The Role of AI in Predicting Subscription Churn & Customer Behavior
The revelation came during Navya's quarterly review meeting. As the subscription analytics lead at a streaming media company, she had been tasked with explaining why their year-over-year churn had suddenly spiked by 3.2% despite increased content spending. While they had sophisticated dashboards showing the exodus, they offered little insight into why subscribers were leaving or—more importantly—how to identify at-risk customers before they departed. "If only we could predict which customers were likely to cancel next month," their CEO lamented, "we could actually do something about it." That moment sparked Navya's obsession with predictive analytics and artificial intelligence in subscription businesses. She immersed herself in research, connected with data science leaders, and implemented their first AI-driven churn prediction model. Six months later, they had reduced churn by 18% through targeted interventions. The experience transformed Navya’s understanding of how AI could revolutionize subscription businesses by making the future visible and actionable. What began as a professional necessity became a passion for understanding the powerful intersection of behavioral data, predictive modeling, and customer retention.
Introduction: The Critical Importance of Churn Prediction in Subscription Economies
In subscription business models, customer retention is the primary driver of profitability. Research from Bain & Company demonstrates that a 5% increase in customer retention can increase profits by 25-95%, while the cost of acquiring new customers typically ranges from 5-25 times more than retaining existing ones. Yet despite this economic reality, subscription businesses have historically relied on reactive approaches to churn—responding only after customers have already canceled.
Artificial intelligence and machine learning are fundamentally transforming this paradigm by enabling predictive approaches to churn management. By analyzing vast quantities of behavioral, transactional, and engagement data, AI models can identify subtle patterns invisible to human analysts and forecast individual customer churn probability with remarkable accuracy. According to research from the Subscription Trade Association, companies implementing AI-driven churn prediction achieve churn reductions of 15-30% on average, representing millions in retained revenue.
As subscription economy expert Tien Tzuo notes, "In the subscription economy, the ability to predict and prevent churn isn't just a competitive advantage—it's the difference between thriving and merely surviving." This article explores how AI is revolutionizing churn prediction and customer behavior analysis in subscription businesses.
1. The Evolution of Churn Prediction: From Rules to AI
Churn prediction approaches have evolved through three distinct generations:
- Rules-based systems (1st generation): Simple heuristics based on obvious warning signs like declining usage or payment failures.
- Statistical modeling (2nd generation): Regression models and statistical approaches that identify correlations between variables and churn probability.
- AI and machine learning (3rd generation): Advanced algorithms that detect complex patterns and non-linear relationships while continuously improving predictive accuracy.
This evolution represents a shift from intuition-based to data-driven approaches, with each generation offering significant improvements in predictive accuracy. According to McKinsey research, advanced AI models improve churn prediction accuracy by 30-50% compared to traditional statistical methods, enabling much more targeted and cost-effective retention efforts.
Netflix exemplifies this evolution, having progressed from basic engagement metrics to sophisticated machine learning models that analyze over 500 variables per subscriber, including viewing patterns, browsing behavior, and device usage. As Netflix's Director of Data Science commented in a recent interview, "Our AI doesn't just tell us who might leave—it tells us why, when, and what specific intervention might work for that individual subscriber."
2. Key AI Approaches Driving Predictive Accuracy
Several AI approaches have proven particularly effective for subscription churn prediction:
- Gradient boosting machines: Ensemble algorithms like XGBoost and LightGBM excel at handling the diverse data types common in subscription businesses, improving prediction accuracy by 15-20% over single models.
- Deep learning neural networks: Particularly effective for identifying complex patterns in unstructured data (e.g., customer service interactions, product usage patterns).
- Survival analysis models: Time-based approaches that predict not just if a customer will churn, but when, enabling more precisely timed interventions.
- Natural language processing (NLP): Analyzes sentiment from customer communications, reviews, and support interactions to identify satisfaction issues before they lead to cancellation.
Spotify leverages these approaches in its churn prediction suite, combining listening patterns, search behavior, playlist creation, and social sharing to identify distinct "pre-churn signatures" weeks before users cancel. According to Spotify's engineering blog, their ensemble AI approach achieves 81% accuracy in predicting churn 30 days in advance—a significant improvement over the 62% accuracy of their previous statistical models.
3. From Prediction to Prescription: How AI Enables Targeted Interventions
The true value of AI in churn prediction extends beyond identifying at-risk customers to determining which specific interventions will most effectively retain each one:
- Intervention optimization: AI models that predict which retention offers or actions will be most effective for specific customer segments or individuals.
- Optimal timing models: Algorithms that identify the ideal moment to intervene—neither too early (wasting incentives on customers who wouldn't have churned) nor too late (when customer decision to leave is irreversible).
- Dynamic incentive sizing: Models that calculate the minimum effective incentive based on customer lifetime value, churn risk, and behavioral indicators.
