The Role of Media Mix Modeling in a Privacy-First Era
In the aftermath of a particularly contentious marketing review, Jesse's digital team was defending the sudden drop in reported campaign performance. "Our tracking is broken," they insisted, pointing to Apple's privacy changes that had decimated their visibility into customer journeys. That's when their veteran CMO leaned forward with a knowing smile: "This is why I never dismantled our media mix modeling program. When everyone became obsessed with cookies and attribution, I kept investing in these old-school econometric models." She opened a presentation showing how their MMM analysis revealed their true performance hadn't actually declined—they just couldn't see it through their broken attribution system. The digital natives on the team (Jesse included) were shocked. How could this statistical approach from the 1960s suddenly be more accurate than their sophisticated digital attribution? That moment sparked Jesse's journey into understanding how these seemingly outdated models were experiencing a renaissance in the privacy-first era, providing insights that their cookie-dependent systems no longer could.
Introduction: When New Privacy Meets Old Math
The digital marketing ecosystem is experiencing seismic shifts as privacy regulations (GDPR, CCPA), platform changes (Apple's ATT, Google's Privacy Sandbox), and evolving consumer expectations dismantle the tracking infrastructure that powered attribution for over a decade. As cookies crumble and IDFAs disappear, marketers face a profound measurement crisis—just as boards demand more accountability from marketing investments.
Amid this disruption, Media Mix Modeling (MMM)—a statistical approach developed in the 1960s to measure marketing effectiveness—is experiencing an unexpected renaissance. By analyzing the relationship between marketing investments and business outcomes at an aggregate level without requiring individual user tracking, MMM provides a privacy-compliant alternative to increasingly restricted user-level attribution.
As Professor P.K. Kannan of the University of Maryland observes, "We're witnessing the convergence of necessity and capability—privacy changes necessitate alternatives to user-level tracking, while advances in computational power and methods enhance MMM's granularity and speed." This convergence is transforming a half-century-old technique into the centerpiece of modern marketing measurement strategy.
1. The Technical Evolution: Modern MMM vs. Traditional Approaches
Today's MMM bears little resemblance to its historical predecessor, having evolved through three generations of technical advancement:
a) Classical MMM (1960s-2000s)
Traditional models used linear regression to estimate media contributions, with substantial limitations:
- Quarterly or monthly granularity limiting tactical insights
- Limited variable incorporation (typically 5-10 channels)
- Significant lag time (often 3-6 months for results)
b) Enhanced MMM (2010s)
Improved computational capabilities enabled more sophisticated approaches:
- Bayesian techniques incorporating prior knowledge
- Machine learning for detecting non-linear relationships
- Semi-automated model construction reducing time-to-insight
c) AI-Powered MMM (Current)
The latest generation leverages artificial intelligence to overcome historical limitations:
- Automated feature engineering identifying optimal variables
- Neural networks capturing complex cross-channel interactions
- Integration with other measurement approaches in unified frameworks
Mars, Inc. exemplifies this evolution, having transformed their quarterly MMM program into a continuous measurement system delivering insights at weekly granularity. Their Global Media Director reports that "new-generation MMM provided actionable insights during COVID market disruptions when all our attribution models failed due to broken baselines."
2. Strategic Applications: Beyond Channel Allocation
Forward-thinking organizations are deploying MMM beyond its traditional role in budget allocation:
a) Long-Term Brand Investment Justification
MMM quantifies the historically elusive value of brand investments. Diageo credits modern MMM with helping justify a 25% increase in brand investment during economic uncertainty by isolating long-term brand effects from short-term performance metrics.
b) Incrementality Validation
Organizations use MMM as a validation layer to verify incrementality claims from platforms. Professor Brett Gordon of Northwestern University notes, "MMM serves as an essential reality check against increasingly unreliable platform-reported metrics."
c) Scenario Planning
Advanced MMM enables sophisticated scenario planning. During COVID-19, Unilever used MMM-powered simulations to rapidly recalibrate marketing investments as consumer behavior shifted dramatically, maintaining market share while competitors struggled with measurement uncertainty.
3. Implementation Challenges: Beyond Technology
While technically feasible, successful MMM implementation requires addressing organizational challenges:
a) Data Integration Complexity
Effective MMM requires consolidating disparate data sources. According to Deloitte's Marketing Measurement Maturity study, organizations with centralized marketing data lakes achieve 31% higher accuracy in their MMM programs.
b) Statistical Expertise Gap
Interpreting MMM results requires statistical literacy. PepsiCo addressed this by creating a "measurement translation layer"—specialists who convert complex statistical outputs into actionable marketing recommendations.
c) Organizational Patience
Unlike attribution, MMM requires observing variations over time. Professor Dominique Hanssens of UCLA cautions that "organizations must resist the instant gratification of flawed attribution and commit to the methodological rigor MMM demands."
