AI & Privacy: How Machine Learning is Reshaping Ad Targeting
Three months ago, Pedro was scrolling through his newsfeed when an advertisement for hiking boots appeared—exactly what he needed for an upcoming trip. His first reaction was appreciation for the relevance, quickly followed by unease: he hadn't searched for hiking gear on that device or shared his travel plans on any platform. This uncanny moment sparked his curiosity about the invisible machinery of ad targeting. As Pedro began investigating the intersection of artificial intelligence and privacy, he discovered something unexpected—the most innovative companies weren't using AI to enhance surveillance but to reinvent targeting in ways that respect privacy while maintaining effectiveness. What began as concern evolved into fascination with how machine learning is fundamentally reimagining the balance between personalization and privacy in digital advertising.
Introduction: The Targeting Paradox
Digital advertising stands at a pivotal crossroads. The industry built trillion-dollar businesses on increasingly precise targeting capabilities, yet this very precision has triggered a powerful privacy backlash. As regulators implement stringent data protection laws, platforms restrict tracking capabilities, and consumers grow wary of surveillance, the foundational technologies of ad targeting face existential challenges.
According to the Pew Research Center, 81% of Americans feel they have little to no control over data companies collect, while 79% express concerns about how their data is used. Simultaneously, Epsilon research indicates that 80% of consumers are more likely to make purchases from brands offering personalized experiences.
This tension creates what Harvard Business School professor John Deighton terms "the targeting paradox"—consumers desire relevance but reject the tracking that traditionally enables it. Resolving this paradox has become an urgent priority as the $500+ billion digital advertising industry adapts to a privacy-first world.
The solution emerging from this challenge isn't the abandonment of targeting but its reinvention through advanced machine learning techniques that fundamentally alter the relationship between personal data and personalization.
1. Privacy-Preserving Machine Learning: The Technological Foundation
At the core of this transformation are innovative approaches that enable personalization without traditional tracking:
Federated Learning
This revolutionary approach trains algorithms across decentralized data sources without centralizing sensitive information. Google's implementation in their Privacy Sandbox initiative allows ad targeting models to learn from user behavior while keeping all personal data on the device. Early tests show federated learning maintaining 93% of conversion performance compared to cookie-based approaches.
Differential Privacy
By adding precisely calibrated noise to datasets, differential privacy provides mathematical guarantees against individual identification while preserving aggregate insights. Apple employs this technique extensively, enabling personalization features while ensuring no individual user data leaves their device. The technique has reduced personal data exposure by over 70% while maintaining targeting effectiveness.
Synthetic Data Generation
Advanced generative AI creates statistically representative but entirely artificial user profiles for model training and testing. Pinterest utilizes this approach to develop targeting algorithms without exposing actual user data, reducing privacy risk while maintaining targeting relevance.
Stanford AI researcher Dr. Fei-Fei Li notes: "The most promising machine learning approaches don't require surveillance to be effective. By rethinking the relationship between data and intelligence, we can build systems that respect privacy by design rather than as an afterthought."
2. Contextual Intelligence: Understanding Content, Not People
Machine learning has dramatically enhanced contextual targeting capabilities, moving beyond basic keyword matching to deep content understanding:
Natural Language Understanding
Advanced language models analyze content with near-human comprehension. The New York Times' first-party ad platform employs NLP to understand content sentiment and themes, enabling advertisers to reach readers in contextually relevant environments without tracking them across the web. This approach has yielded 70% of the performance of cookie-based targeting.
Computer Vision Analysis
AI systems now recognize objects, scenes, emotions, and brand safety signals in visual content. YouTube's content-based video targeting leverages computer vision to enable advertisers to place messages alongside specifically relevant content without relying on user profiles. Internal studies show this approach achieving 82% of audience-based targeting performance.
Predictive Context Matching
Rather than targeting users based on profiles, AI predicts which contexts will perform best for specific advertising messages. GumGum's contextual intelligence platform uses multimodal AI to analyze both text and images, helping brands like Toyota achieve 37% higher engagement than traditional audience targeting.
As Rishad Tobaccowala, former Chief Strategist at Publicis Groupe explains: "The most sophisticated AI doesn't need to know who you are to be relevant—it needs to understand the moment you're in. That's the key shift from surveillance to contextual intelligence."
3. Cohort-Based Learning: Patterns Without Profiles
Machine learning has enabled a middle ground between individual targeting and contextual approaches through cohort-based methodologies:
Privacy-Preserving Clustering
Google's Topics API uses on-device machine learning to assign browsers to interest categories without sharing individual browsing histories. This approach maintains 89% of conversion value compared to third-party cookies according to Google's internal testing.
Look-alike Modeling Without Identifiers
Pinterest's "taste graph" identifies content relationships rather than user relationships, enabling advertisers to reach users with similar interests without tracking individuals. This method has achieved performance parity with traditional look-alike audiences.
Probabilistic Pattern Recognition
Rather than deterministic user identification, machine learning identifies patterns suggesting similar user intent. Walmart Connect leverages this approach across their retail media network, driving a 41% increase in return on ad spend for CPG brands compared to conventional targeting.
Professor Sandra Matz of Columbia Business School emphasizes: "The future of personalization isn't about knowing everything about someone—it's about identifying meaningful patterns at a group level that respect individual boundaries."
4. First-Party Intelligence Amplification
AI is transforming how organizations extract value from consented first-party data:
Predictive Audience Expansion
Machine learning models trained on first-party data can identify similar consumers in privacy-safe environments. Unilever employed this technique to expand their targetable audience by 57% while maintaining privacy compliance across markets.
Intent Forecasting
Rather than tracking past behavior, AI predicts future intent based on anonymized patterns. Expedia Group's AI-driven intent prediction engine anticipates travel interests without persistent user profiles, increasing conversion rates by 43%.
Customer Data Transformation
AI transforms sparse first-party data into rich insights without additional tracking. IKEA's machine learning platform enhances limited customer data points into comprehensive preference profiles, enabling personalization while minimizing data collection.
As Andrew Ng, AI pioneer and founder of DeepLearning.AI notes: "The companies succeeding with AI and privacy aren't using machine learning to extract more data—they're using it to extract more intelligence from the data they already have with permission."