Dollar Shave Club exemplifies this approach, using their "Churn Reduction Engine" to match at-risk customers with one of seven distinct intervention types based on their specific churn indicators. According to their Chief Analytics Officer, this AI-driven approach "increased retention campaign ROI by 143% by ensuring we used the right message, offer, and timing for each individual subscriber."
Research from Forrester indicates that companies implementing both predictive (identifying who will churn) and prescriptive (determining optimal interventions) AI achieve 2.5x greater retention impact compared to those using prediction alone.
4. Behavioral Signals and Digital Body Language
The most sophisticated AI churn prediction models analyze subtle behavioral signals that constitute what digital behavior expert Paco Underhill calls "digital body language":
- Engagement decay patterns: Gradual reductions in session frequency, duration, or depth that precede churn by weeks or months.
- Feature abandonment: When users stop utilizing key platform features that historically drove their value perception.
- Reduced "exploration" behavior: Declining rates of discovering new content or features, often indicating diminishing interest.
- Social disconnection: Decreases in sharing, inviting, or connecting behaviors that indicate reduced social embedding in the service.
The New York Times subscription service tracks over 150 such behavioral signals, focusing particularly on reading depth, newsletter engagement, and comment section activity to predict subscriber satisfaction and churn risk. Their AI models identified that subscribers who read at least five articles per month across three different sections have 60% lower churn rates—a non-obvious insight that has shaped both their retention efforts and content strategy.
5. The Future of AI-Driven Subscription Management
Several emerging developments promise to further revolutionize AI's role in subscription churn prediction:
- Causal AI models: Moving beyond correlation to identify the actual causes of churn, enabling more effective strategic interventions.
- Real-time adaptive systems: Continuous learning models that update churn risk scores with each customer interaction, enabling dynamic intervention adjustments.
- Predictive experience personalization: AI systems that preemptively modify the customer experience based on satisfaction predictors before churn risk even develops.
- Emotion AI: Advanced sentiment analysis that detects emotional states from text, voice, and even usage patterns to identify frustration or dissatisfaction.
As Harvard Business School professor and AI expert Karim Lakhani observes, "The future of subscription businesses lies in models that don't just predict churn but actively prevent it through continuous experience optimization."
Conclusion: Turning Prediction into Retention
The integration of artificial intelligence into subscription churn prediction represents a fundamental shift from reactive to proactive customer management. By analyzing complex behavioral patterns, identifying subtle warning signs, and prescribing targeted interventions, AI enables subscription businesses to address retention challenges before they manifest as cancellations.
The most successful implementations share common elements: comprehensive data infrastructure, cross-functional integration between data science and customer experience teams, and closed-loop systems that continuously learn from intervention outcomes. Companies that master these capabilities achieve what subscription economy analyst John Warrillow calls "the retention advantage"—structurally lower churn rates that compound into significant competitive advantage over time.
As AI capabilities continue to advance, the gap between companies with sophisticated predictive capabilities and those without will likely widen, making AI-driven churn prediction not merely a competitive advantage but a business necessity in the subscription economy.
Call to Action
For subscription business leaders looking to harness AI for churn prediction:
- Audit your customer data infrastructure to ensure you're capturing the behavioral signals most predictive of satisfaction and churn
- Invest in cross-functional teams that combine data science expertise with customer experience design capabilities
- Implement A/B testing frameworks to measure intervention effectiveness and create feedback loops for your predictive models
- Start with focused applications that target high-value customer segments before scaling to your entire subscriber base
The organizations that execute on these priorities will not just predict the future of their customer relationships—they will actively shape it, achieving the sustained retention advantage that drives subscription business success.