4. The Emerging Unified Measurement Paradigm
The future of marketing measurement lies not in choosing between approaches but in unifying them:
a) Hybrid Measurement Frameworks
Leading organizations are building integrated frameworks combining:
- MMM for strategic, privacy-compliant insights
- Marketing mix data (MTA) where available for tactical guidance
- Incrementality testing for validation
- Unified reporting interfaces for cross-method synthesis
b) Privacy-Preserving Integration Techniques
Advanced methods enable integration without compromising privacy:
- Federated analytics combining insights without raw data sharing
- Privacy-preserving machine learning maintaining user anonymity
- Synthetic data generation for measurement model training
Professor Garrett Johnson of Boston University observes that "the most sophisticated measurement approaches now use MMM as the backbone while strategically incorporating user-level signals where available and permissible."
5. The AI Transformation: Democratizing Advanced Measurement
Artificial intelligence is addressing MMM's historical limitations:
a) Automated Model Development
AI systems now automate previously manual modeling steps:
- Automated variable selection and transformation
- Hyperparameter optimization
- Anomaly detection and baseline adjustments
b) Explanatory Interfaces
Modern MMM platforms include intuitive interfaces:
- Scenario planning simulators for non-technical users
- Natural language explanations of statistical findings
- Visual exploration of model results
Google's Marketing Mix Model partners program exemplifies this trend, using machine learning to automate model development while maintaining methodological rigor.
Conclusion: The Measurement Renaissance
As privacy changes fragment the digital identity landscape, media mix modeling is evolving from a complementary measurement technique to a