Why Privacy-Preserving AI is the Future of Digital Personalization
Last year, while checking his social media feed, Pedro noticed an advertisement for a product he had only discussed verbally with a friend. The eerie accuracy of this targeting sparked both fascination and concern. Was his phone listening to him? Had his data been collected without his knowledge? This unsettling experience led him down a rabbit hole of research into digital privacy. What he discovered was a brewing revolution: privacy-preserving AI technologies that promise the seemingly impossible – delivering personalized experiences without compromising personal data. This tension between personalization and privacy isn't just a technical challenge; it represents one of the most significant pivots in modern marketing strategy.
Introduction: The Personalization-Privacy Paradox
The digital marketing landscape stands at a critical inflection point. For years, advertisers have relied on increasingly granular user data to power personalization engines, creating tailored experiences that drive engagement and conversion. However, this data-hungry approach has collided with rising privacy concerns, regulatory frameworks like GDPR and CCPA, and the phasing out of third-party cookies. The result is what marketing strategist Shoshana Zuboff calls "surveillance capitalism" – a system that commodifies personal data at the expense of consumer privacy.
The stakes couldn't be higher: 91% of consumers express concern about how companies use their data, while 72% still expect personalized experiences (McKinsey, 2023). This paradox has accelerated the development of privacy-preserving AI – technologies that maintain personalization capabilities while fundamentally transforming how consumer data is collected, processed, and utilized.
1. The Technical Foundation of Privacy-Preserving AI
Privacy-preserving AI encompasses a constellation of technologies designed to protect user data while enabling machine learning:
a) Federated Learning
Rather than centralizing data, federated learning brings the algorithm to the data, training models directly on user devices. Only model updates – not raw data – are shared with central servers.
Example: Google's implementation of federated learning in Gboard keyboard predictions improves text suggestions without sending sensitive typing data to the cloud. The model learns from user behavior locally, maintaining privacy while continuously improving.
b) Differential Privacy
This mathematical framework introduces calibrated noise into datasets, making it impossible to identify individuals while preserving aggregate insights.
Example: Apple employs differential privacy in iOS to collect usage patterns that inform product improvements while mathematically guaranteeing user anonymity. This approach enables them to identify trends without compromising individual privacy.
c) Homomorphic Encryption
This revolutionary technique allows computations to be performed on encrypted data without decryption, enabling analysis while maintaining absolute privacy.
Example: Healthcare provider Anthem uses homomorphic encryption to analyze sensitive patient data for personalized health recommendations while keeping the underlying information fully encrypted.
2. Strategic Business Applications
Privacy-preserving AI is transcending theoretical applications and becoming a strategic imperative:
a) Zero-Party Data Strategies
Brands are shifting toward explicit data voluntarily shared by consumers, combining it with privacy-preserving technologies.
Example: Cosmetics retailer Sephora's "Beauty Insider" program collects preference data directly from users, combining these explicit inputs with federated learning to deliver personalized recommendations without storing sensitive browsing behavior.
b) Contextual Intelligence
Advanced AI can derive intent from context rather than identity, respecting privacy while maintaining relevance.
Example: The New York Times has pioneered privacy-focused advertising through its Perspective contextual targeting platform, which analyzes article content rather than user data to place relevant advertisements, increasing engagement by 40% while eliminating privacy concerns.
c) Privacy as Competitive Advantage
Forward-thinking companies are repositioning privacy protection as a core brand value.
Example: DuckDuckGo has built its entire business model around privacy preservation, growing to over 100 million daily searches by appealing to privacy-conscious consumers, demonstrating the market demand for privacy-respecting services.
3. Overcoming Implementation Challenges
Despite its promise, privacy-preserving AI faces significant obstacles:
a) Computational Complexity
Privacy-preserving techniques often demand greater computational resources and infrastructure investments.
b) Performance Tradeoffs
Early implementations may sacrifice some predictive accuracy compared to privacy-invasive approaches.
c) Organizational Readiness
Companies must develop new skill sets and mindsets to implement these technologies effectively.
Marketing leaders can address these challenges through phased implementation approaches, hybrid systems that balance privacy with performance, and cross-functional privacy governance frameworks that align technical capabilities with ethical considerations.
4. The Future Landscape
Privacy-preserving AI is evolving rapidly, with several emerging trends:
a) Regulatory Acceleration
As privacy regulations expand globally, privacy-preserving AI will become not just ethically desirable but legally necessary.
b) AI-Powered Consent Management
Meta-AI systems will help consumers understand and control their privacy settings with unprecedented granularity.
c) Privacy-Preserving Synthetic Data
AI-generated synthetic datasets will enable training of sophisticated models without exposing real user data.
Professor Sandra Wachter of Oxford University's Internet Institute predicts that "privacy-preserving AI will not merely be a technical solution but a fundamental rethinking of the relationship between consumers, their data, and the brands that serve them."
Conclusion: The Privacy-Personalization Synthesis
Privacy-preserving AI represents more than a technological adaptation; it signals a paradigm shift in digital marketing philosophy. Rather than viewing privacy and personalization as opposing forces, this approach recognizes them as complementary values that, when properly balanced, create more sustainable customer relationships.
As marketing futurist Rishad Tobaccowala observes, "The future belongs to companies that can deliver magic without the creepy." Privacy-preserving AI delivers precisely this balance – maintaining the "magic" of personalization while eliminating the "creepy" aspects of surveillance-based marketing.
The brands that thrive in this new era will be those that recognize privacy not as a constraint but as a catalyst for more meaningful, trust-based customer relationships. These technologies enable a fundamental reset of the digital social contract – moving from extraction to exchange, from surveillance to service.
Call to Action
For marketing leaders navigating this transition:
- Audit your current personalization practices through a privacy-first lens
- Invest in pilot projects using federated learning or differential privacy
- Develop a first-party and zero-party data strategy that prioritizes transparent value exchange
- Build cross-functional teams that unite data scientists, privacy officers, and marketers
- Proactively communicate your privacy-preserving approaches as a competitive differentiator
The privacy-preserving revolution has begun. The question is not whether your organization will participate, but how quickly you'll adapt to this inevitable transformation in digital personalization.