Featured Blogs
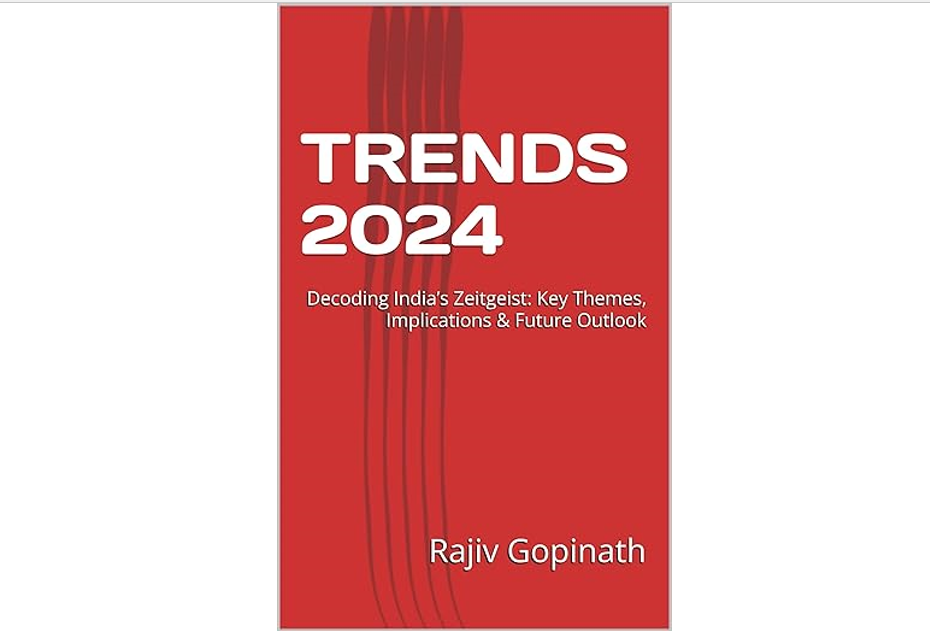
TRENDS 2024: Decoding India’s Zeitgeist: Key Themes, Implications & Future Outlook