5. The Ethics-First Framework: Beyond Technical Solutions
Leading organizations implement governance frameworks ensuring responsible AI deployment:
Algorithmic Fairness Testing
Advanced techniques identify and mitigate potential bias in targeting models. IBM's AI Fairness 360 toolkit helps advertisers ensure their algorithms don't inadvertently discriminate, addressing a critical ethical dimension of AI targeting.
Explainability Mechanisms
Transparency-enhancing techniques make AI targeting decisions interpretable to both marketers and consumers. Microsoft's Responsible AI principles require all advertising algorithms to provide human-understandable explanations for targeting decisions.
User Control Integration
Machine learning systems that adapt to explicit user feedback rather than just implicit behavior. Netflix's recommendation system incorporates direct user feedback to continuously refine content suggestions, creating a virtuous cycle of improvement and trust.
Conclusion: The Reimagined Future
The convergence of AI innovation and privacy imperatives isn't merely creating workarounds for cookie deprecation—it's fundamentally reimagining how advertising targeting functions. By shifting from surveillance-based approaches to intelligence-based models, machine learning is establishing a new paradigm that respects privacy while maintaining personalization.
Organizations embracing this shift aren't just adapting to new constraints—they're building more sustainable, ethical targeting capabilities aligned with evolving consumer expectations and regulatory requirements. This