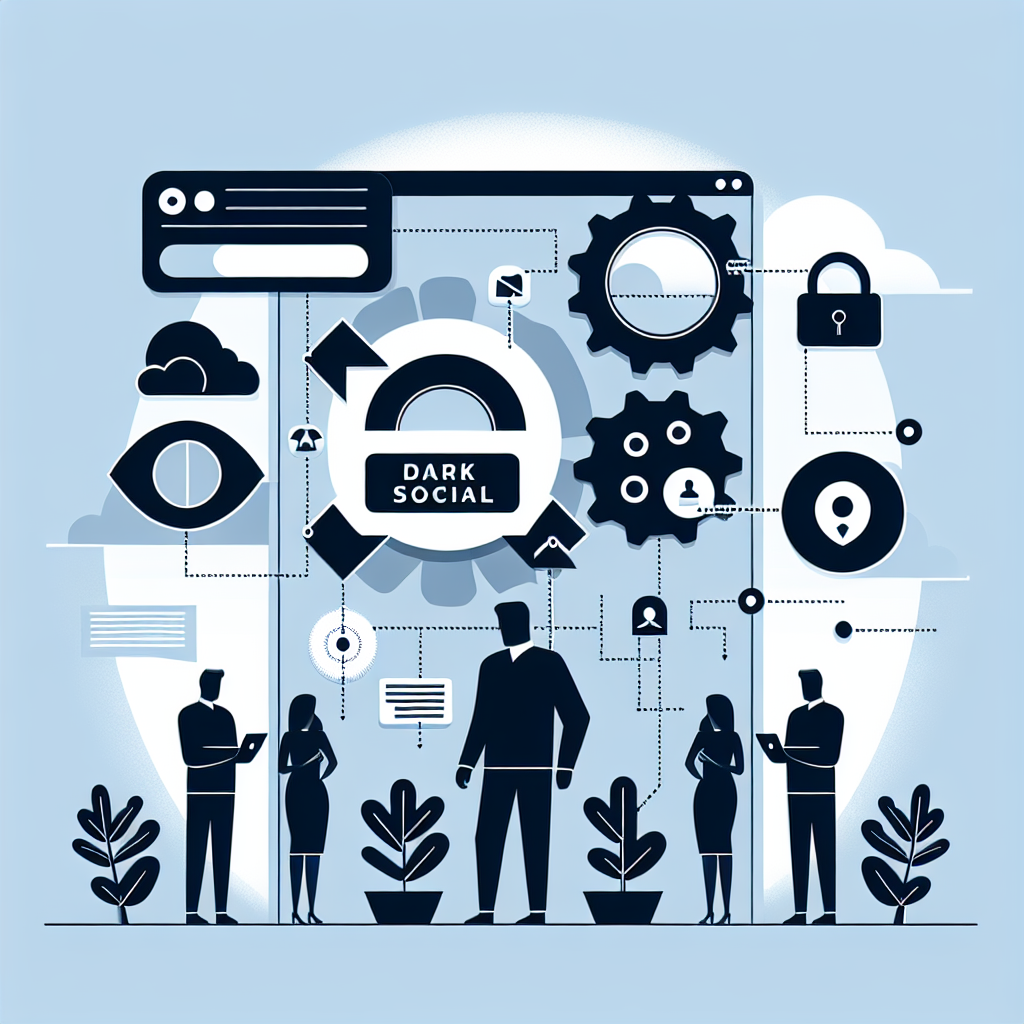
The Rise of Dark Social and Its Impact on Marketing Measurement
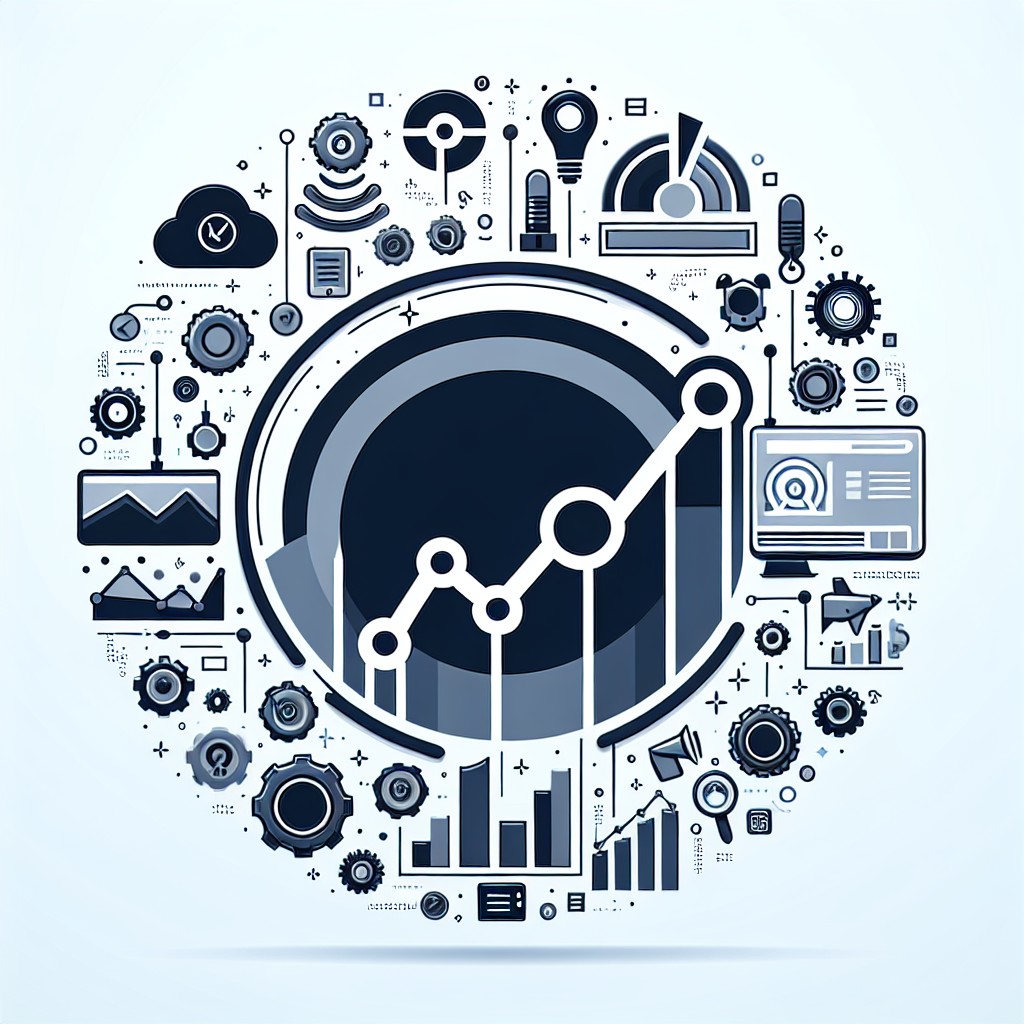