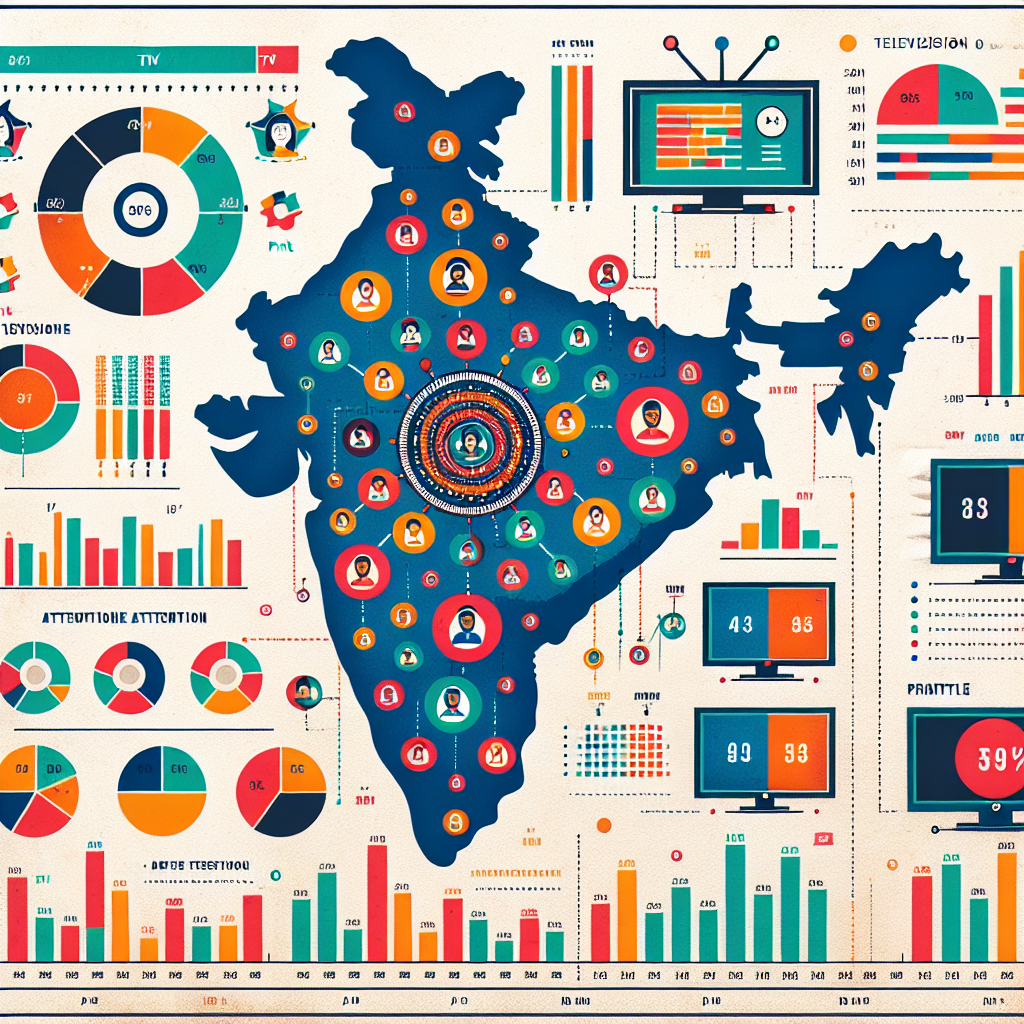
How to better quantify attention in TV and Print in India
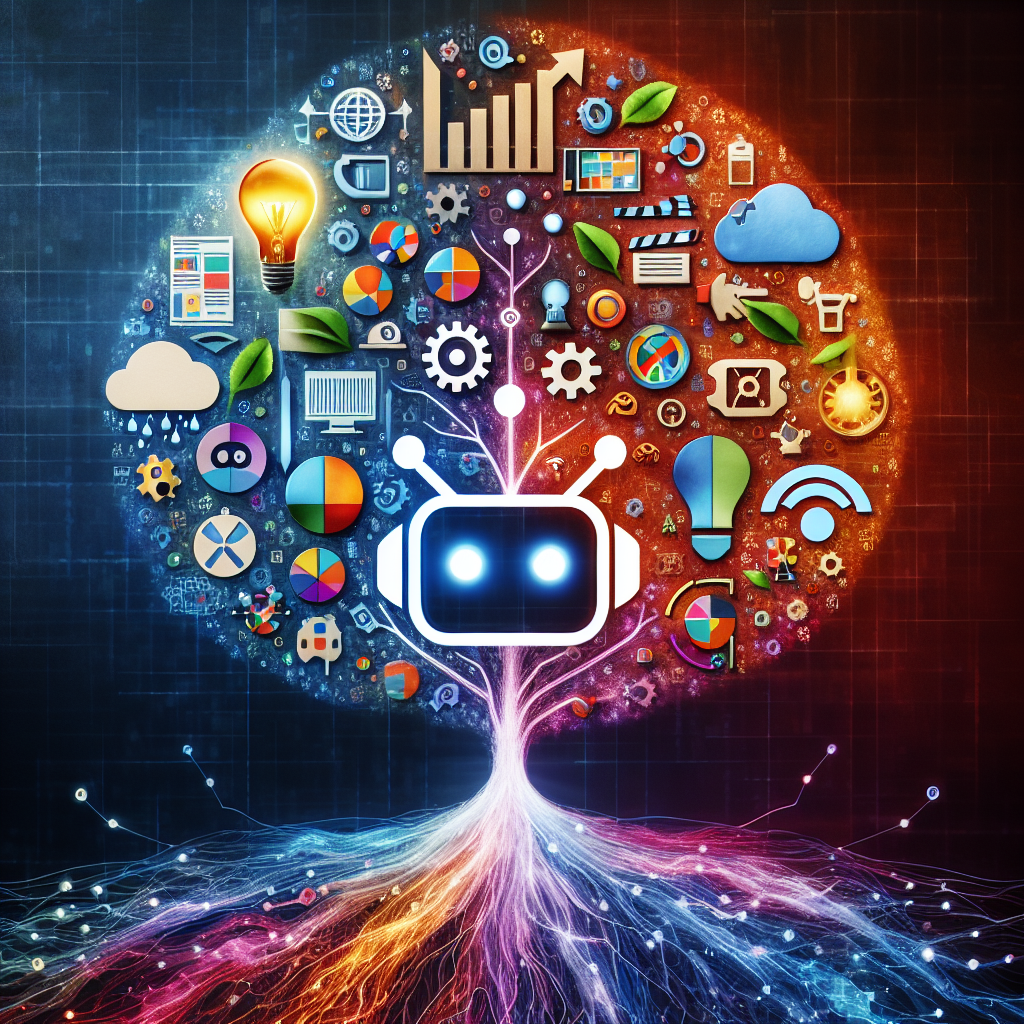