Featured Blogs
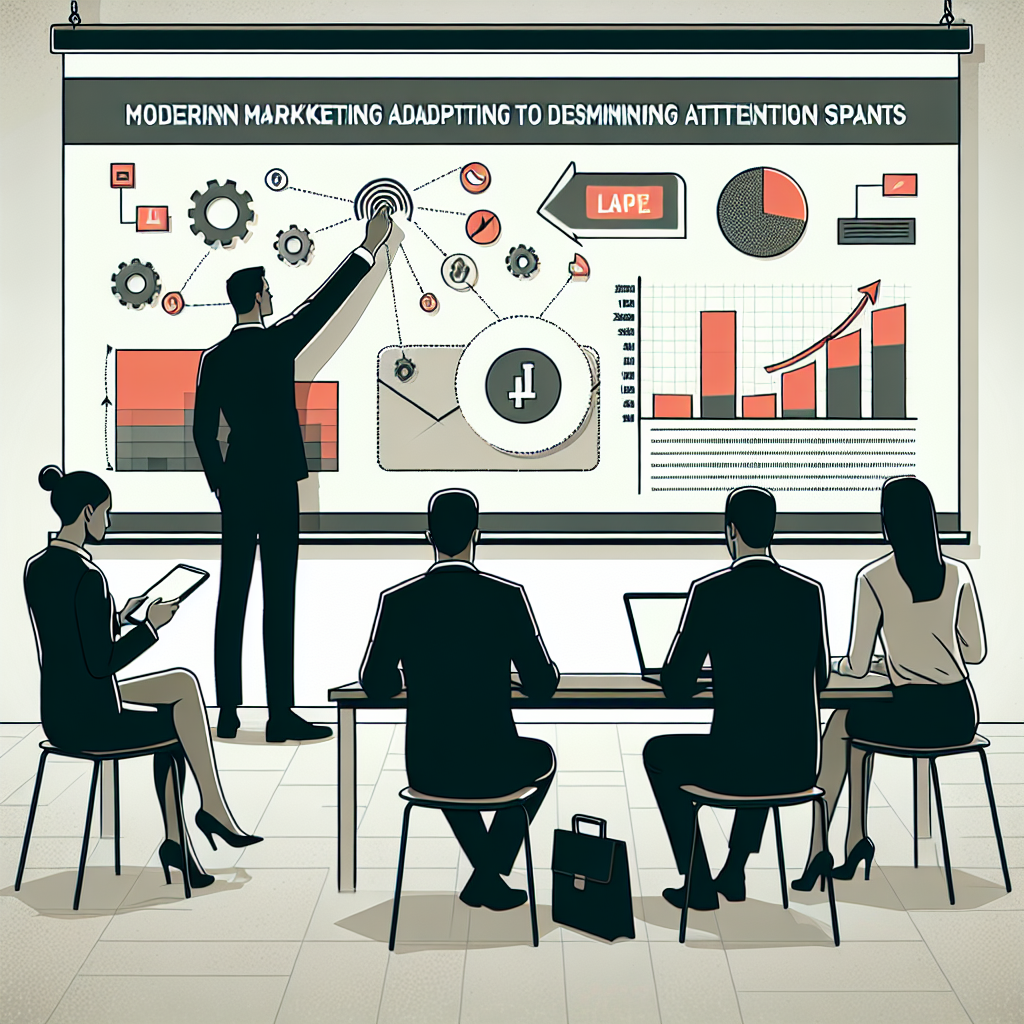
How the Attention Recession Is Changing Marketing
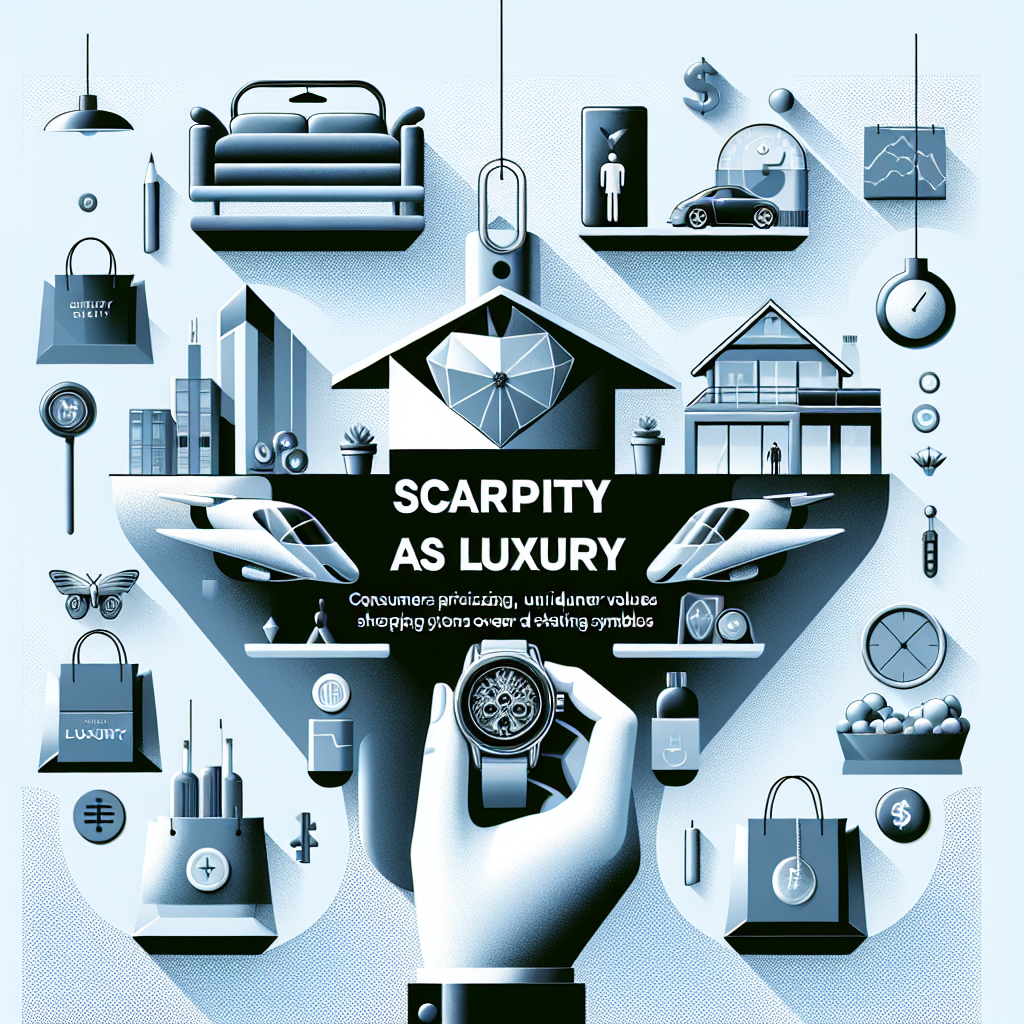