transformation represents not the end of targeting precision but its evolution into forms that can thrive in a privacy-first digital ecosystem.
Call to Action
For marketing leaders navigating this transformation:
- Audit your current targeting stack to identify dependencies on deprecated tracking technologies
- Invest in AI capabilities that enhance first-party data value rather than expanding data collection
- Implement testing frameworks comparing privacy-preserving targeting approaches against traditional methods
- Develop clear data governance policies that incorporate ethics alongside compliance considerations
- Explore partnerships with privacy-preserving advertising platforms pioneering new targeting methodologies
The organizations that lead in reconciling AI-powered personalization with privacy protection won't just maintain targeting capabilities—they'll establish new competitive advantages through consumer trust and technological innovation in the privacy-first era.
Featured Blogs
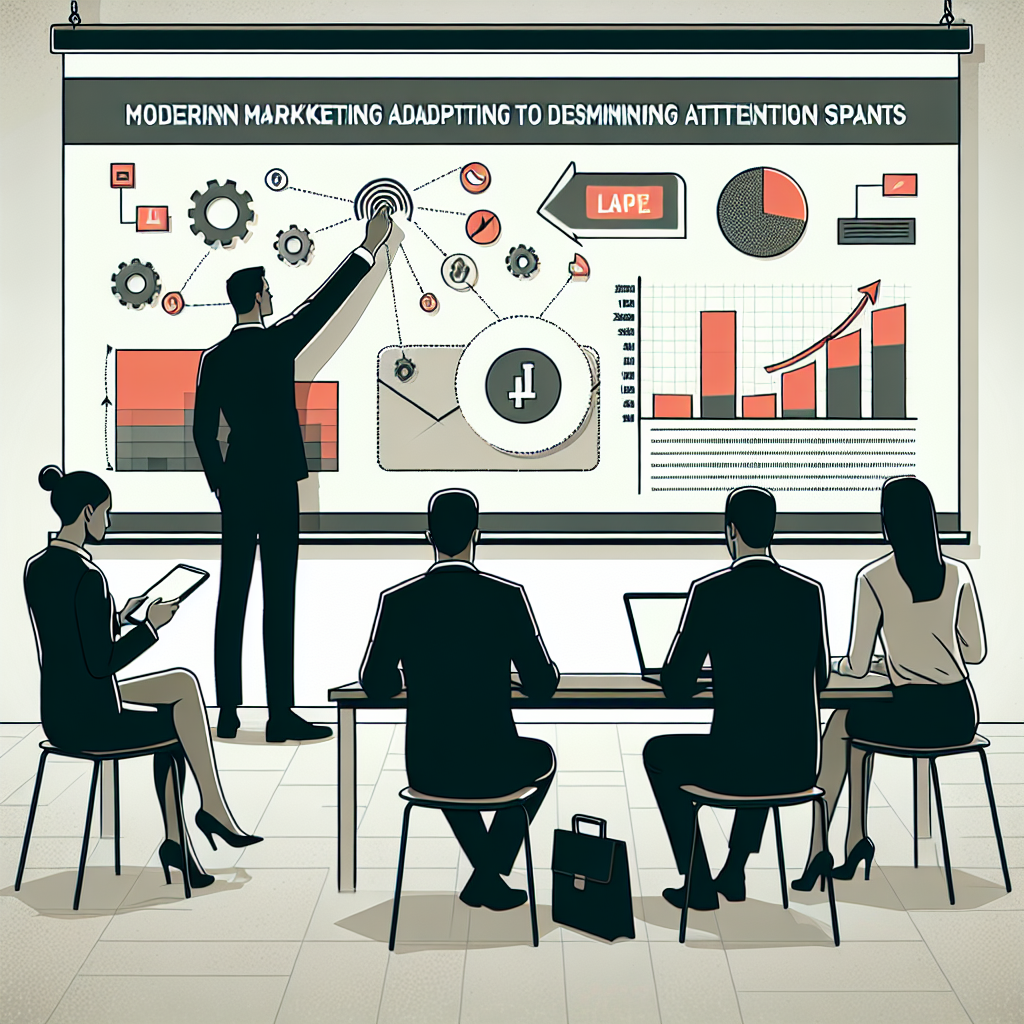
How the Attention Recession Is Changing Marketing