AI in media agencies: Transforming data into actionable insights for strategic growth
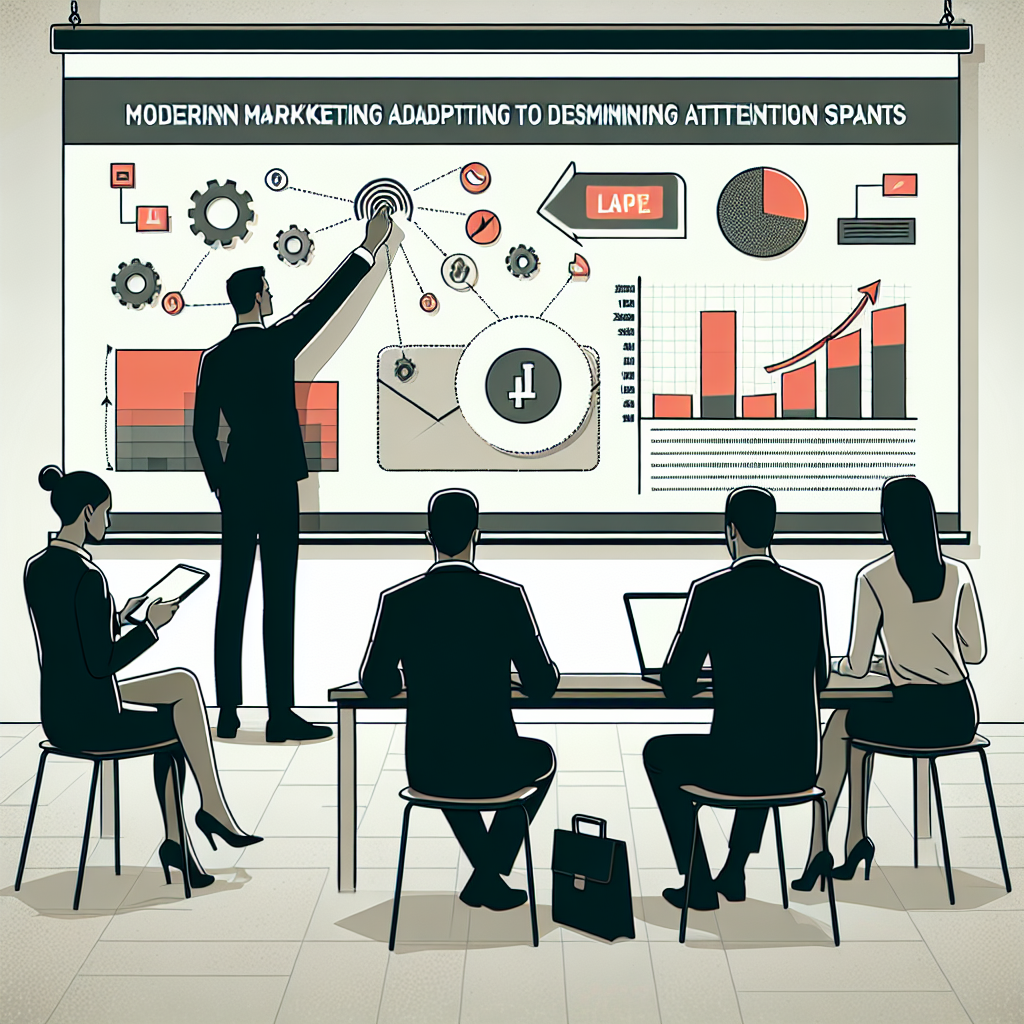
How the Attention Recession Is Changing Marketing