The New Luxury Why Consumers Now Value Scarcity Over Status
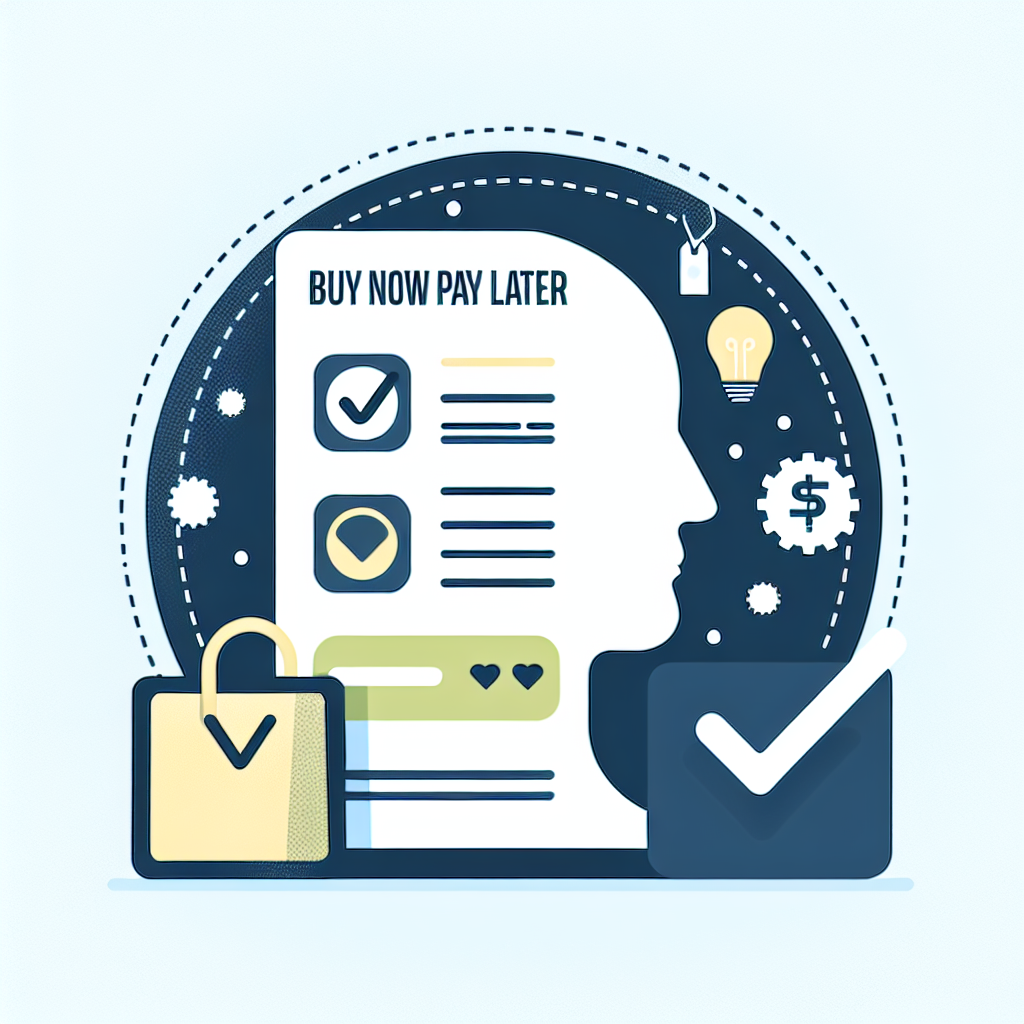
The Psychology Behind Buy Now Pay later
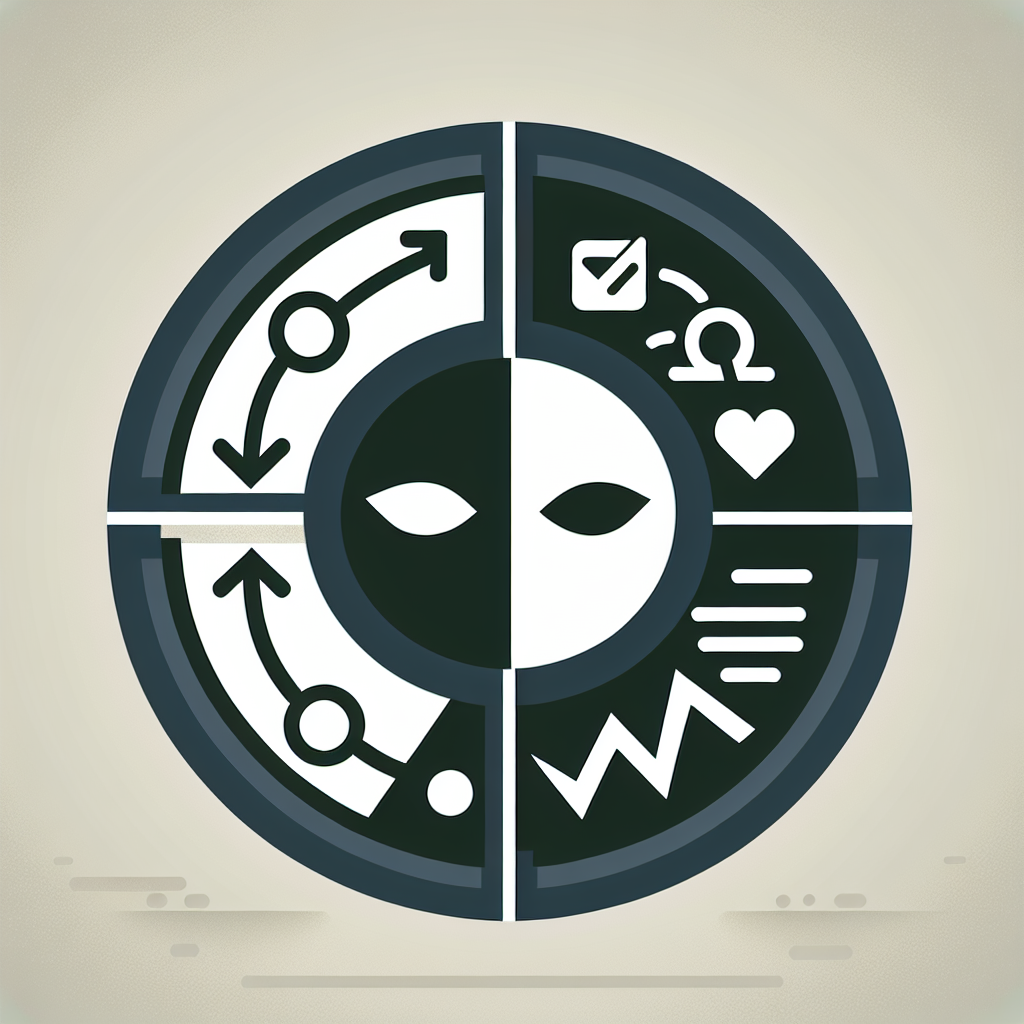