foundational approach in the marketing analytics stack. Organizations that embrace this renaissance gain both privacy compliance and deeper marketing insights.
The greatest irony of the privacy-first era may be that by forcing marketers to abandon their obsession with individual-level tracking, privacy regulations are driving adoption of more holistic, sophisticated measurement approaches that ultimately provide more robust strategic guidance—even if they surrender some tactical precision.
As Professor Scott Neslin of Dartmouth's Tuck School of Business observes, "The pendulum swung too far toward attribution at the expense of strategic understanding. Privacy changes are forcing a healthy recalibration toward measuring what matters most—the true incremental impact of marketing on business outcomes."
Call to Action
For marketing leaders navigating measurement disruption:
- Assess your organization's readiness for MMM by evaluating data accessibility, statistical capabilities, and organizational patience
- Consider pilot programs focused on specific brands or regions before full-scale implementation
- Invest in cross-functional data infrastructure connecting marketing investments with business outcomes
- Develop hybrid measurement frameworks integrating MMM with available user-level signals
The organizations that view privacy changes not as a measurement crisis but as an opportunity to build more robust, privacy-compliant analytics capabilities will emerge with a substantial competitive advantage—the ability to measure marketing effectiveness accurately even as traditional tracking approaches continue to erode.
Featured Blogs
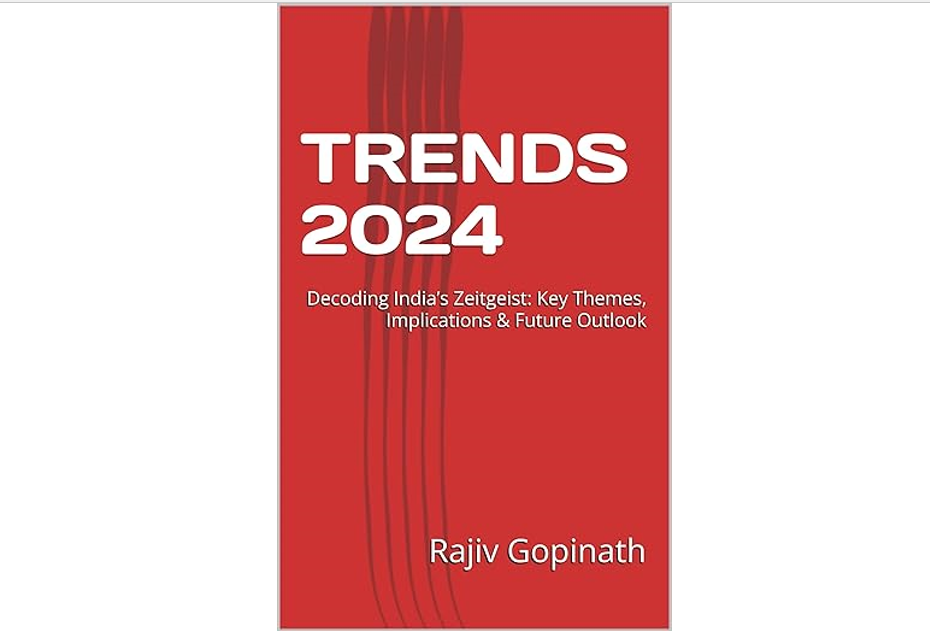
TRENDS 2024: Decoding India’s Zeitgeist: Key Themes, Implications & Future Outlook
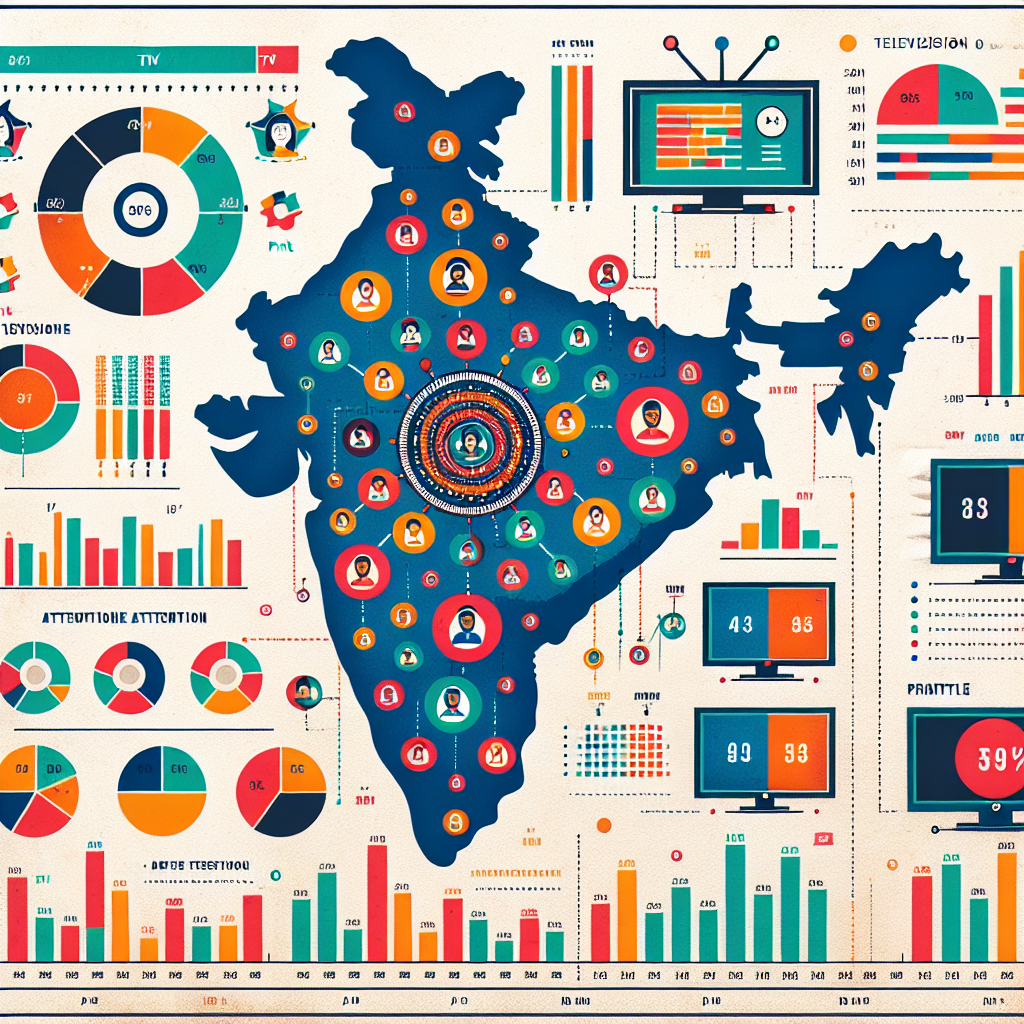
How to better quantify attention in TV and Print in India
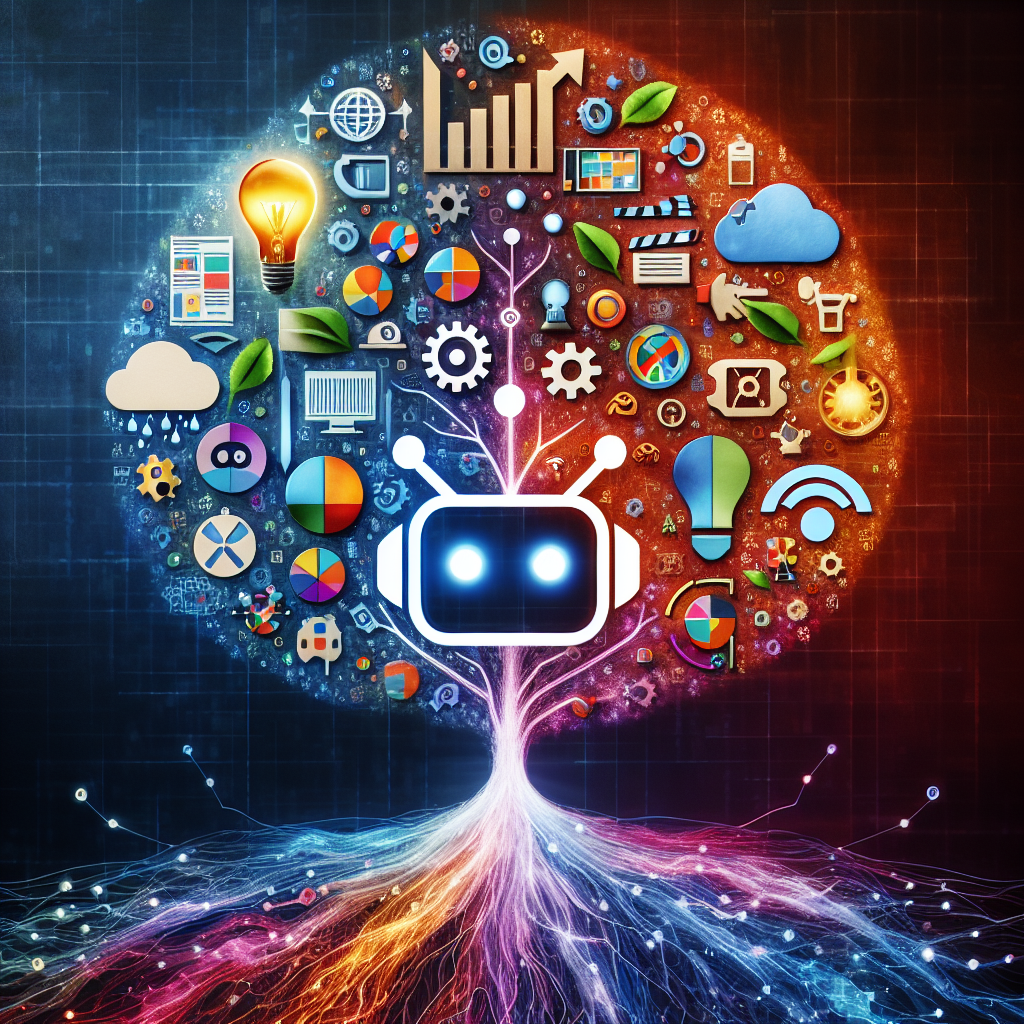