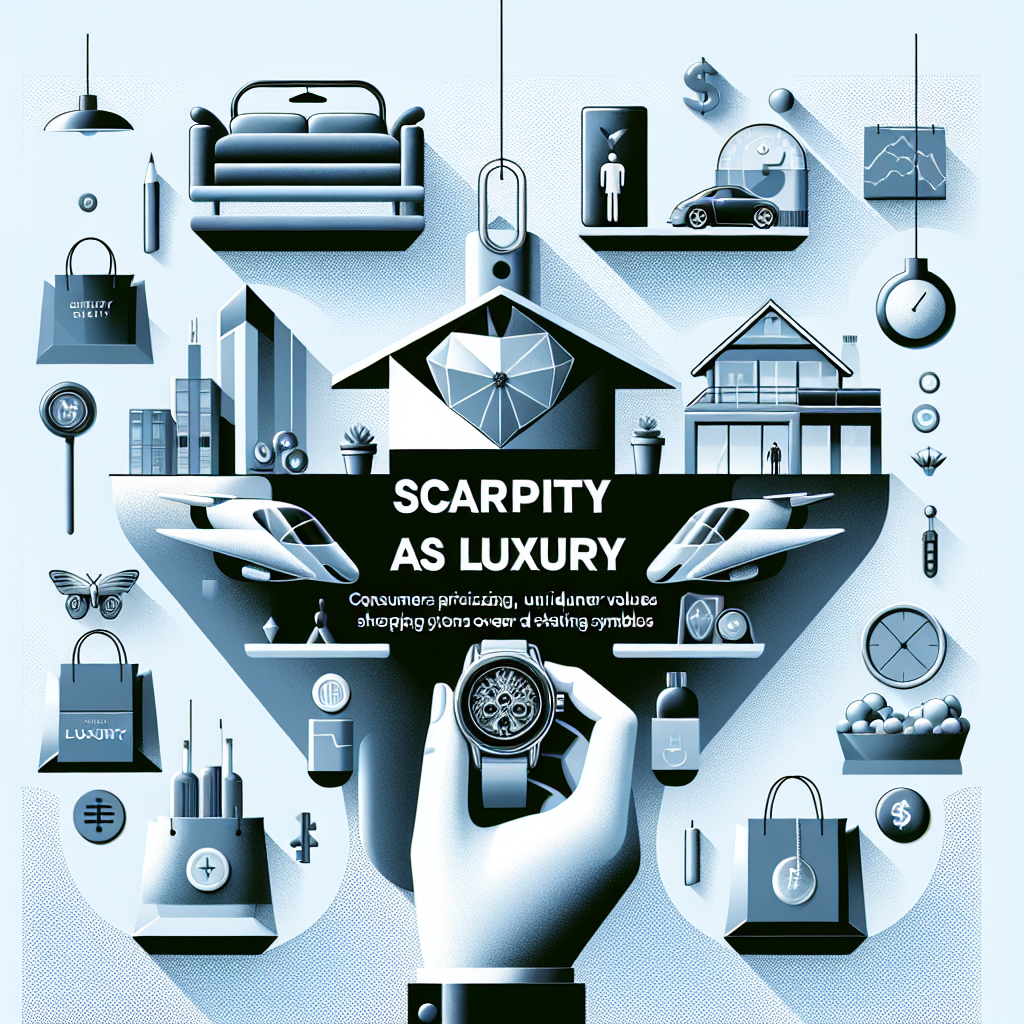
The New Luxury Why Consumers Now Value Scarcity Over Status
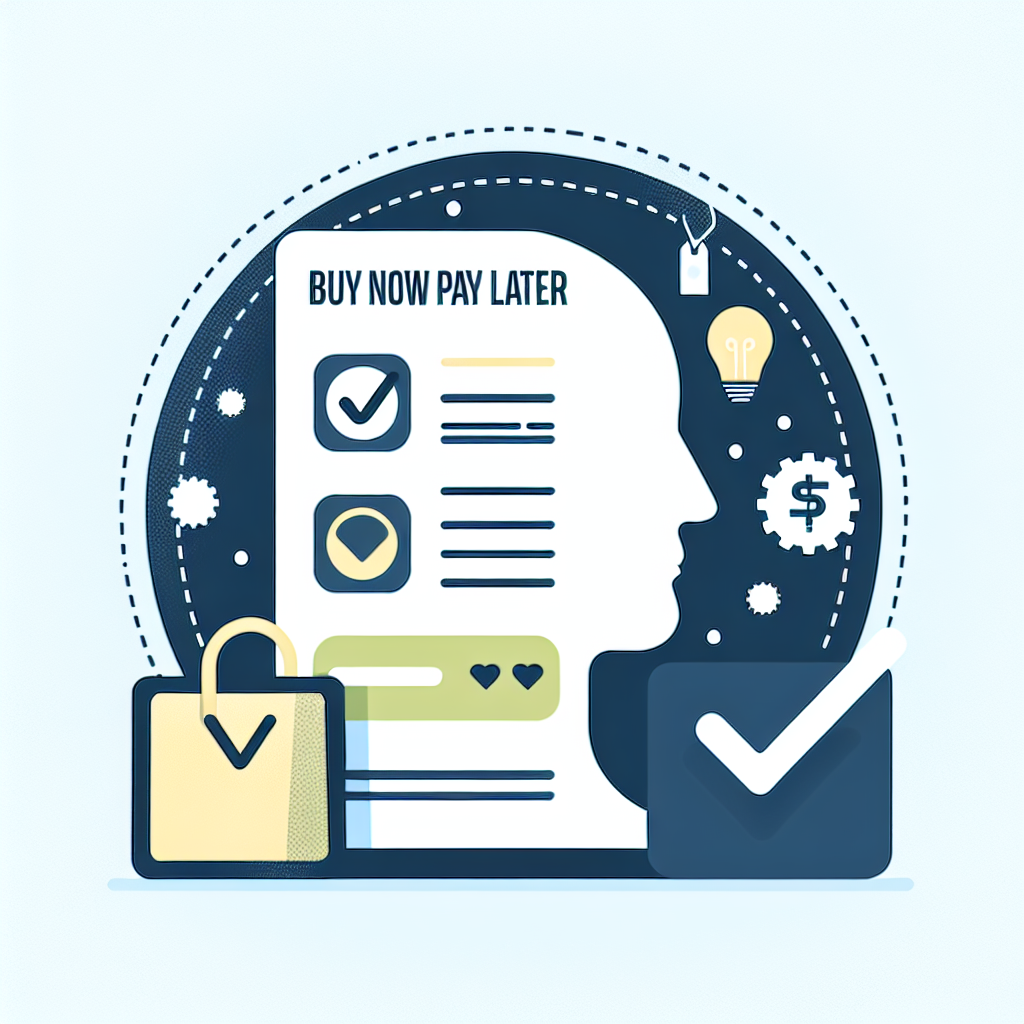