The Role of Dark Patterns in Digital Marketing and Ethical Concerns
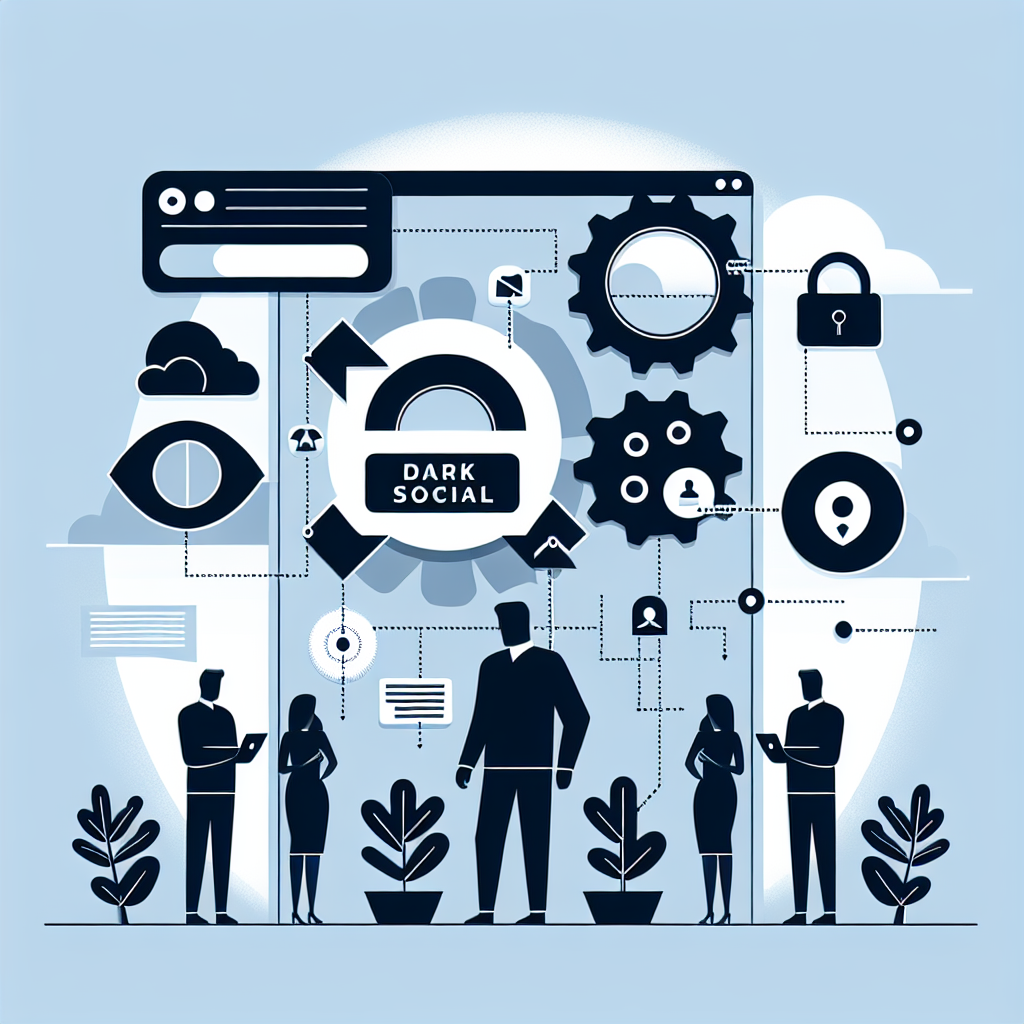
The Rise of Dark Social and Its Impact on Marketing Measurement