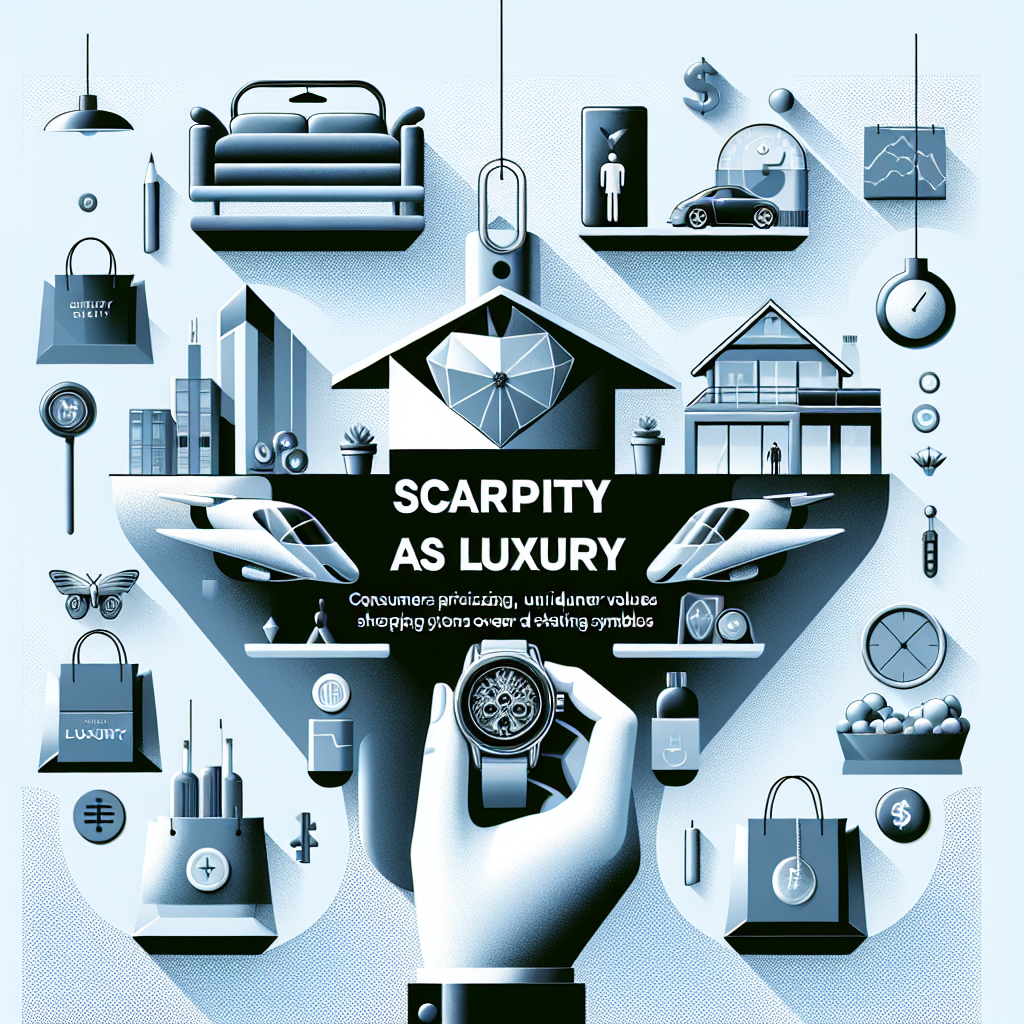
The New Luxury Why Consumers Now Value Scarcity Over Status
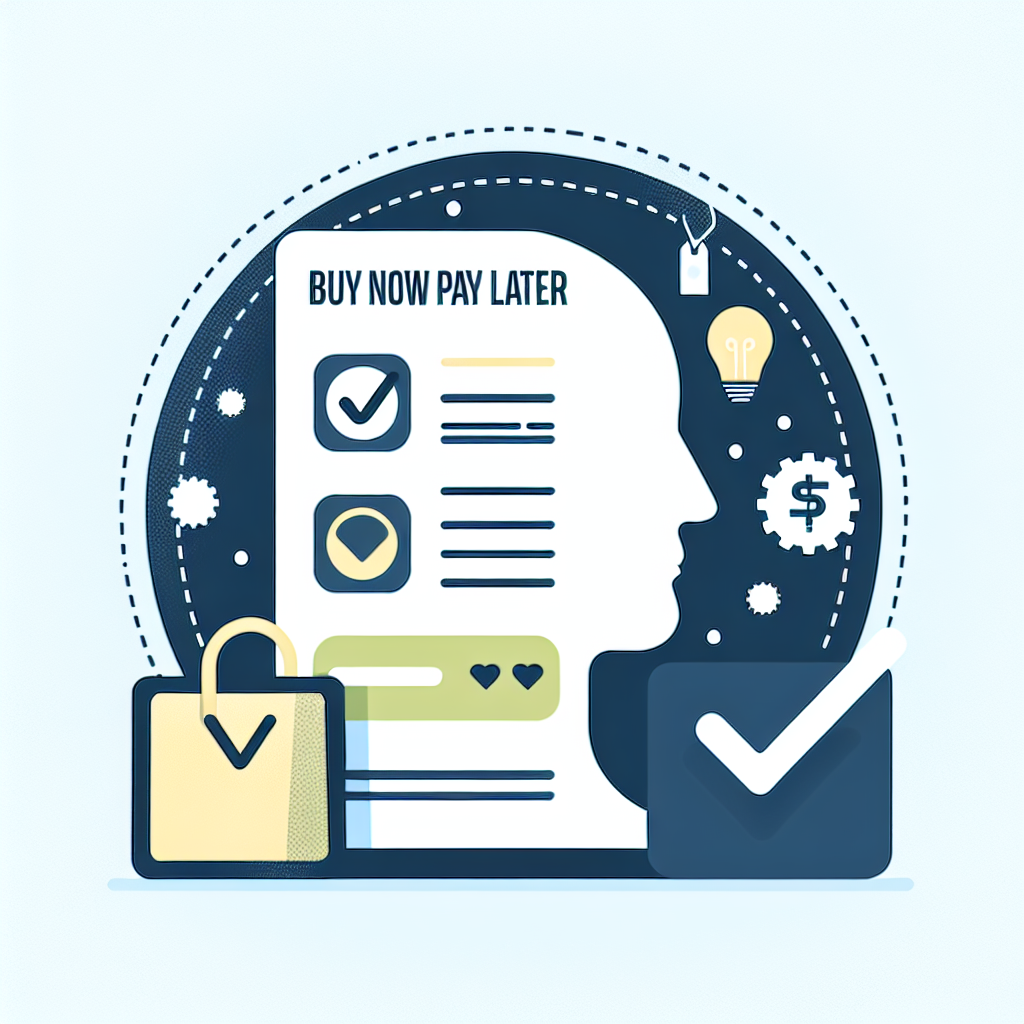
The Psychology Behind Buy Now Pay later
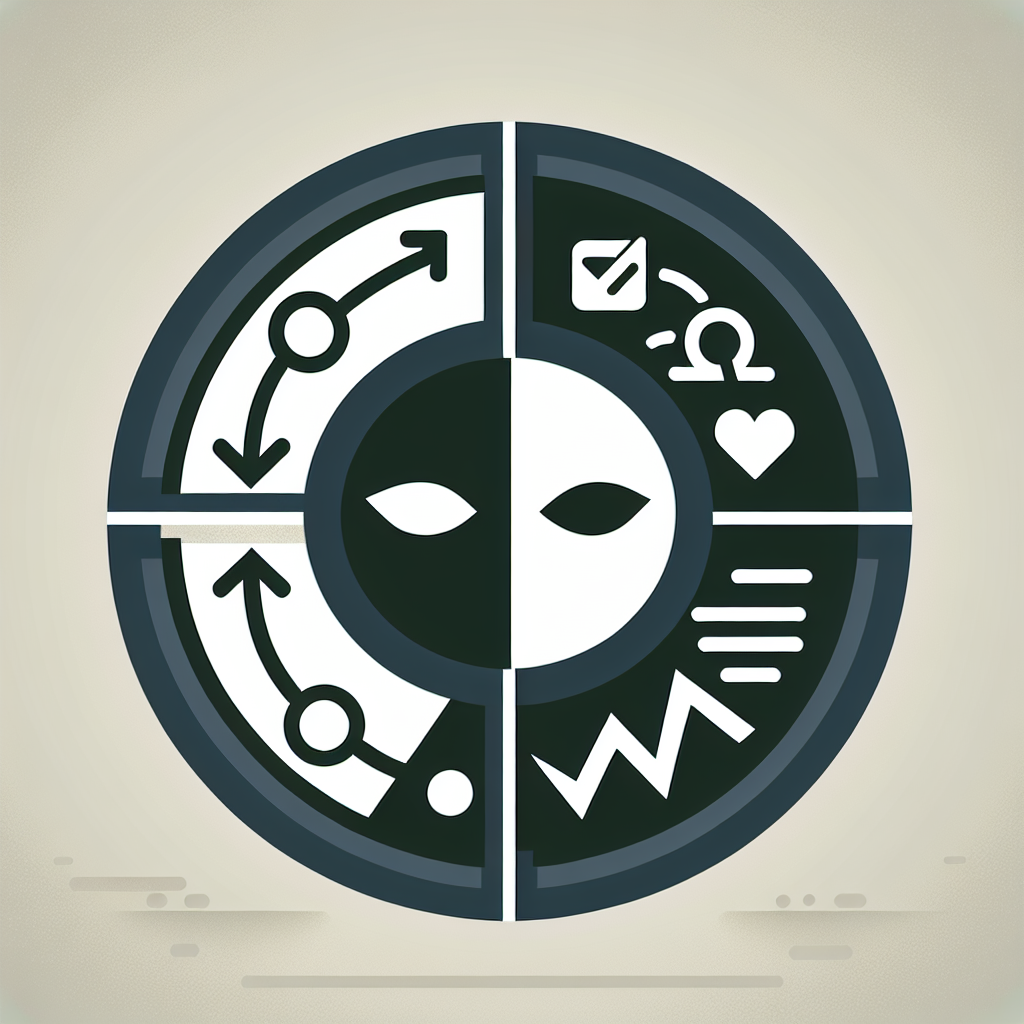
The Role of Dark Patterns in Digital Marketing and Ethical Concerns
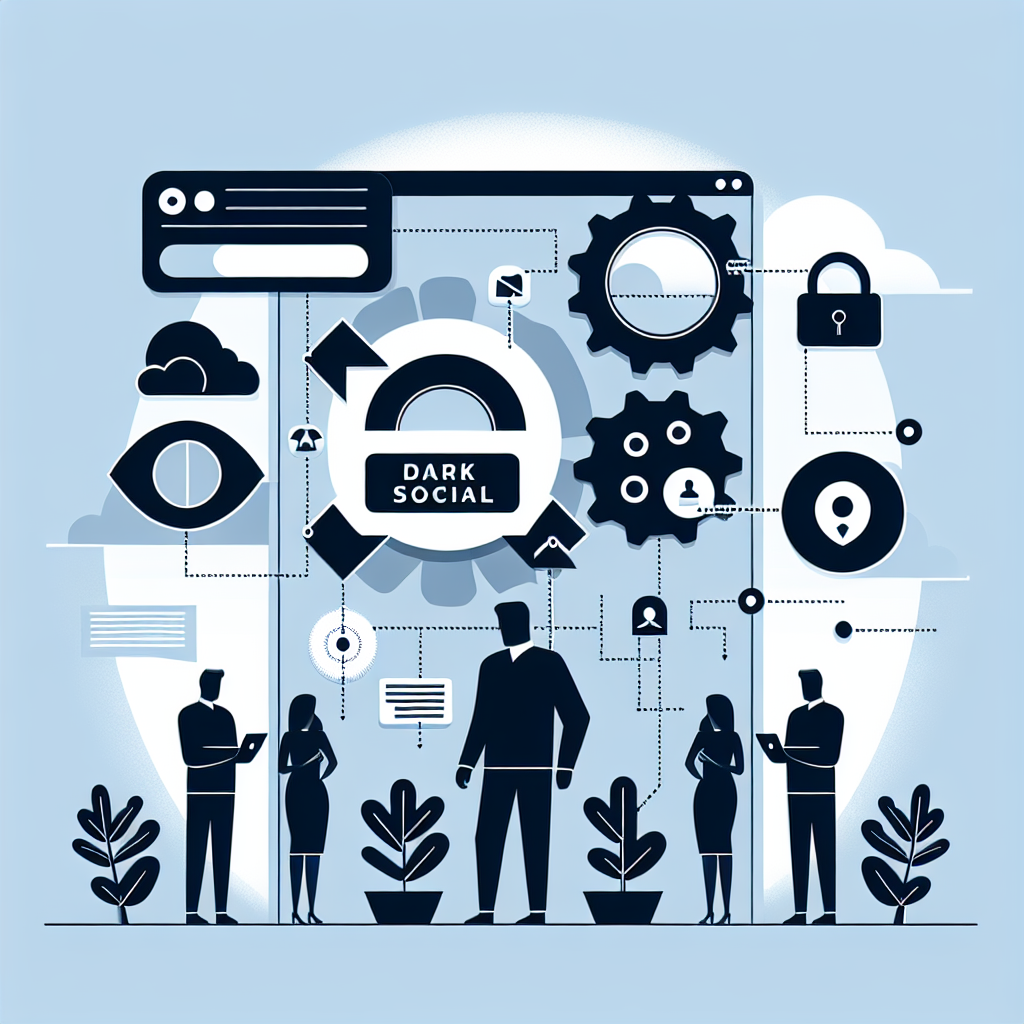
The Rise of Dark Social and Its Impact on Marketing Measurement
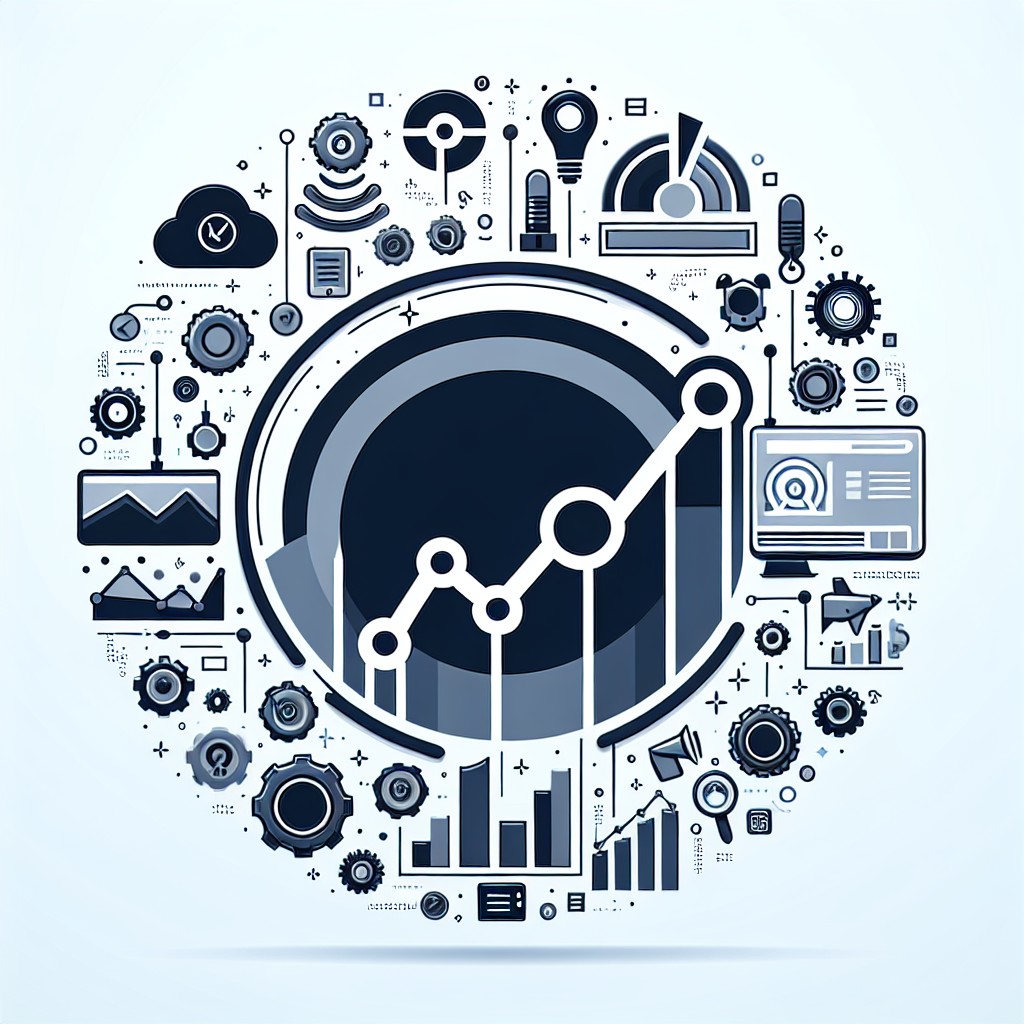
The Future of Retail Media Networks and What Marketers Should Know
Recent Blogs