The Psychology Behind Buy Now Pay later
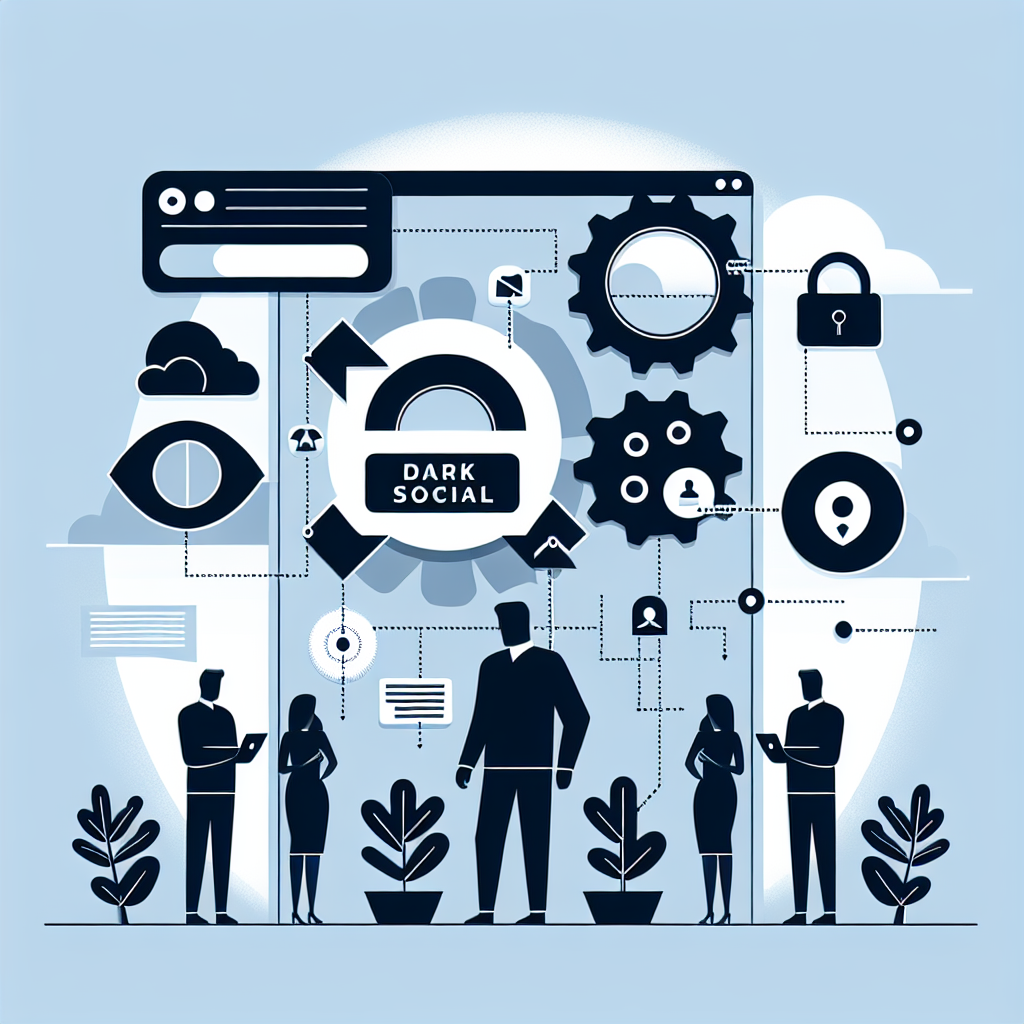
The Rise of Dark Social and Its Impact on Marketing Measurement