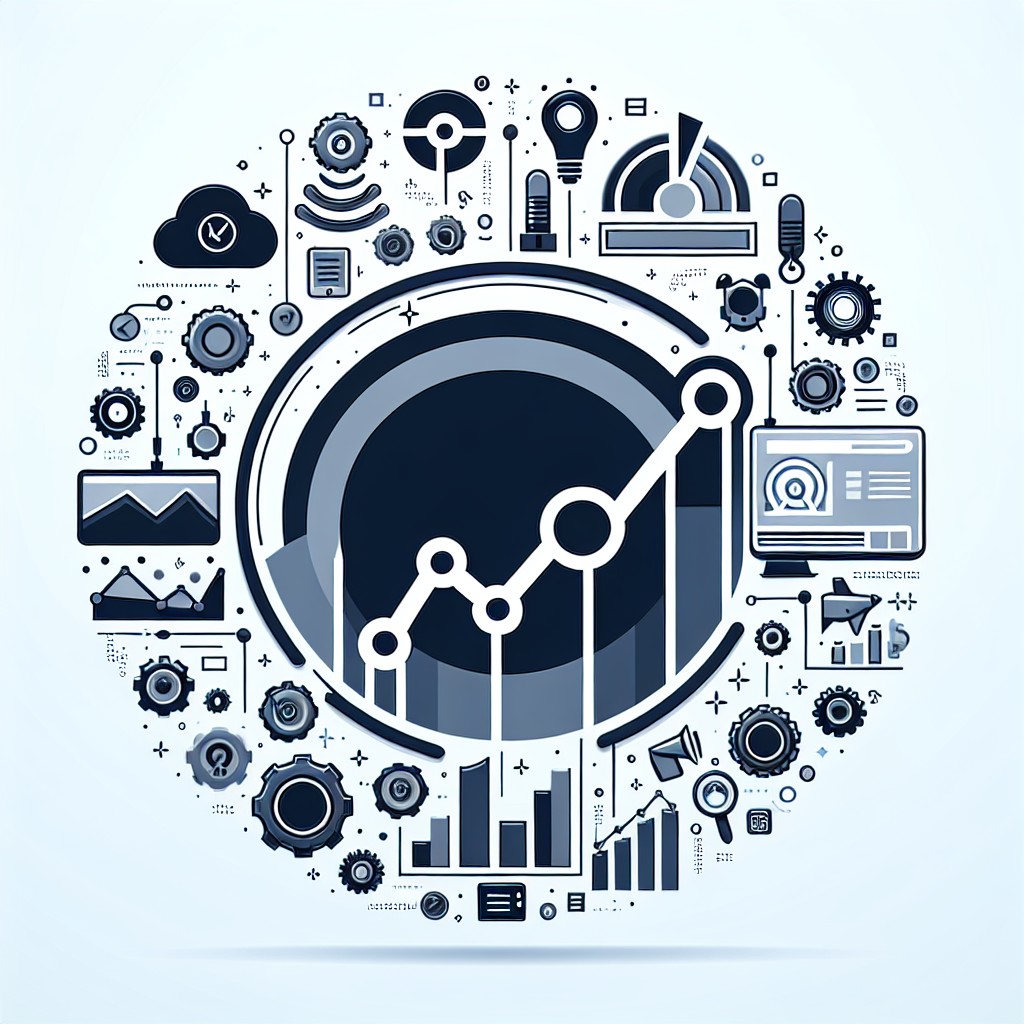
The Future of Retail Media Networks and What Marketers Should Know
Recent Blogs
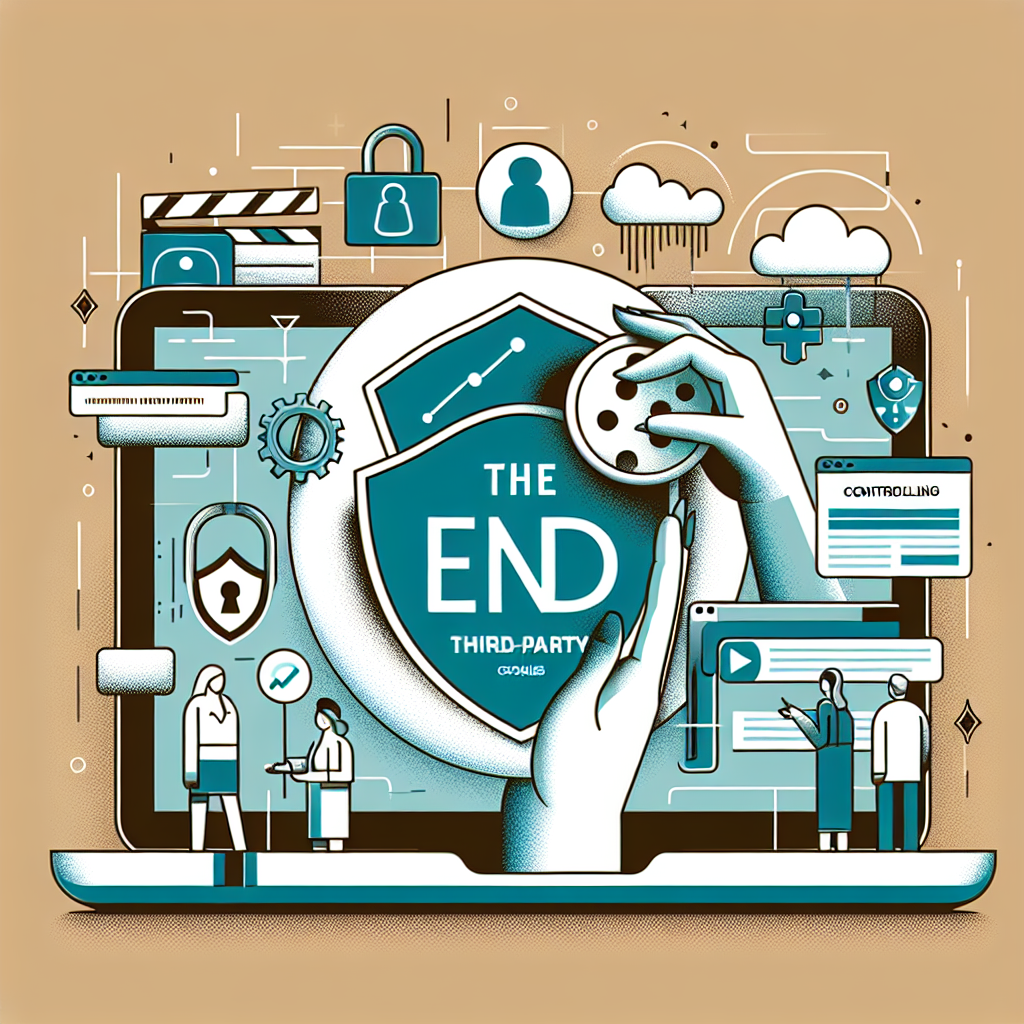