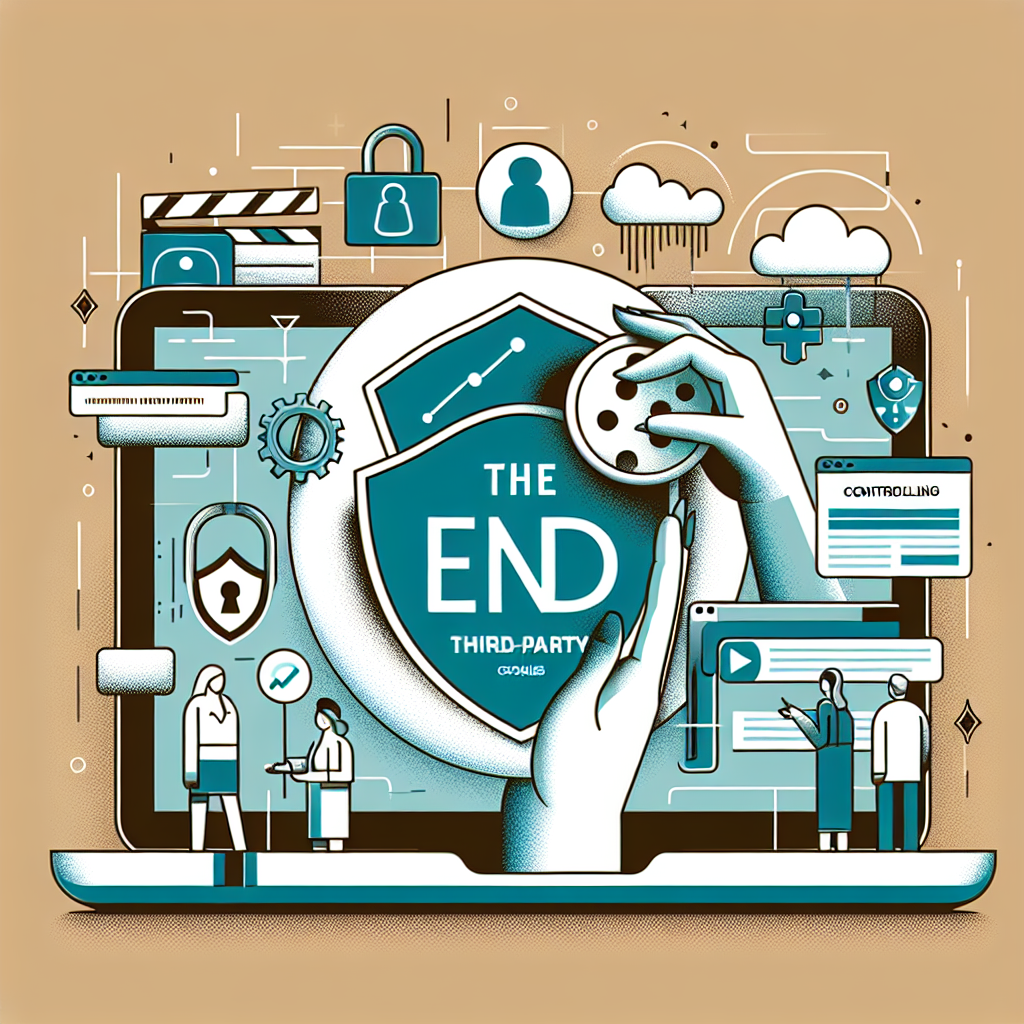
Why the Death of Third-Party Cookies is a Win for Consumer Privacy
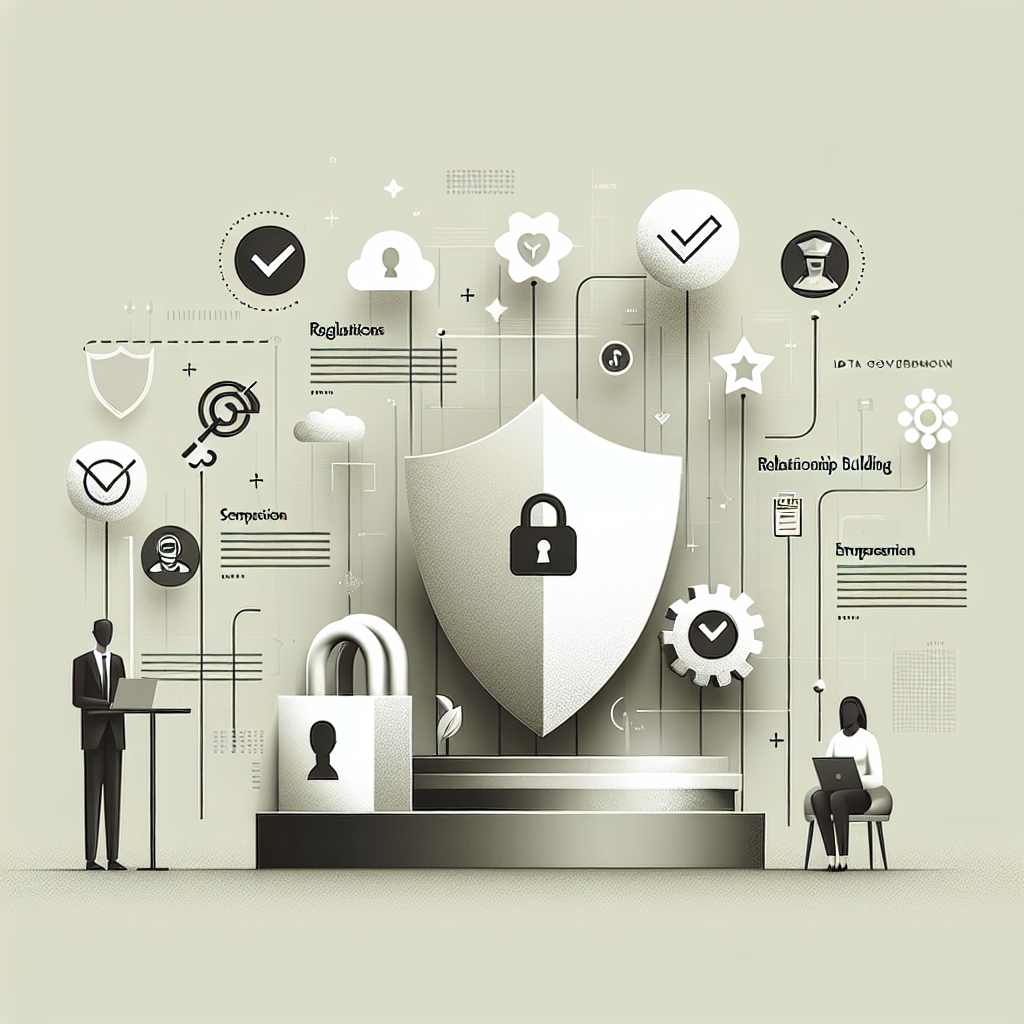
Why Brands Need to Rethink Data Governance in a Privacy-First Era
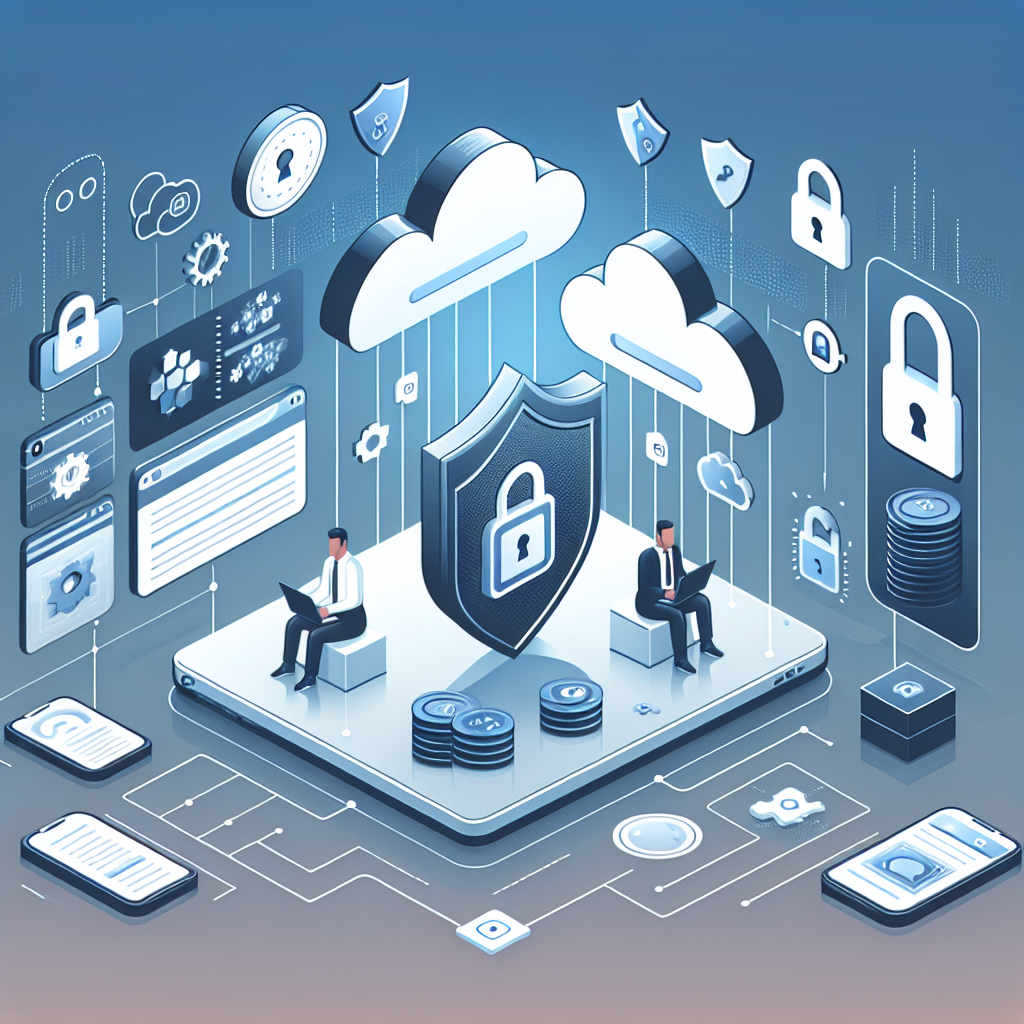
The Shift to a Privacy-First Digital Landscape
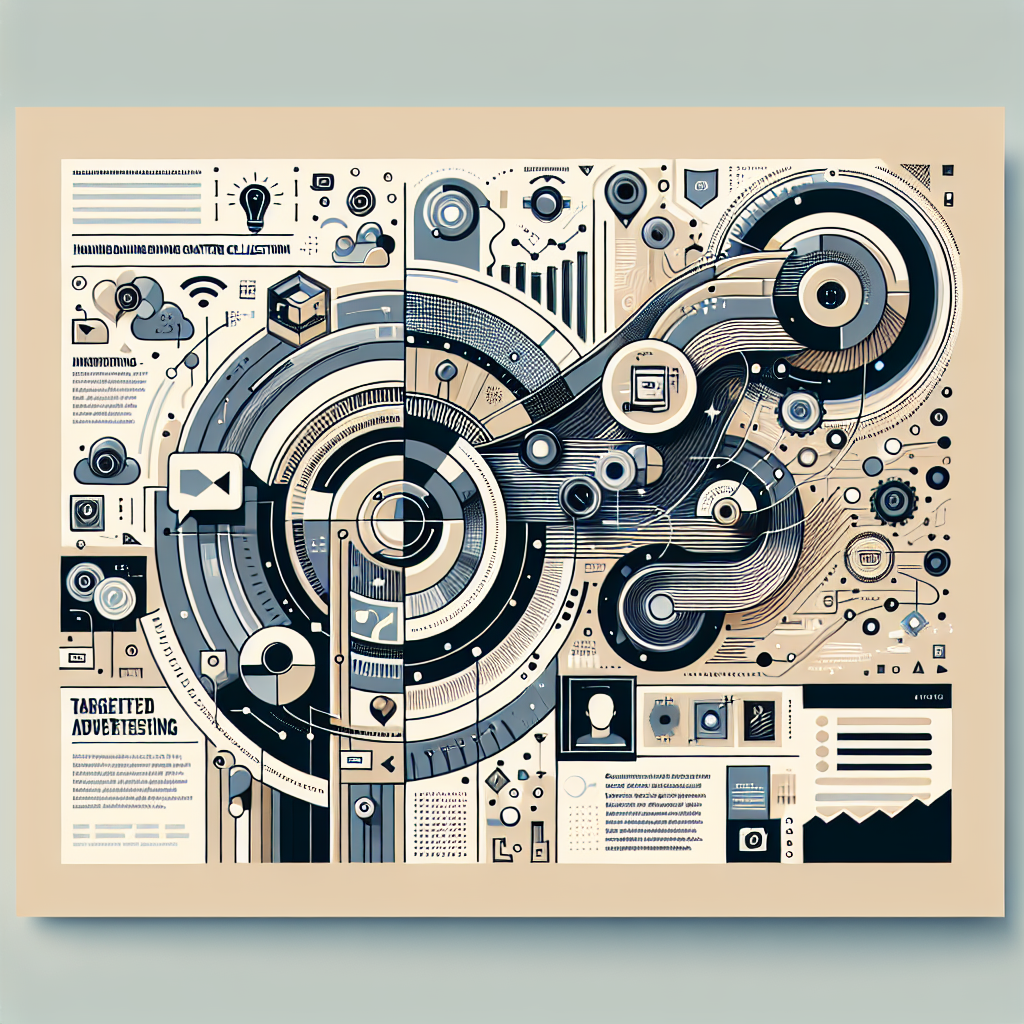
What Google's Privacy Sandbox Means for Marketers
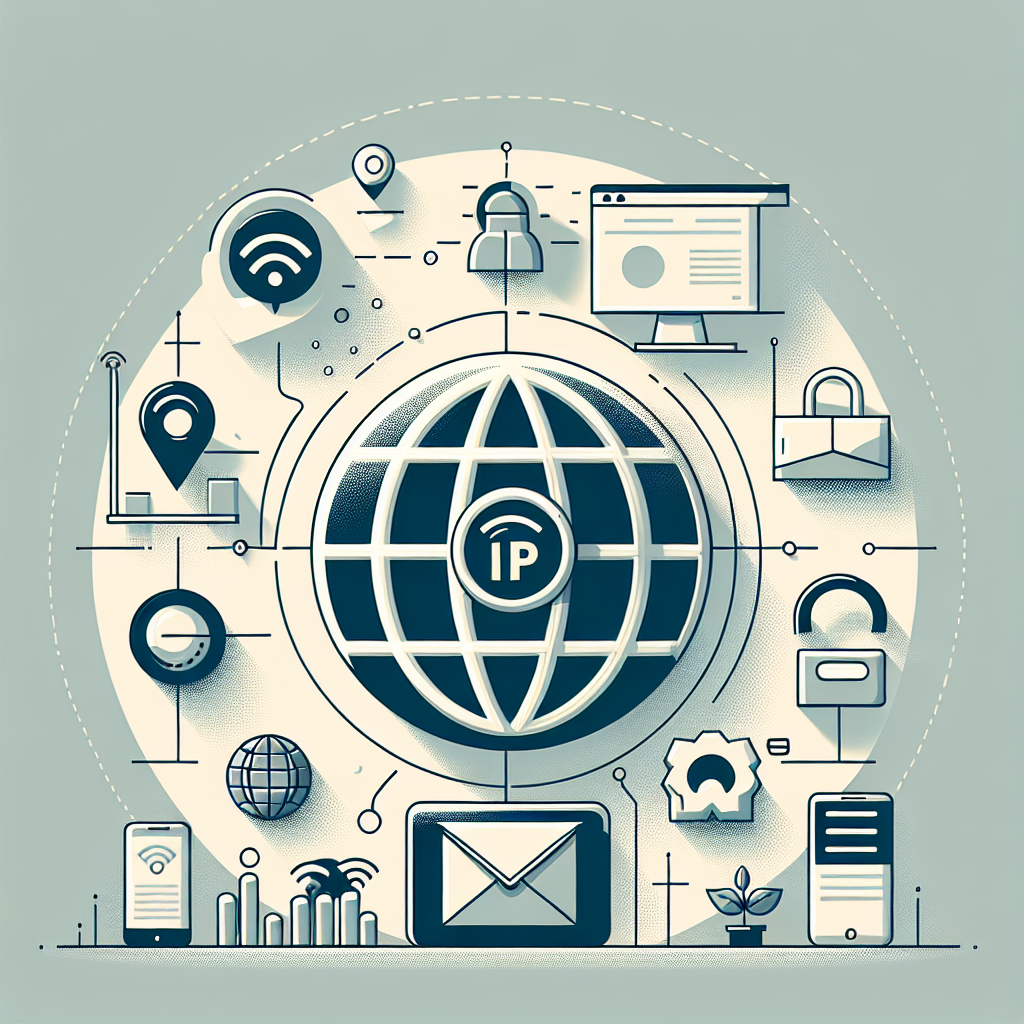
The Role of IP-Based Targeting in a Post-Cookie World
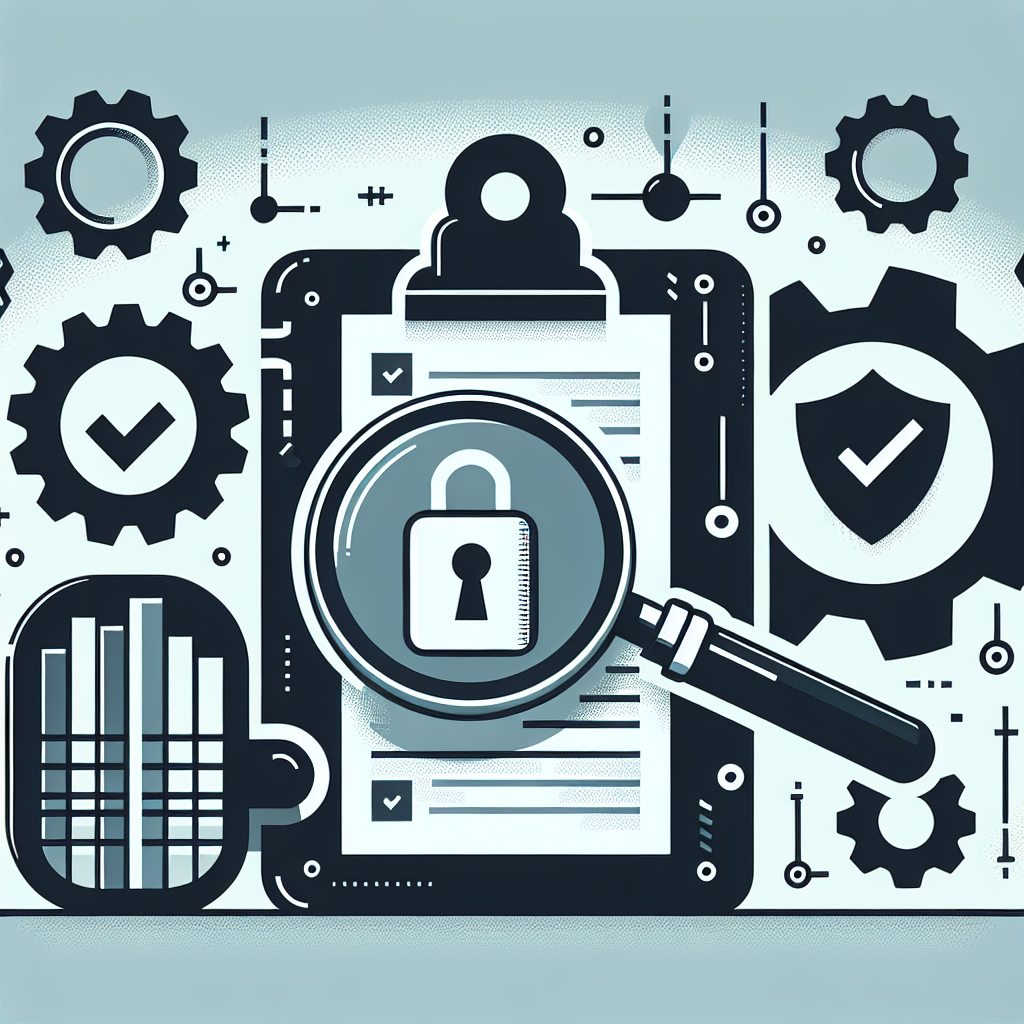