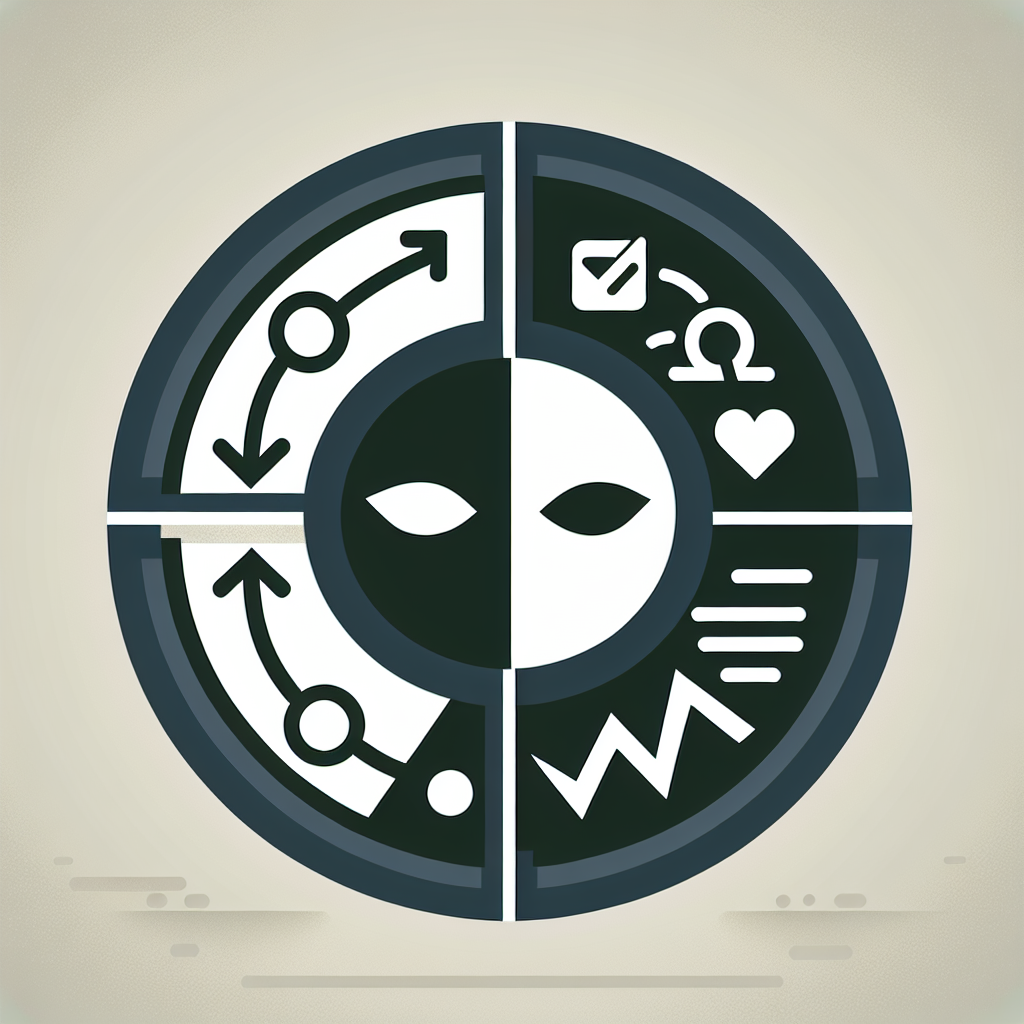
The Role of Dark Patterns in Digital Marketing and Ethical Concerns
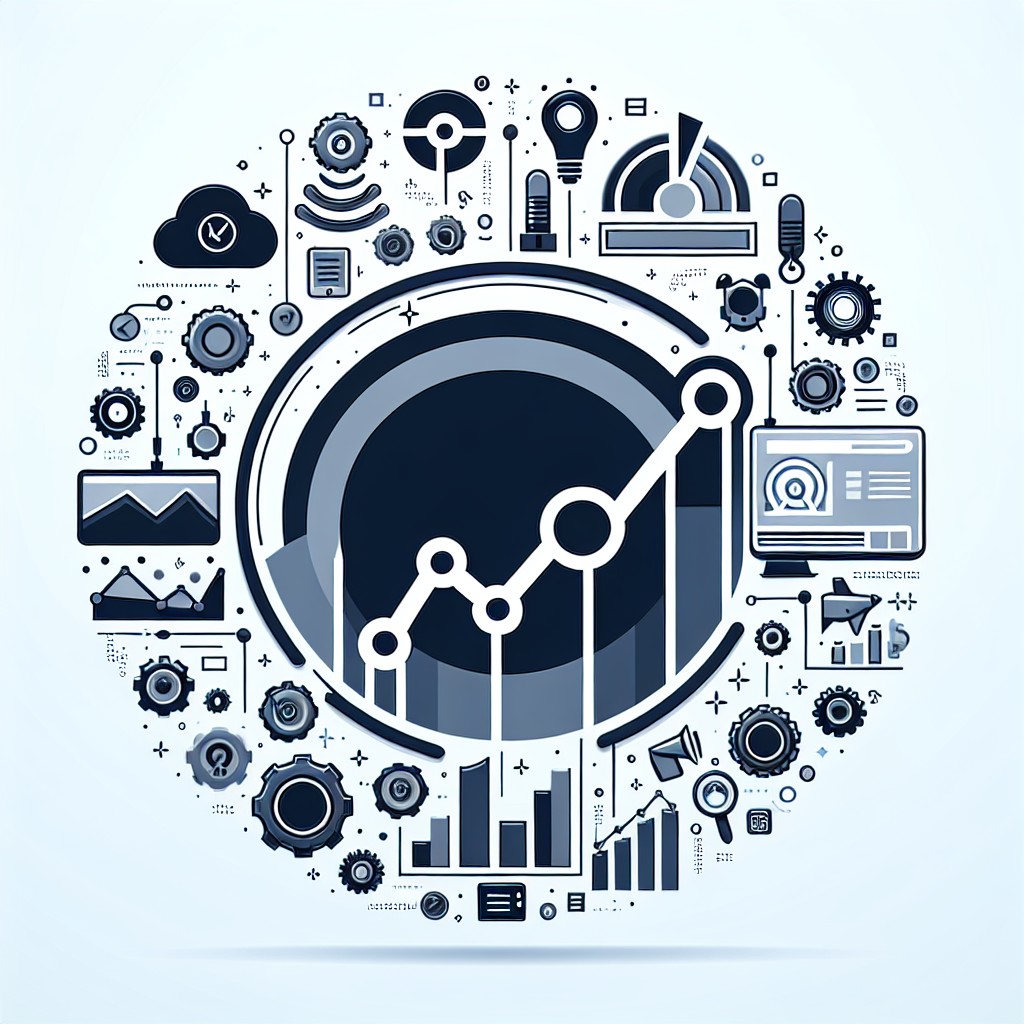