AI in media agencies: Transforming data into actionable insights for strategic growth
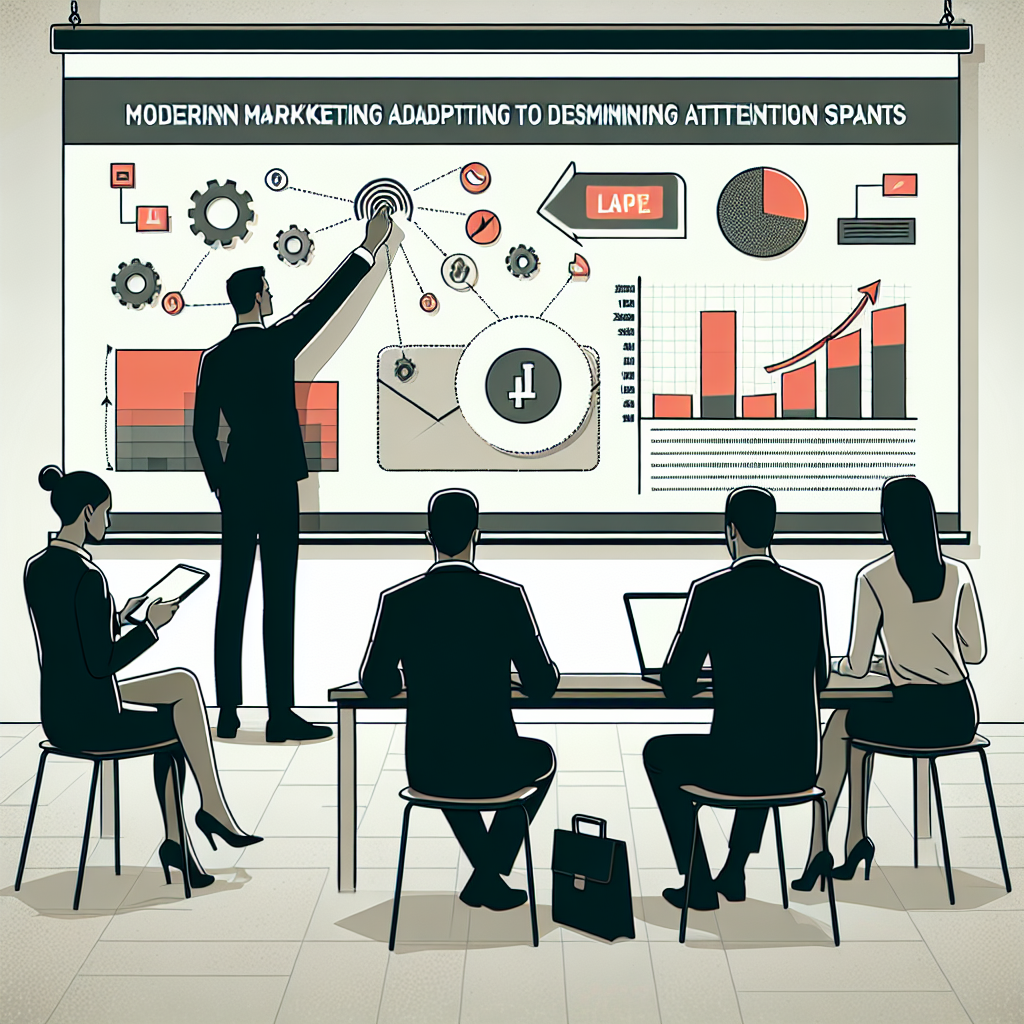
How the Attention Recession Is Changing Marketing
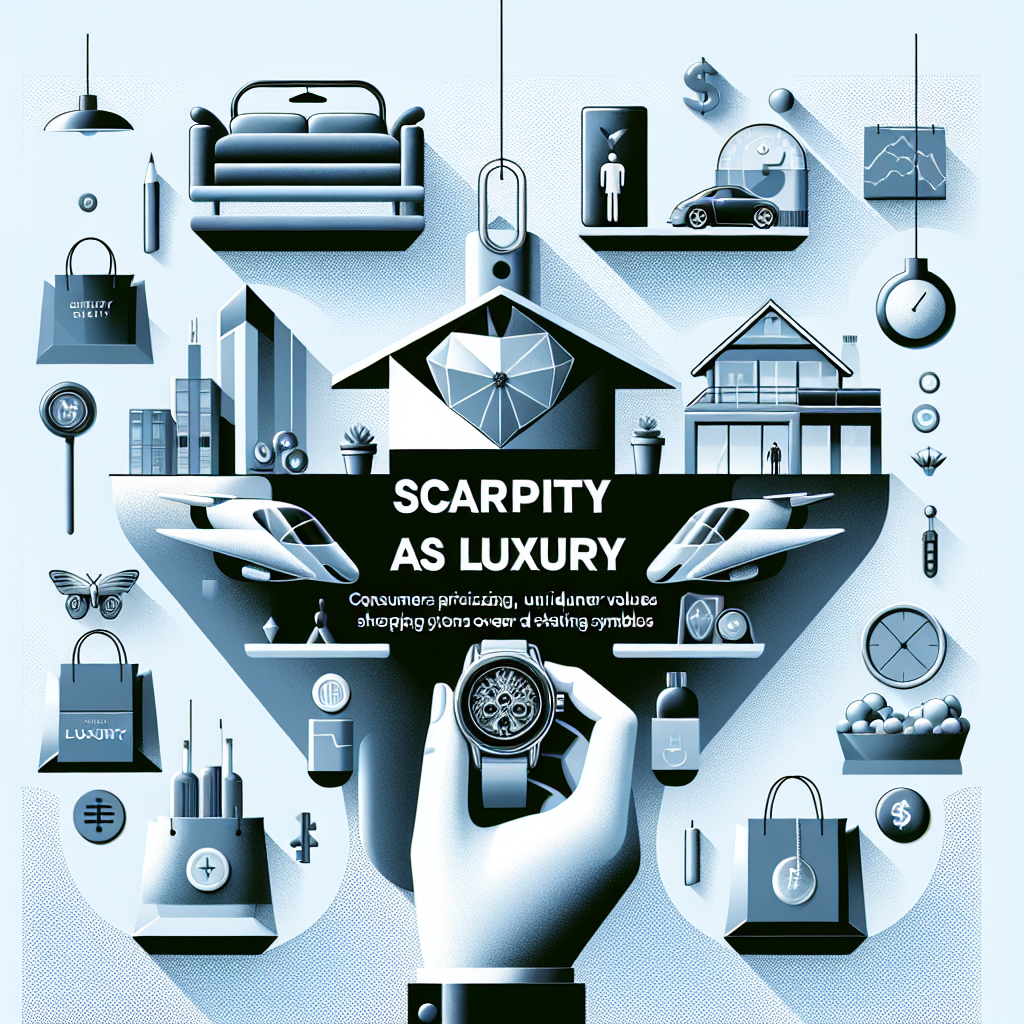
The New Luxury Why Consumers Now Value Scarcity Over Status
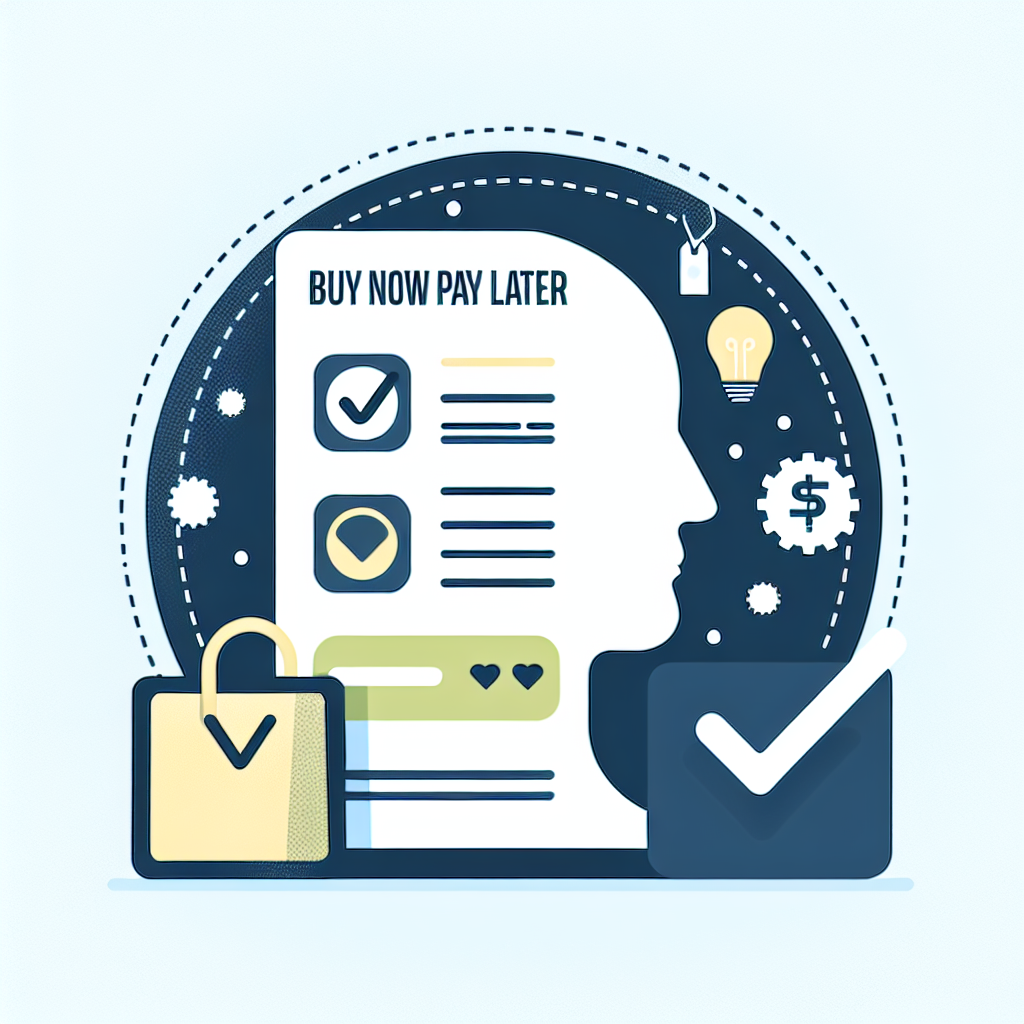
The Psychology Behind Buy Now Pay later
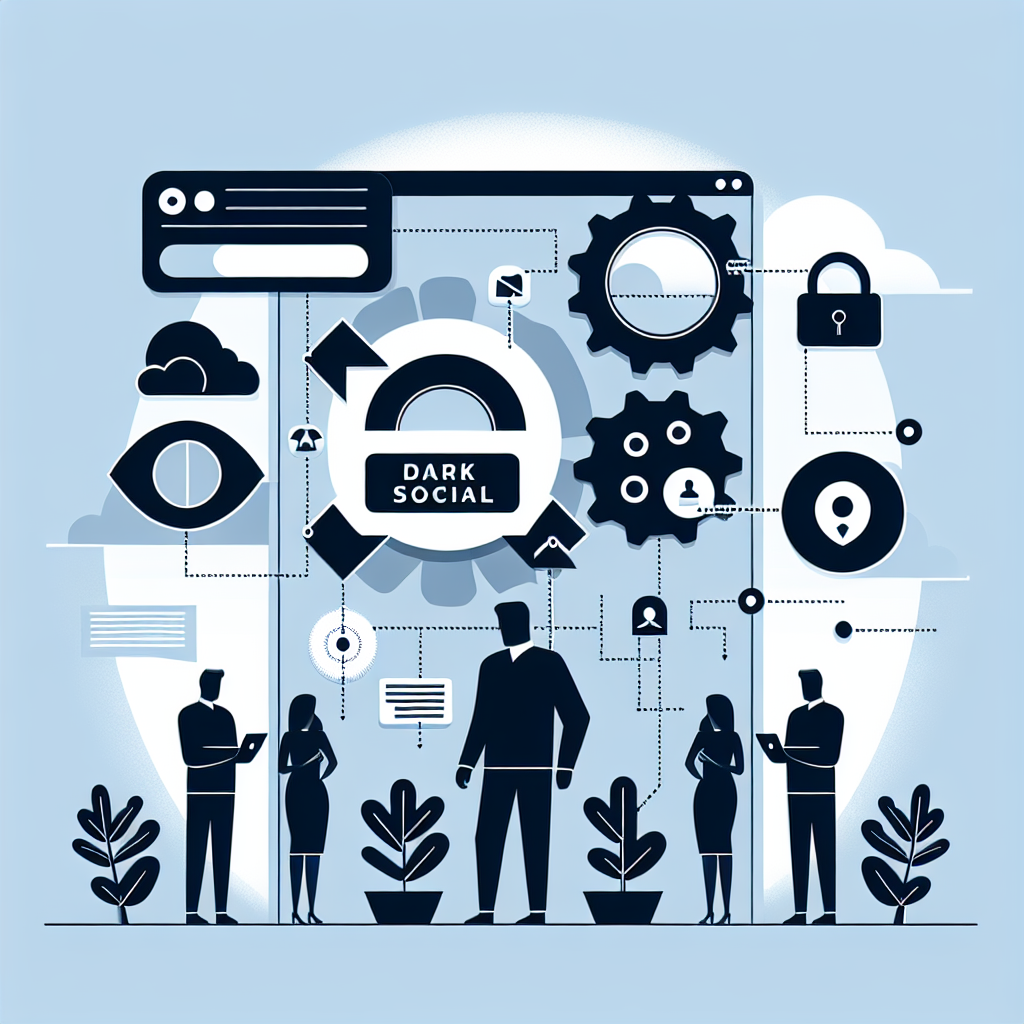
The Rise of Dark Social and Its Impact on Marketing Measurement
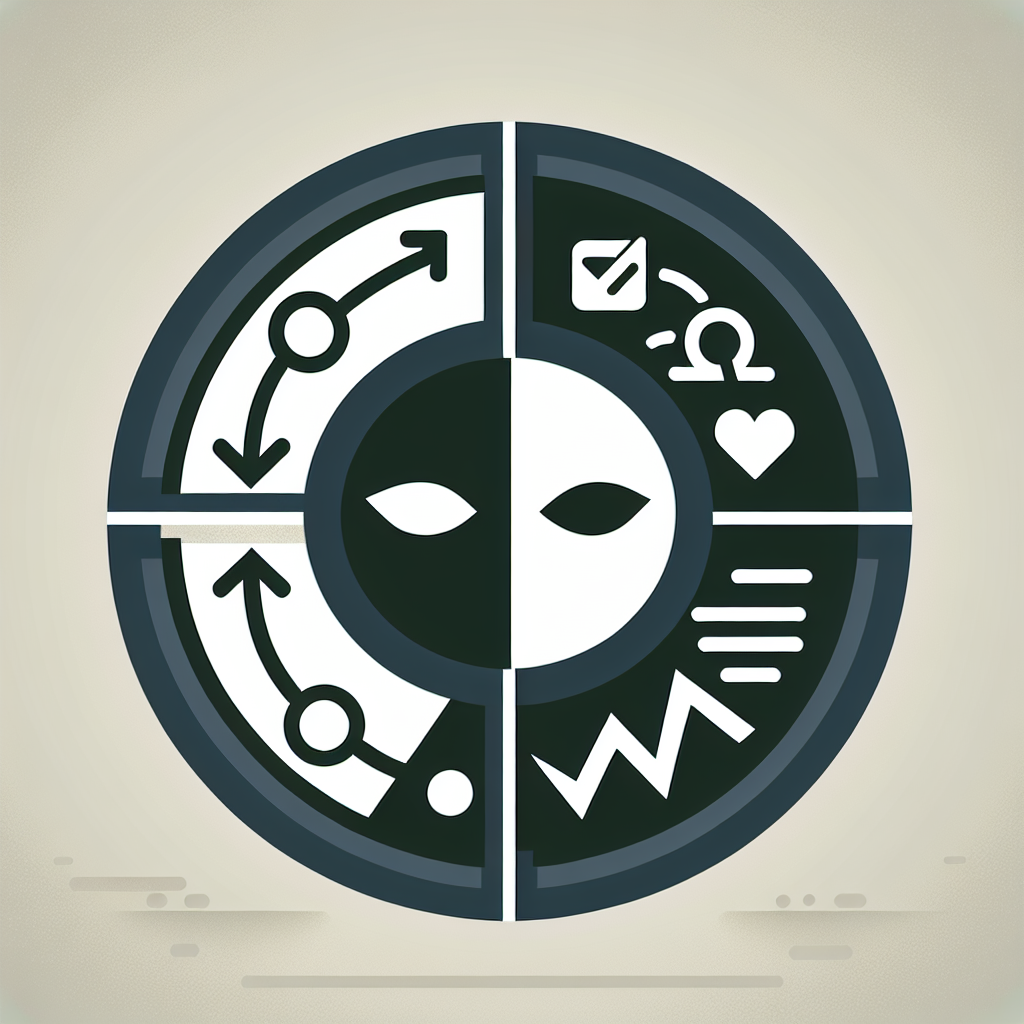
The Role of Dark Patterns in Digital Marketing and Ethical Concerns
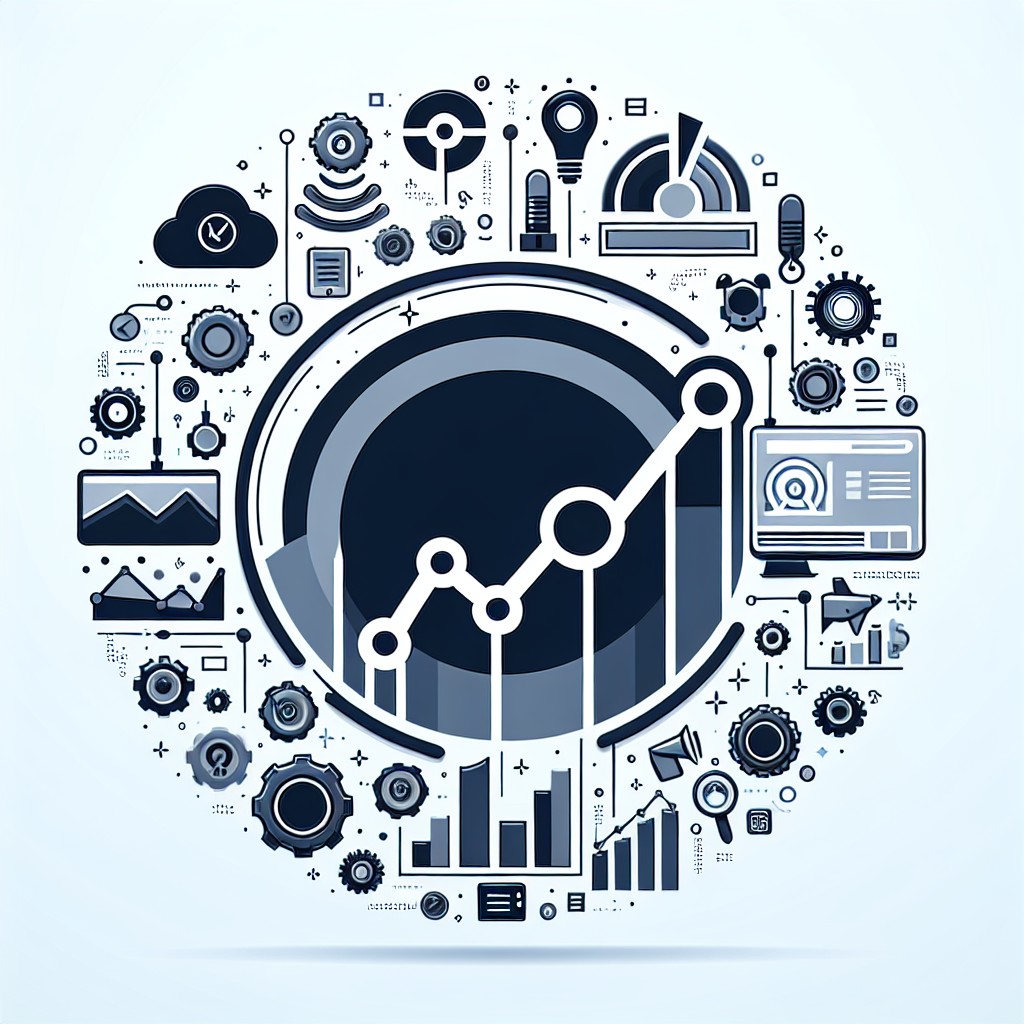