Why the Death of Third-Party Cookies is a Win for Consumer Privacy
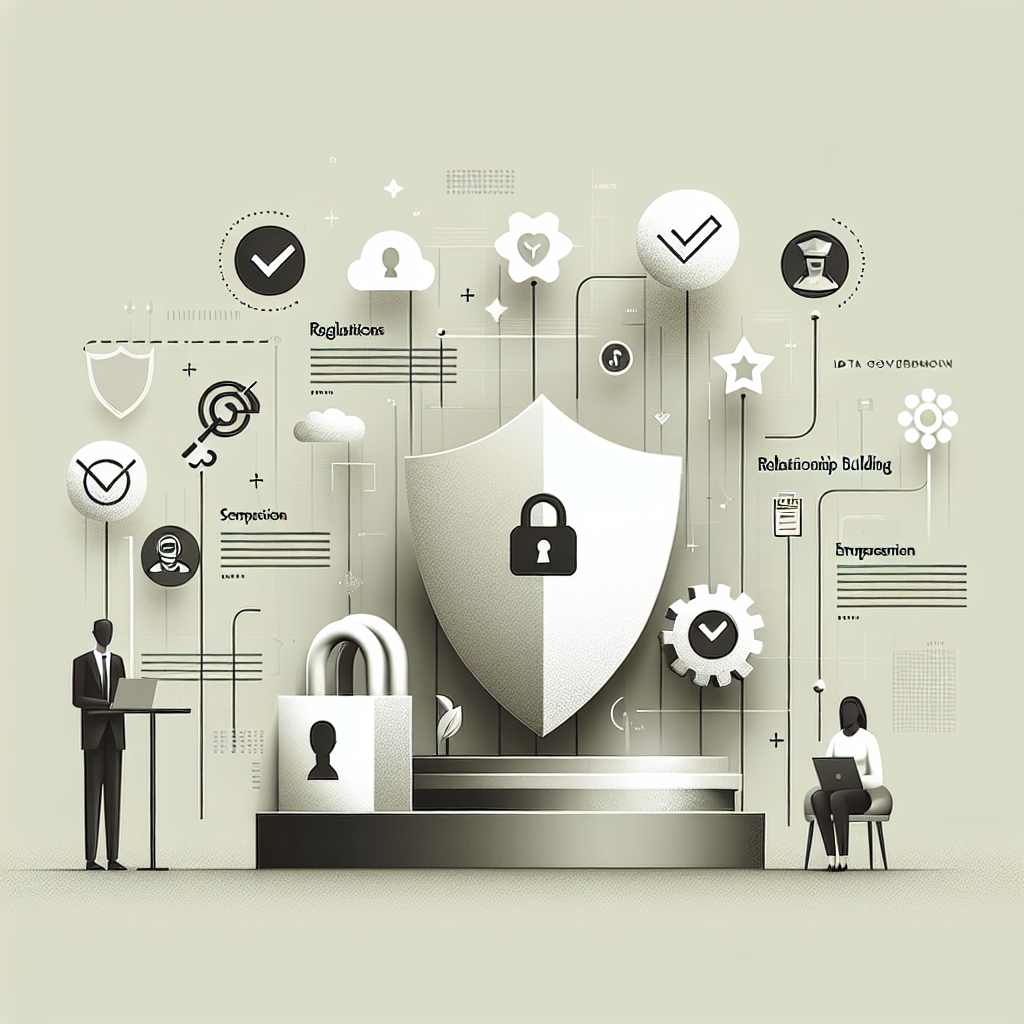
Why Brands Need to Rethink Data Governance in a Privacy-First Era
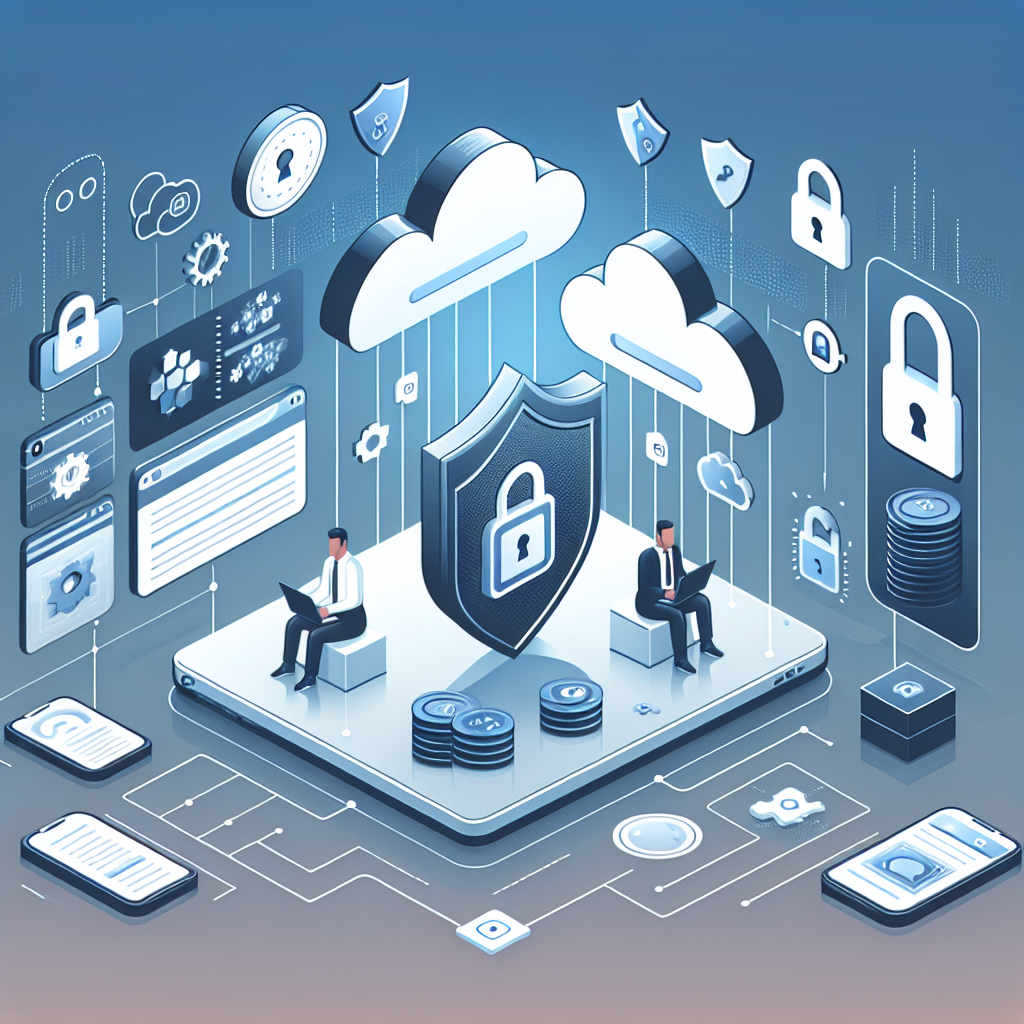
The Shift to a Privacy-First Digital Landscape
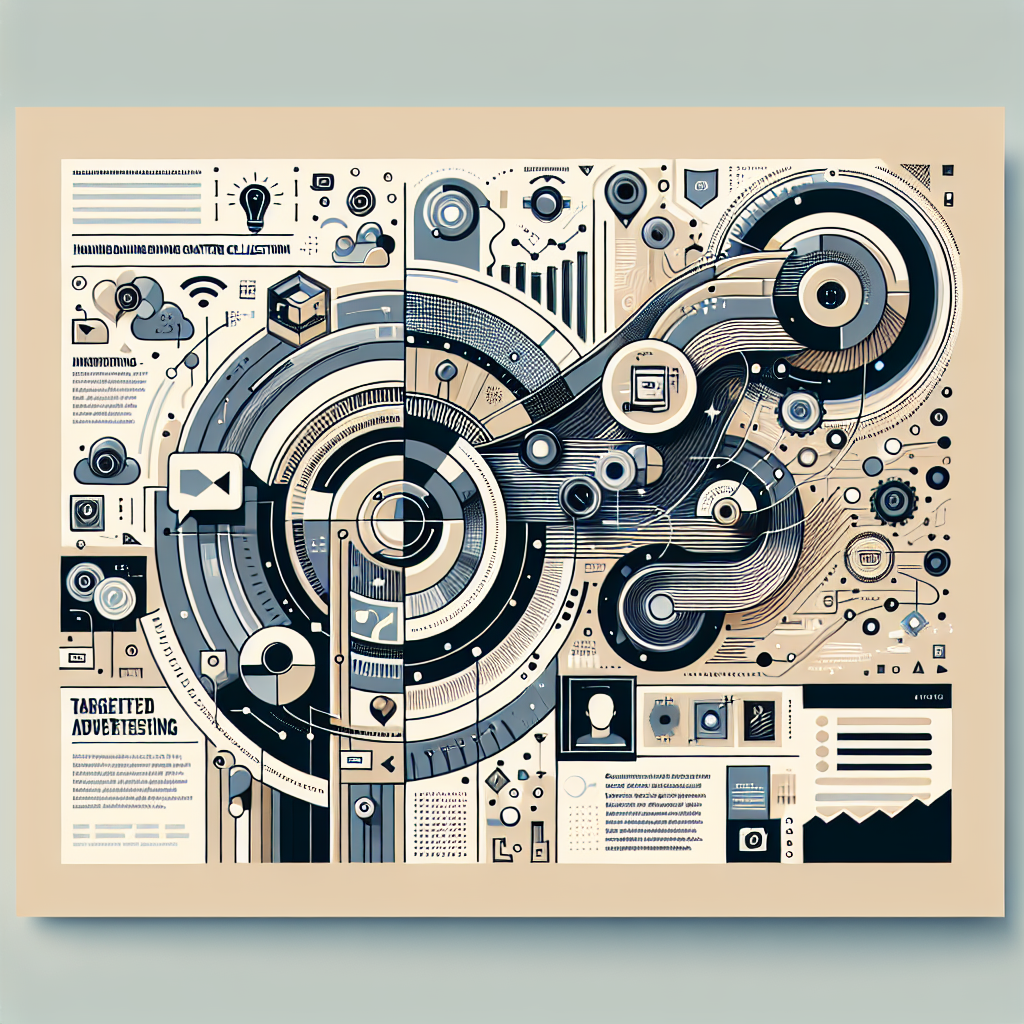
What Google's Privacy Sandbox Means for Marketers
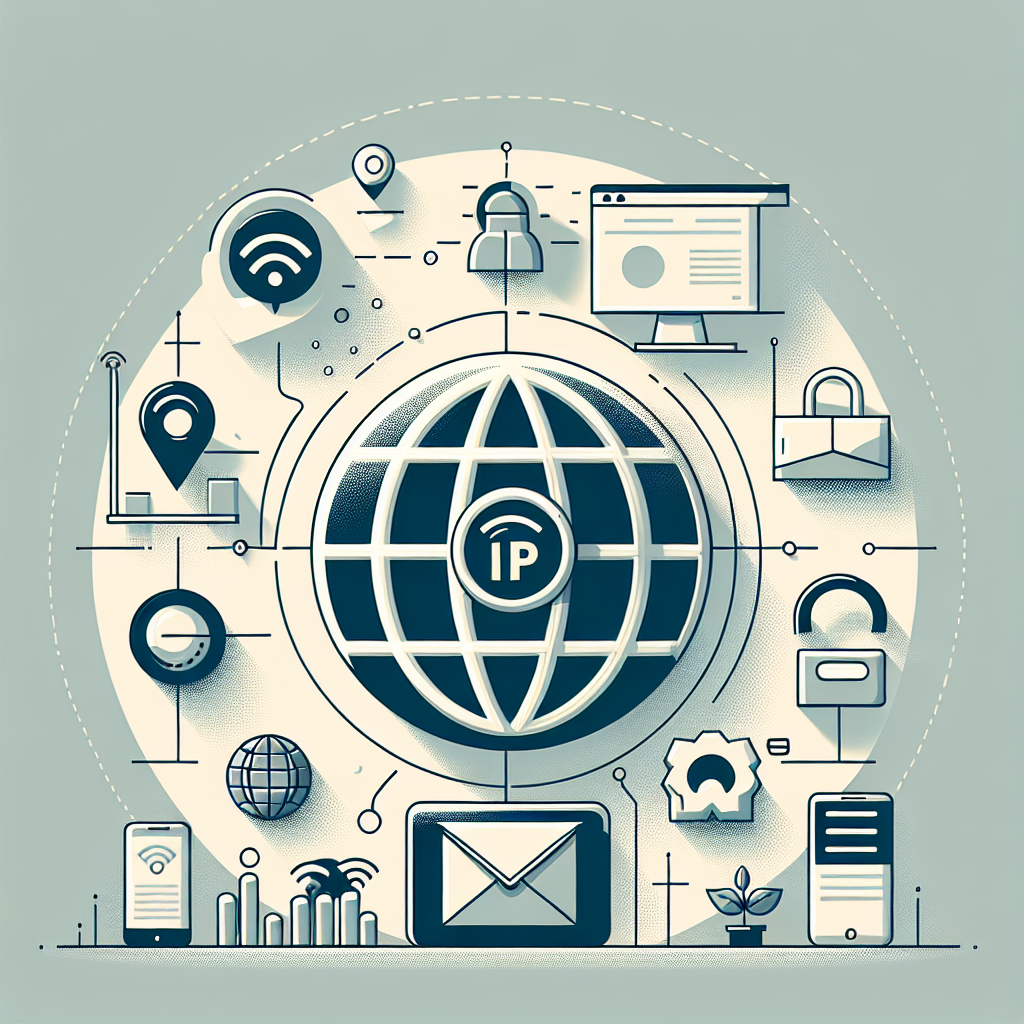
The Role of IP-Based Targeting in a Post-Cookie World
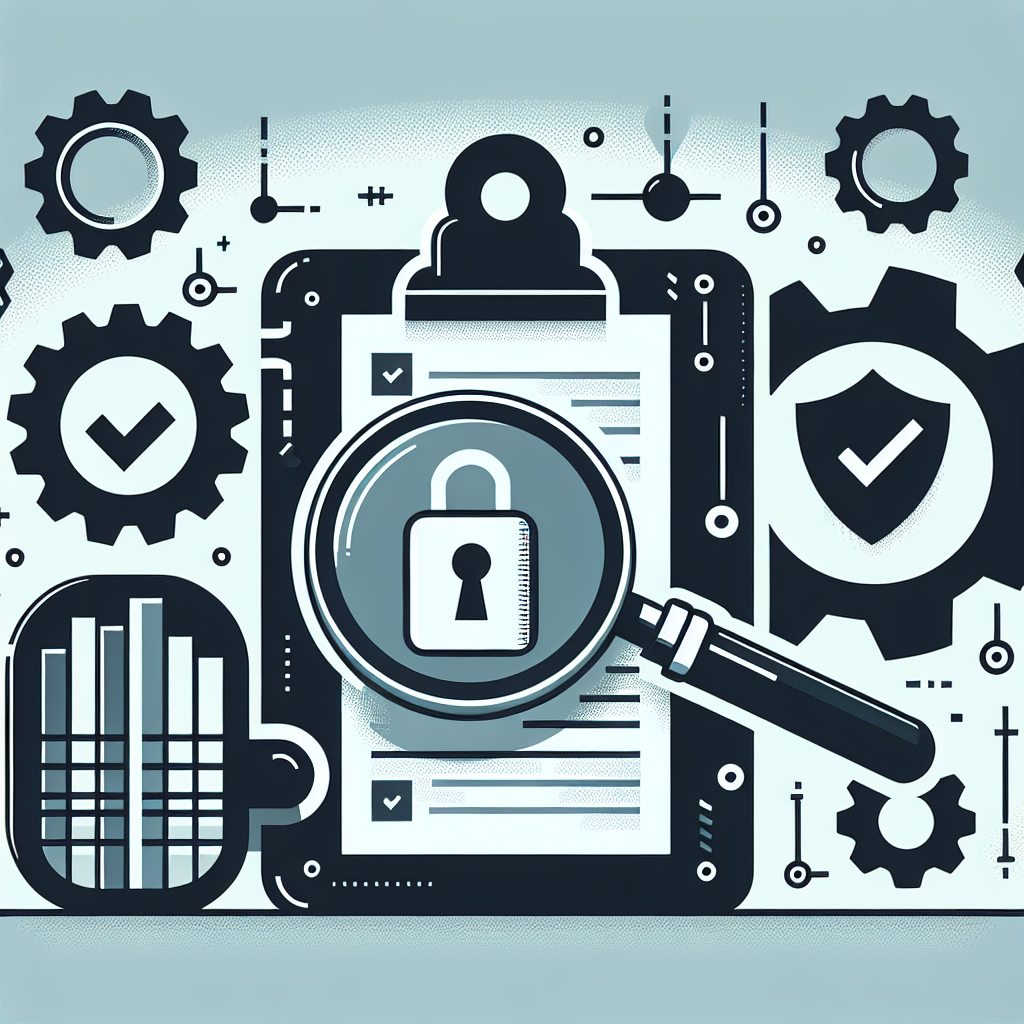